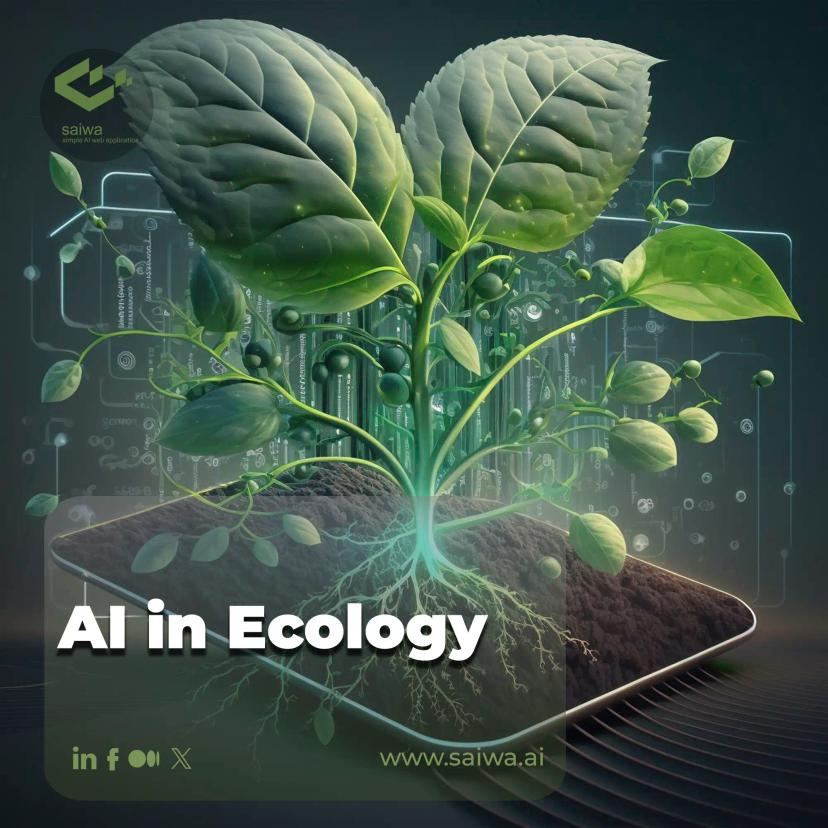
AI in Ecology | Expanding Ecology's Vision with AI
Understanding and preserving the Earth's precious biodiversity is an immense responsibility. Nowhere is this more evident than in the plant kingdom, which forms the foundation of terrestrial ecosystems. But ecology faces the challenge of studying plants at vast scales while managing humanity's growing agricultural footprint. This is where artificial intelligence (AI) holds great promise.
In this article, we explore emerging techniques of AI and machine learning in ecology that are enabling new ecological capabilities and knowledge specifically related to plant communities and agricultural systems. We survey applications in plant identification, agricultural monitoring, and climate change adaptation. The goal is to demonstrate how AI-driven intelligence, when thoughtfully applied, can advance both ecological science and environmental sustainability.
The Role of Ai in Ecology
AI in ecology techniques are enhancing many aspects of ecological data workflows:
- Automated Species Identification: Computer vision techniques can automatically identify species from all kinds of animals and plants from camera trap images to scale data collection.
- Environmental Condition Monitoring: Networks of AI-guided sensors adaptively monitor ecosystems in real-time, tracking dynamics.
- Satellite-Based Habitat Mapping: AI analysis of satellite imagery can map vegetation, species distributions, and land use at high resolutions over large areas to reveal spatial patterns.
- Ecological Data Mining: Machine learning data mining techniques help uncover hidden relationships and trends in massive ecological datasets that are impossible to discern manually.
- Literature Synthesis: AI text analysis can parse thousands of research papers to identify connections between findings a human can’t feasibly synthesize.
By augmenting data collection and mining insights from growing ecological data, AI enables scientific understanding at unprecedented scope and scale.
