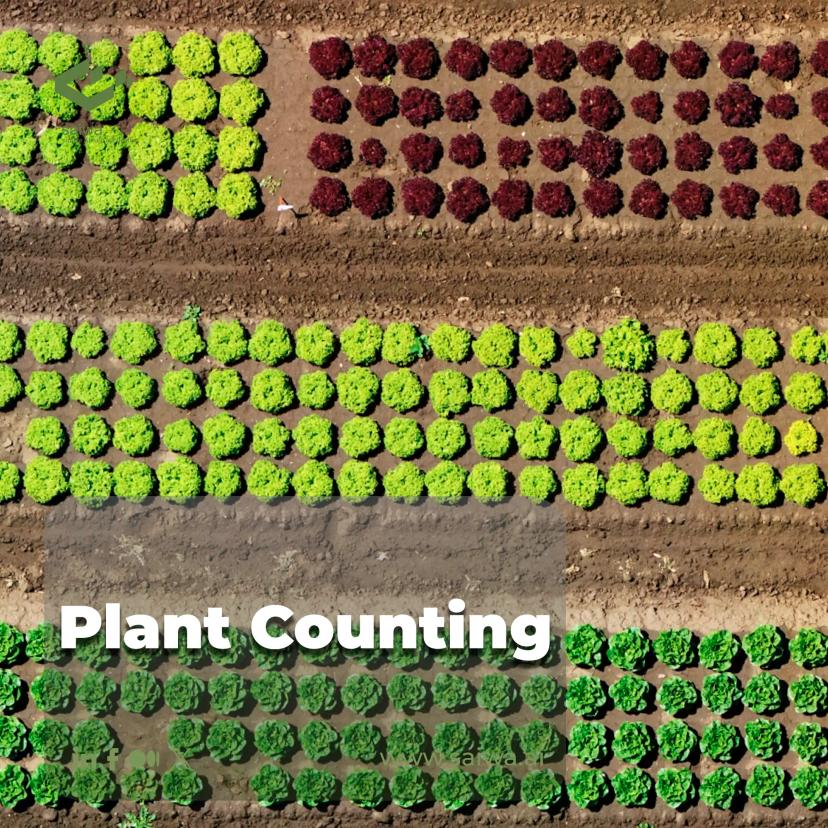
The Surprising Value of Plant Counting
As a nature lover, have you ever wondered why researchers go to such great lengths to count plants in forests, prairies, and wetlands? It may seem tedious to tally trees, measure meadow flowers, and track weeds. However, it turns out that carefully counting plants provides vital data to guide conservation efforts and inform environmental policy. In this article, we’ll explore why Plant Counting matters, how it’s traditionally been done, and some cool new technologies like drones and AI that are revolutionizing plant counts to help us better understand and manage fragile ecosystems.
Read Also: Sustainability Through AI |Counting Trees for a Better Tomorrow
Why Counting Plants Matters More Than You’d Think
There are many important reasons experts still spend lots of time methodically counting plants species by species across the landscape:
Understanding Biodiversity Health
The types and distribution of plants serve as a measure of overall ecosystem diversity and stability. Declines in key plant populations can be an early warning of environmental threats from climate change to invasive species.
Estimating Biomass and Carbon
The number and sizes of plants in an area allow more accurate estimates of total biomass and carbon stocks – critical data points in climate and carbon cycle research.
Informing Land Management
Quantifying plant populations provides key insights for parks departments, forest managers, and farmers seeking to conserve or harvest vegetation sustainably.
Tracking Invasive Species
An increasing count of invasive plants can prompt action to control their spread and impacts on native species.
Modeling Ecosystem Changes
Plant counts serve as inputs to ecological models that aim to predict the effects of changes from climate shifts to development.
Conserving At-Risk Plants
Carefully monitoring numbers of rare, threatened, or endangered plants is vital to recovery programs seeking to boost their survival. So, while counting foliage isn’t as glamorous as tracking tigers, plant census data provides an essential foundation for many scientific studies, conservation policies, and environmental protection. Let’s look at how the work gets done.
