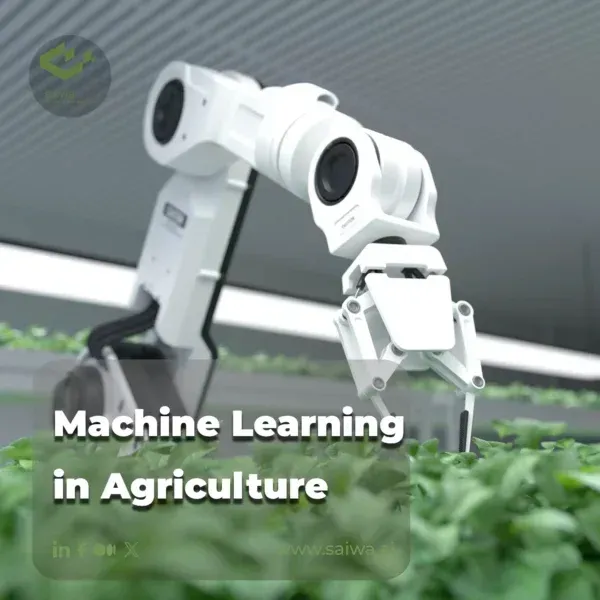
Agriculture is a pillar of civilization, essential to feeding the world's growing population. However, climate change, water scarcity, land degradation, and other pressures pose immense challenges to global food security. Advanced technologies such as artificial intelligence (AI) and machine learning offer new opportunities to improve agricultural productivity, efficiency, and sustainability.
In this article, we define machine learning and its subset, deep learning, review applications of Machine learning in agriculture, and discuss research frontiers. We aim to provide a technical overview of how machine learning can address agricultural challenges to support food security.
Machine Learning's Unprecedented Capabilities
Before delving into the applications, it's crucial to comprehend why machine learning is a game-changer in agriculture. Unlike traditional methods that often rely on guesswork and intuition, machine learning operates on data-driven insights. By analyzing historical and real-time data, machine learning algorithms can identify patterns, correlations, and anomalies that would be humanly impossible to detect.
Why machine learning in agriculture is important?
The agricultural industry is facing many risks and uncertainties due to the change in climatic conditions and market trends, which cause significant losses in production and waste of resources. In recent decades, experience combined with accurate weather data has helped farmers make accurate guesses in the field of agriculture, but more success and diversity can still be achieved in this field.
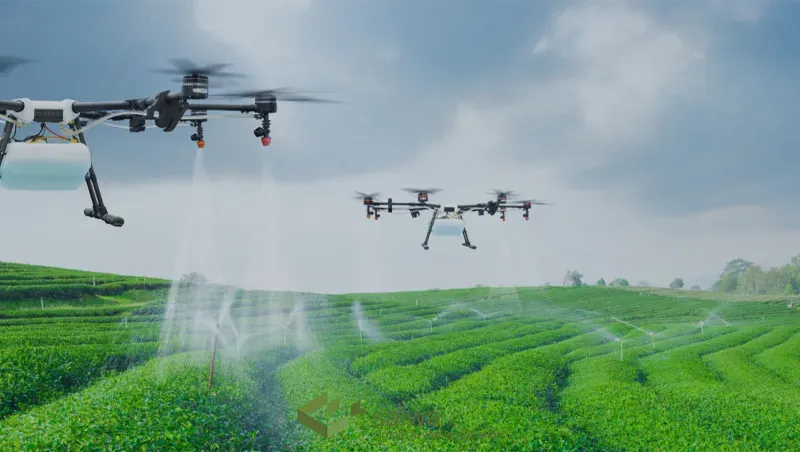
Benefits of machine learning in agriculture
Machine learning in agriculture creates advantages that being aware of can help a lot to people working in this field.
Reducing environmental risks: Machine learning helps farmers to optimize irrigation schedules, fertilizer use, and pesticide use to reduce waste and environmental damage. It can also predict environmental conditions, helping farmers proactively adjust their practices to safeguard ecosystems.
Save time and labor: Machine learning automates field mapping, crop health monitoring, and fertilizer application. This issue can save farmers time and money and reduce their need for hired labor.
