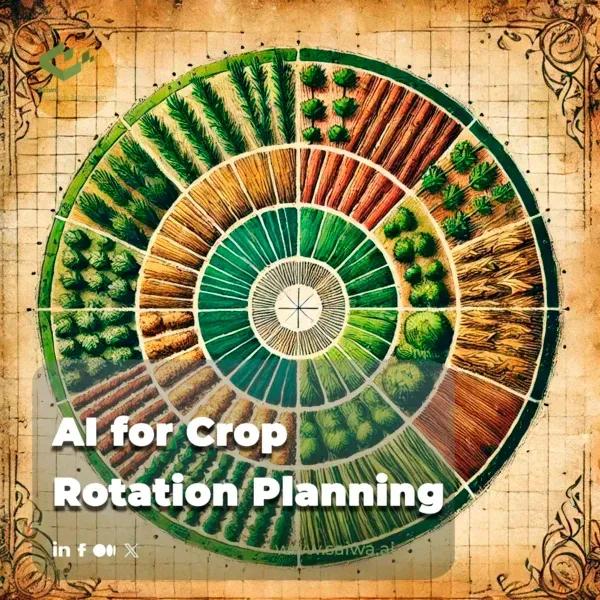
AI for Crop Rotation Planning | Transforming Sustainable Agriculture
Crop rotation, a cornerstone of sustainable agriculture, has been practiced for centuries to improve soil health, increase crop yields, and minimize pest and disease pressure. Traditional crop rotation planning relies on established knowledge, farmer experience, and regional guidelines. However, these methods often lack the precision and adaptability needed to address the increasing complexities of modern agriculture, including climate change, resource scarcity, and evolving pest dynamics. The advent of artificial intelligence (AI) offers a transformative opportunity to revolutionize crop rotation planning, enabling data-driven decision-making, optimizing resource use, and promoting sustainable agricultural practices.
Sairone, developed by Saiwa, is an advanced AI and machine learning platform specifically tailored for agricultural applications. By leveraging drone technology, Sairone provides detailed insights into crop detection and nitrogen content estimation.
This technology enables farmers to optimize crop sequences and soil management, directly linking Sairone's capabilities to the enhancement of AI-driven crop rotation planning. This integration enables precise, data-driven decisions that adapt to the complexities of modern agriculture.
This article explores the intersection of AI for Crop Rotation Planning, examining the techniques, applications, challenges, and emerging trends in this rapidly evolving field.
Understanding Crop Rotation
What is crop rotation?
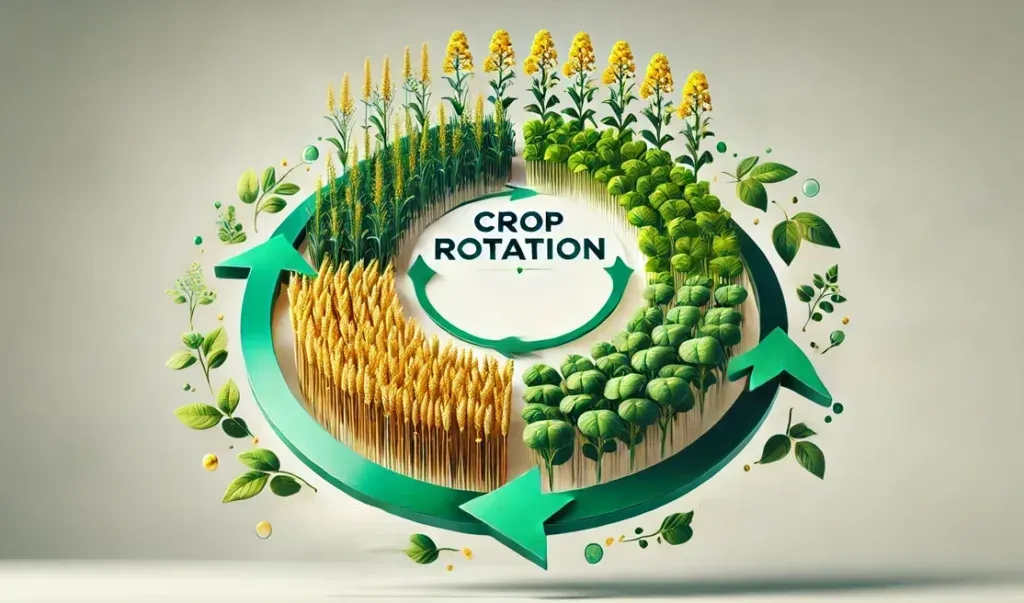
Crop rotation is the practice of growing different types of crops in a planned sequence on the same piece of land over several years. This practice contrasts with monoculture, where the same crop is grown repeatedly, depleting soil nutrients and increasing susceptibility to pests and diseases.