Traditional Plant Counting
Before emerging technologies opened new possibilities, plant counting was done through ground surveys and manual techniques that required many boots on the ground:
Visual Surveys: Researchers would slowly walk through an ecosystem systematically, visually tallying and recording observed plant species and numbers.
Sample Plots: Counting plants in small controlled sample areas that represent the broader region of interest.
Transects: Counting plants within a set distance on either side of straight survey lines traversing the habitat.
Mark-Recapture: Tagging, counting, removing, and replanting plants to estimate the total population based on recaptured tagged plants.
Quadrat Sampling: Tossing down grid frames of a standardized size in random spots and counting plants within the square.
Night and Weather Constraints: Many plants only bloom at night or during rain which creates visibility challenges.
Accessibility: Rugged, remote, or hazardous landscapes make access for field teams very difficult. This leaves some ecosystems under-surveyed.
Observer Fatigue: Visual plant counting demands high levels of consistent concentration. Fatigue inevitability sets in leading to declining accuracy.
These traditional methods, while reliable, are very time and labor-intensive. Accuracy depends heavily on observer diligence and conditions. Large and remote wild areas were especially difficult to survey thoroughly.
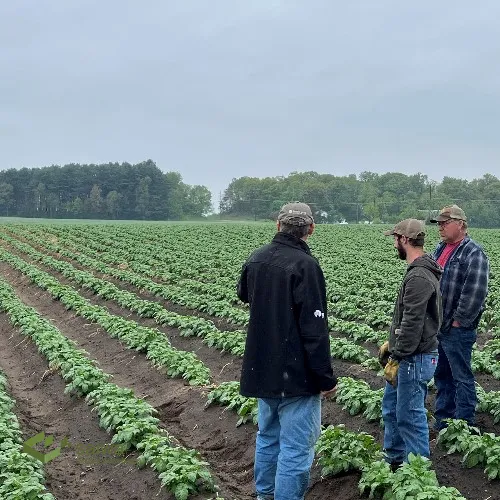
New Tech Revitalizing Plant Counting
Exciting new technologies are supercharging scientists’ plant counting capabilities:
Satellite Imagery Analytics: Processing overhead images to detect tree canopies and vegetation indices, providing insights into plant counts.
Drone-Based Aerial Photography: Detailed photographic surveys from drones and airplanes that can be manually or automatically analyzed to count plants.
LiDAR Remote Sensing: Airborne laser pulses that generate detailed 3D models of vegetation structure, allowing density estimation.
Proximal Ground Sensors: Portable on-the-ground sensors that measure indicators related to the presence and number of nearby plants.
Computer Vision: Sophisticated algorithms that can automate the identification and counting of plant species in field photographs.
These technologies enable much wider-scale surveys across far larger areas than boots-on-the-ground methods allow. However, ground validation is still essential to verify the accuracy of analysis based on remote sensing data.
Read Also: The impact of machine learning in agriculture
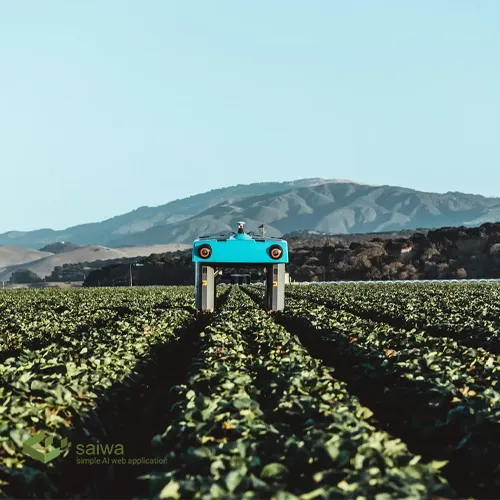
Plant Counting with Ai
The latest wave of innovation leveraging machine learning and AI in agriculture holds incredible promise to revolutionize ecological efforts to census plants:
ML Image Classification: Models can categorize overhead and ground-level images into plant versus non-plant features as an efficient proxy for counting.
