Machine learning online demo
Massive amounts of data are now available everywhere. As a result, it is required to evaluate these data to extract relevant information and then develop an algorithm based on this evaluation. This may be accomplished through data mining and machine learning. Machine learning is a subset of artificial intelligence that creates algorithms based on data patterns and historical associations between data. Machine learning is utilized in various industries, including bioinformatics, intrusion detection, information retrieval, gaming, marketing, virus detection, picture deconvolution, etc. This article provides various machine learning-based technologies specifically created and developed in saiwa and also you cant try these machine learning online demos.
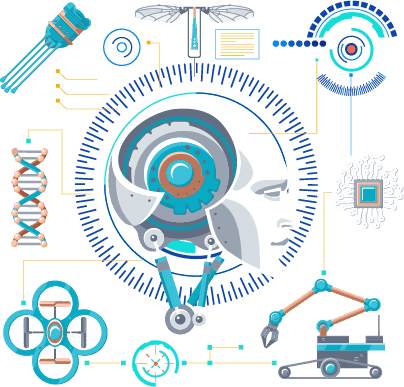
Boundary Annotation
In the boundary annotation service, labeling process could be completed as quickly as possible by utilizing the interactive features for creating tight borders around items. Line segments of different sizes and shapes specify the borders.
The object’s boundary is distinct from the edges of an image. The contour around an object reflects its boundary, while the edges depict the quick shift in pixel intensity levels that may belong to boundary of an object or not. As the name suggests, a “boundary” online image annotation is something whose ownership changes. When pixel ownership changes from one surface to another, the border shows in the image.
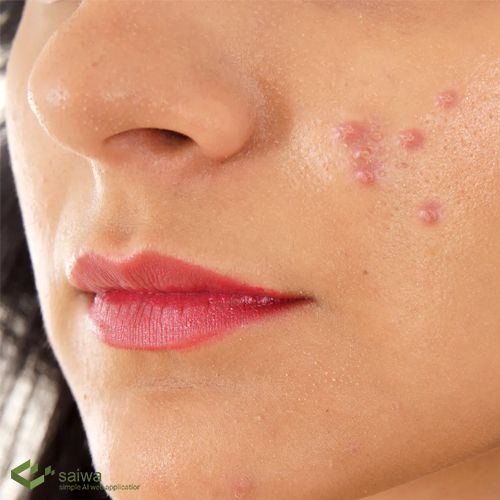
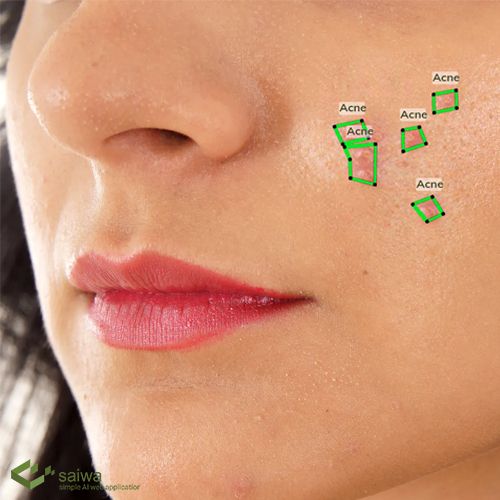
Bounding Box Annotation
The bounding-box annotation service provides everything a machine learning specialist needs to classify images quickly. Multiple bounding-boxes within an image may be defined with a few clicks. One or more labels are attached to each bounding-box.
Bounding-box annotation labels or describes specific elements in picture data using bounding-boxes or rectangular boxes. Bounding box annotation makes it easier for machine learning models to perform object identification and localization tasks more efficiently and reliably. This might be used to describe the size and placement of an item in an image, but it also has other applications. Bounding-boxes are commonly used in developing feature detectors for computer vision applications such as image categorization and object recognition.
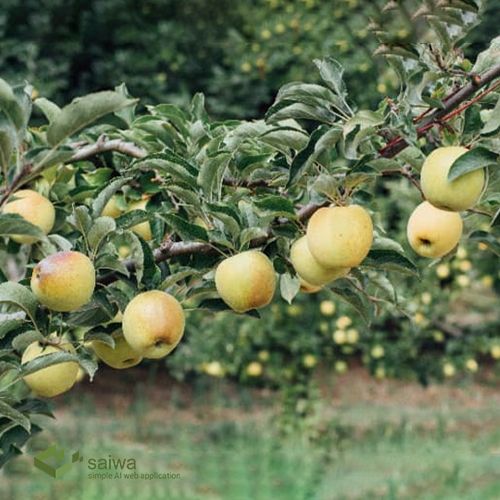
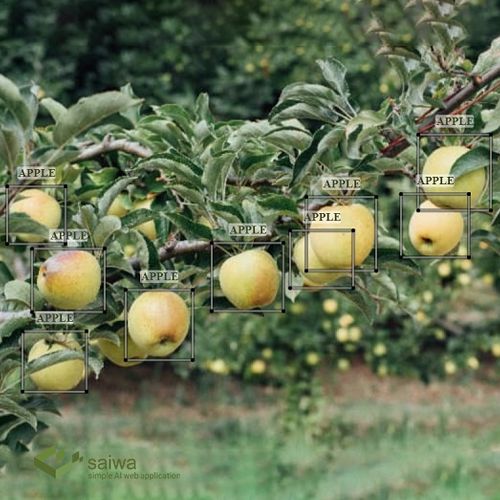
Classification Annotation
Classification is an entire-image annotation that detects attributes in the input images. Each image may have a single label or multiple labels. This annotation is employed when a machine learning model is trained to classify unlabeled images using known labeled images. Examples of image classification applications include texture classification, medical detection, defect detection, scene detection, and other applications. Classification annotation is the simplest and quickest type of annotation.
