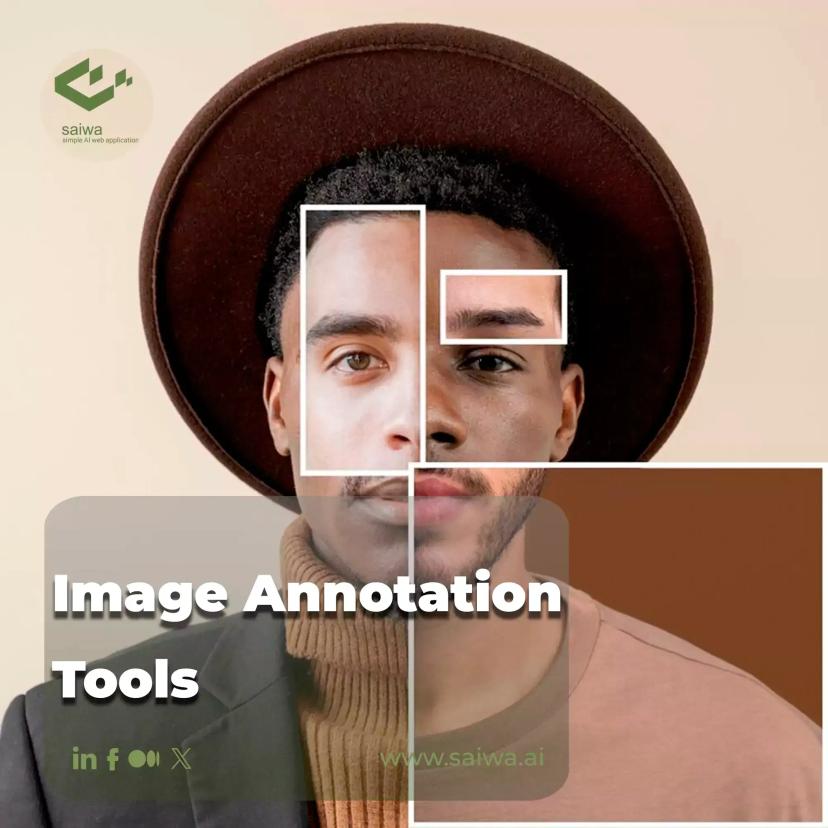
Image Annotation Tools | The Top +10
Selecting the right tools for computer vision, data labeling, and classification is essential. If annotating data is time-consuming, there is a better way. The proper labeling tools allow you to speed up data annotations and get precise results immediately. Even though the quantity and variety of your image data are probably growing daily, getting pictures annotated following your requirements may be challenging. This might slow down your project and, as a result, your speed to market. Your image annotation tools, resources, and staff choices should be carefully considered. These tools are widely available, but having numerous options could make you feel like you're searching through a hay field. Fortunately, there is a more sensible choice. This article teaches how to select the correct video and image annotation tools.
What is image annotation?
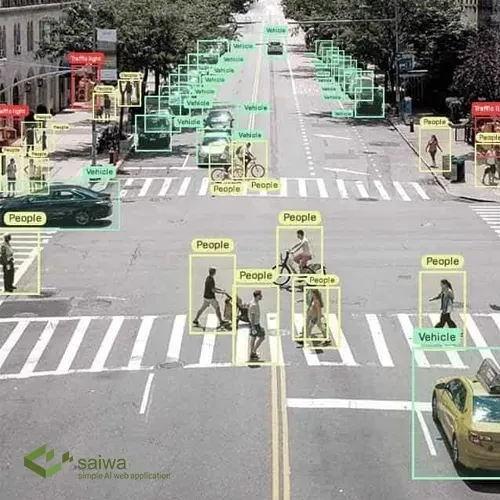
Labeling a picture or series of photos is known as an image annotation. Although some image annotation jobs can be partially automated, they are often manual. Image annotation teaches computer vision algorithms how to recognize objects and classify images. Machine learning experts first decide on the labels for the model and then create a labeled dataset with many example photos allocated to each label to train and test these algorithms. This dataset was created using the picture annotation procedure. For instance, you could ask your annotators to identify the cars in a set of photographs. The collected data can be used to train a model that can distinguish between vehicles and other possible road hazards, including pedestrians, traffic lights, and intersections. Autonomous driving is one example of how image annotation fuels computer vision.
Read more: What is Image Annotation?
How does image annotation work?
Now let's discuss the specifics of how image annotation functions in practice. You need an image annotation tool and enough high-quality training data to begin classifying your images. We need to ask the correct questions in order to identify the image annotation tools that best suits our use case among the abundance of image annotation tools available. A thorough grasp of the type of data being annotated and the task at hand is necessary to select the appropriate annotation tool. You should pay close attention to:
The type of data being used
The appropriate type of annotation
The format in which annotations must be stored
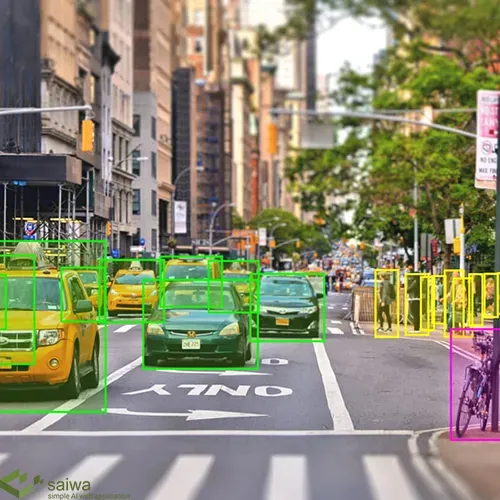
Moreover, annotating can be carried out on an individual or group level, or it can be contracted out to independent contractors or businesses that provide annotating services. Here is a brief tutorial on how to begin annotating photographs.
Get your raw footage or image data
It is necessary to prepare raw data in the form of photographs or videos as the initial stage in the annotation process. Before being submitted in for annotation, data is often cleansed and processed in order to eliminate duplicates and poor-quality material. You have two options: either gather and analyze your own data, or use publicly accessible datasets, which are nearly always offered with a particular kind of annotation.
Find out the appropriate label types to employ
The kind of task the algorithm is being taught has a direct impact on the type of annotation that should be used. Labels take the form of class numbers when the algorithm is learning how to classify images. On the other side, if the system is learning image segmentation or object detection, the annotation would be boundary box coordinates or semantic masks, respectively.
For each object you wish to label, create a class
The majority of supervised deep learning methods require fixed-class data to be used. In order to avoid creating duplicate classes or labeling comparable objects under different class names, it may be helpful to set up a fixed number of labels and their names in advance.
Use the correct tools for annotating
Following the selection of the class labels, you may begin annotating your image data. Depending on the computer vision task the annotation is being done for, the corresponding object region may be annotated or picture tags may be added. You should supply class labels for each of these areas of interest after the demarcation phase. Bounding boxes, segment maps, and polygons are examples of complex annotations that should be as precise as possible.
