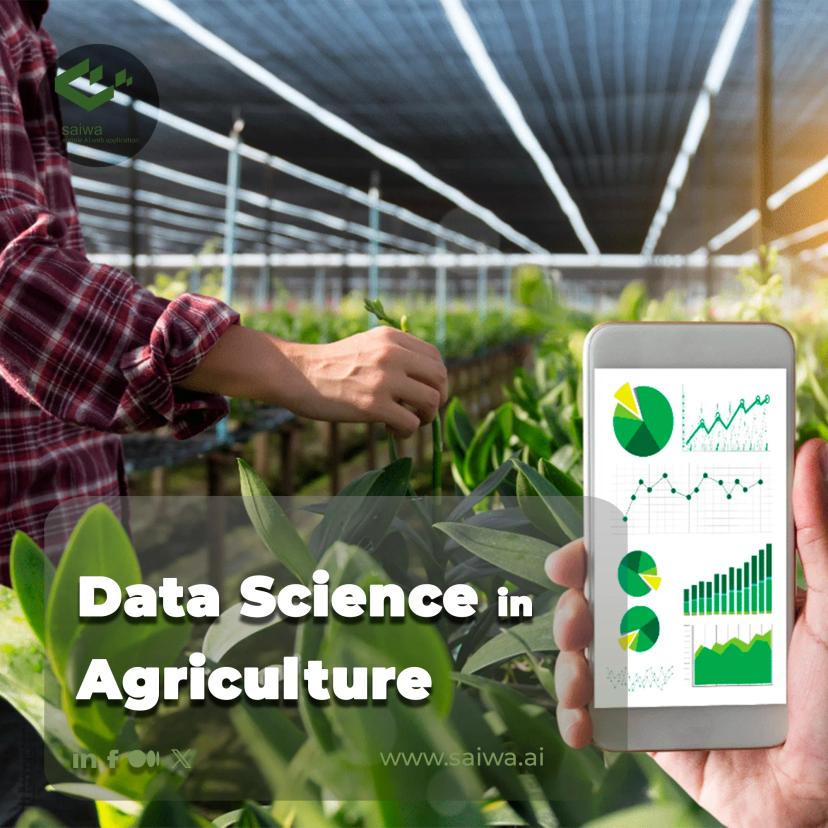
The Role of Data Science in Agriculture
The agriculture industry is in the midst of a technological revolution driven by the rise of data science and advanced analytics. Farmers, agribusinesses, and policymakers are leveraging a variety of data sources to make better strategic and operational decisions. Data science extracts insights from agricultural data using techniques like machine learning, predictive modeling, deep learning, and Big Data analytics. This enables optimization across the value chain from crop planning to post-harvest supply chains.
The integration of analytics, IoT sensors, satellite imagery, predictive models, and more is often referred to as "smart farming" or "digital agriculture." These technologies deliver benefits like higher yields, cost savings, and risk management through data-driven farming. According to the American Farm Bureau Federation, smart farming technologies could create over $47 billion in annual economic benefits for U.S. farmers by 2050. With growing populations and environmental concerns, data science in agriculture role is only set to grow.
Read Also: Practical Application & Future of AI in Agriculture
Key Data Sources Enabling Agricultural Data Science
Modern agriculture generates a vast amount of data from digital technologies that can be mined for predictive insights:
Data Collection Methods in Agriculture
On-farm sensor networks, drones, satellite imagery, weather stations, machinery telematics, and precision equipment are driving digital data collection:
IoT Sensor Networks: Low-power wide area networks connect arrays of wireless sensors that capture soil attributes, crop growth, livestock movement, etc. Companies like Semios enable these.
Drones and UAVs: Drones fly over farms collecting multi-spectral imagery for crop health assessment, field mapping, scouting, and spraying operations. Using Sairone, you can have access to different data such as drone imagery, data collection, mapping, and classification with a precise analysis for your farm.
Satellite Imagery: Public and private satellites continuously capture high-resolution spectral imagery of the earth's surface usable for agriculture applications.