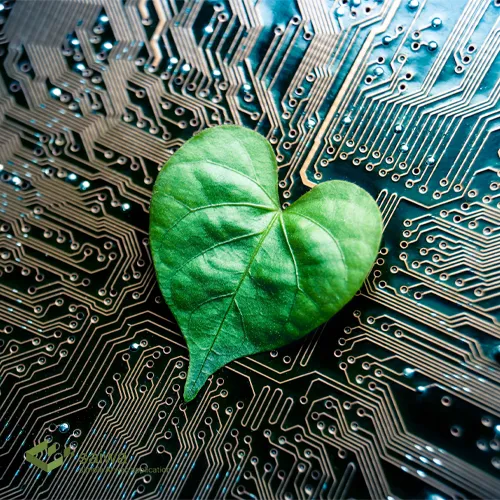
Understanding Plant Species with AI
Beyond identification, AI-driven data collection methods have transformed the way ecologists gather information about plant species. Remote sensing technologies equipped with AI algorithms allow for automated data collection, reducing the need for labor-intensive fieldwork. This innovation not only expedites data acquisition but also ensures a more comprehensive and continuous understanding of plant species distribution and behavior.
Agricultural Monitoring
Agriculture Monitoring is a subset of using AI in ecology and machine learning in agriculture. A major interface between plant ecology and humanity is agriculture. Here AI is revolutionizing monitoring capabilities and analytics:
Invasive Species Detection
UAVs equipped with deep learning vision models, such as drones, autonomously survey fields continually, identifying locations of invasive or herbicide-resistant weeds. This enables early intervention before they spread using precise, targeted spraying only where needed.
Crop Health Assessment
High-resolution aerial imagery captured via drones, planes or satellites is analyzed using AI to detect crop biotic and abiotic stresses. This allows pinpointing areas with nutrient deficiencies, drought stress, disease outbreaks, or pest damage for prompt mitigation.
Yield Estimation
Complex machine learning algorithms can continually integrate historical yield data, crop growth models based on the development stage, weather records, and real-time aerial imagery of fields to predict expected yields. This supports the optimization of harvest logistics and commodity transportation planning.
Workforce Multiplication
Camera-equipped robotic systems manually weed nursery rows without herbicide, guided by computer vision models identifying crop seedlings versus weeds. Other automated sensor platforms directed by AI accelerate tedious phenotyping processes like measuring crop traits. This expands agronomists’ capabilities.
Prescriptive Input Application
Drone imagery and ground-based sensors that track indicators such as canopy temperature and soil moisture are processed by AI models to determine the optimal amount of irrigation, fertilizer, or pesticide to apply variably across fields. This matches inputs to needs, preventing environmentally damaging over-application while maintaining yields.
Thoughtfully designed, such AI systems can increase agricultural productivity while minimizing environmental impact through continuous data-driven monitoring, analytics, and field automation. These technologies may prove essential to sustainably addressing global food security challenges.
Plant species Identification and Counting
A fundamental challenge in ecology is the cataloging of plant biodiversity. It is estimated that 10-20% of plant species remain undiscovered. As mentioned above, AI in ecology can help us identify these species. For example, machine learning is now speeding up species identification and plant counting or Counting Trees through automated image analysis. Key techniques include
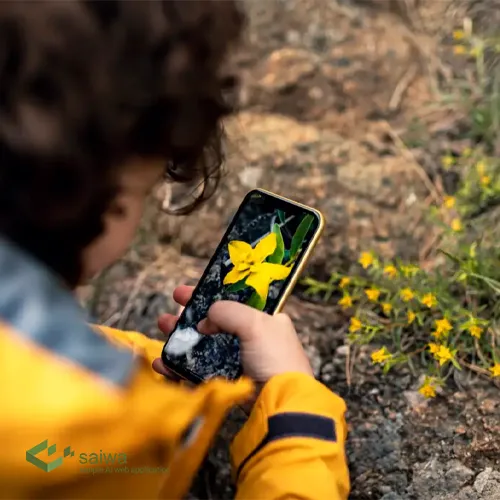
Image Classification
Convolutional neural networks classify species from herbarium scans or field images with accuracy rivalling human experts when trained on sufficient examples.
