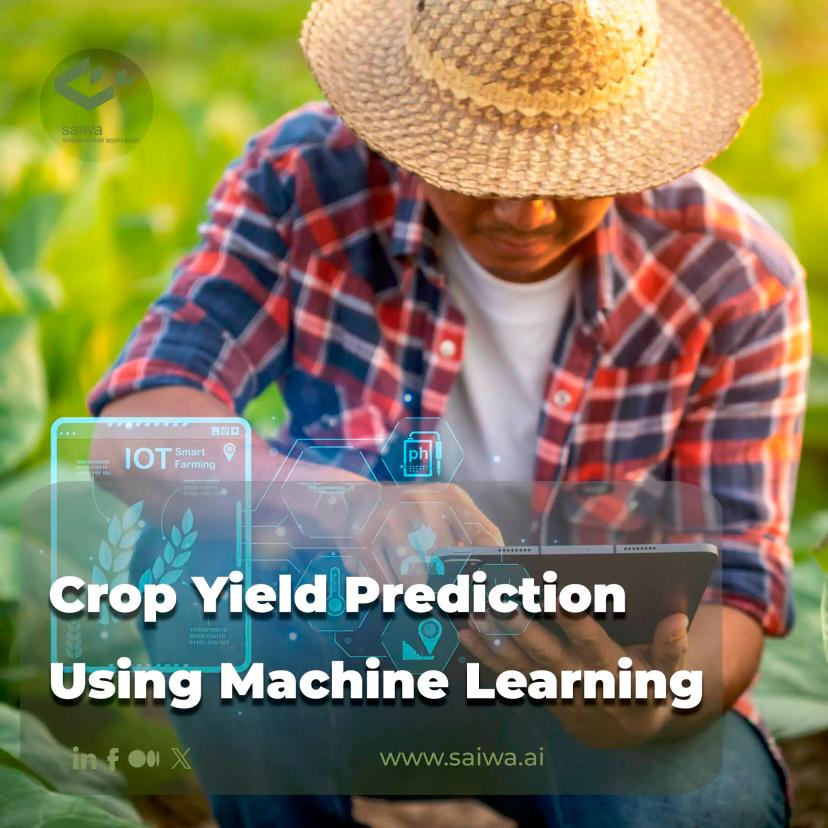
Improving Crop Yield Prediction Using Machine Learning
Accurately predicting crop yields is crucial for supporting many aspects of the agriculture industry, including optimizing cultivation practices, estimating harvest labor needs, setting commodity prices, and gauging food availability. However, traditional yield estimation methods based on crop models, expert opinion, and statistics have limitations in capturing the full complexity of how genetics, environment, and farm management interact to determine yields
Recent advances in artificial intelligence offer new opportunities to improve crop yield prediction using machine learning from historical agricultural data. Machine learning techniques can uncover subtle correlations and patterns within weather records, soil profiles, and geospatial datasets that impact crop growth and productivity.
This blog post provides an overview of machine learning techniques being applied for agricultural yield forecasting, along with real-world examples. We discuss key data sources, model evaluation strategies, potential applications, and current limitations. While still early, machine learning shows immense promise to transform future yield prediction capabilities if challenges around data integration and model generalization can be overcome.
Read Also: The impact of machine learning in agriculture
Crop Yield Prediction Using Machine Learning Models
A variety of machine learning algorithms demonstrate potential for forecasting crop yields:
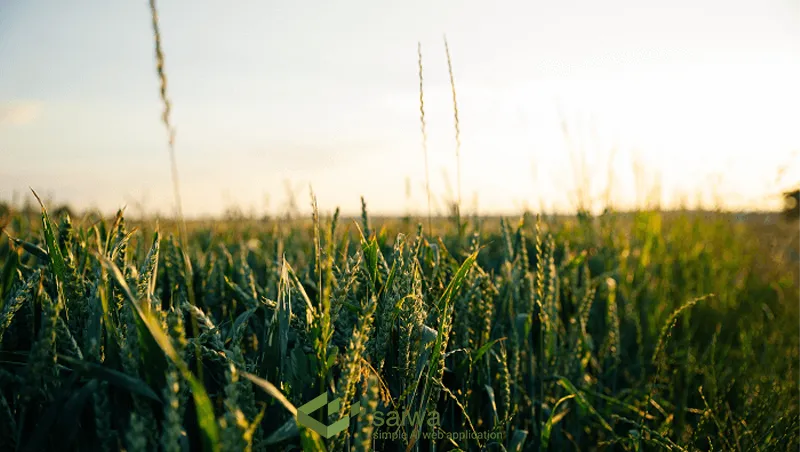
Regression Techniques
Regression models like linear regression, regression trees, and neural networks directly predict numeric yield values at a field scale based on relevant input variables. Long-term weather data, soil types, and average farm yields provide informative training data. Regression is effective at site-specific yield estimation.
