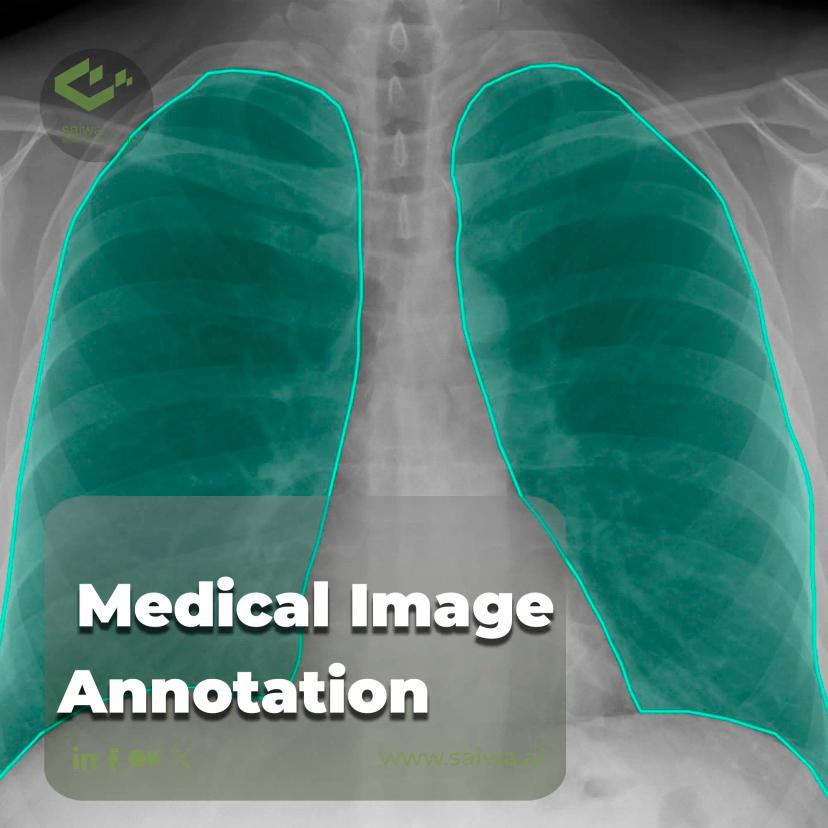
The Ultimate Medical Image Annotation Guide
Machine learning is revolutionizing the world of medicine and healthcare, allowing professionals to identify patients better and faster than before. Still, we need high-quality medical image annotation to train ML models. This is where medical image annotation comes in.
ML can potentially change the entire medical pipeline, from when a patient enters the hospital to when they leave. It has been noted that training neural networks and ML models is a complex task and requires a large amount of high-quality labeled data. This is where medical data labeling is important. This article will cover all the information you require regarding this subject.
Read more: AI in Healthcare
What is medical image annotation?
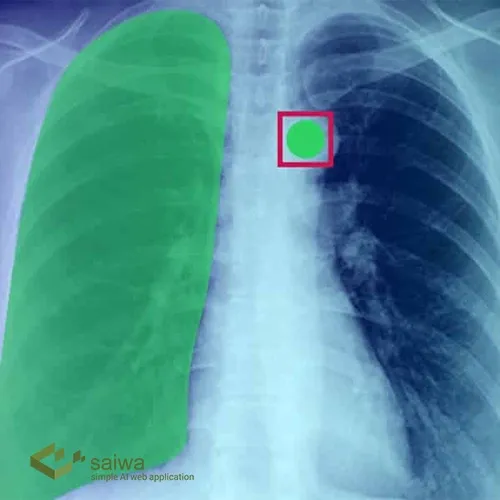
Medical image annotation is the process of labeling medical image data such as X-rays, CT scans, MRI scans, mammograms, or ultrasounds. It is used to train artificial intelligence algorithms for medical image analysis and diagnosis to help physicians save time, make better decisions, and improve patient outcomes. Considering this issue, you should remember that the said process will not be as easy as it seems.
Medical image annotation is very important in the healthcare sector, so we would like to discuss the role and importance of medical image annotation in this article. It is interesting to know that various medical images can be annotated to create training datasets for different diseases.