Multimodal Analysis
Combining images with plant chemical signatures, genetics, and geography improves identification accuracy, especially for closely related species.
Herbarium Digitization
Algorithms extract species labels, traits, and metadata from centuries of accumulated herbarium sheets to unlock their research value.
Smartphone Assisted Monitoring
Apps like PlantSnap allow hikers to snap geotagged photos identified by AI, crowdsourcing new species distribution data at scales impossible manually.
AI visual plant identification makes accessible the documentation of Earth’s plant diversity. It produces invaluable data for conservation planning and enables near real-time tracking of range shifts with climate change.
Optimizing Conservation Strategies and Policy
AI in ecology also helps strategically optimize ecological interventions and policies:
- Adaptive Management via Reinforcement Learning: Agents iteratively model different conservation interventions, receive ecosystem feedback, and determine optimal dynamic policies balancing tradeoffs.
- AI-Guided Sustainable Development Planning: Optimization algorithms determine development plans minimizing habitat loss by assessing tradeoffs with socioeconomic factors.
- Conservation Investment Prioritization: Algorithms integrate public health, ecological, social, and economic data to indicate where investments can best benefit communities and ecosystems.
- Dynamic Resource Allocation: Forest fire lookout towers equipped with AI assist dispatchers optimizing real-time decisions of where to steer resources as new blazes appear.
Climate Change Adaptation
The climate crisis poses grave risks to ecosystems. AI in ecology can help us out in many different aspects. Here too AI can help model and guide adaptation. Relevant techniques include:
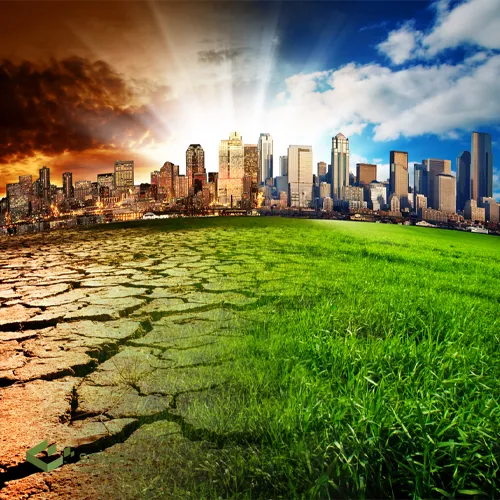
Species Distribution Modeling
By correlating environmental variables like temperature, rainfall, and soils with known species occurrences, AI can project shifting geographic ranges under climate change scenarios. This indicates where to target conservation.
Climate-Smart Cropping
AI systems integrate climate forecasts, crop growth models, and yield data to recommend ideal planting areas, cultivation timing, and crop varieties, given changing local conditions. This guides farmers’ adaptive decisions.
Assisted Migration
Algorithms help model which species declining in native ranges may persist if carefully introduced to new climatically suitable regions in a controlled manner. This indicates proactive conservation strategies.
Ecological Impact Simulation
Detailed AI in ecology models allows running some sorts of simulations of the effects of warming, land conversion, etc., on biodiversity to preview the effectiveness of potential interventions.
Leveraging these predictive capabilities aids strategic climate adaptation planning to protect biodiversity through uncertain changes ahead.
Biodiversity Assessment
Cataloging and sustaining biodiversity is central to ecological science. Here too AI shows high potential:
Species Distribution Mapping
By combining ground observations, remote sensing, and environmental data, ML models can map distributions of plant species, identifying hotspots of diversity.
Population Trend Estimation
Automatic analysis of repeated survey data determines trends of key plant populations over time. This focuses conservation efforts on at-risk species.