Crop rotation offers various benefits, including improved soil health, enhanced nutrient cycling, reduced pest and disease pressure, increased crop yields, and improved water use efficiency. Different crops have different nutrient needs and root systems, contributing to a more balanced and resilient soil ecosystem. For example, legumes such as beans and peas fix nitrogen in the soil, benefiting subsequent crops such as corn or wheat.
Why AI is a Game-Changer in Crop Rotation Planning
Traditional crop rotation planning often relies on generalized recommendations and historical data, which may not be optimal for specific field conditions or evolving environmental factors. AI offers a paradigm shift by enabling data-driven crop rotation planning that considers various factors, including soil properties, weather patterns, pest and disease prevalence, market demands, and farmer preferences.
AI algorithms can analyze vast amounts of data to identify optimal crop sequences that maximize yields, minimize input costs, and improve environmental sustainability. This precision and adaptability are critical to meeting the challenges of modern agriculture and ensuring food security in a changing climate.
The Techniques of AI for Crop Rotation Planning
Several AI techniques are being employed to optimize crop rotation planning
Reinforcement Learning
Reinforcement learning (RL) is a type of machine learning in which an agent learns to make optimal decisions by interacting with its environment. In the context of crop rotation, the agent can be an AI algorithm that learns to select the best crop sequence for a given field based on feedback from the environment, which includes factors such as soil conditions, weather patterns, and pest pressure.
The RL agent receives rewards for positive outcomes, such as high yields and minimal environmental impact, and penalties for negative outcomes, such as crop failure or excessive resource use. Through trial and error, the RL agent learns to optimize crop rotation strategies for specific field conditions and long-term sustainability.
Machine Learning Models
Various machine learning models, such as support vector machines (SVMs), random forests, and neural networks, can be trained on historical crop data, soil properties, weather information, and other relevant factors to predict crop yields and optimize crop rotation sequences.
These models can identify complex relationships between different variables and predict the performance of different crop combinations under different conditions. For example, an ML model can predict the yield of corn following soybeans based on historical data, soil type, and expected weather patterns.
Remote Sensing and Satellite Data Integration
Integrating remote sensing data from drones and satellites with AI algorithms provides valuable insights into field conditions, crop health, and environmental factors. This data can be used to monitor crop growth, detect stress indicators, and assess the impact of different crop rotation strategies. For example, drone imagery can be used to identify areas of a field with nutrient deficiencies, allowing for targeted fertilizer application and optimized crop selection in subsequent rotations.
Applications of AI in Crop Rotation
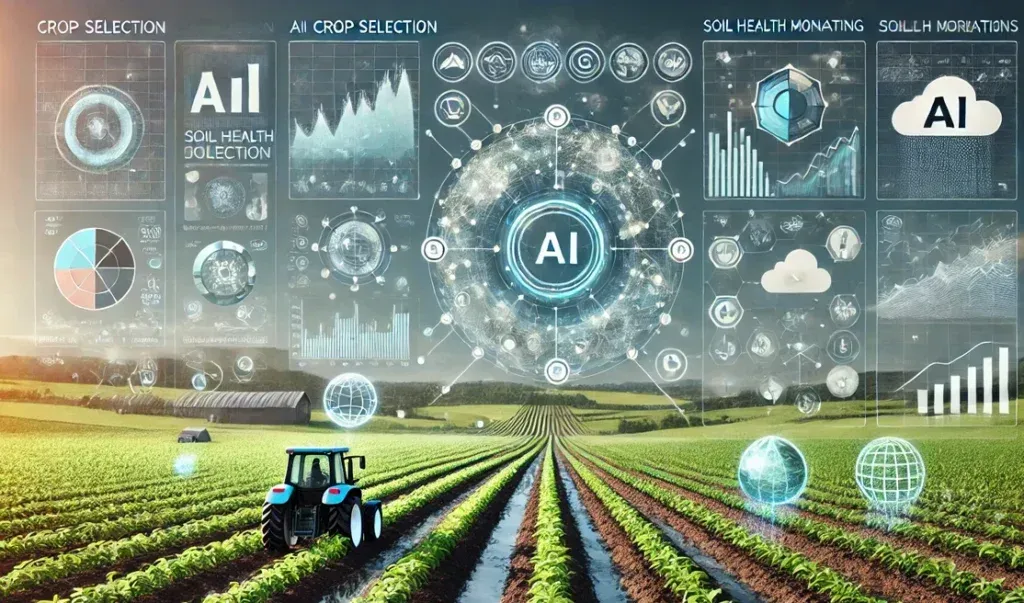
AI-powered crop rotation planning can be applied across various farming systems:
Organic Farming
AI can assist organic farmers in designing crop rotations that enhance soil health, suppress weeds, manage pests and crop disease detection without the use of synthetic chemicals. AI models can optimize the use of cover crops, green manures, and other organic practices to maximize yields and maintain soil fertility.
