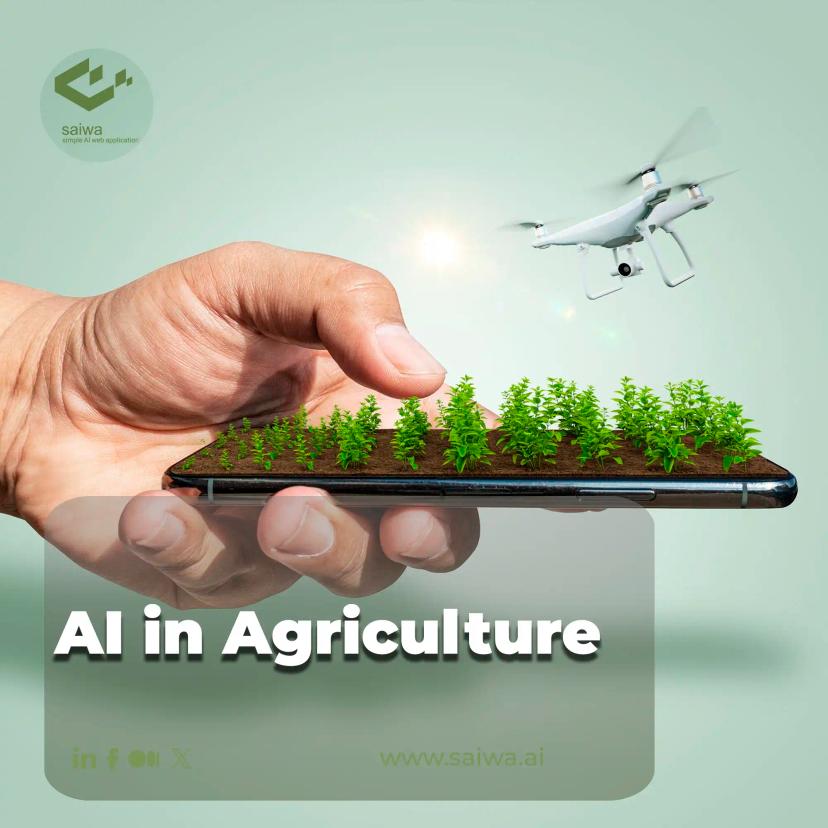
Practical Application & Future of AI in Agriculture
Agriculture is one of the oldest human activities. It provides food, fiber, and fuel for billions of people worldwide. But agriculture is not easy. It faces many challenges, including climate change, population growth, environmental degradation, and resource scarcity. Agriculture must adopt innovative technologies and practices to meet these challenges and ensure food security and sustainability. One of the most promising technologies is artificial intelligence (AI), which can help farmers optimize their operations, increase their productivity and profitability, and reduce their environmental impact. In this blog, we will explore some of the practical applications of AI in Agriculture and how AI is transforming the agriculture industry.
Read Also: The impact of machine learning in agriculture
How Was AI Used in Agriculture in the First Place?
The high-tech robots and self-driving tractors of modern agriculture might surprise you, but the roots of AI used in agriculture are humbler. Imagine a time before PCs, when cutting-edge technology filled entire rooms. That's what AI used in agriculture was in the 1960s and 70s – a complex system programmed with agricultural data.
These pioneering applications were called expert systems. Think of them as the original digital farm advisors, capturing the knowledge of seasoned farmers and researchers. Encoded with data on soil composition, historical yields, and pest management, these early applications of AI used in agriculture offered guidance to farmers facing new challenges.
For example, a 1970s farmer with declining corn yields could consult an AI used in agriculture system with historical farm data like soil makeup, weather patterns, and past pest outbreaks. The system would analyze the situation, suggesting potential causes like a new disease or nutrient deficiency. While lacking modern processing power, these early AI used in agriculture systems were revolutionary. Farmers could access a vast repository of agricultural knowledge, enabling data-driven decisions about their crops.
The 1980s saw a significant milestone with AI used in agriculture. In 1985, McKinion and Lemmon developed GOSSYM, a groundbreaking cotton crop simulation model. This model harnessed the power of expert systems to optimize cotton production by considering factors like irrigation, fertilization, and even climate. GOSSYM paved the way for more sophisticated AI applications in agriculture that led to today's precision agriculture techniques.
The story of AI used in agriculture is one of continuous innovation, transforming how we grow food. As technology advances, the future of agriculture promises to be even more efficient, sustainable, and productive.
How artificial intelligence is revolutionizing the agriculture industry?
AI projects in agriculture are transforming the sector by providing creative answers to urgent problems including resource constraints, climate change, and rising food consumption. AI is essential in converting conventional farming methods into intelligent, data-driven operations that help farmers make wise choices and streamline agricultural procedures.
The decrease of the effects of climate change is an important field in which AI projects in agriculture are having significant effects. Artificial intelligence (AI) systems use advanced algorithms to assess enormous volumes of data, like past weather trends, crop performance, and soil conditions. These systems can offer important insights on climate-related risks such as extreme weather occurrences, droughts, and temperature swings, by spotting trends and patterns. Equipped with this expertise, farmers can put adaptable plans into place to reduce crop losses and guarantee that their agricultural enterprises remain resilient in the face of shifting environmental circumstances.