Read more: The Comprehensive Guide to Image Annotation
Medical image annotation is used in two main ways:
Research: Researchers use labeled medical data to train machine learning models to develop new medical treatments and diagnostic tools. This is a critical step in building AI for healthcare because it gives researchers the ability to test and improve their models on large real-world datasets.
Clinical Applications: medical image annotation has many clinical applications, including radiology and anomaly detection. Machine learning models can help radiologists diagnose diseases by analyzing medical images. Anomaly detection models can help identify patients who are at risk of developing certain diseases or who are experiencing nonconductive behavior.
Both approaches are essential to advancing AI in healthcare. Researchers use tagged data to develop new AI tools while clinical applications use AI tools to improve patient care.
Medical Image Data Preparation
The existing difficulties in creating reliable machine learning models can be attributed to the limited availability of medical picture data, which is a significant problem.
Application-limited algorithms result from bottlenecks caused by tiny sample sizes from small geographic areas and the time- and money-consuming process of data preparation.
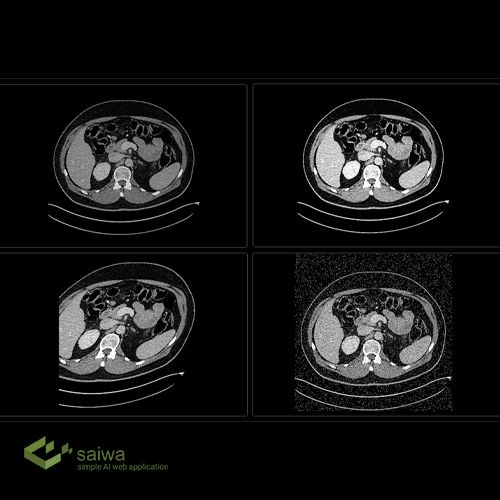
The process of preparing medical data for annotation:
Variety of data sets: Your data must not come from the same source or be the same. To increase reliability, you need a model that has been trained on diverse data sets. If your model is only trained on a subset of data, or on data that is similar, it won't know what to do when you show it different data.
Data set review process: When the data set is diverse, you should check it to ensure high quality. This includes checking for errors, inconsistencies, and missing data. It is useful to divide your data set into training, validation, and testing. Training includes about 80% of the data.
Data set size: Recent advances in machine learning have shown that quality in training models is as important as quantity. That is, a small but high-quality data set usually performs better than a large, low-quality data set.
Data set format: Two common medical imaging formats include DICOM and TIFF files. DICOM is the industry standard for radiologists. DICOM and TIFF files can optionally contain multiple images or slices and metadata about the patient and the image itself. Good medical annotation platforms support both formats.
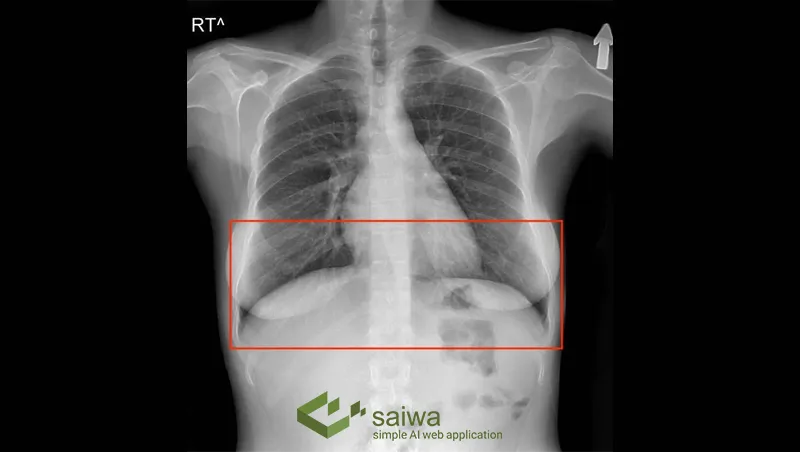
The role of medical image annotation in AI-based medical diagnostics
The potential of artificial intelligence in medical image diagnosis is very significant, and the healthcare industry is taking a lot of help from artificial intelligence and ML to provide faster and more reliable diagnoses for patients. Image annotation is one of the primary steps of ML algorithms. Some of the use cases of image annotation and ML in healthcare and AI medical diagnosis are as follows:
Cancer Diagnosis
Perhaps the biggest role of artificial intelligence in medical image analysis is detecting cancer cells. When the models are trained on large sets of medical imaging data, it helps the model to accurately identify and predict the growth of cancer cells in organs. This largely eliminates the possibility of human error and false positives.