Improving yield: Machine learning helps farmers to optimize resources, resulting in increased crop yields, which can help improve food security and reduce hunger. This improvement can help enhancing food security, reduce hunger, and provide more stable food supplies for growing populations.
Cost reduction: Machine learning helps farmers save on vital resources such as water, fertilizers, and pesticides, which can increase profitability and make agriculture more sustainable in the long run.
Improved decision-making: Machine learning in agriculture gives farmers the ability to make better decisions about when to plant, how to irrigate, and when to use fertilizer.
Increased safety: Machine learning helps farmers avoid dangerous tasks such as working with tractors. This can improve the safety and health of farm workers. By automating dangerous tasks, farmers can reduce the risk of accidents and injuries on the farm.
Providing personalized advice: Machine learning can provide personalized recommendations for planting, watering and fertilizing to farmers.
Building resilience to climate change: Machine learning in agriculture helps farmers adapt their practices to cope with weather patterns that can create resilience to climate change.
Biodiversity Conservation: Machine learning helps farmers manage their land in a way that preserves biodiversity. This can protect ecosystem services and conserve natural resources. Machine learning in agriculture helps monitor soil health, wildlife, and plant diversity, supporting sustainable land management practices.
Improving food quality: Machine learning helps farmers produce safer and better-quality food. This can improve public health and increase profitability for farmers. This result of machine learning in agriculture in higher food safety standards, better public health outcomes, and potentially greater profitability for farmers.
Importance of Agriculture and Its Challenges
Agriculture provides the fundamental biological sustenance underpinning human societies. Key challenges in coming decades include:
Feeding 10 billion people: The global population will approach 10 billion by 2050, requiring boosting food production by an estimated 50%. More output must be sustainably achieved from the same or even diminished resources.
Climate change stresses: Rising temperatures, changing precipitation patterns, and more extreme events will pressure crop yields and livestock health. Adaptation and resilience to maintain production is imperative.
Environmental degradation: Soil erosion, aquifer depletion, biodiversity decline, and agricultural runoff damage ecosystems but must be curbed to ensure long-term viability.
Changing food preferences: Rising incomes drive greater consumption of resource-intensive meat, dairy, and processed foods, increasing agricultural burdens.
Post-Harvest Losses: A considerable proportion of global food is lost due to inadequate storage, transportation, and processing infrastructure. Enhancing the efficiency of supply chain is vital to reducing these losses and ensuring more food reaches consumers.
Technological Divide: While precision agriculture and smart farming technologies are transforming agriculture, many small-scale and subsistence farmers lack access to these innovations due to financial and infrastructural constraints. It is therefore imperative to address this divide in order to ensure equitable growth.
Declining Pollinator Populations: The reduction in pollinators, such as bees, due to habitat loss and pesticide use poses a serious threat to crop pollination. Addressing this issue is vital for the maintenance of food production.
Satisfying global nutrition needs in coming decades under these intensifying constraints presents a monumental challenge requiring transformational innovation. Advanced technologies like AI and machine learning offer promising capabilities.
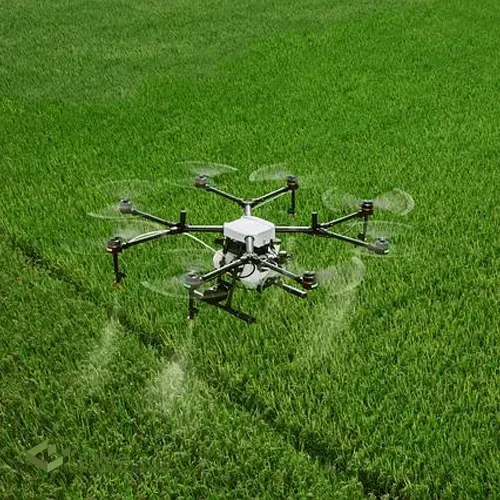
Machine Learning vs. Artificial Intelligence in Agriculture
Machine learning, which falls under the umbrella of artificial intelligence, focuses on algorithms that derive insights from data, allowing them to formulate predictions or decisions without explicit programming instructions. The key distinction is that machine learning emphasizes automated, data-driven learning, while AI can encompass broader capabilities, including human-coded rules and logic. However, machine learning underpins many applied AI solutions because it allows systems to refine themselves based on new agricultural data patterns continuously. So, while AI may use machine learning, it also involves knowledge engineering, which requires human expertise.