Furthermore, by making the best use of scarce resources like water, land, and fertilizers, AI initiatives in agriculture are assisting in addressing the issue of resource scarcity. AI systems examine data from sensors, drones, and satellite photography to generate comprehensive maps of fields that highlight areas with particular moisture or nutrient deficiencies using methods like precision agriculture. Farmers may optimize crop yields while reducing waste and environmental effects by carefully regulating inputs like fertilization and irrigation.
By tackling issues with climate change, resource scarcity, and food demand, AI projects in agriculture are causing a revolutionary change in the sector. Farmers can enhance agricultural methods, increase productivity, and guarantee the long-term sustainability of food production systems by utilizing AI algorithms and data analytics.
Read Also: The Role of Data Science in Agriculture
Practical Application of AI in Agriculture
AI technology has revolutionized traditional farming practices, enabling more efficient and sustainable farming methods. In this section, we will explore the practical applications of AI in agriculture and how it is reshaping how we grow crops.
Monitoring Crops and Soil Health
One of the most important applications of AI in agriculture is crop and soil monitoring. AI can help farmers collect and analyze data from multiple sources, such as satellites, drones, sensors, and cameras, to monitor their crops and soil's health and condition. This is one of the most important examples of AI transforming agriculture. AI can also use machine learning and computer vision to identify weeds, pests, diseases, nutrient deficiencies, water stress, and other issues that affect crop growth. Farmers receive high-quality recommendations for irrigation, fertilization, and pest control using these new technologies. AI increases yields and minimizes environmental impacts by optimizing soil conditions and reducing resource waste.
Observing crop maturity
Observing and assessing crop maturity is a big deal for farmers. Finding the perfect time to harvest is an important factor in maximizing yield and quality. AI image recognition techniques using drones and robots can accurately monitor crop maturity. Meanwhile, AI algorithms analyze factors such as color, texture, and size to provide real-time insights. These technologies can help farmers minimize damage and loss and effectively plan their harvests.
Autonomous Tractors
Autonomous tractors are self-driving cars that can perform various tasks on the farm, such as plowing, planting, spraying, weeding, harvesting, and hauling. Autonomous tractors use AI algorithms and GPS to navigate the ground, avoid obstacles, follow pre-defined routes, and communicate with other vehicles and equipment. Autonomous tractors can help farmers save time, labor, fuel, and money by increasing efficiency and accuracy. These intelligent machines are revolutionizing farming. Autonomous tractors reduce physical labor, improve precision, increase productivity, and reduce costs.
Agricultural Robotics
To answer the question of how AI is transforming agriculture, here's another article. Agricultural robotics is another area where AI is making a difference in agriculture. These robots, equipped with advanced sensors and algorithms, can distinguish between crops and weeds, allowing for precise weed removal without damaging crops. Agricultural robotics can help farmers improve quality and consistency with less time and money wasted.
Insect and plant disease detection
Pest and disease detection is identifying harmful insects and diseases that affect crops and plants. Using a database of known pests and pathogens, AI-based systems employ image recognition and machine learning algorithms to identify signs of disease or pests in plants. By analyzing vast amounts of data and historical records, AI enables growers to detect and respond to potential problems immediately. This approach minimizes the spread of disease and reduces yield loss.
Ensuring Livestock Health
Livestock health monitoring is the process of tracking the health and well-being of animals on the farm. Using AI algorithms, wearable devices, and various sensors can monitor and analyze animal behavior, feeding patterns, heart rate, activity, and other indicators. Over time, this data collection can be used by farmers to prevent livestock diseases, optimize breeding programs, and increase productivity.
Intelligent spraying
Traditional spraying methods often result in excessive use of pesticides, creating environmental concerns and costs. Here is another example of how AI is transforming agriculture. Smart spraying in agriculture is applying pesticides, herbicides, fertilizers, and other chemicals to crops and plants in a precise and targeted manner. AI technology identifies areas that need treatment and delivers precise amounts of pesticides or fertilizers and types of chemicals to each area. It's also programmable, using various sensors and weather forecast data to adjust spraying parameters such as speed, pressure, and nozzle size. This approach reduces chemical use while maintaining effective pest control.
Precision Farming with Predictive Analytics
Precision farming with predictive analytics uses data and AI to optimize agricultural operations and outcomes. Data collection from the soil, crops, livestock, weather, etc., can be guided by AI to generate insights and predictions about various aspects of farming, such as crop selection, planting density, etc., used for precision farming.
Ultimately, precision farming allows farmers to produce more food with fewer resources while protecting the environment and natural resources. These kinds of things that AI can do are literally transforming the entire agriculture industry.
Algorithmic Insights
AI algorithms process vast datasets encompassing weather patterns, soil conditions, crop health, and more. By discerning patterns and correlations, these algorithms offer farmers actionable insights. For instance, predicting weather anomalies enables timely interventions to safeguard crops.
Optimizing Crop Yields
Predictive analytics aids in optimizing crop yields. Through machine learning, AI models identify optimal planting times, recommend suitable crop varieties, and even predict potential pest invasions. This level of precision empowers farmers to enhance productivity sustainably.
Resource Management
AI-driven predictive analytics extends to resource management. Efficient utilization of water, fertilizers, and pesticides is achieved by forecasting needs based on environmental conditions. Application of artificial intelligence in farming not only improves resource efficiency but also aligns with sustainable farming practices.
