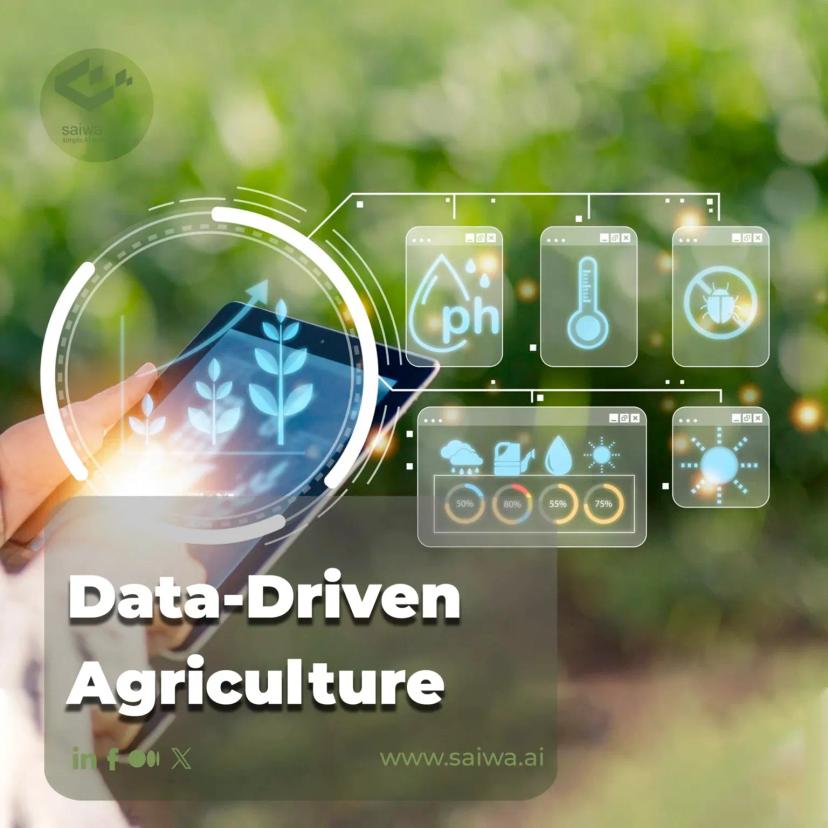
Data-Driven Agriculture | A New Era in Smart Farming
Data-driven agriculture involves analyzing the relationship between inputs and farm outputs, as well as the interactions between farm variables. Artificial intelligence algorithms are used to effectively manage the complexity of agricultural data. This article discusses data-driven agriculture. Stay with us until the end to learn about its potential.
What is data-driven agriculture?
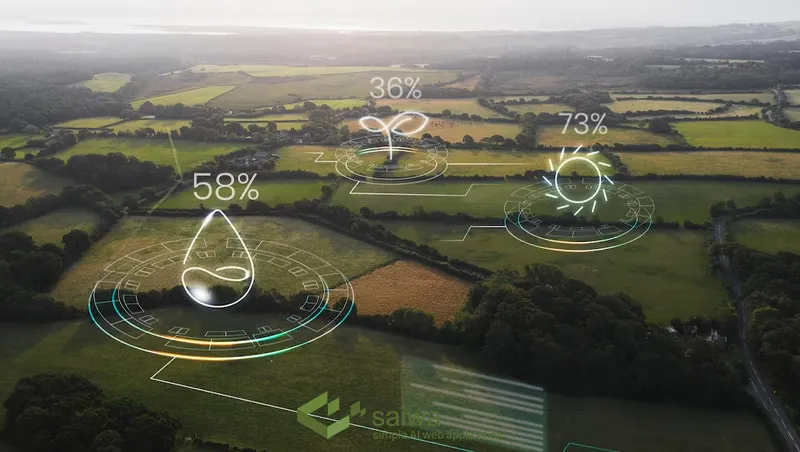
Data-driven agriculture refers to maximizing insights from data to achieve a sustainable return on investment. Precision agriculture has enabled the collection of various types of data from farms, despite the complexity of agricultural processes and the numerous variables involved. A data-driven approach provides farmers and growers with actionable information extracted from data to optimize their practices.
How is data used in agriculture?
Precision agriculture generates a large amount of data, making it challenging to extract useful insights. Allow us to illustrate this complexity with an example: The impact of nitrogen fertilizer, water, and herbicide on yield is well-established. By holding all other factors constant, one can determine the optimal amount of each input to maximize returns.