Read more: AI in Agriculture | Practical Application & Future of AI in Agriculture
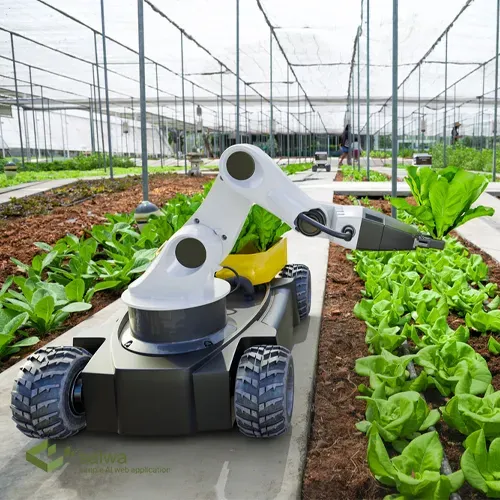
How Machine Learning Can Be Used in Agriculture?
Machine learning in agriculture offers diverse capabilities to address agricultural challenges:
Crop and soil modeling
Simulate and predict crop development and yields based on variables like genetics, management practices, weather, and soil conditions using supervised learning regression techniques. Allows optimizing interventions. Machine learning in agriculture can also aid in precision fertilization, ensuring crops receive optimal nutrient levels. It can help predict long-term soil health trends, enabling sustainable farming practices.
Disease/pest detection
Classify plant diseases and insect pests from imagery using convolutional neural networks for early intervention and targeted spraying. This reduces crop losses and minimizes the need for blanket pesticide applications. Continuous learning algorithms can adapt to emerging pathogens and pests, keeping detection methods up to date.
Weed/crop segmentation
Detect locations of unwanted weed species amidst crops using pixel-wise semantic segmentation models on UAV imagery to guide selective treatment. These techniques reduce herbicide usage, contributing to environmentally friendly farming. Real-time segmentation can also assist in autonomous farming equipment for precision weeding.
Water management
Apply reinforcement learning to dynamically control irrigation to maximize crop yield versus water use efficiency. Machine learning in agriculture solutions can account for weather forecasts and soil moisture in real-time to optimize watering schedules. Furthermore, machine learning models can help predict water demand for specific crop cycles, aiding resource planning.
Livestock monitoring
Track animal movement, behavior, and health with computer vision, wearables, and anomaly detection algorithms. Enables early disease diagnosis. These systems can also provide insights into feed efficiency and breeding patterns. Advanced monitoring tools can predict stress levels in livestock, improving overall animal welfare.
Agrichemical optimization
Tune precision variable rate applications of fertilizers, pesticides, and herbicides across fields using ML models integrating soil, weather, and crop data. Minimizes ecological impacts. Machine learning provides both predictive insights to guide decisions as well as operational capabilities assisting farmers in applying interventions.
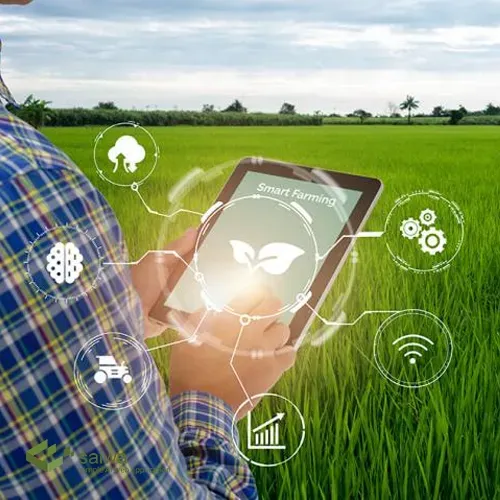
Yield prediction
The process of predicting returns is essential for the global and regional economy. Knowing when to harvest and what crops to grow to meet market needs is important for any farm. Many parameters can affect the yield quantity, including environmental factors, phenotypic data, and weather information. Modern machine learning models can analyze all factors to increase the accuracy of yield prediction.