Export your dataset after modifying it
Several formats for exporting data are available depending on how it will be utilized. JSON, XML, and pickle are common export formats. However, there are additional export formats that can be used to train deep learning algorithms, such as COCO and Pascal VOC. These formats first came into usage when deep learning algorithms were tailored to match them. By exporting a dataset in the COCO format, we may avoid the added burden of adapting the dataset to the model inputs and simply plug it into a model that supports that format.
Why is image annotation useful?
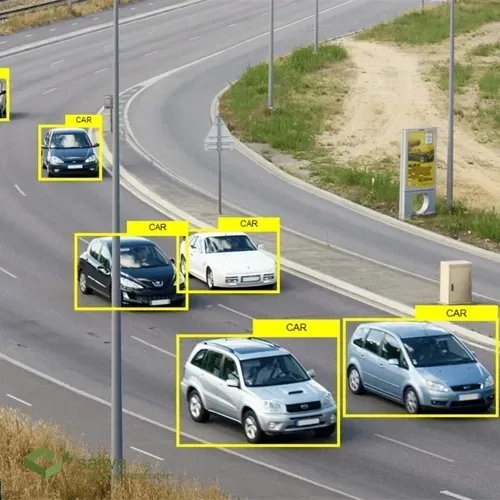
Image annotation is crucial for machine learning and artificial intelligence when using computer vision (CV) models. The benefits and importance of image annotation services are starting to be understood across industries. The business for data solutions utilizing AI and machine learning is expected to grow to $1.2 billion by 2023. These are some instances of how businesses in various industries might benefit from image annotation tools and services.
Transportation
CV is utilized by self-driving cars, which are now a reality. Cutting-edge machine-learning algorithms must power these vehicles to guarantee their effectiveness and safety. With these driverless vehicles, automakers may develop smart apps with image annotation.
Healthcare
In the field of healthcare, CV can help improve diagnostic accuracy and care quality. Using image annotation, CV systems can concentrate on patterns and issues like tumors or hairline fractures using CT scans, MRIs, and other diagnostic technologies.
Agriculture
Mixes traditional farming practices with cutting-edge technology to boost efficiency, productivity, and sustainability. It is now possible to predict agricultural output, evaluate the health of plants, and enhance soil quality thanks to CV systems. Drones, robotics, and GPS sensors all have essential roles to play in contemporary agriculture.
eCommerce and retail
Annotating images may enhance consumer-client interactions and help them choose the finest products. Each item on the website will have exact labels and details if an annotation is done correctly. The precise categorization of the products is ensured through annotation, which enhances search results. It also aids in enhancing visual search.
Designers
This is one of the best advantages of Online image annotation. This technology is very popular among designers and for many reasons it makes their design process easier. With the help of Online image annotation tools, designers can enjoy a hassle-free correction process by receiving quick feedback from their colleagues.
Creative agencies
Just like designers, creative agencies deal with a lot of visual content that needs to be reviewed. Instead of a Pandora's box of asking for feedback via email, most creatives prefer to use Online image annotation tools to make the image review process easier.
Teachers and students
Teachers need easier ways to mark assignments in their workflow, and Online image annotation tools can give them the break they need. With the help of Online image annotation tools, students can submit their visual content assignments, presentations, art projects, and other assignments for review, and then teachers will quickly access those assignments and provide feedback easily. they give.
Remote teams
Remote teams are always looking for new ways to improve their collaboration. Remote workers can use Online image annotation tools to simplify communication among their distributed team members without the need for face-to-face interactions.
Advantages of Online image annotation
Online image annotation has advantages that we will describe in this section:
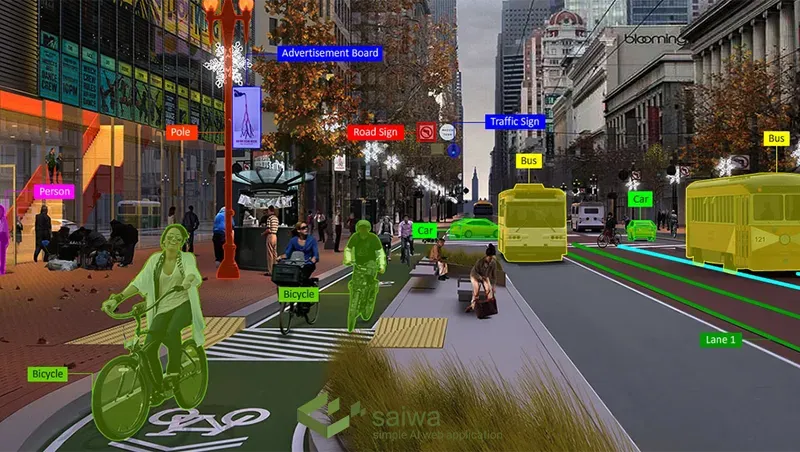
Improved visual content
Feedback is more effective when it is in text form because it is easier to understand. Online image annotation tools help designers and clients speak the same language and speed up the review process through relevant comments pinned to elements that need fixing.
Frictionless collaboration and communication
Online image annotation tools facilitate remote collaboration by allowing design markups to be shared with colleagues via links. Reviewers can then simply open the markup in their web browser and provide textual comments. Add with just a few clicks. As a result, there is no need for endless email chains or long messages or multiple meetings. In this way, communication becomes simple and the process of cooperation becomes frictionless.
Real-time feedback
When using the Online image annotation tools, your colleagues can see the added feedback in real-time, and you will have a feedback archive with all the comments in one repository.
Shorter feedback loops
Because Online image annotation makes it easier to get feedback the first time, teams can skip endless rounds of reviews and get project registrations faster. As a result, you will suddenly be in the final draft much faster than you thought.
Types of image annotation
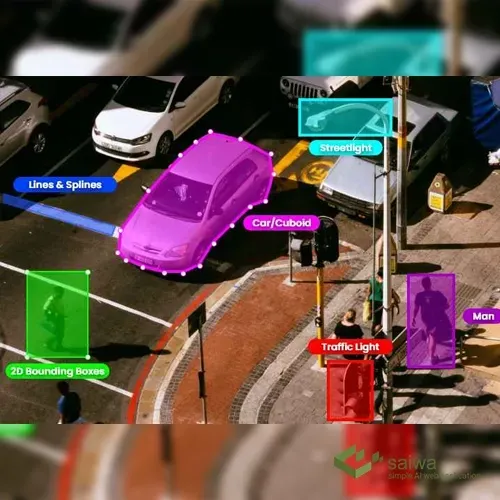
Image annotation refers to adding metadata or labels to an image that can help identify and understand its contents. There are several types of image annotation techniques, including
Bounding box annotation
This involves drawing a rectangle around an object in the image, which can be used to identify the location and size of the object.
Polygon annotation
This technique involves drawing a polygonal shape around an object in an image. It is often used when the object in the image has irregular or complex shapes.
Semantic segmentation
This technique involves dividing an image into different regions or segments and assigning each segment a label representing the object or feature it contains.
Instance segmentation
This technique is similar to semantic segmentation, but instead of grouping pixels by object class, it groups pixels by an object instance. This means that each object in the image is assigned a unique label.
Landmark Annotation
This identifies specific points or landmarks in an image. It is often used for face recognition or object tracking.