By analyzing soil composition, pest and disease prevalence, and historical crop performance, AI can recommend optimal crop sequences that minimize disease risk and enhance natural pest control mechanisms. This data-driven approach enables more effective and sustainable organic farming practices.
Conventional Farming
AI can help conventional farmers optimize crop rotations to maximize yields, minimize input costs, and reduce the environmental impact of chemical fertilizers and pesticides. AI-powered recommendations can guide farmers in selecting the most appropriate crops and inputs for specific field conditions and market demands.
By considering factors like soil nutrient levels, weather patterns, and pest forecasts, AI can optimize fertilizer and pesticide application, minimizing waste and reducing environmental impact while maintaining or increasing productivity. This precision approach contributes to both economic and environmental sustainability.
Field-Specific Recommendations
AI algorithms can generate field-specific crop rotation recommendations based on detailed soil analysis, historical yield data, weather forecasts, and other relevant factors. This personalized approach ensures that crop rotation plans are tailored to the unique characteristics of each field, maximizing productivity and sustainability.
By integrating data from multiple sources, including soil sensors, drones, and weather stations, AI can create highly customized rotation plans that address the specific needs of each field, optimizing resource allocation and maximizing profitability while minimizing environmental impact. This site-specific approach represents a significant advancement in precision agriculture.
Challenges in AI-Based Crop Rotation Planning
While AI offers significant potential for improving crop rotation planning, several challenges need to be addressed:
Data limitations and quality issues
AI models require large, high-quality datasets for training and validation. In many regions, access to reliable agricultural data, such as historical yields, soil properties, and weather patterns, may be limited, hindering the development and deployment of effective AI tools.
Data quality issues, such as inconsistencies, errors, and missing values, can also affect the accuracy and reliability of AI models. Furthermore, data standardization and interoperability remain significant hurdles, making it difficult to combine data from different sources and create comprehensive datasets for training robust AI models. Addressing these data challenges is crucial for developing effective AI for Crop Rotation Planning systems.
Complexity of multi-objective optimization
Crop rotation planning often involves optimizing multiple objectives simultaneously, such as maximizing yields, minimizing input costs, and reducing environmental impacts. Developing AI algorithms that can effectively balance these competing, sometimes conflicting, objectives is a complex task.
Defining appropriate weighting factors for each objective and developing algorithms that can efficiently explore the solution space to find optimal trade-offs are ongoing research areas. The inherent complexity of agricultural systems further complicates this optimization process.
Integration of AI models with traditional farming practices
Integrating AI-based recommendations with existing farming practices and decision-making processes can be challenging. Farmers need user-friendly tools and interfaces that allow them to easily access, interpret, and implement AI-generated insights.
Bridging the gap between complex AI models and practical farm management requires careful consideration of farmer knowledge, experience, and preferences. Developing intuitive and accessible decision-support systems is crucial for facilitating the adoption of AI-based crop rotation planning.
Cost and accessibility of AI tools for small-scale farmers
The cost of developing and deploying AI tools, including software, hardware, and data acquisition infrastructure, can be prohibitive for small-scale farmers, limiting their access to these potentially beneficial technologies.
Efforts are needed to make AI tools more affordable and accessible to all farmers, regardless of their farm size or economic resources. Developing open-source AI tools, providing training and support, and promoting collaborative data-sharing initiatives can help bridge this digital divide and ensure equitable access to the benefits of AI in agriculture.