Selective breeding
Traditional crop breeding follows the principle of Mendelian inheritance and states that one gene controls each trait. However, more recent research has shown that most traits are controlled by multiple genes that interact with each other and the environment in complex ways. This shows that traditional breeding methods are usually inefficient and can take several generations and time to get the desired results. On the other hand, artificial intelligence can identify patterns in data that may not be obvious to humans, so it can quickly and more efficiently identify desired traits for modification.
Agricultural Knowledge Mining
There is a wealth of agricultural knowledge and insights contained in large volumes of unstructured data like research papers, government reports, articles, manuals, and documents. But extracting useful information from these text sources has been difficult. Recent advances in natural language processing and text mining using machine learning now enable systematically mining such corpora to discover hidden patterns and actionable knowledge:
Topic modeling algorithms can rapidly parse thousands of agricultural documents to extract frequently discussed concepts and trends. This allows aggregating knowledge on specific issues like pest management or irrigation practices.
Text classifiers developed on labeled text can categorize agricultural documents into domains like crop science, horticulture, animal husbandry, etc. This enables filtering sources by relevance.
Entity recognition techniques can identify key objects like crop varieties, fertilizers, pests, etc., and their relationships in unstructured text. This automates knowledge graph construction.
Sentiment analysis of the text can discern farmer reactions and opinions on agricultural technologies and policies guiding R&D.
Summarization algorithms can distill lengthy reports into concise insights farmers can readily absorb.
Overall, text mining using machine learning, combined with knowledge engineering, helps unlock the knowledge trapped in agricultural literature to augment farmer skills.
Machine learning approaches in agriculture
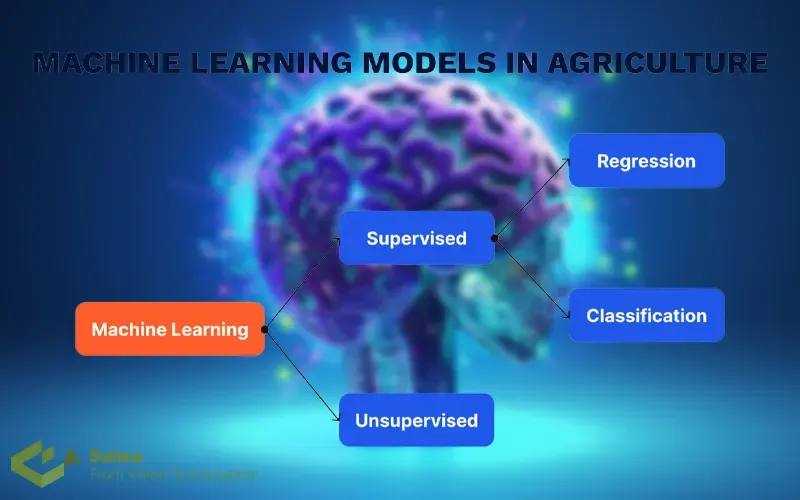
Machine learning applications in agriculture are transforming traditional farming methods. ML models are classified into supervised and unsupervised learning paradigms, depending on the nature of the training data.
Supervised models
Regression algorithms excel at predicting continuous values. Between machine learning applications in agriculture, they can forecast crop yields by considering factors such as historical data, weather patterns, soil composition, and management practices. This information empowers farmers to optimize resource allocation and make informed decisions. Additionally, regression models aid in selective breeding programs by identifying crops with desired traits like disease resistance or higher nutritional content.
Classification algorithms, another cornerstone of supervised learning, categorize data into predefined classes. In the agricultural context, they can be employed to classify crop varieties, detect plant diseases, or predict pest outbreaks. Early plant disease identification enables timely interventions, preventing significant crop losses.
Unsupervised models
These models uncover hidden patterns within data without prior labeling. By applying clustering algorithms, farmers can group plants based on similar characteristics, genetic makeup, or environmental preferences. This knowledge facilitates precision agriculture practices, such as targeted fertilization or irrigation. Anomaly detection, another unsupervised technique, helps identify unusual patterns that may indicate potential problems, such as soil nutrient deficiencies or irrigation issues.
The integration of machine learning applications in agriculture is poised to revolutionize the industry. By harnessing the power of data and advanced algorithms, farmers can enhance productivity, sustainability, and profitability while addressing global challenges like food security and climate change.