Plant Trait Characterization
Computer vision and spectroscopy linked with plant trait models allow rapidly assessing characteristics to elucidate ecological strategies.
Ecological Interaction Mapping
Computer vision tracking of activity like pollinator movements integrates with graph algorithms to map plant-pollinator networks, identifying critical symbiotic relationships.
Microbiome Analysis
High-throughput DNA sequencing combined with unsupervised learning categorizes plant microbiomes. These profiles plant-microbe associations and their functions.
AI advances provide unprecedented insights into biodiversity patterns, traits, trends and ecological interconnections at scales necessary to manage communities amid ongoing change.
Optimizing Agriculture Practices
At the intersection of ecology and agriculture, using AI in ecology fields such as agriculture also enhances production and environmental performance:
- Monitoring and Control Systems: Real-time sensor data on soil, water, and crop needs fed into AI models allows dynamically controlling irrigation, fertilizers and pesticides precisely when needed. This cuts waste while maintaining yields.
- Yield Prediction: Complex machine learning algorithms integrate genetics, management practices, and environmental conditions to forecast crop productivity. This facilitates planning.
- Supply Chain Optimization: AI scheduling and control coordinates seeding, watering, chemicals, and harvesting operations across farms for maximum efficiency. This simplifies operations.
- Soil Management: ML techniques prescribe precise soil amendments tailored to soil types and crop needs based on analysis of soil chemistry. This nurtures soil health.
- Decision Support Systems: Easy-to-use apps provide farmers real-time AI-generated recommendations on best practices as conditions change to improve economic and environmental performance.
Thoughtfully implemented, AI can achieve the win-win of increased agricultural productivity with minimized environmental impacts - an essential balance for global food security.
Ecological Modeling With AI
Finally, in leveraging AI in ecology field, machine learning shows promise for modeling complex ecological phenomena:
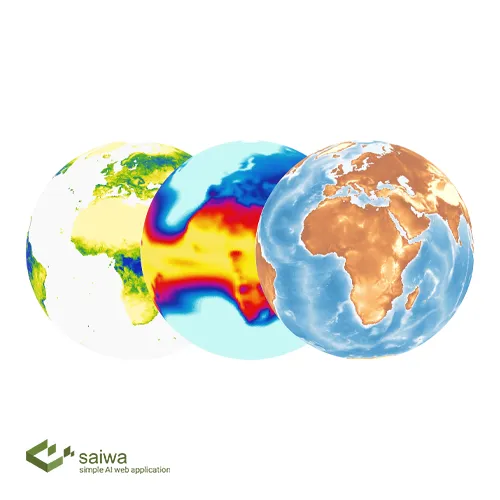
Plant-Soil Interactions
Advanced neural networks help decipher highly multivariate relationships between plants, microbes, nutrients, and soils to clarify below-ground mechanisms.
Microbiome Analysis
Powerful unsupervised learning categorizes plant microbial symbionts and their functions from massive DNA sequencing datasets to elucidate the plant holobiont.
Ecosystem Simulation
Detailed agent-based models approximate complex dynamics between organisms, resources, climate, and landscapes over time-based on machine learning insights.
Biodiversity-Function Relationships
Algorithms reveal multivariate associations between taxonomic, functional, and genetic diversity and ecosystem processes like biomass production or nutrient cycling.
These techniques provide key insights into the intricate workings of natural systems for predictive ecology.
ChatGPT-4 and the future of environmental monitoring: Ai-driven ecosystem assessment and protection
Due to new developments in artificial intelligence technology, the future of environmental monitoring will be much better than before. The development of ChatGPT-4, an Ai-driven ecosystem assessment and protection, is going to revolutionize the way we monitor and protect our environment. With its ability to analyze large amounts of data and make accurate predictions, ChatGPT-4 is poised to become a valuable resource for environmental scientists, policymakers, and conservationists.