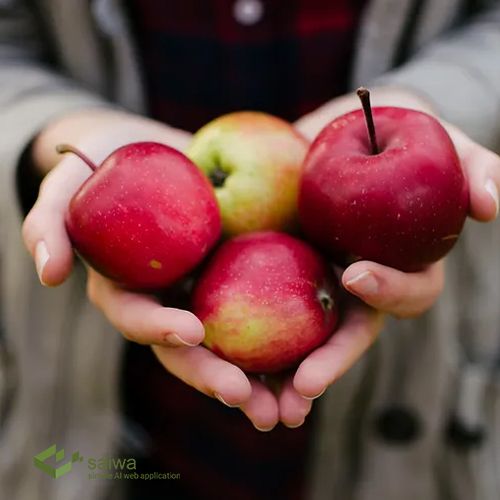
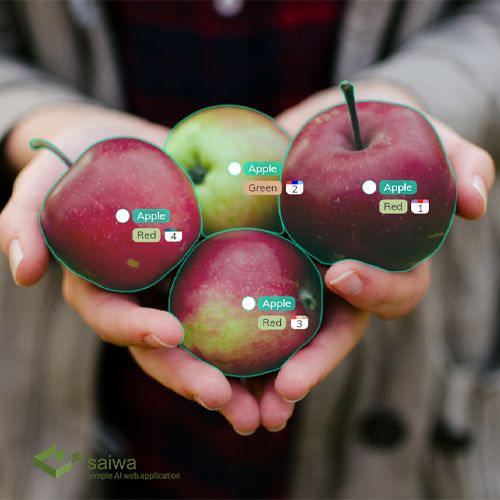
Object Detection
Object detection is a computer vision technology that determines the location of objects in images or videos. Object detection algorithms often use machine learning or deep learning to obtain relevant results. We may quickly recognize and find items of interest when we look at images or videos. Object recognition aims to use computers to mimic this intelligence. The procedure is done indirectly in this method; therefore, the model is trained first, then the outputs are transmitted to the tool, and the user can see the outputs.
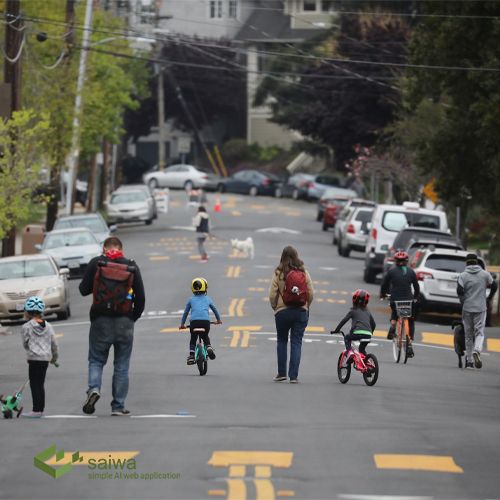
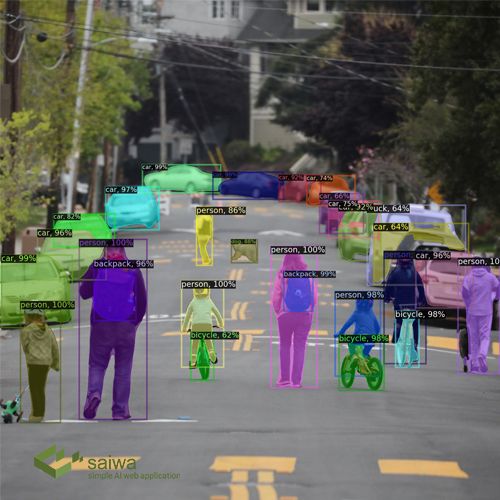
Count Objects
Counting objects using artificial intelligence vision algorithms is a shared computer vision approach for detecting and counting objects in a scene. Machine learning models are developed to recognize specific items in video images. Camera-based industrial vision systems identify and count items, parts, and cartons. This machine-learning-based technique uses a pre-trained model to count objects. This service has several potential applications in agriculture, industry, and medicine. An essential preprocessing procedure called “object modeling” must first be completed to count objects.
Deep Learning
Deep learning is a subset of machine learning that seeks to simulate the activity of the machine learning experts for feature extraction allowing it to “learn” from enormous volumes of data. While a single-layer neural network may still make approximations, more hidden layers can help improve and tune it for accuracy.
Many artificial intelligence (AI) programs and services rely on deep learning to enhance automation by conducting analytical and physical activities without human interaction. Deep learning as a service technology is at the heart of commonplace products and services (such as virtual assistants, voice-enabled TV remotes, and credit card fraud detection) and upcoming innovations (such as self-driving cars).
The Benefits of Using Machine Learning Online Demo
Our interactive machine learning online demo offers a failproof platform to examine machine learning functions this presents information about how data can be processed electrically. You will witness it before your very eyes machine learning models trained on data for prediction purposes. Machine learning online demo offers a multitude of benefits:
Demystify Machine Learning
Machine Learning often appears complicated due to complex terminologies. You would be surprised Machine Learning online demo putting across difficult ideas in several simple steps that even the likes of you would find it very easy.
Experiment Without Risk
Trying out new technologies can be expensive and time-consuming. Machine learning online demo provide a safe space to experiment with different algorithms and datasets without any commitment.
Boost Decision-Making
See firsthand how machine learning can analyze vast amounts of data to identify patterns and trends that might be missed by humans. This can empower you to make more informed decisions in your business or personal life.
Discover Potential Applications
The machine learning online demo shows the various applications of machine learning across various industries. You might discover how it can make your workflow, personalize customer experiences, or optimize resource allocation.