Deep Learning in Agriculture
A subset of machine learning seeing massive agricultural investment is deep learning. Deep neural networks composed of many layers discover layer-wise data representations. Key applications of Deep Learning for Agriculture include:
Computer vision
Deep convolutional neural networks (CNNs) accurately analyze imagery for plant identification, disease spotting, and weed mapping tasks.
Time series analytics
Recurrent neural networks (RNNs) reveal insights from temporal data like weather records, crop growth curves, and commodity prices.
Genomics
Deep learning extracts meaningful patterns from vast DNA/RNA sequencing data to support molecular breeding and microbiome manipulation.
Language processing
Techniques such as Long-Short Term Memory (LSTM) networks analyze masses of unstructured agricultural text and spoken dialog for search, translation, and decision support. Deep learning overcomes the limitations of earlier machine learning, which relied on hand-crafted data inputs and simplified models that were unable to harness agriculture's massive amounts of structured (imagery, genomics) and unstructured (language, sensors) data.
Read Also: An Over View Applications of Computer Vision in Agriculture
Applications Of Machine Learning in Agriculture
We will overview several real-world examples that demonstrate the transformative potential of machine learning in agriculture:
Reimagining Crop Management
One of the most important applications of machine learning in agriculture is crop management. Predicting crop yields, disease outbreaks, and optimal planting times is critical to efficient farming. Machine learning models can incorporate a variety of factors (weather patterns, soil conditions, historical yield data, and even satellite imagery) to make accurate predictions. Armed with this knowledge, farmers can make strategic decisions that optimize crop production while minimizing the waste of resources.
Precision Agriculture
Precision agriculture is a trendy term, and machine learning is at its core. This approach involves tailoring farming practices to the unique needs of different sections of a field. Machine learning algorithms process data from IoT devices, drones, and sensors to create detailed maps of soil characteristics, moisture levels, and crop health. These insights enable farmers to apply fertilizer, irrigation, and pesticides precisely where they are needed, reducing environmental impact and optimizing resource use.
Detecting and Preventing Crop Diseases
The ability to Crop Disease Detection early is critical to preventing their spread and minimizing economic losses. Machine learning in agriculture excels at image recognition, enabling the development of models that can distinguish between healthy and diseased crops based on images captured by drones or smartphones. These models can provide real-time insights to farmers, allowing them to take quick action and implement targeted treatment plans.
Supply Chain Optimization
Agriculture doesn't conclude at the farm gate; it extends to the entire supply chain. Machine learning algorithms can enhance logistics and distribution by predicting demand, optimizing routes, and minimizing food wastage by considering factors such as consumer preferences, market trends, and transportation constraints; machine learning-driven supply chain management ensures that produce reaches its destination in optimal condition and quantity.
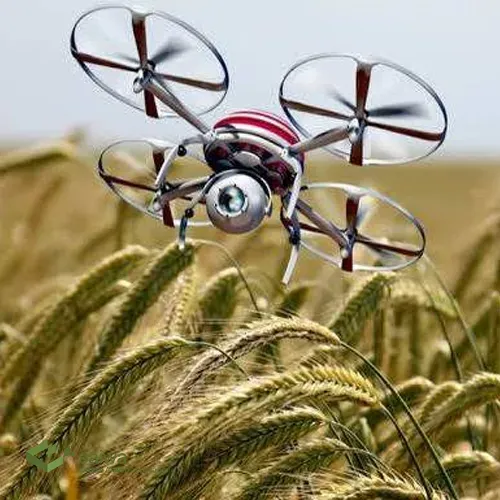
How IoT and Machine Learning are Transforming Agriculture
While machine learning offers a powerful arsenal for agricultural optimization, its effectiveness hinges on the quality and quantity of data it receives. This is where the Internet of Things (IoT) emerges as a critical enabler, acting as a pervasive sensor network that supercharges the capabilities of ML agriculture applications.
Beyond Basic Data Collection
Traditional data collection methods often rely on manual measurements or infrequent sampling, leading to static data points and potentially inaccurate information for ML agriculture. However, the deployment of IoT sensors across the agricultural landscape – embedded in soil, crops, and machinery – fundamentally transforms this dynamic. These sensors continuously gather real-time data on a multitude of parameters, encompassing soil moisture levels, nutrient content, minute changes in plant temperature, and light absorption rates.