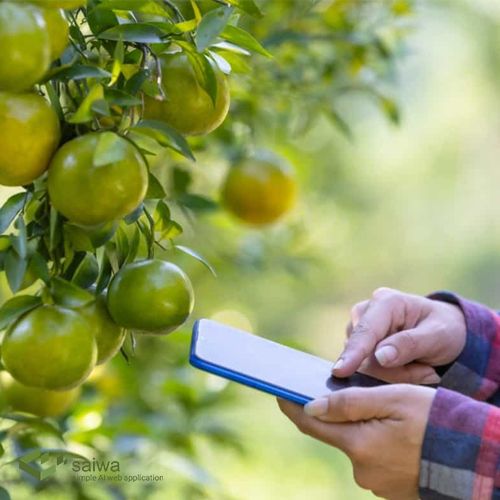
Market demand analysis
Market demand analysis, which is one of the ai in agriculture examples, is a vital aspect in modern agriculture. Artificial intelligence can help farmers choose the best crop to grow and sell. Some companies offer AI-based platforms to help farmers assess market demand. These researchers develop machine learning algorithms to analyze satellite imagery and weather data, providing valuable insights into optimal planting times and the best crops to grow. By analyzing data patterns, market demand for specific products is predicted, helping farmers maximize their profits.
Risk management
Through forecasting and predictive analytics, farmers can minimize the risk of crop failure, it's one the best ai in agriculture examples. Some companies are using artificial intelligence to help farmers analyze the quality of their crops and reduce food loss. These companies develop software products that use artificial intelligence and computer vision algorithms to analyze fruits and vegetables and provide insights about quality, size and more. These AI tools can detect defects and diseases in crops, giving farmers the ability to take preventive measures before crops are affected.
Cultivation of seeds
By collecting data on plant growth, artificial intelligence can help produce crops that are less susceptible to disease and better adapted to climate conditions. With the help of artificial intelligence, scientists can identify the best performing plant species and combine them to create better hybrids. The hybrid process has been used in the agricultural industry for many years. However, gathering genomic information of seeds through artificial intelligence technologies can help speed up the process and increase the probability of success.
Crop protection
Artificial intelligence can monitor the condition of plants to identify and predict diseases, identify and remove weeds, and recommend effective pest treatments. Technologies based on artificial intelligence can identify and classify diseases and pests with high accuracy, and can also suggest the most effective treatment for pests and eliminate the need for widespread insecticides that can harm beneficial insects. lead to the resistance against the drops, reduce.
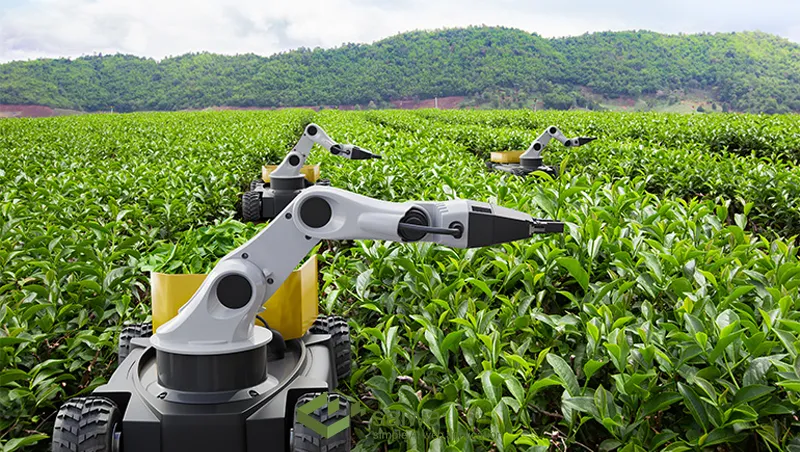
Optimization of automatic irrigation systems
Artificial intelligence algorithms lead to independent product and agricultural data management, this is one of the best applications of AI in agriculture. When combined with IoT sensors that monitor soil moisture levels and weather conditions, algorithms can decide in real-time how much water to provide to crops. An independent crop irrigation system is designed to conserve water and promote sustainable farming practices.
AI Used in Agriculture for Crop Breeding
Here is another item of AI application in agriculture, AI used in agriculture for crop breeding involves analyzing genetic data to develop new crop varieties that are more resilient, productive, and adapted to changing environmental conditions.
Genomic Selection: In AI used in agriculture, AI algorithms analyze genetic markers and phenotypic data to identify desirable traits in plants. This process, known as genomic selection, accelerates the breeding of crops with improved yield, disease resistance, and environmental adaptability.
Phenotyping: AI-powered imaging technologies and sensors monitor plant growth and development. By analyzing phenotypic traits such as plant height, leaf shape, and root structure, AI provides insights into the genetic basis of these traits, aiding in the selection of superior crop varieties.
Hybrid Breeding: AI assists in hybrid breeding by predicting the performance of hybrid combinations. By analyzing genetic data from parent plants, AI models in AI application in agriculture field forecast the traits and yield potential of their offspring, enabling breeders to select the best hybrid combinations.
AI for Analyzing Aerial Data and Imagery
Satellites and high-altitude drones outfitted with spectral, infrared, and RGB imaging sensors accumulate immense geospatial data about farm landscapes. But deriving insights depends on AI analysis. Specific applications for AI projects in agriculture include:
- Crop health monitoring - algorithms detect crop stress, pests, and nutrient variability across fields through aerial imaging to direct interventions.
- Irrigation optimization - software platforms combine imagery with weather forecasts to target watering only where needed and avoid waste.
