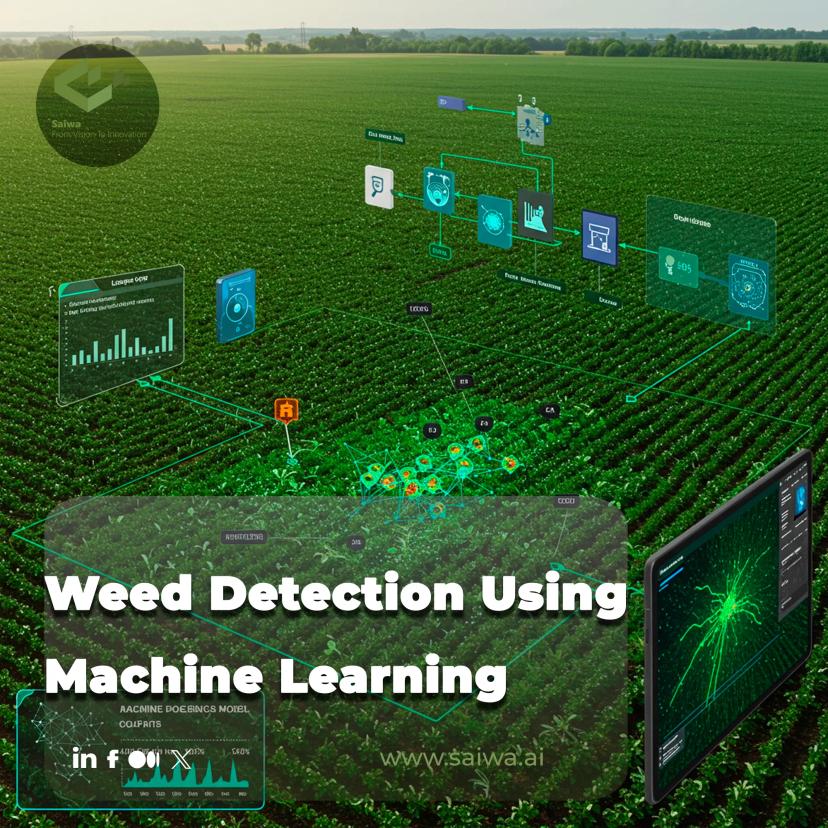
Weed Detection Using Machine Learning , The New Standard in Precision Agriculture
Weeds are a major challenge for farmers, competing with crops for vital resources such as water, sunlight and nutrients. This competition not only reduces crop yields, but also affects crop quality, resulting in financial losses and higher food production costs. Traditional weed control methods, such as manual removal and widespread use of herbicides, are often inefficient, expensive and harmful to the environment.
Sairone, developed by Saiwa, offers a smarter solution to this problem. This AI/ML-powered drone platform uses machine learning to quickly identify weeds from images, scanning large fields in minutes. By targeting specific weeds and minimizing herbicide use, it helps reduce costs, lower environmental impact, and improve farm efficiency. With Sairone, growers can control weeds early and accurately, optimizing productivity and allowing them to focus on what matters most.
In this article, we'll discuss how machine learning is reshaping weed detection, the benefits of this technology, and its potential to revolutionize agriculture.