Hyper-local Environmental Mapping
Dense sensor networks strategically placed throughout fields create a hyper-local environmental map. This unveils microclimates within a single field, allowing for the application of water and fertilizers with pinpoint accuracy. This optimization minimizes waste and environmental impact while maximizing resource efficiency. However, field mapping can be done leveraging AI drones for precise farming as well.
Micro-Precision: Moving Beyond Averages
The deployment of IoT sensors facilitates a shift towards micro-precision in ML agriculture. Dense networks of sensors create detailed maps of environmental variations within a single field. This empowers farmers to tailor resource application to the specific needs of each micro-zone, leading to significant reductions in waste and a more ecologically sustainable approach to farming. This fine-grained data allows ML agriculture to not only predict overall yield but also identify areas that might underperform due to specific deficiencies.
Machine Learning and Agriculture Sustainable Practices
As the world grapples with the pressing challenges of climate change, environmental degradation, and the need for sustainable food production, machine learning and agriculture have emerged as powerful tools to drive sustainable practices in the agricultural sector. Machine learning algorithms can analyze vast amounts of data from various sources, including weather patterns, soil composition, crop yields, and resource utilization, to identify areas where sustainability can be improved. By leveraging this data-driven approach, farmers can optimize their operations, reducing waste, minimizing the use of harmful chemicals, and promoting more eco-friendly farming methods.
One of the applications of machine learning and agriculture in sustainable practices is precision agriculture. Through the use of advanced sensors, drones, and satellite imagery, machine learning systems can precisely monitor crop growth, soil conditions, and water usage, enabling farmers to apply inputs such as fertilizers, pesticides, and water in a targeted and efficient manner. This not only reduces the environmental impact of agricultural activities but also contributes to better resource management and increased profitability for farmers.
Machine learning and agriculture can play a crucial role in promoting regenerative agriculture practices, which focus on rebuilding soil health, enhancing biodiversity, and sequestering carbon. Machine learning algorithms can analyze complex data patterns and provide insights into optimal crop rotations, cover cropping strategies, and the integration of agroforestry systems. By embracing these sustainable practices of machine learning and agriculture, farmers can not only improve the long-term productivity of their land but also contribute to mitigating the effects of climate change and preserving the natural ecosystem.
Predictive Analytics for Crop Yield Forecasting
Predictive analytics have transformed crop yield forecasting, providing farmers with critical insights for planning and resource management. Machine learning in agriculture is an integral part of this transformation. Traditional methods often rely on historical data and expert intuition, but these approaches can be limited by their inability to account for complex and interacting factors. In contrast, machine learning in agriculture has the capacity to analyze vast datasets, include:
Historical weather data: Rainfall, temperature, humidity, and sunlight patterns over many years.
Soil characteristics: Nutrient levels, pH, moisture content, and soil type.
Crop data: Variety, planting date, fertilizer application, and pest/disease incidence.
Remote sensing data: Satellite imagery providing information on crop health, growth stage, and stress levels.
By training on this diverse data, machine learning algorithms can identify complex relationships and patterns that are not readily apparent to humans. This allows for more accurate and timely predictions of crop yields. Machine learning in agriculture helps farmers to make informed decisions regarding:
Planting strategies: Choosing the optimal crop variety and planting time based on predicted weather and soil conditions.
Resource management: Optimizing fertilizer and irrigation schedules to maximize yields and minimize waste.
Harvest planning: Anticipating harvest dates and yields to arrange for labor, storage, and transportation.
Market strategies: Making informed decisions about pricing and sales based on yield forecasts.
The application of machine learning in agriculture for yield prediction is not without its challenges. Models must be validated and calibrated regularly to ensure accuracy. However, as data collection and analysis techniques improve, predictive analytics will become an even more powerful tool for optimizing crop production and ensuring food security.
Read More: Predictive Analytics in Agriculture | Fields of Insight
Machine Learning Models Used in Agriculture for a Fresher Future
Imagine biting into a perfectly ripe strawberry, even if it was picked weeks ago. That's the potential of machine learning models used in agriculture! These powerful tools aren't just for growing crops – they can also revolutionize how we store and transport food, minimizing waste and keeping things fresher for longer.