- Yield prediction - by tracking crop growth progress via temporal imagery and machine learning, yields can be forecasted to inform agribusiness decisions.
- Land use classification - remote sensing data processed by AI distinguishes forests, grasslands, and crops to inform land management.
- Soil composition assessment - hyperspectral imaging can help gauge soil chemistry, moisture, and workability through proxy signals from vegetation.
From small farms to national ministries of agriculture, stakeholders utilize AI interpretation of aerial data to enhance production and sustainability.
The Role of Big Data Analytics in AI for Agriculture:
The agricultural sector has traditionally been data-rich but lacking in the tools and techniques to effectively leverage that data for decision-making. However, the advent of big data analytics and artificial intelligence (AI) has opened new doors for transforming the way data is collected, processed, and utilized in agriculture. Big data analytics, combined with AI technologies such as machine learning and deep learning, is playing a pivotal role in unlocking the full potential of data-driven agriculture.
At the core of big data analytics in agriculture lies the ability to collect and integrate data from various sources, including sensors, drones, satellites, weather stations, and historical records. This vast amount of data, often referred to as "big data," encompasses a wide range of information, such as soil conditions, weather patterns, crop growth stages, pest infestations, and market trends.
Key Applications of Artificial Intelligence in Farming
One key application of big data analytics in artificial intelligence in farming is the development of predictive models for crop yield forecasting. By analyzing historical data on weather conditions, soil properties, and crop performance, AI algorithms can predict future yields with a high degree of accuracy. This information is invaluable for farmers, enabling them to make informed decisions about crop selection, planting schedules, and resource allocation.
Another important application of artificial intelligence in farming is the optimization of resource utilization, such as water, fertilizers, and pesticides. Big data analytics can help identify areas of over- or under-application, leading to more efficient and targeted application of resources, reducing waste and minimizing environmental impact.
AI in Weather Prediction for Farming
Weather Forecasting Models: AI utilizes machine learning algorithms to analyze historical weather data, satellite imagery, and current meteorological conditions. These models in AI used in agriculture field provide highly accurate short-term and long-term weather forecasts, enabling farmers to make timely decisions about planting, irrigation, and harvesting.
Climate Risk Assessment: AI assesses climate risks by analyzing patterns of extreme weather events, such as droughts, floods, and storms. By identifying areas and periods of high risk, farmers can implement strategies to mitigate potential damage, such as adjusting planting dates or adopting resilient crop varieties.
Frost Prediction: Frost can cause significant damage to crops. AI systems predict frost events by analyzing temperature patterns, humidity levels, and wind conditions. Farmers receive early warnings, allowing them to take protective measures, such as using frost blankets or heaters, to safeguard their crops.
AI and IoT in Agriculture
Agriculture, humanity's time-honored endeavor, is on the brink of a technological revolution with the fusion of the Internet of Things (IoT) and application of Artificial Intelligence in farming. This powerful alliance is rewriting the playbook of conventional farming practices, ushering in an era of unparalleled efficiency, sustainability, and productivity.
Application of Artificial Intelligence in Farming | Automating and Monitoring
Precision Agriculture: The landscape of agriculture is changing. AI processes a deluge of data from IoT sensors embedded in machinery and drones. The result is precision agriculture, where automated systems tweak planting depth, seed spacing, and irrigation. The optimized resource use not only enhances efficiency but also aligns with the sustainable farming practices of the future.
Livestock Monitoring: The partnership between AI and the combination of IoT and agriculture is a game-changer. Sensors attached to livestock, feeding data into the AI engine, enable real-time health monitoring. From detecting illnesses in their infancy to predicting calving times, this technology ensures the well-being of livestock. The age-old challenges of animal health are met with modern solutions.
Agricultural Waste Reduction: In AI used in agriculture, Optimizing the application of costly inputs like water, pesticides, and fertilizers based on analytics prevents overuse and economic losses. Precision breeding and harvesting driven by AI also reduces potential food waste stemming from crop failures or timing issues. Meeting demand more closely curbs spoilage factors.
Optimized Breeding: Genetic and phenomic data accumulated from prior seasons and generations feeds into AI models projecting optimal breeding selection for subsequent generations focused on crop yield output and flavor. This facilitates genetic experimentation in silico before investing resources into modifications years down the road.
Weather Forecasting: Satellite imagery, climate data, and weather station networks paired with deep learning predictive models greatly outperform traditional forecasting. Generating various scenarios projecting impacts of weather events well in advance allows for risk mitigation contingency development to protect crop investments.
Finance and Insurance in AI for Agriculture
AI for Agriculture has the potential to revolutionize the agricultural finance and insurance sectors, providing farmers with access to innovative financial products and risk management solutions. By analyzing historical data on crop yields, weather patterns, market trends, and other relevant factors, AI algorithms can assess risk profiles and generate accurate pricing models for crop insurance policies. AI for Agriculture can facilitate more informed decision-making by financial institutions. AI-powered credit scoring models can evaluate a wide range of data points, including farm productivity, soil quality, and climate conditions, to determine a farmer's creditworthiness and tailor loan products accordingly. This data-driven approach not only reduces risk for lenders but also increases access to affordable financing for farmers, enabling them to invest in new technologies and sustainable practices.