The Importance of Early Weed Detection
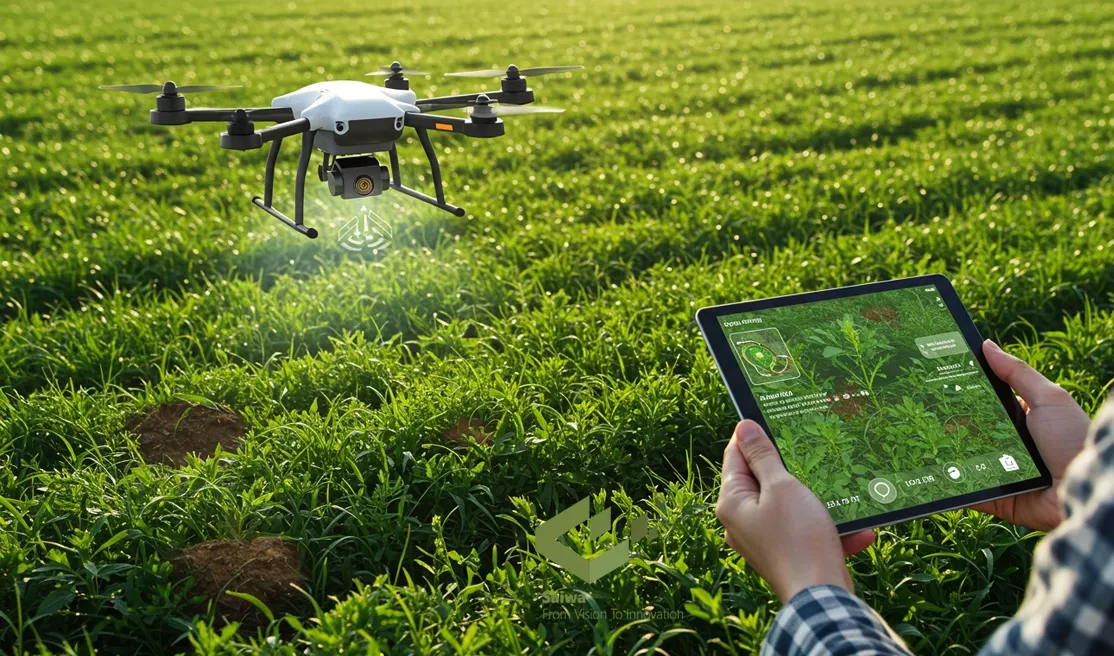
Early and accurate weed detection is crucial for minimizing crop loss and optimizing resource use in agriculture. Timely identification of weeds allows for targeted interventions, preventing weed infestations from spreading and minimizing their impact on crop growth.
Early detection also enables the use of site-specific weed control methods, reducing the need for blanket herbicide applications and minimizing the environmental impact of weed management practices. By precisely targeting weeds, farmers can optimize the use of herbicides, water, and other resources, promoting sustainable and efficient agricultural practices.
Machine Learning Approaches to Weed Detection
Various machine learning in agriculture techniques are employed for weed detection, each with its own strengths and limitations.
Convolutional Neural Networks (CNNs)
Convolutional Neural Networks (CNNs) are a class of deep learning algorithms particularly well-suited for image analysis and object detection. CNNs are designed to automatically learn hierarchical features from images, enabling them to distinguish between weeds and crops based on their visual characteristics, such as shape, color, and texture.
CNNs have demonstrated remarkable success in weed detection tasks, achieving high accuracy in classifying different weed species and distinguishing them from crops in various field conditions.
Vision Transformers (ViTs)
Vision Transformers (ViTs) are a relatively new class of deep learning models that have shown promising results in image recognition tasks, including weed detection. Unlike CNNs, which process images locally, ViTs process images globally, allowing them to capture long-range dependencies and contextual information within the image.
This global processing capability can be particularly advantageous in weed detection, where the spatial arrangement of plants and the surrounding environment can provide valuable cues for distinguishing weeds from crops.
Data Augmentation to Overcome Class Imbalance
Class imbalance, where one class (e.g., weeds) is significantly under-represented compared to another class (e.g., crops) in the training dataset, is a common challenge in machine learning for weed detection.
Data augmentation in deep learning techniques, such as image rotation, flipping, scaling, and color jittering, can be used to artificially increase the number of weed samples in the training dataset, mitigating the effects of class imbalance and improving the robustness and performance of machine learning models.
Practical Applications of Machine Learning for Weed Control
Machine learning is being integrated into various practical applications for weed control, transforming traditional weed management practices.