Image classification
It entails assigning a label or category to an entire image based on its content. It is often used in applications like image search or content-based image retrieval. These are some of the types of image annotation techniques. The choice of technique depends on the specific requirements of the task at hand. In online image annotation, image classification involves assigning a single class or label that describes the overall image, rather than labeling segmented objects within the image.
Read Also: Image Deraining
What are image annotation tools?
Image annotation refers to the practice of attaching labels to an image or set of images. It usually involves manual work, although some image annotation tasks can be semi-automated. The purpose of image annotation is to train computer vision algorithms for tasks such as image classification and object recognition. To train and evaluate these algorithms, machine learning engineers first determine model labels and generate a labeled dataset with a large number of image samples assigned to each label. Image annotation is the process of creating a dataset. image annotation tools make it easier for teams to examine large collections of images and apply tags to entire images or specific parts of an image. The structured dataset generated by these tools can be utilized to develop computer vision algorithms.
The Factors to Evaluate Image Annotation Tools
You can evaluate the usefulness of an image annotation tool by taking the following factors into account:
Formats
Images can be annotated in various formats, including the text files (such as txt and CSV), image masks, TFRecords, COCO JSONs, and Pascal VOC XMLs. Even though you can convert your annotations to multiple forms, it is advantageous if your annotation tool can generate annotations throughout the required format. Directly generating annotations in the appropriate format speeds up and simplifies the preprocessing data workflow.
Access
Make sure the tool matches the skills of your team members, including data scientists and labeling teams, and that the learning curve is not steep.
Usability
The tool you choose should be user-friendly and straightforward to save time and ensure a quick annotation process. Remember that the tool has a low degree of difficulty and is also suited for the specific skills of the various team members (data scientists and labeling teams).
Support
make sure your programs are supported by the tool. Some tools only support programs that run in a window or on the web. Just a web application can be used to access web-based tools. You must refrain from submitting confidential information to an unknown third-party online application if your image annotation project requires it to protect the data's privacy and security.
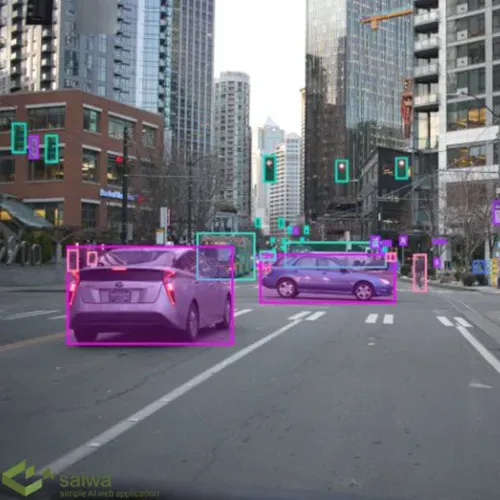
How is image annotation different from image labeling?
Since an image needs to be categorized, labeled, and then used for annotation and prediction purposes, image labeling and image annotation are often activities that are carried out together. Although both terms are used interchangeably, there are some very clear differences, such as:
Image annotation is more complicated than image labeling and categorizing photos; it requires a larger workspace to be most effective; it is used for a specific machine learning goal and for a specific audience or algorithm. Compared to image labeling technologies, image annotation requires much more time and knowledge.
To successfully implement AI systems, it is a much more difficult process and requires a large number of classified or annotated images to work with. It also requires a large vocabulary and a higher level of intelligence. Finally, image annotation adds captions and metadata to digital photographs.
Imagine labeling tool is easier to use. Unlike image annotation, this type of tool is also effective at smaller scales. Using image labeling software is a common practice, often done for all audiences and without any particular goal in mind. It can be done quickly and with a minimum of experience because it is a simple process. Its purpose is to organize and categorize photos by mapping their locations and assigning labels so that robots and other systems can be controlled.
Image labeling online tools categorize or classify the photos by recognizing the objects contained in them and can be used without a higher intellect using regular, simple terminology.
Important standards for analyzing online image labeling tools:
Here are some of the key standards offered by an image labeling tool.
Prices:
A user must choose the image labeling tool online that best suits their needs from the various options, as both on-device and cloud-based versions are available. The cloud-based system is only free for the first few uses; if you want to use it again, there is a small charge. However, on-device use is free.
Labels coverage:
The next most important factor is the number of labels that are available for you to use. For example, on-device users have access to 400 or more labels, most of which are common items that are used frequently, but cloud users have access to more than 10,000 labels that fall into different categories.
Unique entity IDs:
Another thing that is a requirement and available to cloud and on-device users is the use of knowledge graphs and entity IDs, which is a separate ID for each object in the labels and has help available to help you with the process.
Challenges of Online image annotation
Online image annotation, while essential for training accurate and robust computer vision models, comes with its own set of challenges. From dealing with vast datasets to ensuring inter-annotator agreement, addressing these challenges is crucial to produce high-quality annotations that yield reliable machine learning results. Let's delve deeper into the key challenges faced in image annotation:
Annotation Consistency Over Time:
In long-term projects or when multiple iterations of annotation are involved, keeping consistency in annotations becomes crucial. Annotators may introduce variations in labeling over time, affecting the quality of the dataset.
Ensuring Ethical Considerations:
Online image annotation may sometimes involve sensitive or private data, raising ethical concerns regarding data privacy and security. Safeguarding user privacy and adhering to ethical guidelines is crucial in any image annotation project.
Handling Complex Images with Overlapping Objects or Occlusions:
Some images contain complex scenes with multiple overlapping objects or occlusions, making accurate annotation challenging. Annotating such images with precision, especially in tasks like object detection or segmentation, requires careful consideration and expertise.
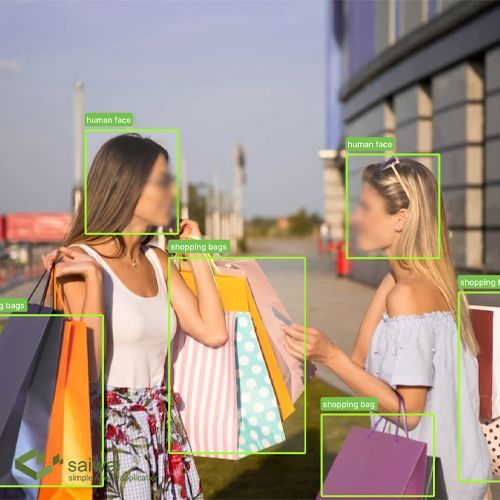
Legal and Privacy Considerations
As image annotation becomes more prevalent in various industries and applications, it brings about legal and privacy considerations that must be carefully addressed to protect individuals' rights and comply with data protection regulations. These considerations encompass data ownership, copyright, data privacy, and ethical handling of sensitive information. Let's delve deeper into the key legal and privacy challenges associated with image annotation:
Data Ownership and Copyright:
The ownership of annotated data must be clearly defined to avoid disputes over intellectual property rights. In cases where third-party data is annotated, explicit agreements on data ownership and copyright should be established between the data provider and the annotation service provider. Properly documenting data ownership is essential to prevent legal complications in the future.