One of the most important challenges of environmental monitoring is the large amount of data that must be collected, analyzed and interpreted. Traditional methods usually rely on manual data collection and analysis, which can be time-consuming, intensive, and prone to human error. With the advent of ChatGPT-4, these limitations and challenges are quickly becoming a thing of the past. This tool, which is based on artificial intelligence, can process a large amount of data in real time and provide scientists with accurate and new information about various environmental parameters.
ChatGPT-4 can also analyze data from multiple sources such as satellite images, weather data and social media feeds to create a comprehensive picture of the current state of the environment. This ability to combine information from different sources allows for a more comprehensive understanding of the ecosystem, which is very important for effective environmental monitoring and protection.
In addition to data analysis capabilities, ChatGPT-4 can make significant predictions. By using machine learning algorithms, artificial intelligence can identify patterns and trends in data and make accurate predictions about future environmental conditions. This foresight is of great value to policymakers and conservationists because it enables them to make informed decisions about resource allocation and conservation measures.
For example, the predictive capabilities of ChatGPT-4 can be used to identify areas at high risk of deforestation, giving conservationists the ability to prioritize their efforts and allocate resources effectively. Artificial intelligence can predict the impact of climate change on different ecosystems and gives policymakers the ability to create targeted strategies to reduce the effects of global warming.
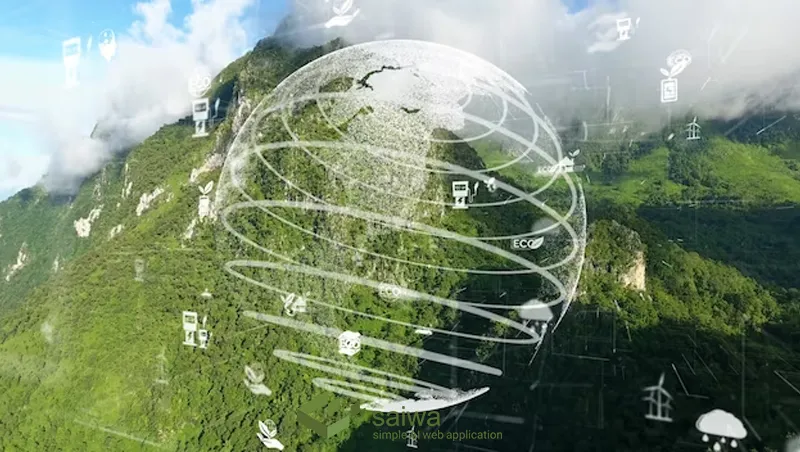
How can ecology inspire artificial intelligence and vice versa?
Most of today's artificial intelligence systems are almost modeled after the human brain. Now researchers suggest that another branch of biology and ecology could inspire a new generation of artificial intelligence ecology to be stronger, more flexible, and more responsible. Artificial intelligence ecology can help us get real knowledge from vast data without having to go through all the usual steps. In addition to helping scientists in various fields, artificial intelligence can lead to progress for us through the principles of ecology.
Of course, there is the idea that artificial intelligence ecology can be very good at some tasks while still not useful at others and that the development of artificial intelligence runs into obstacles that environmental principles can help overcome.
The types of problems that we regularly deal with in the environment are challenges that artificial intelligence can take advantage of in terms of pure innovation. Also, these problems are things that if artificial intelligence ecology can help, it will be of great benefit to the world and useful to humanity as well.
Many ecologists use artificial intelligence to search for patterns in large data sets and make more accurate predictions. However, new research claims that there are many more possibilities for using artificial intelligence in the environment, such as in synthesizing big data and finding missing links in complex systems.
Scientists usually try to understand the world by comparing two variables at a time, for example, how population density affects the number of cases of an infectious disease. But the problem is that, like most complex ecological systems, disease prediction depends on many variables. Ecologists are not always aware of what these variables are. They are also limited to physical factors that can be easily measured, unlike social and cultural factors.