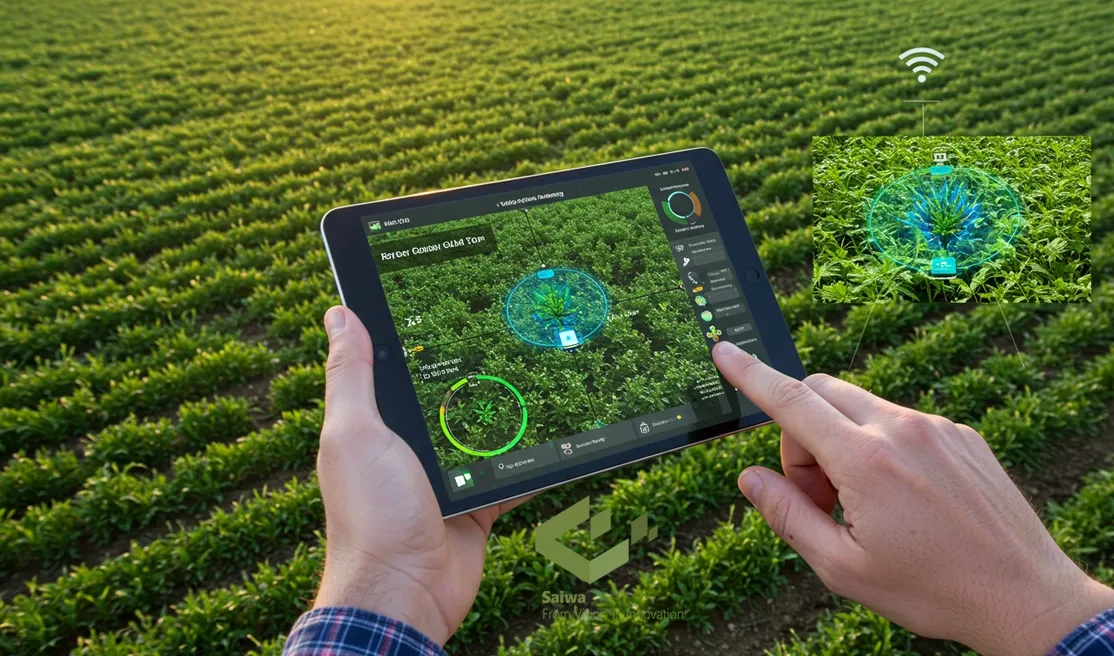
AI in Weed Detection via Drone and Satellite Imagery
Drones and satellites equipped with high-resolution cameras provide a cost-effective and efficient platform for large-scale weed mapping and monitoring.
Machine learning algorithms can analyze satellite and done imagery to identify weed infestations, map their spatial distribution, and assess their severity. This information can be used to guide site-specific weed control interventions, optimizing herbicide application and minimizing environmental impact.
Autonomous Systems for Precision Weed Removal
Machine learning is enabling the development of autonomous systems for precision weed removal, such as robotic weeders and targeted herbicide applicators. These systems use computer vision and machine learning algorithms to identify and locate weeds in real-time, enabling precise and targeted weed removal without damaging crops.
Autonomous weed control systems can significantly reduce labor costs, improve weed management efficiency, and minimize herbicide use.
AI-Driven Weed Control Using Laser Technology
Laser weeding is an emerging technology that uses high-powered lasers to destroy weeds without the use of herbicides. Machine learning algorithms can be used to guide laser weeding systems, identifying and targeting weeds with high precision, minimizing damage to crops and reducing the environmental impact of weed control. Laser weeding offers a sustainable and environmentally friendly alternative to traditional herbicide-based methods.
Advantages of Weed Detection Using Machine Learning
Machine learning offers several advantages for weed detection and control.
Precision
Machine learning enables precise identification and localization of weeds, distinguishing them from crops based on their visual characteristics, even at early growth stages. This precise identification allows for targeted weed control interventions, such as site-specific herbicide application or robotic weeding, minimizing the need for blanket herbicide applications that can harm crops and the environment.
This precision reduces herbicide use, minimizes environmental impact, and promotes sustainable agricultural practices, leading to increased crop yields and reduced input costs.
Scalability
Machine learning algorithms can analyze large datasets of aerial imagery and sensor data acquired from drones, satellites, and other sources, enabling large-scale weed mapping and monitoring across vast agricultural areas.
This scalability allows for efficient and comprehensive weed management, providing valuable insights into weed distribution, density, and growth patterns. By analyzing large-scale data, machine learning can identify weed hotspots, track the spread of invasive species, and optimize resource allocation for targeted interventions. As a consequence, this improves overall farm productivity and resource use efficiency.
Cost-Effectiveness
Machine learning-based weed detection and control can significantly reduce labor costs associated with manual weed removal, which is often labor-intensive and time-consuming. By automating weed detection and enabling targeted interventions, machine learning improves the efficiency of herbicide application, reducing the amount of herbicide needed and minimizing the cost of weed control.
This optimization of resource use, combined with reduced labor costs, increases profitability for farmers and promotes sustainable agricultural practices.
Environmental Sustainability
By enabling precision weed control, machine learning minimizes herbicide use, reducing the negative impacts of herbicides on the environment, such as soil and water contamination, and promoting sustainable agricultural practices.