Data Privacy and Security:
Annotated images may contain personal or sensitive information, such as faces, license plates, or medical images. Ensuring data privacy and security is paramount to protect individuals from potential harm or misuse of their data. Annotators and annotation service providers should implement robust data security measures to prevent unauthorized access or data breaches.
Informed Consent:
When annotating images that include identifiable individuals or sensitive data, obtaining informed consent from data subjects is essential. Annotators must clearly communicate the purpose of data collection and obtain explicit consent from individuals before using their data for annotation. Informed consent is particularly critical in medical imaging and research projects.
Evaluating Annotation Quality
The quality of the annotated dataset in online image annotation directly impacts the performance of the trained machine learning model. Some best practices for ensuring annotation quality include:
Detailed annotation guidelines that clearly explain the annotation schema, object classes, labels, etc. This ensures consistency across annotators.
Qualifications and expertise are required for annotators working on a specific project. Relevant knowledge improves understanding of images and context.
Annotator training and certification process using a subset of gold standard annotated images. Reduces incorrect labels.
Multiple annotators label each image to average out individual errors and biases. Cross-validation reveals inconsistencies.
Interim QA during the annotation process by auditing samples. Gives early feedback to annotators.
Confidence scores for annotations to focus review on uncertain cases.
Statistical metrics like Cohen's kappa coefficient to quantify annotator agreement. Values over 0.8 indicate strong agreement.
Using annotation software capabilities like consensus annotations, adjudication of conflicts, and automatic quality evaluation of completed datasets.
Periodic re-annotation or "refreshing" of datasets to improve quality over time as needed.
Investing resources into continuous evaluation and enhancement of online image annotation quality ultimately results in better training data and machine learning outcomes.
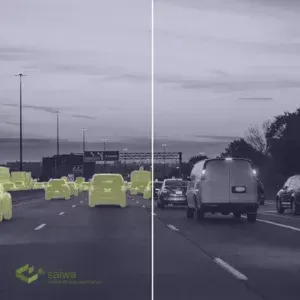
Introducing some of the best image Annotation Tools
Keylabs
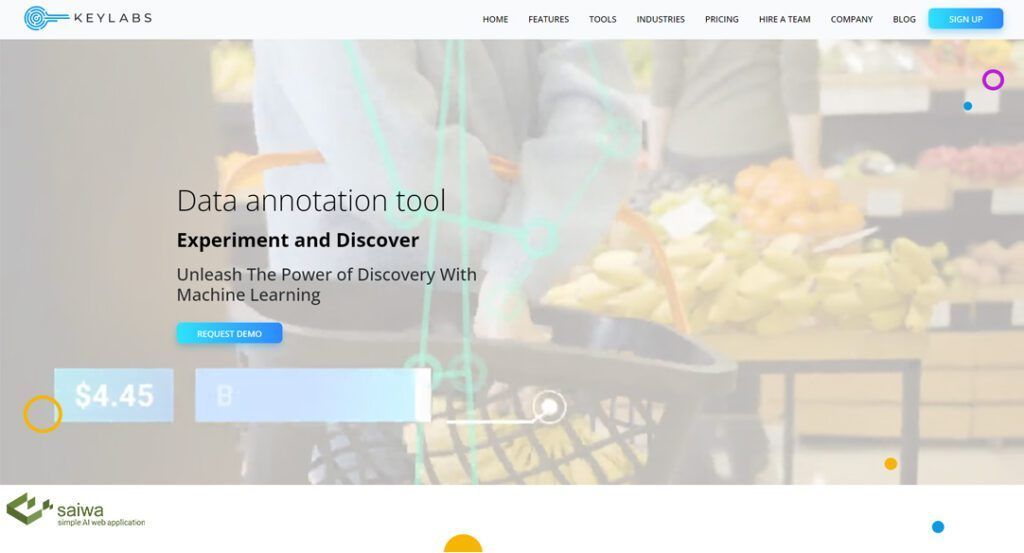
By adding boundary boxes, key points, and semantic segmentation to photographs, users can annotate them utilizing Keylabs. According to Keylabs, AI developers can annotate photos more effectively. This system provides programmers with a wide range of options for picture annotation styles and techniques. Due to the program's user-friendly Interface, users can quickly and accurately identify photos. It allows users to collaborate with team members while offering several tools to manage workflows and track progress.
V7 Labs
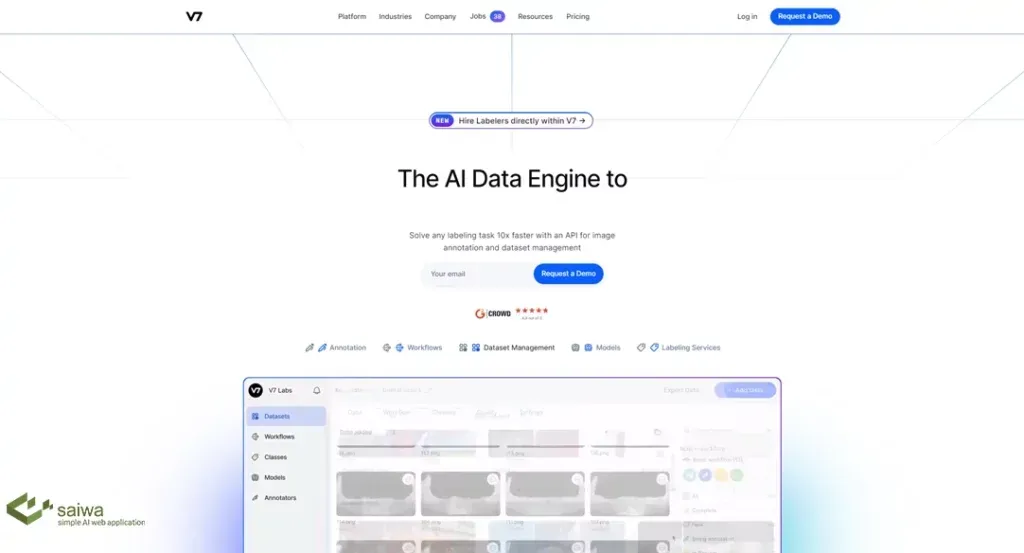
An image annotation tool called V7 Labs aims to help people and companies create precise, high-quality datasets for computer recognition and machine learning applications. This tool's comprehensive collection of annotation capabilities makes it useful in various circumstances. One of V7 Labs' key features is its ability to deliver high-quality training data quickly and efficiently. The tool reduces the time and labor necessary to create reliable datasets by accelerating the annotation process with AI-assisted annotation.