Compared to other statistical models, AI can accommodate larger amounts of data and diverse data sources, and this may help us discover new interactions and stimuli that we might not think are important at all.
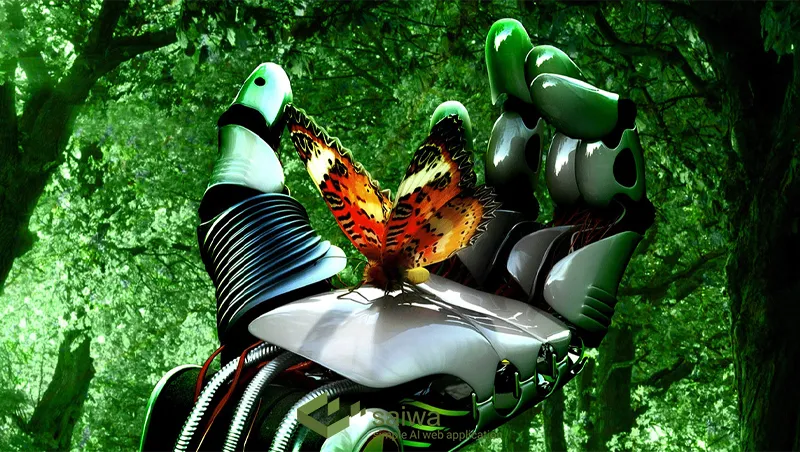
How AI can help save endangered species?
AI support ecosystem presents a powerful tool in the efforts to conserve and protect endangered species. Its applications span across various domains, contributing to more effective monitoring, management, and conservation strategies. Here's a glimpse into how AI support ecosystem can play a crucial role in saving endangered species:
- Habitat Monitoring: AI-powered satellite imagery and remote sensing technologies enable real-time monitoring of habitats. Machine learning algorithms can analyze vast amounts of data, detecting changes in land use, deforestation, and habitat degradation. This information is invaluable for conservationists to identify areas at risk and implement timely interventions.
- Wildlife Tracking and Identification: AI facilitates advanced tracking and identification of endangered species. Camera traps and sensor networks equipped with AI algorithms can accurately identify individual animals, track their movements, and assess population dynamics. This data aids in understanding migration patterns, habitat preferences, and population health, essential for targeted conservation efforts.
- Anti-Poaching Initiatives: AI contributes significantly to anti-poaching efforts. Smart sensors, drones, and intelligent camera systems can detect and alert authorities to potential poaching activities in real time. Predictive modeling using AI can help predict high-risk areas for poaching, allowing for proactive measures to safeguard vulnerable species.
- Conservation Genetics: AI is instrumental in analyzing vast datasets in the field of conservation genetics. It aids researchers in studying the genetic diversity of endangered populations, identifying key genes for adaptation, and making informed decisions about breeding programs to enhance genetic resilience.
- Ecological Modeling: AI-driven ecological modeling helps scientists simulate and understand complex ecosystems. By analyzing environmental variables and species interactions, AI can predict the impacts of climate change, human activities, and other factors on endangered species. This foresight allows for adaptive conservation strategies.
- Public Awareness and Engagement: AI can be utilized to raise public awareness and engagement in conservation efforts. Social media analytics and AI-driven campaigns help organizations tailor their messaging to specific audiences, fostering a sense of responsibility and encouraging public support for endangered species conservation.