Food loss and waste are major problems, with a significant portion of food spoiling before it reaches consumers. Machine learning models used in agriculture can change that. By analyzing past data on factors like temperature, humidity, and even how food is handled, these models can predict how quickly a specific batch of produce will deteriorate.
Furthermore, these models can be used to create dynamic storage recommendations. Machine learning models used in agriculture can recommend the ideal storage conditions for each batch. They can analyze real-time data from sensors in warehouses and trucks, suggesting adjustments to temperature, humidity, or even the atmosphere to extend shelf life. This not only reduces waste but also ensures tastier, higher-quality produce reaches your table.
Machine learning models used in agriculture can also play a role in optimizing packaging and transportation. By analyzing data on factors like vibration, temperature fluctuations, and travel time, these models can help design packaging that minimizes damage and spoilage during transport. Additionally, they can be used to create optimized delivery routes that minimize travel time and ensure produce arrives at its destination in peak condition.
By implementing these solutions powered by machine learning models used in agriculture, the entire food supply chain can become more efficient and sustainable. This translates to reduced food waste, higher profits for farmers and retailers, and ultimately, fresher, more nutritious food for consumers.
Ethical Considerations in Machine Learning in Farming
The rapid advancement of machine learning in farming has ushered in a new era for agriculture, promising increased efficiency, productivity, and sustainability. While the potential benefits of machine learning in farming are immense, it's crucial to address the ethical implications associated with this technology.
Data Privacy
The efficacy of machine learning in farming algorithms hinges on vast datasets often brimming with sensitive information about farming practices, soil composition, crop yields, and financial details. A data breach could lead to severe consequences, including financial ruin, reputational damage, and compromised competitive advantage. To safeguard this critical asset, robust data protection measures are imperative. This entails meticulous data handling, including minimizing collection, anonymizing personal information wherever feasible, employing robust encryption, strictly controlling data access, and conducting regular security audits to identify and rectify vulnerabilities.
Job displacement
Machine learning in farming undoubtedly enhances efficiency, so it's crucial to consider the human element. A proactive approach is essential to mitigate the negative repercussions of automation. This involves equipping farmers with the necessary skills to navigate the evolving technological landscape through retraining and upskilling programs. Creating new roles synergizing human expertise with machine learning capabilities can also help. Additionally, establishing social safety nets for farmers impacted by job displacement is crucial.
Environmental impact
While machine learning in farming holds the potential to optimize resource utilization and reduce environmental damage, it could inadvertently exacerbate existing problems if not carefully considered. Sustainable practices must be at the core of machine learning in farming applications. A comprehensive life cycle assessment of these systems, from development to disposal, is essential. Precision agriculture, enabled by machine learning, can optimize resource inputs, reduce waste, and minimize environmental harm. Moreover, preserving biodiversity and ecosystem health should be paramount.
Addressing Common Misconceptions in Machine Learning in Agriculture
As with any transformative technology, the integration of machine learning in agriculture has been accompanied by certain misconceptions. It's crucial to address these to foster realistic expectations and encourage wider adoption. Let's clarify some common misunderstandings:
Machine Learning is Exclusively for Large-Scale Operations: While large agribusinesses can leverage complex ML systems, the technology is increasingly accessible to smaller farms. Machine learning in agriculture is not a monolithic entity; it encompasses a spectrum of tools, from sophisticated predictive models to simple, smartphone-based applications for tasks like pest identification or soil moisture assessment.
Implementing Machine Learning Requires Extensive Technical Expertise: The narrative that one needs advanced data science skills to benefit from ML is misleading. The agricultural technology sector is rapidly developing user-friendly platforms and interfaces that abstract away the underlying complexity. The emphasis is on delivering actionable insights to farmers, not requiring them to become algorithm experts.
Machine Learning Will Entirely Replace Human Judgment: This is a fundamental misunderstanding of the role of AI in agriculture. Machine learning in agriculture is designed to augment human decision-making, not supplant it. Algorithms process vast datasets and identify patterns that would be impossible for humans to discern, providing data-driven insights.