Labelbox
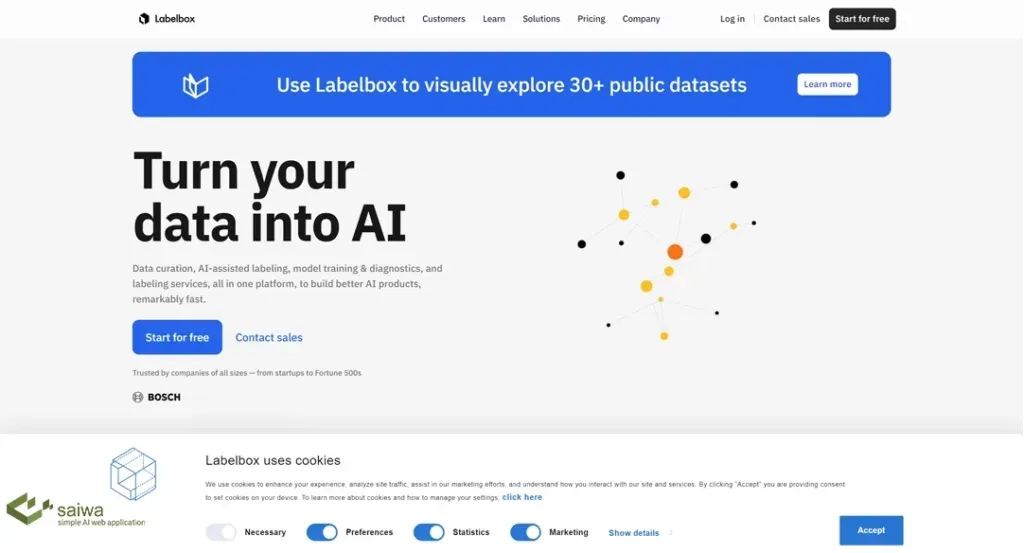
The AI-assisted label image annotation application tags images and videos on every platform quickly and accurately. Labelbox is simple to operate and offers a variety of options. Such features include quality assurance, performance analytics, and semantic segmentation. But you have to pay more for sophisticated features.
Scale Ai
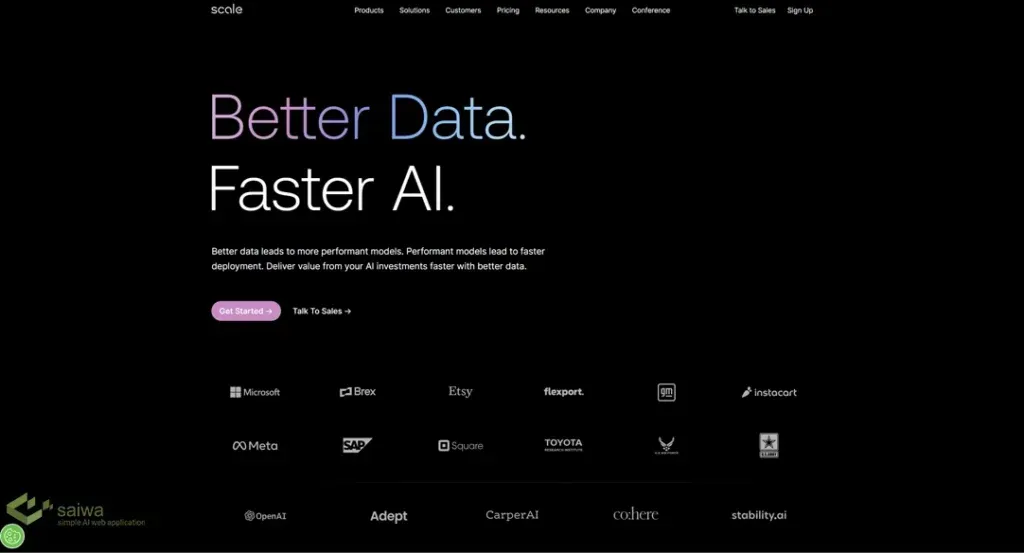
In addition to dataset management, document processing, and AI-assisted data annotation, Scale also provides ML-powered pre-labeling, an automatic quality control system, and data analysis for driverless cars. Several data formats are supported by this data annotation tool, which may be utilized for a range of computer vision applications like object detection, categorization, and text recognition.
CVAT
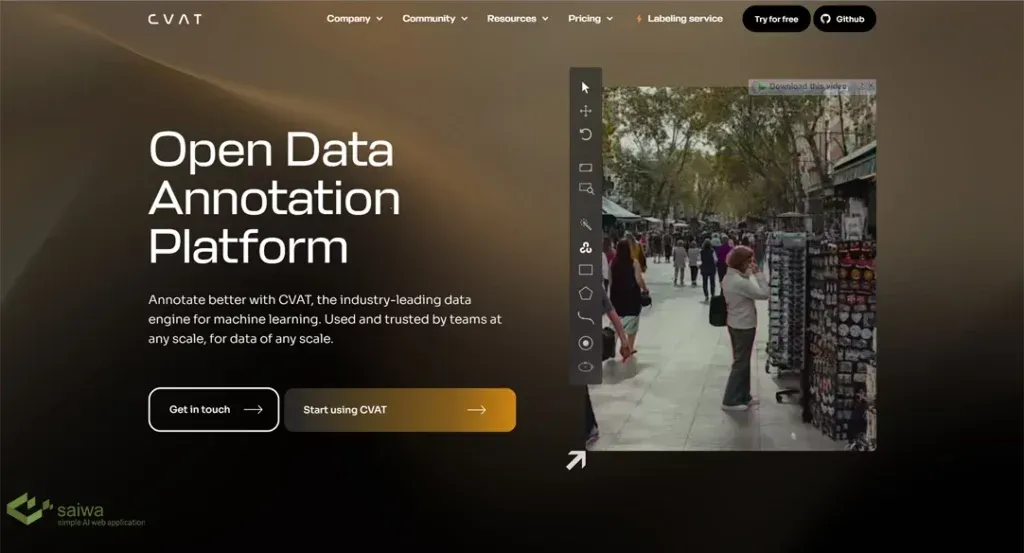
Intel's CVAT is a simple-to-use web image annotation tool. Using computer vision software, you can annotate images with words, lines, and shapes. The video annotation tool allows for computer vision tagging. Furthermore, semi-automatic annotation is available.
LabelMe
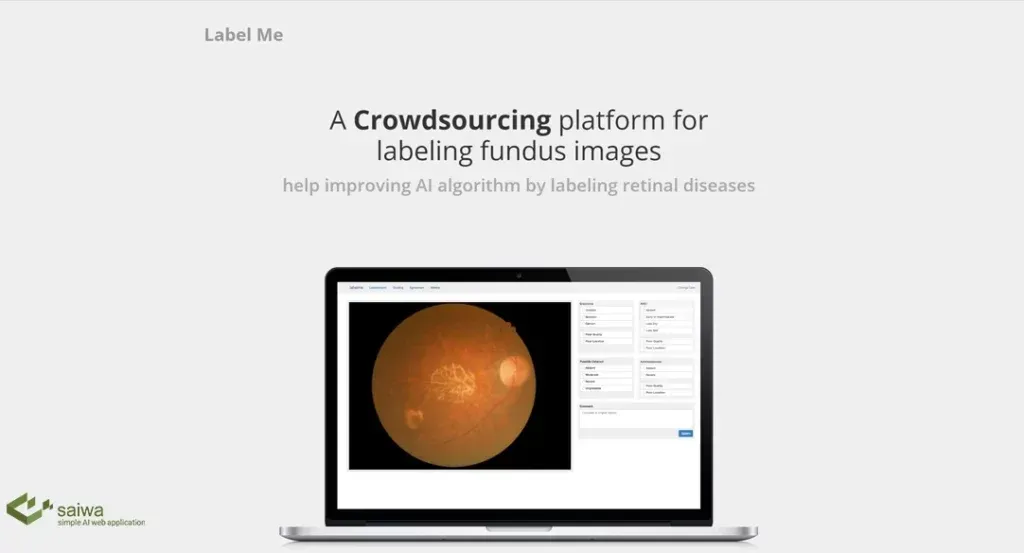
You can recognize images and videos for machine learning with this free tool for image annotation. It is capable of many different things. For instance, drawing rectangles around an object, a box, or a set of lines is an option. You must use JSON as a format, which is a drawback. Python expertise is also required.