However, in agriculture, it is impossible to keep all other factors constant. Variables change simultaneously and are interdependent. Rain and irrigation can wash away nitrogen and herbicides, affecting their effectiveness. Weeds compete with plants for water absorption and also take up nitrogen from the soil. Due to the complexity of agriculture, data-based decision-making is not a simple task, even with all the data collected.
How does artificial intelligence help data-driven agriculture?
Artificial intelligence algorithms are designed to effectively handle complex data, finding hidden patterns and predicting outcomes to enable proactive, data-driven decision-making. Reliable machine learning models are created through a data-driven process, similar to that of agriculture. This step transforms artificial intelligence insights into actionable intelligence for decision-making.
Read More: Practical Application & Future of AI in Agriculture
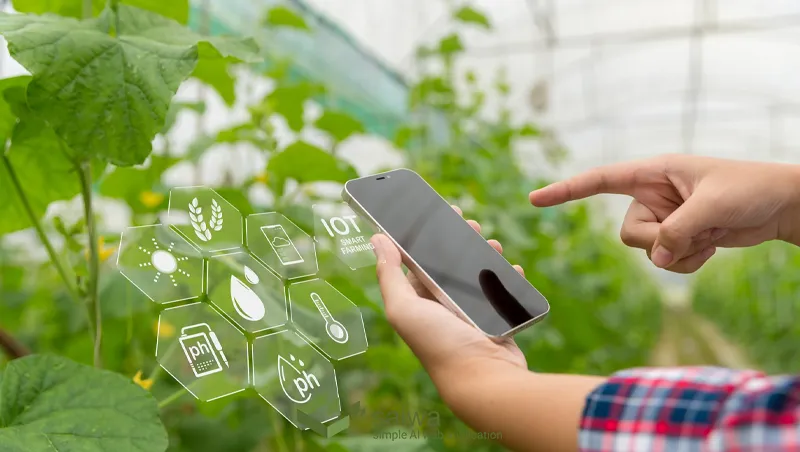
The benefits of data-driven agriculture
Data-driven agriculture has numerous benefits that can significantly impact the agriculture industry. This section will explore some of these benefits.
Precision farming
Data-driven agriculture enables precision farming techniques and farmers can adjust their practices based on specific crop needs. By analyzing data related to crop health, soil moisture, and nutrient levels, farmers can apply fertilizers, pesticides, and water where and when they are needed. This is a targeted approach and leads to a reduction in waste, as well as minimizing the environmental impact and improving the overall quality of the product.
Read Also: Revolutionizing Agriculture | Enhanced Soil Monitoring Using AI Insights
Predictive analytics
Data analytics tools can provide farmers with valuable insights into future crop performance. Predictive analytics can identify potential threats, such as pest or disease outbreaks, enabling farmers to take preventive measures promptly. This proactive approach reduces crop losses and increases yield.
Resource optimization
Data-driven agriculture enables efficient resource management. By analyzing data, farmers can optimize the use of water, fertilizer, and other resources, minimizing waste and costs. This approach promotes sustainable farming practices and is economically beneficial for farmers.
What kind of data can be used in agriculture?
Weather data: temperature, humidity, rainfall, wind speed, and solar radiation. These factors can help predict the yield of the crop and determine the right time for planting, harvesting, and irrigation.
Soil information: soil type, moisture, pH, and nutrient content. Soil data helps to choose the right crops, optimize fertilizer use, and manage soil health.
Crop data: crop types, planting dates, growth stages, pest or disease incidents. These data can help to choose the appropriate crop rotation, monitor crop health, and apply targeted control measures against pests and diseases.
Satellite and Aerial Images: Remote sensing data from satellites and AI drones including growth, stress, and general field conditions can lead to precision farming practices such as variable rate technologies, targeted irrigation, and pest location. and guide diseases in their early stages.
Machinery and equipment data: tractors, harvesters, and sensors equipped with GPS. Monitoring this data can monitor machine performance, schedule maintenance, and reduce downtime.
Market and economic data: prices of agricultural products, supply-demand trends, and attention to industry trends can help farmers make informed decisions in product selection and marketing strategies.
Data Driven Insights for Ranching to Protect Your Land with Fire Risk Management
Wildfires pose a significant threat to ranches, jeopardizing crops, infrastructure, and the land's overall health. Fortunately, data driven insights for ranching can empower ranchers to become proactive in fire risk management.