Additionally, AI for Agriculture can support the development of innovative financial instruments, such as parametric insurance products that provide payouts based on predefined triggers, like extreme weather events or yield shortfalls. By leveraging AI-driven predictive analytics and real-time data monitoring, these insurance products can offer timely and transparent payouts, providing farmers with a crucial safety net and enhancing their resilience to climate-related risks.
The Outlook for Mainstream Adoption
Increasing Adoption Rates: Despite the challenges, there's a noticeable uptick in the adoption of AI and IoT technologies in agriculture. Governments worldwide are incentivizing modernization through preferential project awards and discounted insurance rates for firms demonstrating technological commitments. The winds of change are palpable.
Role of Research and Development: The journey toward mainstream adoption hinges on continuous research and development. The customization of these technologies to suit the diverse needs of farmers globally is paramount. Governments, private enterprises, and research institutions play a pivotal role in making these technologies more accessible and tailored.
Detection of leaks or damage to irrigation systems
Another applications of AI in agriculture is that Artificial intelligence plays an important role in detecting leaks in irrigation systems. By analyzing the data, algorithms can examine and identify patterns and anomalies that indicate a potential leak. Machine learning models can be trained to detect specific signs of a leak, including changes in water flow or pressure. The real-time monitoring and analysis process allows for early detection, preventing water wastage and possible product damage.
Also, artificial intelligence combines weather data with crop water requirements to identify areas with excessive water consumption. By automating the process of detecting leaks and providing alerts, AI technology increases water efficiency and helps farmers conserve natural resources.
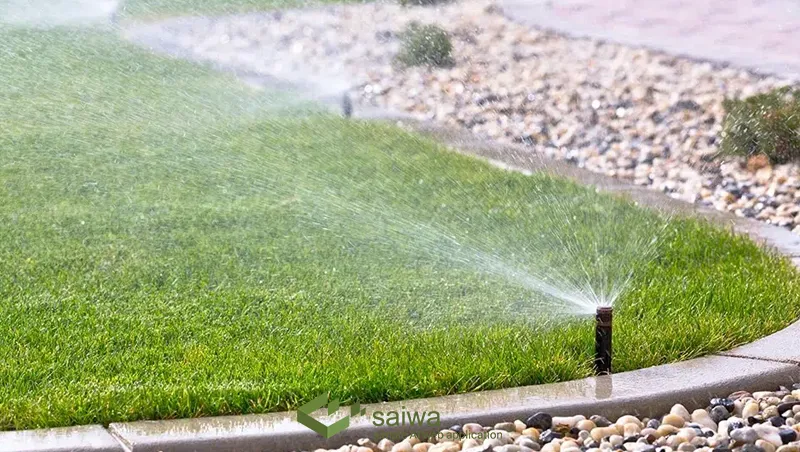
Smart use of pesticides
Currently, farmers know that the use of pesticides is for optimization. However, both manual and automated application processes have important limitations.
Manual application of pesticides provides greater precision in targeting specific areas. This process may be helpful but it is difficult. Automatic spraying of insecticides is faster and does less work, but it is usually imprecise and pollutes the environment.
Drones that are equipped with artificial intelligence offer the best advantages of each approach and avoid their disadvantages. These drones use computer vision to determine how much repellent to spray on each area. This is still in its early stages but is rapidly becoming more accurate.
Read More: AI-Based Drone Operation| AI in Drones use cases
Data Collection and Analysis
AI in agriculture techniques helps extract insights from new agriculture data sources:
Satellite multispectral imagery analyzed using machine learning provides regional-scale crop health monitoring based on changes in spectral reflectance corresponding to plant vigor and development issues. This enables assessing needs over thousands of acres.
Computer vision techniques on drone imagery identify crop rows, detect weeds, and measure canopy cover to guide highly targeted in-field interventions, maximizing efficiency. Autonomous robotic systems traverse fields collecting soil, plant, and microclimate data for AI modeling.
In greenhouses, dense, inexpensive sensor networks continuously monitor temperature, humidity, light, and other environmental parameters at different heights and locations to feed control systems and predictive models optimizing growing conditions.
On cattle farms, wearable sensors, computer vision in feeding areas, and even sound analysis to detect coughing allow tracking animal health and well-being to address issues and ensure optimal feed utilization proactively.
Together, these sources provide invaluable data for AI systems to optimize, predict, and automate across the agriculture industry.
AI-Driven Precision Livestock Farming:
Artificial intelligence in farming for livestock plays a critical role in meeting the global demand for animal-based products and ensuring food security. However, traditional livestock farming practices often face challenges related to animal health, welfare, productivity, and environmental impact. The integration of artificial intelligence (AI) technologies into livestock farming operations, known as precision livestock farming (PLF), has the potential to address these challenges and revolutionize the way livestock is raised.
At the heart of AI-driven precision livestock farming lies the ability to collect and analyze vast amounts of data from various sources, including sensors, cameras, and other monitoring devices. This data can provide valuable insights into animal behavior, health, and environmental conditions, enabling data-driven decision-making and automated interventions.
One of the key applications of Artificial intelligence in farming for livestock is the monitoring and early detection of animal health issues. AI algorithms can analyze data from wearable sensors, cameras, and other monitoring devices to identify potential health problems, such as respiratory diseases, lameness, or reproductive issues. By detecting these issues early, farmers can take proactive measures, such as administering targeted treatments or adjusting environmental conditions, potentially reducing the risk of disease outbreaks, and improving animal welfare.