SuperAnnotate
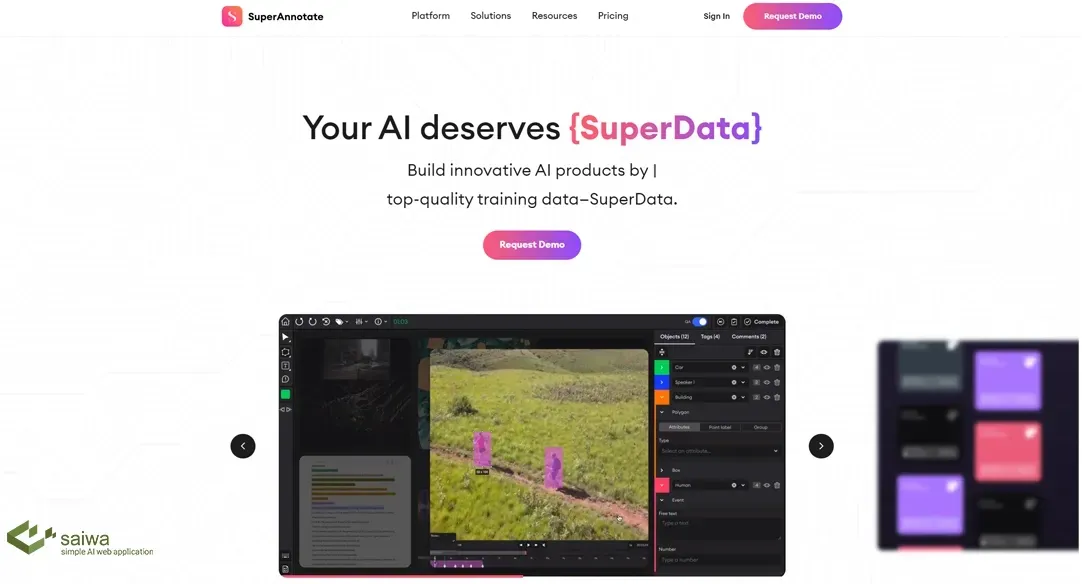
With the help of SuperAnnotate's robust annotation features, you may annotate documents quickly and with a few clicks. The program includes tools for image annotation, semantic segmentation of images with nearby pixels, keypoint tagging, video tracking, and more. It works excellent for accelerating computer vision tasks if you can afford it.
VoTT
VoTT is a free online image annotation tool that enables you to type on images, mark specific details, and insert patterns. The annotation picture tool is excellent for individuals on a restricted budget because it is free and open-source. Data can be imported and exported using cloud service providers. Nevertheless, Microsoft no longer offers support for the platform.
Saiwa
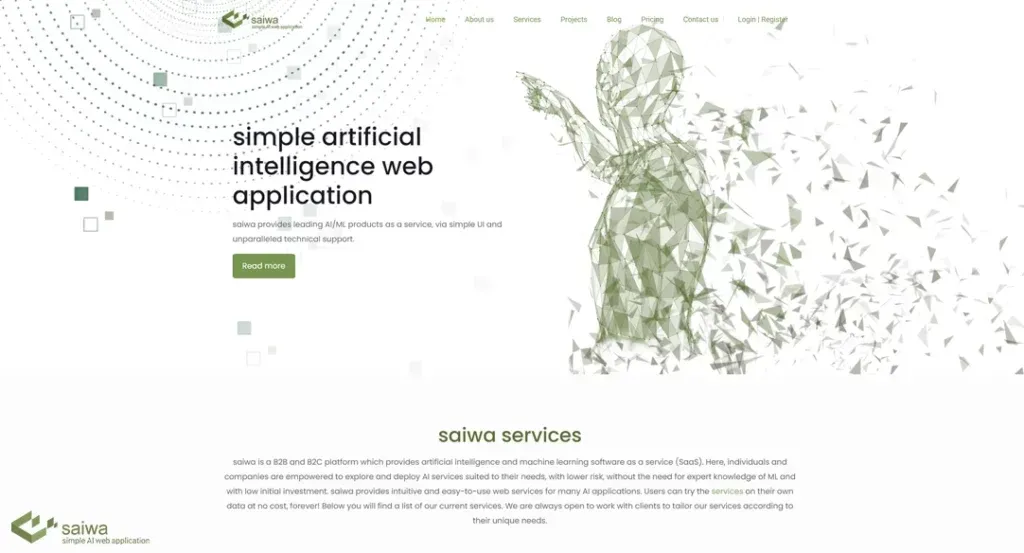
Saiwa is a company that provides a range of AI services, including image annotation. Saiwa's image annotation service offers a variety of annotation types, including:
Object detection involves drawing a bounding box or a boundary around the object of interest in an image and labeling it.
Image classification: This involves labeling an image with one or more categories or tags that describe the contents of the image.
Semantic segmentation involves labeling each pixel in an image with a corresponding class label, which can be used for image segmentation and object recognition tasks.
Saiwa's image annotation service can be used for various applications, such as training machine learning models, improving search algorithms, and enhancing image recognition systems.
Saiwa's image annotation service helps companies and organizations better understand and utilize their images' content by adding metadata and labels.
Supervise.ly
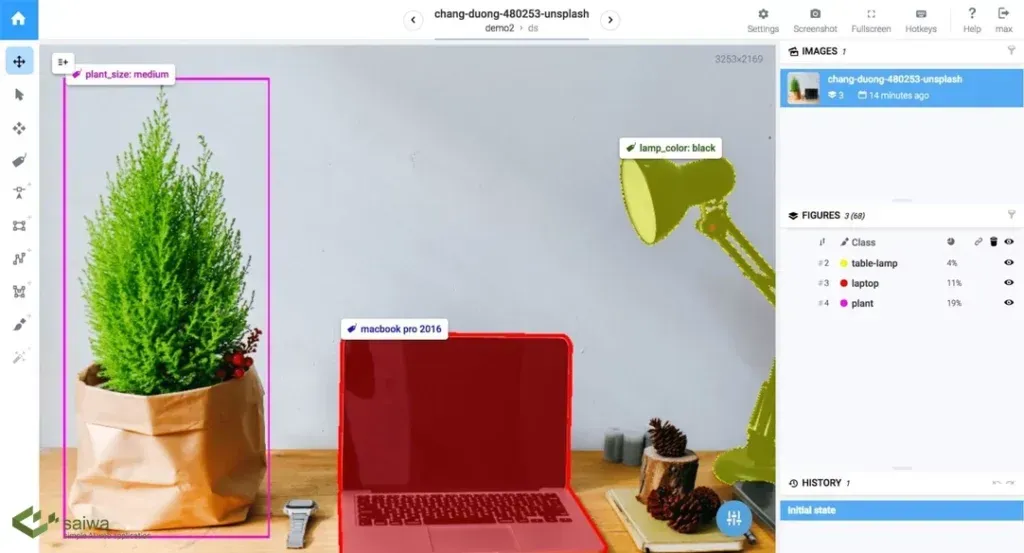
Supervisely is an AI-powered computer vision platform that provides tools for image annotation, training, and deployment. Image annotation is a critical step in training computer vision models, and Supervisely provides a set of annotation tools that make the process easy and efficient. In Supervisely, image annotation involves adding labels or annotations to images to identify and classify specific objects or regions of interest. These annotations can take various forms, such as bounding boxes, polygons, lines, points, or semantic segmentation masks. To annotate images in Supervisely, you can either use the built-in annotation tools or import annotations from other sources. The platform provides various annotation tools, including bounding box, polygon, point, line, and semantic segmentation tools, to help you quickly create accurate annotations.