Reduced herbicide use also minimizes the development of herbicide-resistant weeds, preserving the effectiveness of herbicides for future use. This contributes to a healthier and more resilient ecosystem, protecting biodiversity and promoting long-term environmental sustainability.
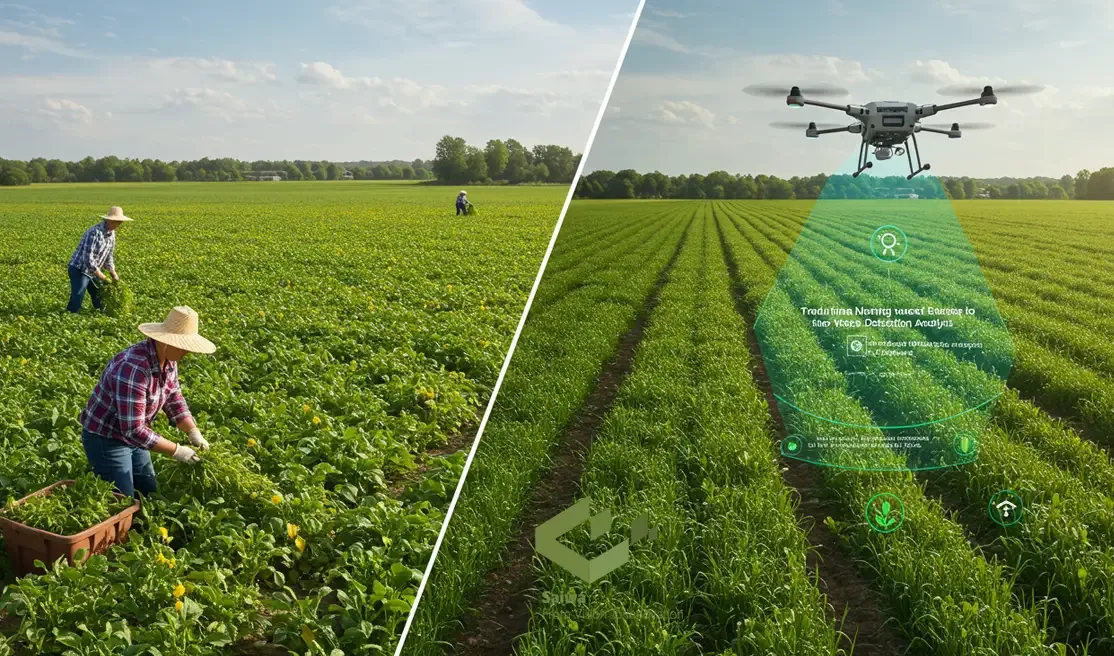
Challenges in Implementing Machine Learning for Weed Detection
Despite its potential, implementing machine learning for weed detection faces several challenges.
Data Quality and Availability
Machine learning algorithms, particularly deep learning models, require large, diverse, and accurately annotated datasets for training and validation. Acquiring and annotating such datasets can be time-consuming, costly, and challenging, particularly for diverse weed species and varying field conditions.
The quality of the data, including image resolution, lighting conditions, and annotation accuracy, directly impacts the performance and reliability of machine learning models. Addressing this challenge requires developing efficient data collection and annotation strategies, exploring data augmentation techniques, and leveraging publicly available datasets.
Technological and Operational Barriers
Integrating machine learning models into existing agricultural equipment and workflows can be technically challenging, requiring specialized expertise and infrastructure.
Deploying and operating machine learning-based weed detection systems in real-time also requires robust hardware and software infrastructure, including powerful processors, high-speed internet connectivity, and reliable power sources, which can be a barrier for some farmers.
Overcoming these barriers requires developing user-friendly software interfaces, providing technical support and training to farmers, and exploring cloud-based solutions for data processing and storage.
Accuracy and Generalization
Machine learning models for weed detection need to be accurate and robust across diverse environmental conditions, such as varying lighting, soil types, crop growth stages, and weather conditions.
Achieving high accuracy and generalization requires careful model selection, hyperparameter tuning, robust training procedures, and rigorous validation using diverse datasets. Furthermore, ongoing adaptation and retraining of models are necessary to account for changing field conditions and maintain reliable weed detection performance over time.
Prospects of Weed Detection Using Machine Learning
The future of weed detection using machine learning is promising, with ongoing advancements in technology and increasing integration with other agricultural practices.