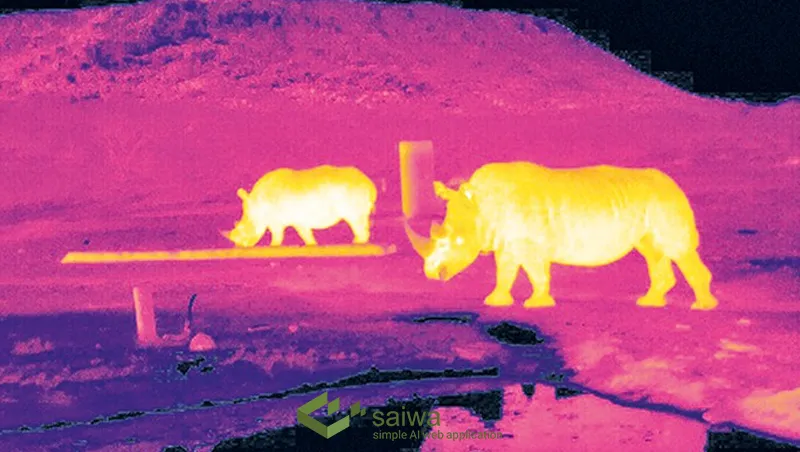
Ethical Considerations and Challenges for Ai in Ecology
As the integration of AI in ecology research and conservation efforts continues to expand, it is crucial to address the ethical considerations and potential challenges that arise. Ensuring the responsible and equitable use of these powerful technologies is paramount to safeguarding the integrity of ecological systems and protecting the well-being of all stakeholders involved.
Privacy and data security concerns are among the most pressing ethical issues surrounding the use of AI in ecology. The collection and analysis of vast amounts of ecological data, including sensitive information about species distributions, habitats, and environmental conditions, raise legitimate concerns about data privacy and the potential misuse of this information. Stringent measures must be implemented to ensure the secure handling and storage of data, as well as strict access controls and data governance policies.
Collaboration and Interdisciplinary Approaches
The successful application of AI in ecology demands collaboration and interdisciplinary approaches that bridge the gap between different domains and areas of expertise. Fostering collaboration between ecologists, computer scientists, AI experts, and other relevant stakeholders is essential for unlocking the full potential of AI in ecological research and conservation efforts.
Interdisciplinary research teams and projects that bring together diverse perspectives and skills are crucial for developing robust and effective AI solutions tailored to ecological challenges. By combining the domain knowledge of ecologists with the technical expertise of computer scientists and AI specialists, these collaborative efforts can yield innovative approaches that leverage the strengths of each discipline.
Establishing platforms and mechanisms for knowledge sharing, data exchange, and resource pooling across disciplines is also vital. Open access to ecological data, AI models, and computational resources can accelerate research and development efforts, while fostering a culture of transparency and collaboration within the scientific community.
The Future of AI in Ecology
Looking ahead, we can expect AI to take an increasing role across the full spectrum of ecological research and conservation:
- Ever-Expanding Applications: As algorithms, computational power, and ecological data grow, so will AI's capabilities to reveal insights.
- Closer Collaboration Between Domains: Ecologists partnering with data scientists and AI experts will become the standard for project success.
- Development of Best Practices: Experience and responsible innovation will produce guidelines and principles for effectively leveraging AI's strengths while mitigating risks.
- Focus on Creating Hybrid Intelligence: AI in ecology will increasingly become thinking partners amplifying human abilities rather than aiming to replace them.
Overall, thoughtfully designed and empirically validated AI in ecology technologies promise to provide ecology with unprecedented predictive sight and the ability to model the intricacies of nature. This emerging partnership between researchers and machines will deepen ecological understanding to steward ecosystems on an ever more crowded planet sustainably.
Conclusion
Earth's miraculous plant diversity provides humanity with indispensable benefits as well as inherent wonders worthy of preservation. Yet plants are understudied and increasingly threatened. AI in ecology using UAVs offers unprecedented potential to accelerate plant research, improve conservation, optimize agriculture, and model ecological complexity at needed scales. However, appropriate caution and deliberation must be exercised when using nascent technologies to guide real-world conservation decisions. Overall, this assessment shows that thoughtfully designed AI technologies hold the promise of enhancing our collective ability to conserve Earth's irreplaceable plant heritage amidst the uncertainties that lie ahead. However, fully realizing this potential will require cross-disciplinary collaboration, empirical rigor, and a commitment to the equitable advancement of all global communities.