Time Series Analysis
Techniques like autoregressive integrated moving average (ARIMA) model seasonal patterns and inter-annual variability in yield over time. ARIMA combined with exogenous weather regressors shows promise for anticipating the impacts of abnormal weather on critical growth stages.
Computer Vision Analysis
Processing aerial imagery collected through satellites or drones with convolutional neural networks allows estimating yield for different parts of a field based on crop appearance, vegetation indices, canopy cover, and more. This facilitates micro-scale yield mapping.
Model Ensembles
Ensemble methods integrating different modeling approaches help overcome individual limitations. For example, jointly considering computer vision detections, time series trends, and process-based crop growth models improves robustness.
Agricultural Data Sources
A wide variety of agricultural data streams provide key inputs for training and driving crop yield forecasting models. Historical weather records containing temperature, precipitation, humidity, and solar radiation information help quantify crop-environment relationships and model the impacts of weather variability on yield. Characterizations of local soil properties, including texture, pH, organic matter content, salinity levels, and water holding capacity, describe the growing medium and resource availability for crops. Satellite systems provide abundant data on crop health and vegetation vigor over the season through vegetation indices that track greenness and growth.
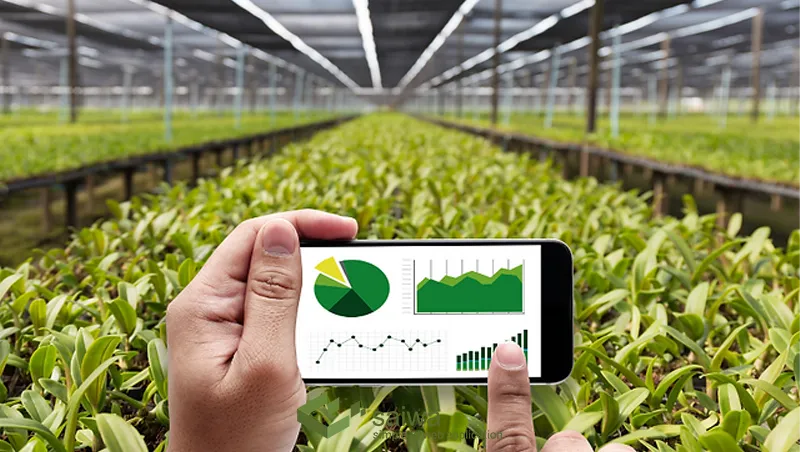
Incorporating farm management details like planting dates, irrigation and fertilizer applications, and cultivar selections adds valuable context to human factors influencing yields. Expert agronomist insights obtained through agricultural extension surveys also contribute experiential knowledge to complement raw data. Long-term regional crop yield statistics allow discerning seasonal patterns and yield change trajectories over decades. Together, these diverse data streams help capture the key factors influencing yield.
Performance Evaluation
Rigorously evaluating the accuracy and generalizability of crop yield prediction using machine learning models requires:
Error metrics like mean absolute error, root mean square error, and R-squared quantify the differences between model-predicted yields and actual observed yields. Lower errors signify better model performance.
Cross-validation techniques like k-fold validation and leave-one-year-out validation provide robust estimates of performance on new unseen data.
Geographical validation by testing on yields from regions not included in training data provides a real-world assessment of transferability.
Uncertainty quantification through confidence intervals and probability forecasts conveys the degree of certainty models have in predictions.
Comparative benchmarking against existing yield estimation methods helps quantify potential improvements over current approaches.