The Cost of Machine Learning is Prohibitively High: While sophisticated systems can involve significant investment, a broad range of cost-effective solutions are emerging. The return on investment, through increased yields, optimized resource use, and reduced losses, often justifies the initial expenditure. Moreover, the availability of cloud-based services and mobile applications is making machine learning in agriculture increasingly accessible and affordable for farms of all sizes.
Integrating Machine Learning with Crop Modeling
Machine learning algorithms is also used in crop modeling which offer a transformative approach to agriculture, leveraging data-driven insights to optimize crop management decisions, enhance yields, and improve resource utilization. This powerful approach combines the strengths of both methodologies, paving the way for more productive and sustainable farming practices.
The benefits of integrating these two fields are significant:
Enhanced Model Accuracy: Machine learning models can improve the accuracy and efficiency of traditional crop models. ML excels at incorporating large datasets and capturing complex, non-linear relationships that may be difficult for conventional models to represent, leading to more precise predictions.
Contextual Understanding: Crop models, in turn, provide a vital framework rooted in physiological and environmental processes. This helps in interpreting the results from machine learning algorithms, ensuring that data-driven insights are grounded in scientific understanding of crop growth and yield.
This integration results in more robust and interpretable predictions. For instance, hybrid frameworks use ML to calibrate or refine crop model parameters, or to integrate diverse data from sources such as satellite imagery and weather stations. These approaches have demonstrated efficacy in enhancing yield predictions, optimizing irrigation, and evaluating the impacts of climate change. The advancement of these hybrid models holds great promise for the enhancement of agricultural efficiency and resilience.
Read More: Machine Learning and Crop Modeling | Innovations in Agricultural Technology
Future Trends in Machine Learning and Agriculture
Machine learning has been instrumental in driving innovation and sustainability in the agricultural sector. Key trends, such as quantum computing, hyper-personalized farming, edge computing, and blockchain integration are set to redefine machine learning in farming.
Hyper-Personalized Farming
Machine learning in farming is undergoing a shift towards hyper-personalization, where algorithms analyze data from individual plots to provide tailored recommendations. This enables farmers to optimize water usage, fertilizer application, and crop selection. This trend, predicated on precision, fosters sustainable agriculture and enhances productivity.
Integration of Edge Computing
Edge computing complements machine learning in farming by bringing data processing closer to the source—farm equipment and IoT sensors. This reduces latency, enabling real-time insights for autonomous machinery, irrigation systems, and pest control measures. By integrating edge devices with machine learning, farmers gain actionable insights without relying heavily on cloud infrastructure.
Quantum Computing
Quantum computing will enhance machine learning in farming by solving complex optimization problems faster than traditional computers. Tasks like weather forecasting, crop simulation, and supply chain logistics will see dramatic improvements, helping farmers make informed decisions even in unpredictable conditions.
Read Also: Quantum Machine Learning | A New Frontier for AI
Blockchain-Enhanced ML for Transparency
The combination of blockchain and machine learning ensures transparency across the food supply chain. From farm to table, every step can be tracked and verified, reducing fraud and inefficiencies. This innovation not only boosts consumer trust but also empowers farmers to receive fair value for their produce.
Generative AI for Crop Design
Generative AI is emerging as a tool for designing crops optimized for specific needs, such as drought resistance, pest tolerance, and higher yields. By analyzing genetic data and environmental conditions, these models propose ideal plant traits, speeding up the crop breeding process. This trend has the potential to address food security challenges in the face of climate change. Generative models can also simulate the effects of various inputs like fertilizers, pesticides, or irrigation methods, enabling better decision-making.
Digital Twin Technology
Digital twin technology, powered by machine learning, is gaining traction in agriculture. A digital twin replicates a farm's physical environment, allowing farmers to simulate various scenarios before implementing changes. These virtual environments help optimize planting schedules, water management, and pest control strategies. Farmers can test new equipment and strategies virtually, saving time and resources.
Read More: The Impact of Digital Twins in Agriculture | Revolutionizing Farming
Conclusion
Advanced deep neural networks are unlocking value from vast new data sources. Machine learning in agriculture offers immense potential for addressing rising pressures on agricultural systems essential to global food security and ecological sustainability. Real-world implementations are proving capabilities to increase yields, reduce costs and environmental impacts, and build resilience.