Dental Imaging
Artificial intelligence-powered models can accurately diagnose medical problems related to teeth and gums, such as tooth decay, abnormalities of tooth structure, cavities, and diseases.
Liver complications
Evaluating medical images for abnormalities can identify and effectively monitor liver-related complications.
Brain disorders
Medical image annotation helps diagnose brain disorders, blood clots, tumors, and other neurological problems.
Skin
Computer vision and medical imaging are widely used to quickly and effectively diagnose skin diseases.
Heart Disease
Artificial intelligence is also widely used in cardiology to diagnose heart abnormalities, heart disease, the need for intervention, and echocardiography interpretation.
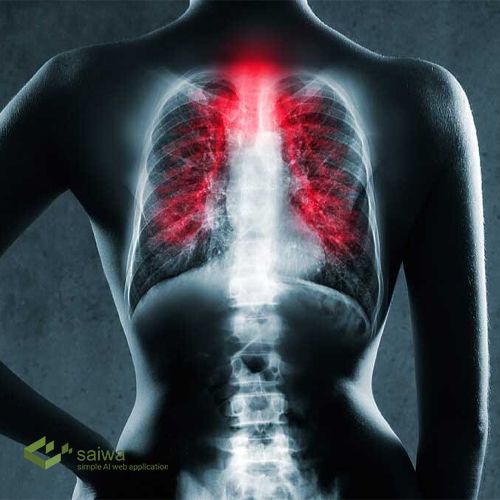
Medical Image Annotation VS Regular Data Annotation
If you are building an ML model for medical imaging, you should be aware that this process differs in many ways from regular image data annotation. Let's start with radiological imaging.
But first, keep this assumption in mind: All the photos and videos you have taken come from a small part of the spectrum called visible light. However, radiological imaging uses X-rays, which are in the invisible light part of the electromagnetic spectrum.
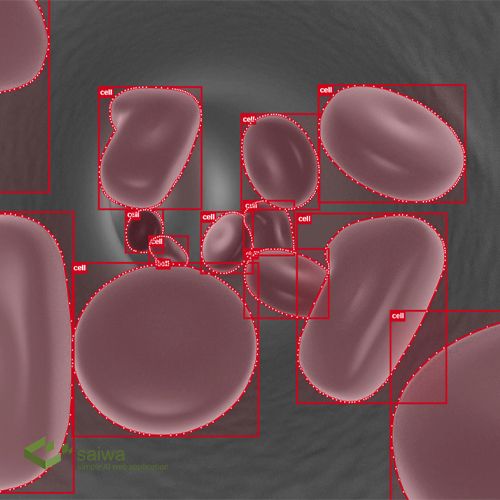
Below is a detailed comparison of medical imaging annotation and traditional data annotation:
Medical Imaging Annotation | Regular Data Annotation |
---|---|
All medical imaging data should be de-identified and protected by Data Processing Agreements (DPA) | Regular images are readily available. |
Medical Images are in DICOM Format | Regular images can be in JPEG, PNG, BMP, and more |
Medical image resolutions are high with a 16-Bit Color profile | Regular images can have an 8-Bit Color profile. |
Medical images also contain units of measurement for medical purposes | Measurements pertain to the camera |
HIPAA compliance is strictly required | Not regulated by compliance |
Multiple images of the same object from different angles and views are provided | Separate images of different objects |
Radiology controls should guide it | Regular camera settings are accepted |
Multiple slice annotations | Single slice annotations |
What makes medical image annotation different from traditional labeling?