Applications and Benefits
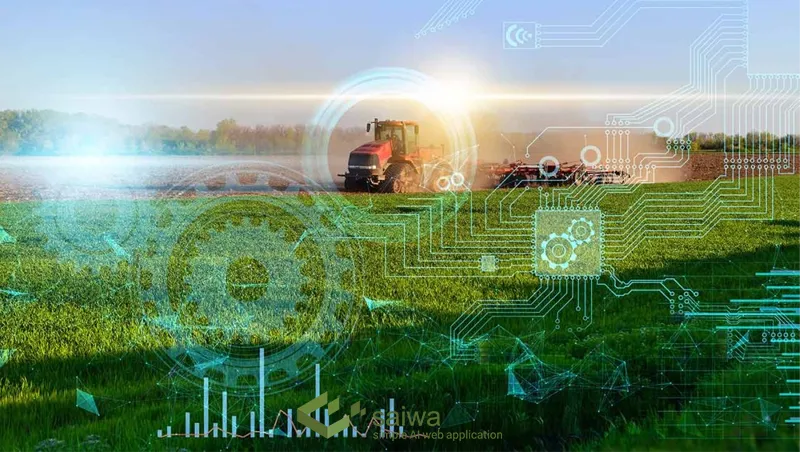
Some impactful applications of data-driven crop yield forecasting include:
Optimizing cultivation practices like planting density, irrigation schedules, and fertilizer amounts on a site-specific basis based on yield response predictions.
Anticipating regional production surpluses and shortfalls for commodity market outlooks, policy planning, and trade decisions.
Forecasting harvest labor, equipment, storage, and transportation needs based on expected yields and acreage.
Designing crop insurance products with dynamic premiums responsive to forecast the risk of yield shortfalls for a given farm.
Tracking yield impacts of conservation practices like cover crops or no-till to evaluate sustainability.
Informing agricultural land valuations and crop investment portfolio decisions based on yield forecast trajectories.
Overall, data-driven yield forecasting facilitates more anticipatory, risk-managed, and sustainable crop production.
Read Also: Practical Application & Future of AI in Agriculture
Real-Time Crop Monitoring and Decision Support
Enabling real-time crop yield prediction using machine learning assessment directly in the field can help farmers take timely actions to protect and maximize yields. With advances in agricultural IoT sensors and AI drones, it is increasingly feasible to monitor crops at much higher temporal and spatial resolutions.
Mobile Application Development
To fully leverage real-time monitoring capabilities, mobile apps need to package and present insights in an intuitive, farmer-friendly interface accessible in the field. Apps should compile and analyze data streams, flag issues, display maps, and trends, and model the impacts of interventions.
Actionable notifications, recommendations, and decision support capabilities based on real-time data help growers determine appropriate interventions around irrigation, fertilizer, pesticides, and harvesting. Apps become trusted advisors integrating AI and human expertise.
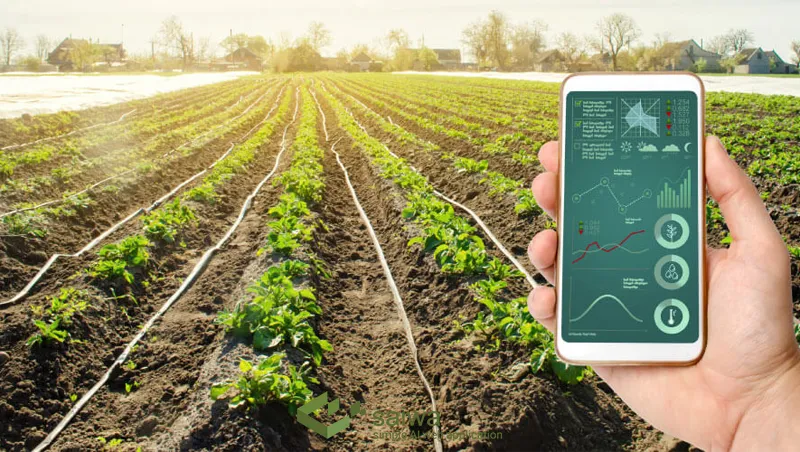
Implementation Challenges and Concerns
However, barriers to operational deployment remain:
Difficulty integrating diverse datasets with different formats, resolutions, and geospatial properties into a consistent modeling framework.