Challenges and Concerns in AI Use
Thoughtful AI adoption balancing benefits and risks includes:
AI systems must seamlessly integrate into often aging on-farm equipment through retrofitting kits and upgrades. Intuitive interfaces are needed for user comfort.
Cybersecurity looms large for sensors, robots, and decision-making systems controlling physical growing operations. Breaches could jeopardize crops.
Harsh outdoor farm conditions challenge technology resilience. Rugged, weatherproofed, agriculture-specific designs are needed.
Predictions of AI in agriculture should aim to augment, not replace, generational farmer wisdom and on-the-ground experience. Transparent human-in-the-loop decision protocols enable trust.
Limited rural broadband access may constrain real-time data transmission. More processing must shift to robust edge devices in the field.
Upfront AI costs may deter adoption by small farms without support mechanisms. Co-ops, partnerships, and government extensions can assist in integration.
Lack of Qualified Staff: AI used in agriculture still has some issues. Optimizing AI farming solutions requires hiring staff capable of utilizing and interpreting the analytics, sensors readings, and software dashboards driving decisions and oversees automated equipment. Digital literacy programs tailored to agriculture technology will have increasing importance to fill personnel gaps.
Privacy Concerns: Some agricultural producers express reservations around privacy, data ownership, and transparency when considering partnerships with AI developers requesting farm data access. Creating clear contractual guidelines for appropriate data usage and securing sensitive information will build necessary trust.
Rural Connectivity Issues: Lack of reliable high-speed internet connectivity in remote farming areas limits feasibility of connected precision agriculture dependant on cloud data transfer. Government programs addressing rural digital divide gaps will hopefully expand access and speed.
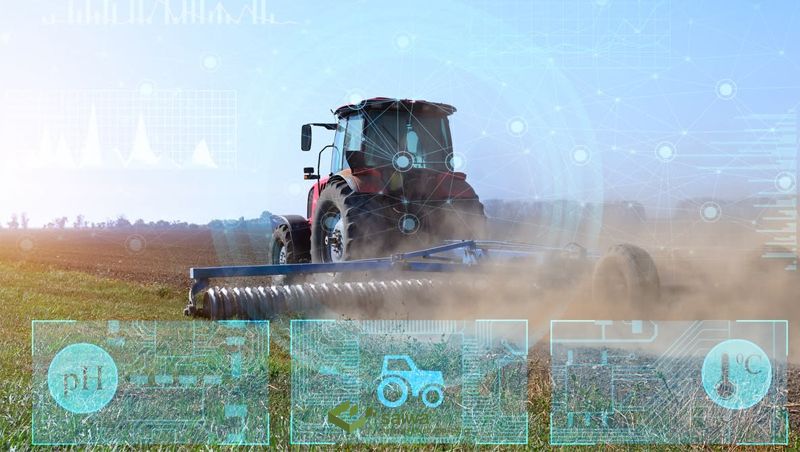
The Role of Government and Policy in Promoting AI in Farming
Governments play a crucial role in fostering the responsible development and adoption of AI in farming technologies. By establishing clear policies, regulations, and standards, governments can ensure that AI solutions are developed and deployed in an ethical, transparent, and sustainable manner. Additionally, governments can provide incentives, funding mechanisms, and supportive infrastructure to promote innovation and encourage the adoption of AI in agriculture, particularly among smallholder farmers and underserved communities.
AI projects in Agriculture | Training and Education of Future Farmers
AI tutors play a pivotal role in this educational model, monitoring students' facial expressions and biometrics to assess their engagement and emotions. Based on this data, teaching strategies are dynamically adjusted to optimize the learning experience for each individual. Additionally, augmented reality is employed to overlay computer-generated sensory information onto real farm environments. This innovative approach enriches the connections between theoretical lessons and the practical application of skills on physical equipment. By blending tradition with innovation, AI is driving the development of new and effective models for agricultural education that can be customized to cater to the needs of rural learners worldwide. This transformative approach not only ensures that the next generation of farmers is well-equipped with the necessary skills but also fosters a deeper and more meaningful understanding of agricultural practices through cutting-edge technology.
Data Security and Integration for Artificial Intelligence in Farming
AI in farming and managing agriculture data flows responsibly enables value:
Blockchains allow securely recording data like crop treatments in tamper-evident ledgers while preserving privacy. This supports supply chain transparency between stakeholders.
Edge computing handles localized data analysis on-site while relegating only key insights to the cloud. This reduces reliance on connections.
Data lakes unify disparate structured and unstructured datasets like weather, equipment logs, and field images to drive enterprise analytics.
Permissions-based access controls on pooled data helps balance openness and privacy. Cybersecurity protections like encryption safeguard farms.
Partnerships across growers, analytics providers, and manufacturers facilitate open data sharing under mutually beneficial terms to unlock artificial intelligence in farming possibilities.
Novel Automated Tasks
AI-driven robotics as a subset of artificial intelligence in farming automate delicate agriculture work:
Machine vision guides robotic arms equipped with soft grippers to harvest ripe produce gently. This automation relieves labor shortages for time-sensitive picking.
Autonomous robotic is another form of leveraging artificial intelligence in farming. Autonomous robotic weeders utilize computer vision to distinguish crops from invasive plants. Precise removal mechanisms like lasers, electrical pulses, or mechanical tools then target weeds without crop damage.
Micro-sprinklers controlled by AI conservation models activate briefly and only when needed in small customizable polygonal patterns based on exact soil moisture data. This significantly cuts water usage.