Hive Data
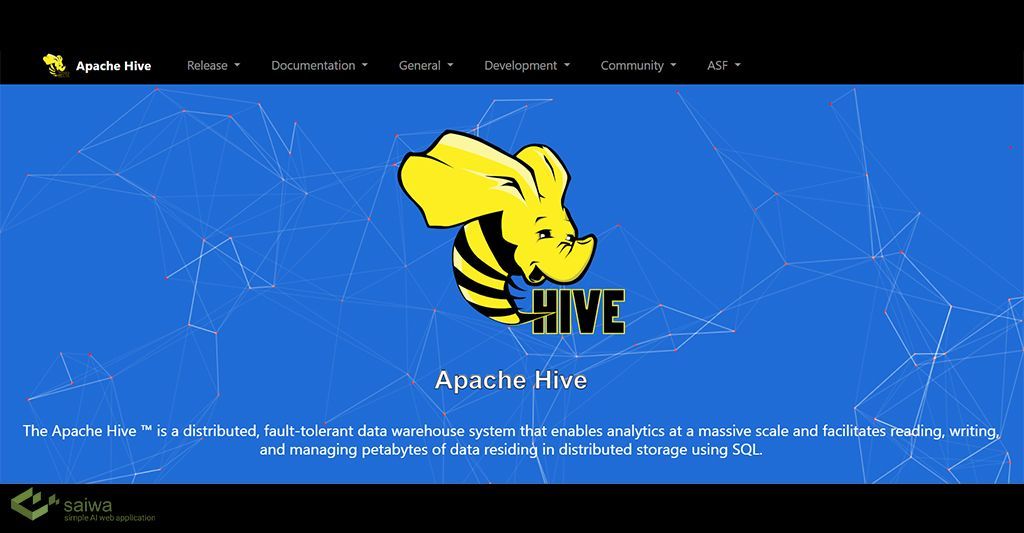
This option is a fully managed data annotation solution for sourcing and annotating training data for ML/AL models. This image annotation tool supports image, video, text, 3D point cloud annotation and data source. It also provides multi-frame object tracking, lines, and 3D panoptic segmentation in addition to basic annotation. Key features of this platform include:
Support for multiple data types
Available data sources
Fully managed data annotation service
The benefits of this platform are
It provides pre-trained models
Advanced project management workflow
Label Studio
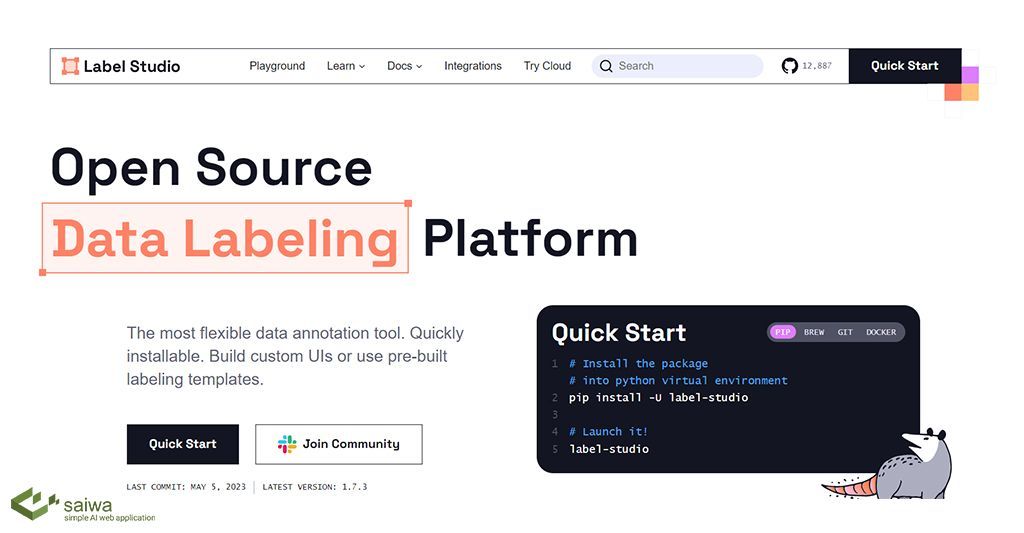
It is an open source data tagging tool that also includes annotation functionality. This is one of the Image Annotation Tools which is provides a simple user interface that allows you to tag different types of data such as text, audio, time series, video, and images and export the information to different model formats. Here are some of the key features of this platform:
Multi-user tagging, which ensures that every annotation you create is linked to your account and allows you to collaborate.
Focusing helps you work on multiple projects at once.
Customizable annotation templates allow you to customize the visual interface according to specific annotation needs.
Support for multiple data types including HTML, audio, images, text, video, and time series.
Import options allow you to import information from files, cloud storage, and archives.
Integration gives you the ability to integrate with machine learning models, visualize and compare predictions from multiple models, and then perform initial labeling.
You can use this platform to provide raw data and enhance existing training data to fine-tune your machine learning models.
VGG Image Annotator
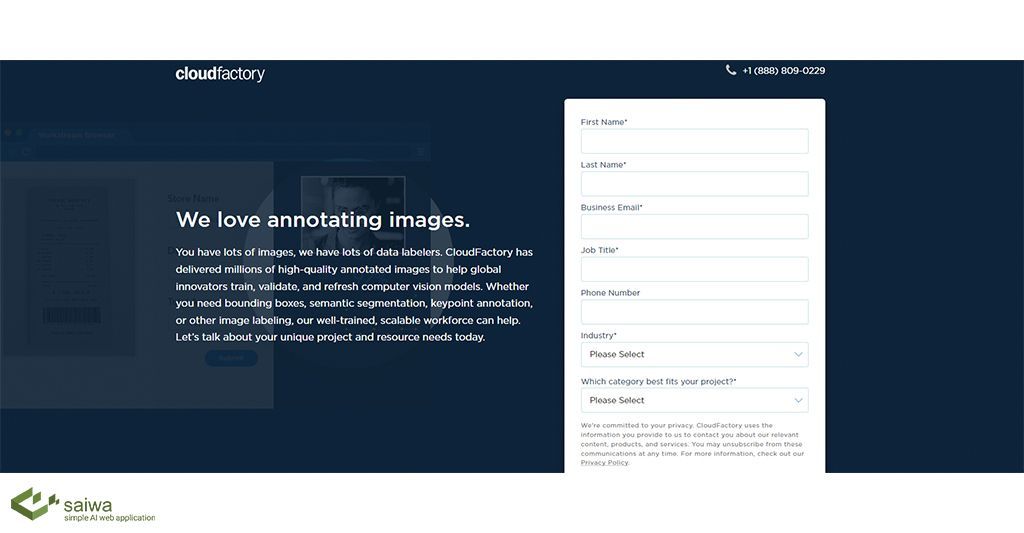
It is an annotation tool for manual annotation of image and video data developed in the VGG Image Geometry group. This tool can be used for both academic and commercial purposes. It is a lightweight tool based on HTML, JavaScript and CSS without any dependency on external libraries. It is a standalone HTML page that is less than 400 KB in size and can be run as an offline application in modern web browsers without any setup or installation process.