Limited availability of large labeled historical yield training data across crops, varieties, and geographies.
Inability to fully capture complex biological and ecological relationships between genotype expression and dynamic environmental conditions.
Data gaps, measurement noise, and missing values that confront models with uncertainty.
Domain expert involvement to appropriately interpret, apply, and act upon model outputs.
Ongoing research in climate science, agronomy, and machine learning aims to address these challenges and unlock the immense potential of data-driven crop yield prediction using machine learning.
Real-World Case Studies
Researchers and startups are demonstrating AI plant counting in diverse agricultural and ecological settings:
Crop yield estimation: Computer vision models count corn stalks, wheat heads, and fruit loads in imagery captured by drones flying over farms. This provides precise yield forecasts to optimize harvesting.
Forest surveys: Airborne LiDAR and hyperspectral data processed by machine learning provide detailed mapped outputs of tree densities, heights, and health to guide reforestation efforts.
Carbon stock assessment: Combining satellite image recognition with ground-based lidar scanning enables estimating biomass and carbon stored in forest areas to support carbon credit markets.
Wildlife habitat monitoring: AI-driven analysis of vegetation and plant biodiversity in aerial wildlife survey footage helps map critical habitats and food sources over time.
Invasive species tracking: Object detection models identify and map populations of invasive plants threatening ecosystems based on low-altitude UAV footage and multi-sensor fusion.
As datasets and model techniques mature, automated plant counting use cases will continue expanding across agriculture, forestry, and environmental domains.
Ethical Considerations in Crop Yield Prediction Using Machine Learning
As many benefits that crop yield prediction using machine learning provides for us, some ethical concerns must be aware of:
Fairness and Bias Mitigation
Machine learning models are only as good as the data they are trained on. Ethical concerns arise when models inadvertently perpetuate biases present in historical data, potentially disadvantaging certain farmers or regions. To mitigate bias, it is crucial to regularly audit and refine algorithms, ensuring fair and equitable predictions for all stakeholders.
Privacy Concerns
The collection and utilization of agricultural data, including farm-specific information, soil health, and crop conditions, raise valid privacy concerns. Respecting the privacy rights of farmers and implementing robust data protection measures become ethical imperatives. Anonymizing and securely managing sensitive data are essential steps in maintaining trust within the agricultural community.
Conclusion
Machine learning holds new promise for strengthening future crop yield prediction capabilities to meet growing food demands. The integration of AI-enhanced forecasting in agriculture represents a significant leap forward. Still, for crop yield prediction using machine learning, it is vital to recognize that achieving robust, generalizable approaches in this endeavor requires a holistic integration of multidisciplinary expertise, responsible data sharing, and meticulous model development. By incorporating machine learning techniques, we can unlock valuable insights from vast and diverse datasets, encompassing weather patterns, soil characteristics, crop varieties, and more. The power of AI lies in its ability to discern complex patterns and relationships within these data streams, enabling the creation of accurate yield prediction models.
Frequently asked questions
What is crop yield prediction, and how does machine learning contribute to it?
Crop yield prediction involves estimating the amount of agricultural output a farm is likely to produce. Machine learning contributes by analyzing historical and real-time data to develop predictive models that factor in various variables affecting crop growth.
What data is used for crop yield prediction with machine learning?
Data used for crop yield prediction includes historical yield data, weather patterns, soil conditions, crop type, planting density, and satellite imagery. Machine learning algorithms analyze these variables to make predictions.
How accurate are machine learning models in predicting crop yields?
The accuracy of machine learning models in predicting crop yields depends on the quality and quantity of data, the complexity of the model, and the precision of the features considered. Well-designed models can achieve high accuracy levels.
Can machine learning predict crop yields for different types of crops?
Yes, machine learning models can be trained to predict yields for various crops. The models can be customized and optimized based on the specific characteristics and growth patterns of different crops.
How does machine learning account for environmental factors in crop yield prediction?
Machine learning models account for environmental factors by analyzing data such as temperature, precipitation, humidity, and sunlight. These factors influence crop growth, and ML algorithms can identify patterns and correlations to make predictions.