Integration with IoT and Precision Agriculture
Integrating machine learning with IoT sensors and precision agriculture technologies, such as GPS-guided tractors and variable-rate applicators, can provide real-time information on weed infestations, soil conditions, crop health, and other relevant parameters.
This Integration of IoT and agriculture enables data-driven decision-making, optimizing resource management, such as irrigation, fertilization, and pesticide application, used in enhanced agricultural productivity and sustainability. By creating a smart and connected agricultural ecosystem, machine learning can contribute to more efficient and environmentally friendly farming practices.
Transfer Learning and Domain Adaptation
Transfer learning and domain adaptation techniques can be used to leverage pre-trained machine learning models for weed detection, both in new environments and for different weed species, therefore, reducing the need for large training datasets and accelerating the development of robust weed detection systems.
These techniques allow for adapting existing models to new geographical locations, different crop types, and varying weed species, making machine learning-based weed detection more accessible and adaptable to diverse agricultural contexts.
Edge Computing
Edge computing, where data processing is performed closer to the source (e.g., on a drone or tractor), can enable real-time weed detection and control in the field, reducing latency and enabling rapid response to weed infestations.
By processing data locally, edge computing minimizes the need for high-bandwidth internet connectivity and enables autonomous operation of weed control systems, such as robotic weeders and targeted herbicide applicators. This real-time processing capability enhances the efficiency and effectiveness of weed management, minimizing crop loss and optimizing resource use.
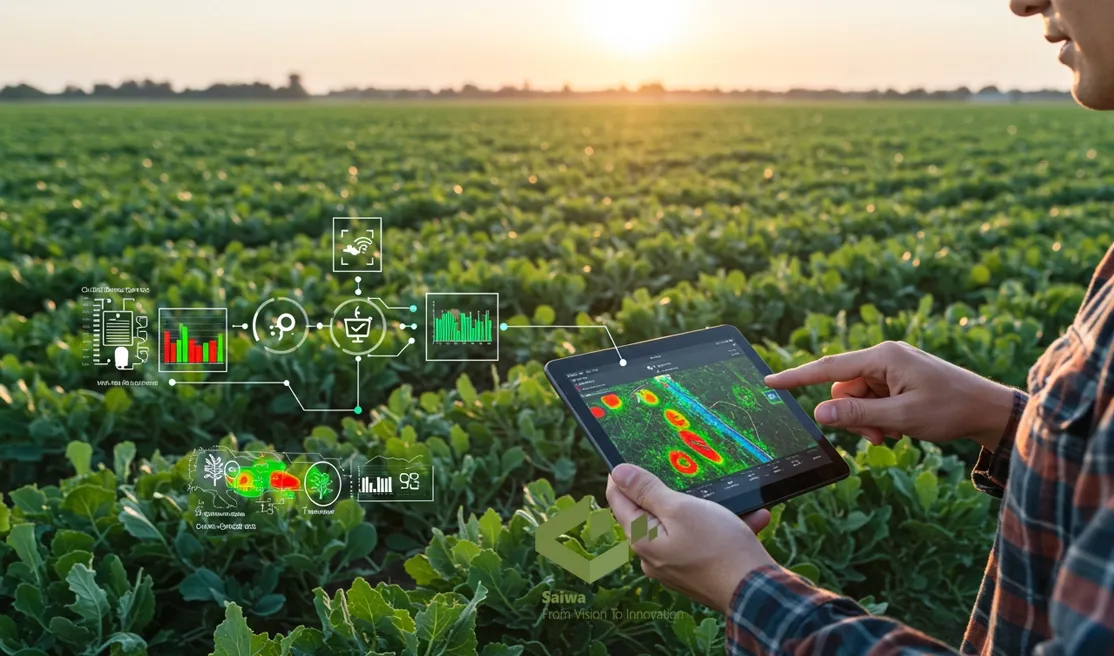
Conclusion
Machine learning is changing the way we approach weed management, offering more accurate and efficient methods that help farmers use fewer resources and reduce their environmental impact. While challenges remain, advances in AI and data technology show great potential to improve weed management and make agriculture more sustainable.
By applying these advances and addressing the challenges that lie ahead, we can create an agricultural system that's not only more efficient, but also better for the environment. With continued innovation, machine learning has the potential to make agriculture more productive and sustainable, benefiting both farmers and the planet.