Equipment Sensors: Precision agriculture equipment like self-driven tractors, combine harvesters, etc. stream operational data through gateways on parameters like yield, fuel consumption, etc.
Manual Data Collection: Handheld devices, smartphones, and surveys continue to be used to collect various agronomic data points.
Data aggregation platforms: combine these heterogeneous data sources enabling farmers to make informed decisions. Standardized data formats and APIs increase interoperability.
Read Also: AI-Based Drone Operation| AI in Drones use cases
Data Processing and Management
On-farm data volumes often run into terabytes or petabytes requiring specialized processing, storage, and analytics infrastructure:
Data Pre-processing: Raw farm data requires extensive cleaning, structuring, and normalization to prepare it for advanced analytics. Metadata management is critical for proper context.
Data Lakes: Low-cost cloud object storage holds large volumes of raw data including images, video, logs, etc. for later querying and analysis. Cataloging enables easy discovery.
Data Warehouses: Structured data is modeled and loaded into analytical databases and data warehouses to simplify aggregation, reporting, and visualization. Optimization is key for fast queries.
Data Security: Farm data contains sensitive personal and financial information requiring access controls, encryption, audit trails, and other cybersecurity measures to mitigate risks.
Cloud technology enables flexible, scalable agricultural data platforms that can handle specialized processing and storage needs. Emerging techniques accelerate analytics.
Applications of Data Science in Agriculture
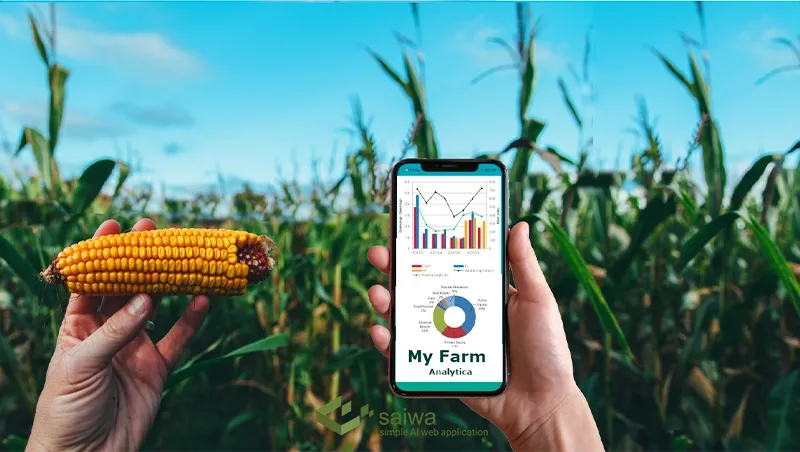
Data science in agriculture techniques enables accurate predictions for optimizing production and extracting maximum value across the agricultural value chain:
Precision Agriculture
Predictive modeling of soil conditions, pests, weather, and crop growth patterns enables site-specific application of water, fertilizers, and pesticides for higher yields.
Livestock Management
Connected sensors on cattle monitor health metrics and behavior while machine vision classifies traits like weight, mobility, and body condition score.
Crop Health Monitoring
Deep learning models identify early signs of disease through vision analytics on drone/satellite imagery while predictive models forecast future outbreaks.
Supply Chain Logistics
Analytics optimize storage, transport, and distribution networks by estimating future supply/demand, monitoring spoilage risks, and improving traceability.
Farm Automation
Self-driving tractors analyze sensor data to optimize routes, navigate obstacles, and run equipment programs. Robotics pick fruits and pack orders efficiently.
Decision Support
Predictive and prescriptive models guide optimal seed selection, land allocation, planting schedules, and other strategic decisions for greater profitability.
These cutting-edge solutions maximize agricultural productivity while minimizing waste and costs through targeted interventions based on empirical observations.