Coordinated swarms of drones can perform pollination efficiently by identifying crops ready for pollination using onboard cameras and surveying fields in optimal sweeping patterns utilizing AI planning algorithms.
Careful automation design focused on addressing genuine pain points allows machines to take over repetitive and delicate tasks while humans focus on higher-judgment responsibilities.
Robots for agriculture
As we have already said, because the global demand for food is increasing, innovative solutions are being created to help optimize operations in agriculture. Robots are the new high-tech workforce in agriculture. These versatile machines can perform a wide range of tasks, from planting and watering to pest control and soil analysis. By automating these processes with AI in agricultural robots, farmers can benefit from the benefits of increasing productivity and reducing labor costs.
By collecting valuable data to fine-tune crop performance and resource management, you know that the future of agriculture will be about smarter, not harder, farming.
Digital FarmHand
It is a robotics platform designed for smallholder farms. With its advanced ability to detect and identify objects in its environment, including plants and weeds, this robot can perform a wide range of tasks such as mapping fields and collecting data on crop health and performance.
The most important advantage of this platform is its potential to revolutionize the agricultural industry. By automating the process of identifying and removing weeds, this robot can help farmers rely less on herbicides and other harmful chemicals, leading to cost savings and a safer, healthier environment.
AGRIM-X
It is a fully autonomous drone used for precision agriculture. This advanced drone uses computer vision algorithms and artificial intelligence to provide farmers with high-resolution images of crops, giving them the insights they need to make informed decisions about their farming practices. With its independent function, this robot can collect and direct data on its own without the need for human intervention. This drone is very lightweight and maneuverable, and this feature gives it the ability to easily maneuver in fields and record data from different angles.
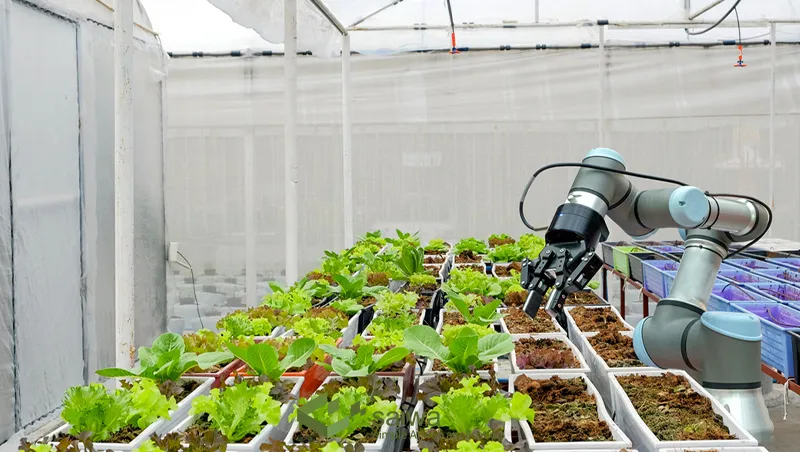
Nitrogen Diagnosis Using AI
Optimizing plant growth hinges on understanding their needs. Traditional methods for assessing nitrogen in plants can be time-consuming and imprecise. This is where Artificial Intelligence (AI) steps in, revolutionizing nitrogen diagnosis.
AI algorithms analyze data from various sources, including spectral imaging of leaves. By identifying subtle variations in how plants reflect light, AI can accurately estimate a plant's nitrogen content. This empowers farmers to make data-driven decisions about fertilizer application, ensuring plants receive the perfect amount of nitrogen in plants.
By using Nitrogen Diagnosis Using AI, farmers can control factors such as:
Nutrient uptake is the process by which plants absorb essential minerals from their environment.
Protein synthesis utilizes nitrogen from nutrients to build the building blocks of life within plants.
Hormone regulation relies on nitrogen to create chemical messengers that orchestrate plant growth and development.
Chlorophyll production requires nitrogen to manufacture the pigment that captures sunlight for photosynthesis.
Nucleic acid formation, the foundation of DNA and RNA, depends on nitrogen for plant growth and reproduction.
AI is ushering in a new era of agricultural intelligence, and nitrogen diagnosis is just the first course. By embracing this innovative technology, farmers can unlock the true potential within their plants, ensuring a thriving future for generations to come.
Read More: Uncovering the Vital Role of Nitrogen in Plants Growth | A Comprehensive Guide
Future of AI in agriculture
In agriculture, the application of artificial intelligence is paving the way for the future of farming. As the world's population grows, so does the need for efficient and sustainable agriculture. AI offers many applications to improve agriculture's efficiency, productivity, and environmental stewardship. Through advanced data analytics, machine learning algorithms, and automation, AI can optimize various aspects of farming, such as crop monitoring, soil analysis, predictive analytics, and robotic automation. Farmers can benefit from real-time insights into crop health, weather patterns, and soil conditions, enabling precise resource allocation and decision-making.
In addition, AI-driven automation, including autonomous machinery and intelligent spraying systems, reduces labor, increases accuracy, and minimizes environmental impact. The integration of AI in agriculture enables farmers to adopt precision farming techniques, reduce waste, conserve resources, and improve overall productivity. As AI continues to advance, the future of agriculture holds great potential for sustainable practices, increased food production, and improved livelihoods for farmers around the world.