By leveraging data on historical fire occurrences, weather patterns, and vegetation types, ranchers can create fire risk maps that pinpoint areas most susceptible to blaze outbreaks. Data driven insights for ranching allow for a targeted approach, enabling ranchers to prioritize fuel reduction efforts in high-risk zones. This may involve prescribed burns conducted during controlled periods, carefully managed grazing strategies to reduce fuel loads, or the strategic removal of invasive plant species that act as fire accelerants.
Data driven insights for ranching extend beyond preventative measures. Real-time weather data integrated with fire risk maps provides invaluable information during critical fire seasons. Ranchers can monitor wind speed, humidity levels, and lightning strike data, allowing them to act early and activate emergency protocols if necessary.
Investing in data-driven fire risk management empowers ranchers to become active stewards of their land. By harnessing the power of data, ranchers can significantly reduce the risk of devastating wildfires, protecting their valuable resources and ensuring the long-term sustainability of their ranches.
Challenges
Some challenges could slow the adoption and wider use of data in agriculture. These challenges include the following:
Data quality and accuracy: Wrong or incomplete data can cause poor decisions and create problems in agricultural products.
Data privacy: Farmers may be hesitant to share their data due to privacy concerns. Farmers should have the right to own and monetize their data when it is used by others.
Integration and Interoperability: Combining data from different sources and extracting real insights can be a complex task that requires specific training and technical support.
Skills gap: A lack of skilled people to analyze and use data in a useful way can limit the benefits that data-driven agriculture can offer.
Data can have a huge impact on the way agriculture works. It also has the potential to enable farmers to take a proactive versus reactive approach to crop management, resulting in more sustainable and efficient practices.
The future of data-driven agriculture
The future of data-driven agriculture is very promising, and technological advances and increased access to data are expected to bring about a greater transformation in agricultural practices. In this section, we will mention the key developments in this field:
Internet of Things integration
The integration of IoT devices provides farmers with more information and enables comprehensive and accurate analysis. Automated irrigation systems, smart sensors, and connected machinery work seamlessly together to optimize agricultural operations and increase crop yields.
Advanced predictive analytics
With the help of improved analysis tools and algorithms, farmers can predict crop performance more accurately. This gives them the ability to make data-driven decisions, anticipate market demands, and optimize yields accordingly.
Robotics and automation
Robotics and AI and automation systems play a very important role in data-driven agriculture. Robots equipped with artificial intelligence can do tasks such as planting, harvesting, and weed control more efficiently and accurately than manual methods. This increase in efficiency will result in higher crop yield and reduce the labor-intensive burden for farmers.
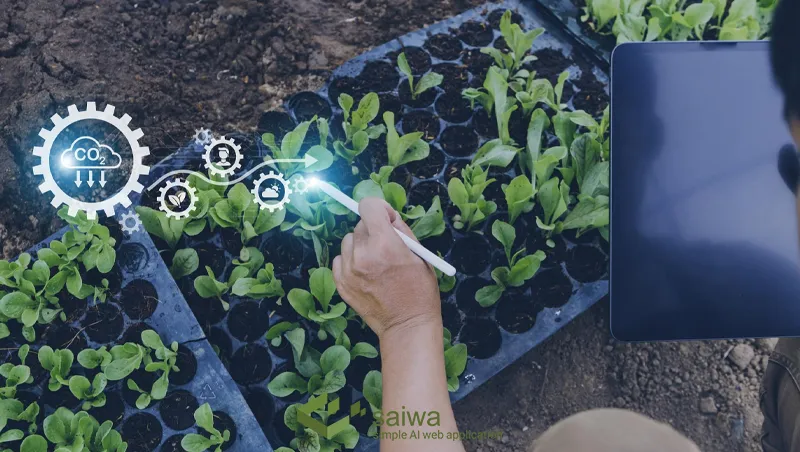
Conclusion
The integration of artificial intelligence in data-driven agriculture is transforming the industry. Despite challenges such as data quality and privacy concerns, the benefits of enhanced sustainability, reduced waste, and increased yields highlight the importance of embracing this technological evolution. The integration of IoT devices, advanced analytics, and robotics promises a more efficient, sustainable, and productive agricultural landscape. This evolution empowers farmers to make informed decisions, navigate complexity, and meet the challenges of an ever-changing agricultural environment.