Read Also: Data-Driven Agriculture | A New Era in Smart Farming
Challenges With Agricultural Data Science
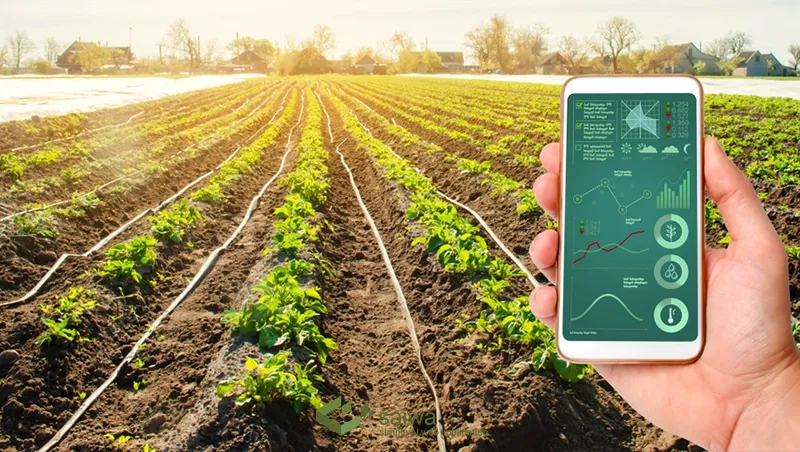
While the potential is immense, the widespread adoption of agricultural data science faces some key obstacles:
Data Availability & Quality
Many farms lack connected infrastructure to capture digital data or face integration issues between disparate sources. Data quality also suffers from errors in labeling, duplication, incompleteness, etc.
Technology Cost & Skills
The capital costs of hardware like sensors, drones, and farm equipment, combined with software and data storage can be prohibitive for small farms. Advanced data skills are also scarce.
Lack of Trust & Resistance
Many farmers lack trust in the prescriptions generated by "black box" AI/ML models and prefer relying on traditional agronomic knowledge. Change management is key for technology adoption.
Privacy & Data Ownership
There are concerns about data access, sharing, and monetization. Clear policies are needed to safeguard farmer interests while enabling analytics.
Resolving these challenges requires collaboration between agricultural producers, technology providers, agronomists, data scientists, and policymakers. Public-private partnerships can steer sustainable innovation. Understanding the sensitive nature of farm data, Saiwa prioritizes data security and privacy. We implement robust anonymization, encryption, and access control measures to safeguard crucial details such as financial information, field locations, and input utilization. Saiwa ensures that farmers' interests are protected throughout the AI-driven agricultural journey.
Our collaboration with equipment manufacturers establishes supportive policies, and fosters public-private collaborations. Saiwa envisions a future where technology adoption in agriculture is not only feasible but also sustainable in the long run.
Future Trends
Here are some of the emerging areas that will shape the future of data science in agriculture:
Generative AI: Sophisticated language AI models can enable farmers to receive vocal advice, explanations on recommendations, summarize insights from complex datasets, etc. reducing the skills barrier.
Synthetic Data: Simulations using digital twins feed generative adversarial networks to create photorealistic synthetic datasets when real data is insufficient. Overcomes data scarcity and privacy concerns.
Reinforcement Learning: These AI/ML models "learn" optimal strategies to achieve objectives through experimentation and feedback. Useful for discovering tailored strategies in complex agriculture scenarios.
Continued investments in research along with public-private collaborations can help realize data science's full potential to transform sustainability and food security.
Conclusion
data science is rapidly emerging as a critical enabler for the agriculture industry to achieve higher efficiency, profitability, and sustainability. By harnessing the power of artificial intelligence, machine learning, big data analytics, and more, farms and agribusinesses can unlock unprecedented value from the rapidly growing digital datasets available today. From precision crop management and livestock health monitoring to optimized supply chains and strategic decisions, technologies like data science in agriculture are providing granular, predictive, and prescriptive insights.
While challenges like data quality, technology access, skills shortages, and trust deficit need to be addressed, there is no doubt that agriculture is on an irreversible path toward complete digital transformation. Future innovations in generative AI and reinforcement learning with other advanced technologies will further accelerate this transition in the coming decade. The full potential of data science in agriculture can only be realized through collaboration among all agricultural stakeholders.