Medical image annotation is a completely different process from conventional image annotation. Here are some of the differences between the two processes:
Availability of data
Most routine images are usually available for free or under a standard NDA, but strict data processing agreements usually protect medical images. This is primarily to protect patient privacy. Obtaining medical imaging data is usually a longer process than other data types.
Technical differences
Images typically have only one layer, are smaller in size, and have a low bit depth. Medical images typically have multiple layers, larger size, and higher bit depth.
In addition, the tagging profiles are different for the two processes. While general annotators label conventional images, medical imaging requires specialized medical professionals. These professionals are used to specific UI and UX paradigms. Therefore, when selecting a platform for data annotation, it is important to consider whether medical professionals can easily use the keyboard control and its user interface.
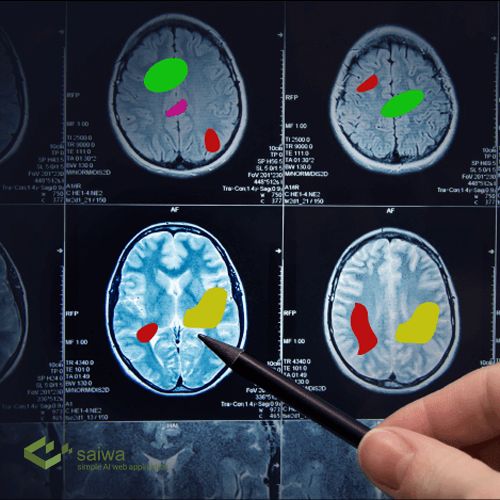
Medical Image Annotation Workflows and Best Practices
Establishing efficient and standardized workflows for medical image annotation is crucial for ensuring high-quality annotations and facilitating the development of reliable AI-based diagnostic tools. One important aspect is the involvement of subject matter experts, such as radiologists or pathologists, throughout the annotation process. Their expertise is invaluable in ensuring accurate and consistent labeling, as well as providing guidance on relevant clinical aspects and edge cases. Another key consideration is the development of clear annotation guidelines and protocols. These guidelines should define the medical image annotation tool tasks, label categories, and conventions to be followed, ensuring consistency across different annotators and datasets. Regular training and calibration sessions for annotators can further reinforce these guidelines and improve annotation quality.
Emerging Technologies in Medical Image Annotation Tool
The field of medical image annotation tool is rapidly evolving, with several emerging technologies and approaches showing promise in improving annotation accuracy, efficiency, and scalability. One such technology is active learning, which involves iteratively selecting the most informative samples for annotation, reducing the amount of labeled data required for training accurate models. Active learning techniques can help optimize the use of limited annotation resources and accelerate model development. Another emerging approach is weakly supervised learning, which leverages various forms of weak or noisy supervision, such as image-level labels or textual reports, to reduce the need for expensive pixel-level annotations. This can be particularly useful in scenarios where obtaining detailed annotations is challenging or resource-intensive.
Semi-supervised learning techniques, which combine small amounts of labeled data with large amounts of unlabeled data, are also gaining traction in medical image annotation tool. These methods can leverage the vast amounts of available unlabeled medical data to improve model performance while minimizing annotation efforts.
Conclusion
Artificial intelligence challenges humans with its capabilities. This issue is currently being seen in different parts of people's lives and is changing everything in the forefront and the background. One aspect that is undergoing many changes is healthcare. Artificial intelligence is revolutionizing many aspects of healthcare, from data analysis to automating the operation of many medical devices. As mentioned above, one of the biggest contributions of AI to this sector is the analysis of medical records for various purposes. As the healthcare sector continues to evolve, it can help provide better patient treatment while improving the business outlook for organizations. Adopting automation is one of the main ways to do this. With the help of artificial intelligence, image analysis can help solve many problems in this sector. With the right data annotation experts working on your image annotation project, your healthcare organization can take advantage of this technology and increase the value of your medical image data.
Empowering Medicine with Saiwa's AI Image Annotation Tools
Machine learning in medicine has several applications such as Automatic diagnosis of diseases, prediction of treatment outcomes and so on. To apply supervised learning methods, an expert must first label the data. The three annotation tools on Saiwa can help a lot here. 1. Boundary Annotation 2. Bounding Box Annotation 3. Classification Annotation