Emerging Trends in AI for Crop Rotation
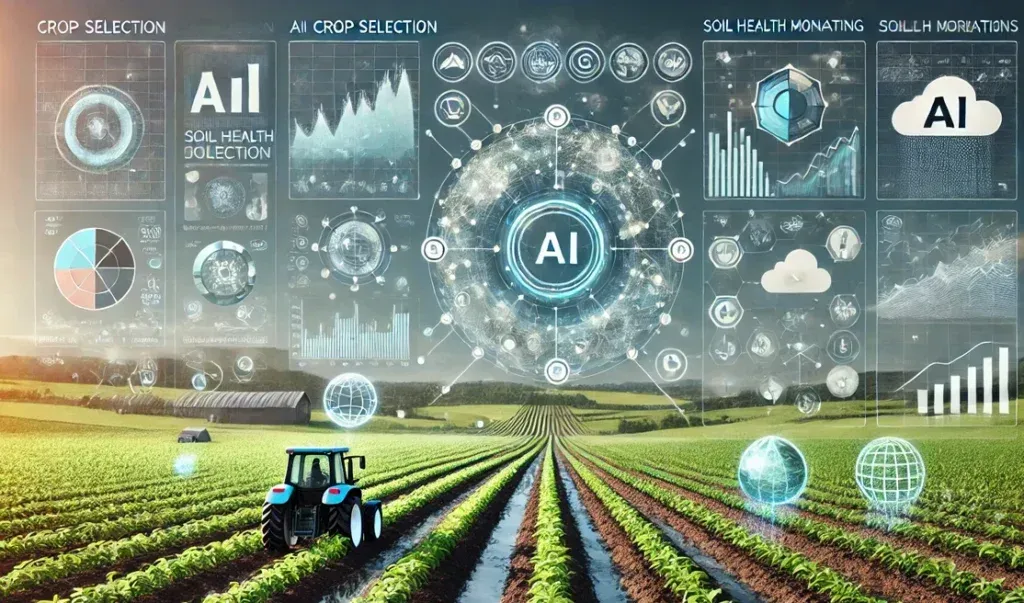
The field of AI for crop rotation is constantly evolving, with several exciting trends emerging:
Use of generative AI for Crop Rotation Planning sequences
Generative AI models, such as Generative Adversarial Networks (GANs), can be used to generate novel crop rotation sequences that may not have been considered by traditional methods. These innovative sequences could lead to significant improvements in yields, resource use efficiency, and environmental sustainability.
By exploring a large and complex solution space, generative AI can identify unconventional crop combinations that optimize multiple objectives simultaneously. This approach can lead to the discovery of more resilient and productive crop rotations, especially in the face of climate change and evolving pest pressures. Further research and development in this area has immense potential to transform agricultural practices.
Combining AI with Industry 4.0 technologies for smarter farms
Integrating AI with other Industry 4.0 technologies, such as the Internet of Things (IoT), blockchain, and robotics, can create smarter and more connected farms. This integration can enable real-time data collection, automated decision-making, and precise control of farming operations.
IoT sensors can monitor soil conditions, weather patterns, and crop health, providing valuable data for AI models. Blockchain technology can improve transparency and traceability in the food supply chain, while robotics can automate tasks such as planting, harvesting, and weeding. This convergence of technologies is paving the way for a new era of precision agriculture.
Future possibilities with hybrid AI approaches
Combining different AI techniques, such as Reinforcement Learning (RL) and Machine Learning (ML), can create hybrid AI approaches that leverage the strengths of each method. These hybrid approaches could lead to more robust and adaptable crop rotation planning systems. For instance, ML models can be used to predict crop yields based on historical data, while RL algorithms can optimize crop sequences based on real-time feedback from the environment.
This synergistic combination of AI techniques can lead to more sophisticated and effective decision-making in crop rotation planning, enabling farmers to adapt to changing conditions and optimize resource utilization. This area of research holds significant promise for the future of sustainable agriculture.
Conclusion
AI has the potential to revolutionize crop rotation planning, enabling data-driven decision-making, optimizing resource utilization, and promoting sustainable agricultural practices. By analyzing vast datasets and considering a wide range of factors, AI algorithms can generate field-specific crop rotation recommendations that maximize yields, minimize input costs, and enhance environmental sustainability.
Addressing the challenges related to data availability, model complexity, and accessibility is crucial for realizing the full potential of AI for Crop Rotation Planning and ensuring a more sustainable and resilient future for agriculture. The ongoing development and integration of AI with other emerging technologies promise to further enhance the precision and effectiveness of crop rotation planning, contributing to a more efficient and sustainable global food system.