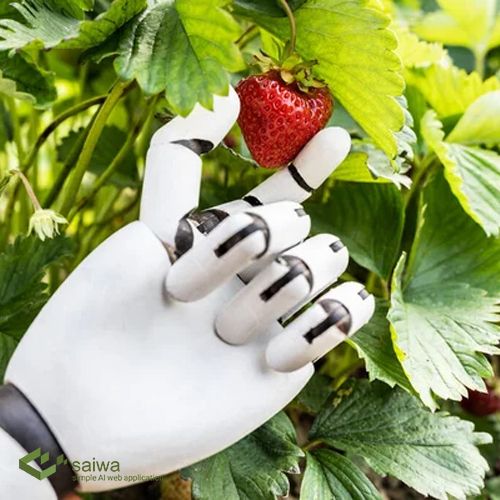
How AI is used to optimize supply chain logistics in agriculture?
Applications of AI in agriculture with examples have significantly transformed supply chain logistics, optimizing various processes from harvesting to distribution. AI-powered technologies are revolutionizing the way farms manage their operations, leading to increased efficiency and reduced costs.
Farm equipment predictive maintenance is a well-known application of AI in supply chain optimization. Farmers can use machine learning algorithms to forecast when maintenance is needed and to track the operation of their equipment in real-time. Tractors and other agricultural equipment, for example, have sensors attached to gather data on temperature, vibration, and usage trends. Artificial intelligence systems then examine this data to find possible problems before they result in equipment failure. Farmers may reduce downtime, increase production, and prolong the life of their gear by proactively scheduling maintenance based on predictive analytics.
The creation of automated harvesting robots is a significant use of AI in supply chain logistics. These robots can identify ripe crops, take them precisely, and sort them according to standards of quality since they are outfitted with sophisticated sensors, computer vision, and machine learning skills. For example, organizations such as Sufficient Robotics have created AI-powered autonomous apple-picking robots that can identify and carefully remove ripe apples from trees. Farmers may overcome labor constraints, save harvesting costs, and increase operational efficiency by automating the harvesting process.
AI is used in agriculture not merely for harvesting but also optimization of processes in storage and distribution. powered by AI storage systems, for instance, can keep an eye on the temperature, humidity, and airflow in warehouses and other storage facilities. Artificial intelligence algorithms can optimize storage conditions and guarantee the quality and freshness of perishable goods by real-time analysis of this data. In a similar vein, distribution systems driven by AI can optimize timetables, routes, and inventory levels to save cash on transportation and cut down on food waste.
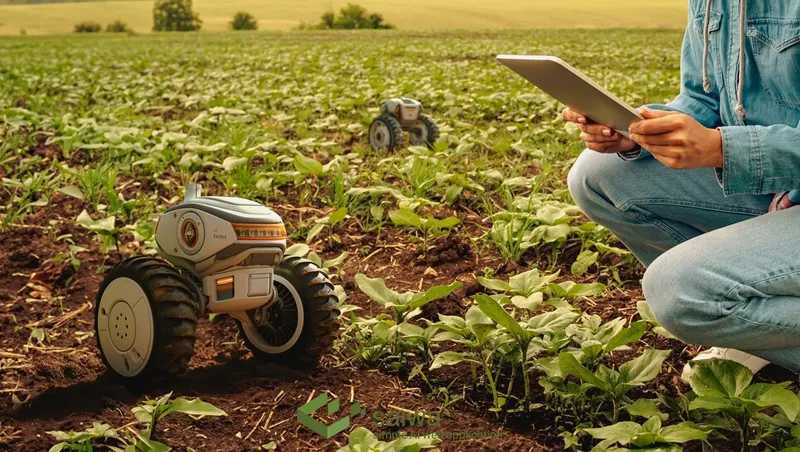
The applications of AI in agriculture with examples show how AI is transforming supply chain logistics from harvesting to distribution. Utilizing autonomous harvesting robots and predictive maintenance for farm equipment, farmers may increase productivity, cut expenses, and improve the sustainability of their operations. Furthermore, producers can minimize waste across the supply chain and maintain product quality and freshness by utilizing AI-powered storage and distribution systems.
Public-Private Partnerships and Collaborative Efforts in AI in Agriculture
The successful development and implementation of artificial intelligence technologies in agriculture require collaborative efforts and strategic partnerships among various stakeholders, including government agencies, research institutions, technology companies, and agricultural communities. The complexity and interdisciplinary nature of AI in agriculture necessitate a comprehensive approach that leverages the expertise, resources, and perspectives of diverse actors.
Public-private partnerships (PPPs) can play a crucial role in driving innovation and facilitating the responsible adoption of AI in agriculture. These partnerships bring together the resources and capabilities of government entities, academic institutions, and private sector companies, fostering an environment conducive to research, development, and practical implementation of AI solutions.
Government agencies can provide policy support, regulatory frameworks, and funding mechanisms to promote the development and adoption of AI technologies in agriculture. By establishing clear guidelines and standards, governments can ensure that AI solutions are developed and deployed in a responsible and ethical manner, addressing concerns related to data privacy, transparency, and environmental sustainability.
Conclusion
The transformative potential of AI in agriculture is a game-changer. From monitoring crop health to autonomous machinery to ensuring the well-being of plants and animals, AI is revolutionizing every form of farming. As this technology continues to evolve, farmers will be equipped with powerful tools to ensure sustainable practices, optimize productivity, and meet the challenges of feeding a growing global population.