Object Detection: Algorithms automatically detect and locate individual plants via bounding boxes drawn around them in images.
Instance Segmentation: Deep learning approaches that differentiate each unique plant at the pixel level for precise segmentation and counting.
Multimodal Data fusion: Combining ground, aerial, and satellite data sources with AI to achieve a more accurate composite view of plant distribution and density.
Active Machine Learning: Humas collaboratively trains models by annotating new photographs to enhance accuracy through continuous feedback.
Saiwa, an AI company, has developed an advanced plant counting tool using AI. Their solution uses cutting-edge algorithms and object detection and Object counting technology to automate accurate plant counting in images. This innovation accelerates data collection, revolutionizing ecological studies and resource management. The versatility to work with various data sources, such as aerial imagery, enhances its applicability. Saiwa's AI tool marks a transformative step towards efficient, accurate, and scalable plant counting, benefiting researchers, conservationists, and land managers alike.
Read Also: Seedling Counting | Importance & Advanced Methods
These AI methods allow plant counting at scales and speeds that are impossible for human researchers alone. However, the performance of the models depends heavily on the quantity and quality of training data provided.
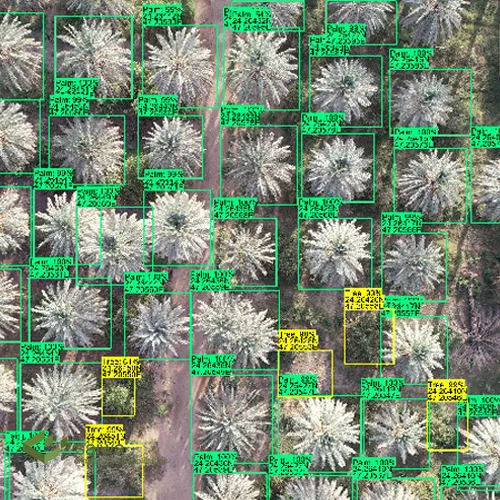
Cutting-Edge Computer Vision Techniques
Modern computer vision and deep learning now enable automating many aspects of plant quantification from imagery:
Instance Segmentation using algorithms like Mask R-CNN can delineate individual plants at the pixel level even with occlusion. This enables precise per plant counting and measurement.
Panoptic segmentation coherently unifies instance segmentation with semantic segmentation for efficient scene understanding. This helps separate plants from background.
3D reconstruction techniques that fuse LiDAR data with imagery provide detailed 3D plant models facilitating trait measurement.
Analyzing time-series image datasets using recurrent networks can identify the same plants across dates to monitor growth.
Weakly-supervised learning methods can count plants using only image-level labels without exhaustively annotating individual plants. This reduces annotation needs.
Overall, computer vision is enabling plant quantification at unprecedented scales using large historical image datasets and real-time streams.
Counting Plants with Machine Learning
To count plants using machine learning or deep learning, a dataset of annotated images is required for model training. The annotations indicate the locations and identities of plants in the images. Common deep learning algorithms like Convolutional Neural Networks (CNNs) and Recurrent Neural Networks (RNNs) are employed to process the image data and perform the counting task.
Benefits and challenges of deep learning for plant counting
Machine learning brings with it both tremendous advantages but also challenges to balance:
Potential Benefits:
Scalability to analyze massive aerial satellite databases spanning large regions.
Automating repetitive identification and counting tasks that are exhausting and time-consuming for humans.
Reducing observer bias and fatigue through consistent algorithmic counting.
Lower long-term expenses compared to extensive manual surveying across huge areas.
Potential Challenges:
ML models rely heavily on costly labeled training image datasets that can contain errors. Garbage in, garbage out.
Algorithmic “black box” analyses are harder to directly interpret compared to field ecologists’ reasoning.
Validation against ground-truth field observations is essential to confirm remotely sensed ML plant counts.
Processing huge 3D LiDAR vegetation maps requires substantial computing resources.
Models may falter when analyzing new regions differing significantly from their training data.
Active Learning and Human-AI Collaboration
While deep learning automation excels at plant counting tasks, human ecologists still play a pivotal role:
Humans-in-the-loop via active learning paradigms to annotate additional useful images enhance model accuracy.
Prioritizing images where the model is unsure for manual labeling focuses efforts on maximally informative samples.
Allowing interactive correction of model predictions and incremental retraining closes the feedback loop.
Selectively combining model-predicted plant counts with manual review balances efficiency with accuracy.
Leveraging domain experts' knowledge through human-AI collaboration overcomes shortcomings of purely manual or automated approaches.
When thoughtfully designed, human-AI teams achieve synergistic results, exceeding what either can accomplish alone.
Achieving Generalizability Across Ecosystems
For reliable applicability across diverse regions, AI plant counting models must exhibit robust generalization:
Domain adaptation techniques enable models trained on datasets from one ecosystem to be applied to new environments with minimal annotation.
Generating synthetic training data approximating diverse conditions improves model resilience to new unseen scenarios.
Extensively evaluating performance on images captured across various biomes, weather, times of day and seasons quantifies generalizability.
Online learning enables models to continuously adapt in real-time as sensor data streams in from new locations and conditions.
Multi-environment model validation, adaptive training, and data augmentation strategies help ensure plant counting reliability across the full spectrum of Earth's ecosystems.
Model Evaluation
Rigorous evaluation methodology is critical to advance plant counting models:
High-quality benchmark datasets with verified ground truth manual counts across diverse species, densities, and imaging conditions enable standardized comparisons.
Common performance metrics like precision, recall, F1 score, and mean average error quantify accuracy for counting, localization, and segmentation tasks.
Model architectures and data representations can be validated by cross-testing on multiple datasets to evaluate generalizability.
Occlusion simulation and noise injection checks resilience to real-world visual challenges.
In situ field measurement provides vital empirical validation of performance before operational rollout.
Without robust evaluation protocols and benchmarks, it is impossible to determine true model readiness to be deployed at scale in the field. Research requires maintaining close collaboration with domain experts and practitioners.
Real-World Case Studies
Researchers and startups are demonstrating AI plant counting in diverse agricultural and ecological settings:
Crop yield estimation - Computer vision models count corn stalks, wheat heads, and fruit loads in imagery captured by drones flying over farms. This provides precise yield forecasts to optimize harvesting.
Forest surveys - Airborne LiDAR and hyperspectral data processed by machine learning provides detailed mapped outputs of tree densities, heights, and health to guide reforestation efforts.
Carbon stock assessment - Combining satellite image recognition with ground-based lidar scanning enables estimating biomass and carbon stored in forest areas to support carbon credit markets.
Wildlife habitat monitoring - AI-driven analysis of vegetation and plant biodiversity in aerial wildlife survey footage helps map critical habitats and food sources over time.
Invasive species tracking - Object detection models identify and map populations of invasive plants threatening ecosystems based on low altitude UAV footage and multi-sensor fusion.
As datasets and model techniques mature, automated plant counting use cases will continue expanding across agriculture, forestry, and environmental domains.
Outlook for Future Research and Commercialization
While research literature establishes feasibility, real-world deployment requires addressing key gaps:
Robust performance benchmarking using large annotated data volumes across diverse crop varieties, growth stages, lighting conditions, and sensor platforms.
Reducing errors from occlusion and complex clustering of plants through enhanced segmentation algorithms and multimodal data integration.
Improving cross-dataset generalizability so models perform reliably even on new unseen geographies and species.
Increasing interpretability to build trust and enable expert oversight of model behaviors.
Streamlining data processing and analysis pipelines for operational use by growers, ecologists, and forest managers.
Integrating capabilities into field robotics for automated continual monitoring at high precision.
Advances in these areas will support commercialization of scalable, economical plant counting services, enabling new data-driven practices across the agriculture and environment sectors.
Conclusion
Thoughtfully designed and validated machine learning technologies have the potential to massively scale up plant counting in ways not possible through manual approaches alone. This could provide transformative ecological insights to guide conservation. However, researchers must apply AI carefully and rigorously test against field data to develop trusted solutions.