Makeense.ai
It is one of the other Image Annotation Tools which is a free and open source tool for tagging images. This tool does not need to be installed, is online and does not store any images. You can use this tool regardless of the operating system you have by simply visiting the website. This tool aims to reduce the time spent on tagging photos by using different artificial intelligence models that automate repetitive activities and provide recommendations. You can use this tool to easily and quickly prepare datasets for small computer vision projects and easily download prepared labels in different formats.
Data-loop
It's one of the solutions for picture annotation that combines a cloud-based annotation platform with embedded tools and automation to produce high-quality datasets. This platform considers the entire life cycle of artificial intelligence including annotation, model evaluation and model improvement using human feedback in the loop. It provides tools for basic computer vision tasks such as detection, classification, key-pointing, and segmentation. Data-loop supports image and video data.
Key features of this platform:
Model-assisted labeling
Support for multiple data types
Advanced team workflow with data indexing and efficient query system
Video support
Advantages of using this platform:
Automation and production pipeline using Python SDK and Rest API
Payment
This is one of the image annotation tools which is a fully managed data labeling back-platform that generates training data for computer vision models. This platform was established in 2015. Playment supports image and video data and offers a wide range of basic annotation tools such as bounding boxes, cubes, polygons or markers. According to the micro principle, the program divides up complex issues into manageable jobs and assigns them to its wide community of skilled annotators.
Features of this platform:
It's fully managed, businesses just need to share data and tagging guidelines
It provides the possibility of feature extraction
Document Management
Advantages of using this platform:
Features product comparison and competitor analysis
Development of advanced quality control tools
Suitable and robust for autonomous driving teams
Labelimg
It is one the image annotation tools which is a graphical image annotation tool for labeling objects using bounding boxes in images written in Python. You can export your annotations as XML files in PASCAL VOC format. In its default version this tool offers only one type of annotation: a bounding box or a rectangle shape. However, it is possible to add another form with a code using a GitHub page.
Key features of this tool:
Annotations are stored as XML fables in PASCAL VOC
Must be installed locally
Image annotation only
ImgLab
It is one of the image annotation tools which is an open-source, web-based image annotation tool. This tool provides several types of overlays such as points, circles, bounding boxes, polygons. It also supports different formats like Pascal VOC, XML, Idlib and COCO
Key features of this tool:
Local web-based versions
Basic features of the IDE
Support multiple types of tags and file formats
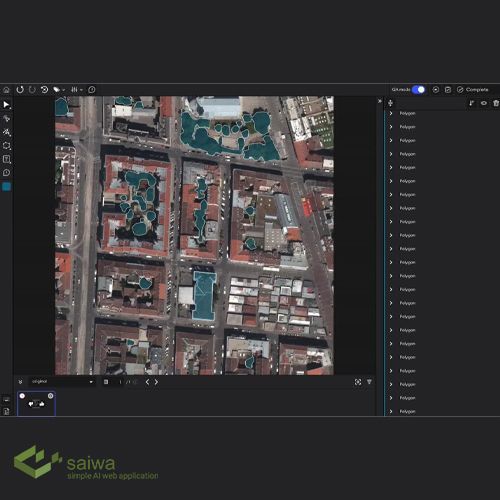
MarkUp.io
It is a real-time visual proofreading and commenting platform that enables users to easily request and provide textual feedback. With the help of this tool, you can annotate images, PDF files, videos, Word documents, live websites and other common file types.
In terms of Online image annotation, this tool supports a wide range of file formats such as JPG, JPEG, PNG, GIF, SVG, BMP, PSD, AI, EPS, TIFF, RTF, and PPTX. To make the proofreading process even easier, the tool has a Loom integration that gives you the ability to attach quick video descriptions to your comments. With this tool, there is no such thing as hard annotation.
Pastel
Pastel is an easy-to-use Online image annotation platform developed for marketing agencies and creative professionals who need to simplify their proofreading process.
The goal of this tool is to simplify creative work by providing a digital environment where teams can easily store, share, and review media fables. Pastel has live web, PDF and Online image annotation capabilities. This means that this platform can be used by designers, marketers, developers, content writers and other professionals who need to review their work. Another feature of this tool is that it supports multiple file types including JPGs, PNGs, and GIFs.
Data-loop
It is an integrated cloud-based blog image annotation tool with embedded tools and automation for generating high-quality datasets. This platform considers the entire life cycle of AI including annotation, model evaluation, and model improvement using human feedback in the loop. It provides tools for basic computer vision tasks including detection, classification, key-points, and segmentation. This platform supports image and video data.
Key Features:
Codel-assisted labeling
Support for multiple data types
Advanced team workflow with data indexing and efficient query system
Video support
Img Lab
It is an open source, web-based blog image annotation tools. This platform offers several types of labels such as points, circles, bounding boxes, polygons. It also supports various formats including dlib, XML, Pascal, VOC and COCO.
Key Features:
Local web-based versions
Basic features of the IDE
support various tag types and file formats
Roboflow Annotate
Used by over 250,000 engineers, this tool is a web-based blog image annotation tool that you can use for image labeling, object detection, classification, and segmentation. The tool has a powerful Label Assist feature that can automatically annotate images in your dataset using a previous version of your model or one of the 50,000 public models in the Roboflow Universe. This tool was developed with collaboration in mind and offers features uniquely designed for working with your team:
Image history; You can see how the annotations on an image have changed over time.
commenting on the image; Annotation team members can discuss annotations on an image.
Ontology locking; To ensure that annotators do not accidentally introduce new classes to your model.
Advanced image allocation features with review stage.
Annotator insights; It shows how many annotations have been made and the acceptance rate of those annotations is divided by annotator, time, and project.
Make Sense
It is a free and open-source computer vision annotation tool licensed under the GPLv3. The hosted version of this tool, at makense.ai, does not require registration or installation. This tool runs completely in the browser and your information never leaves your browser. You must save the annotations you make to your local storage after annotating the photographs. This tool supports export formats including YOO, VOC XML, and VGG JSON. You can annotate using bounding boxes, lines, points and polygons
COCO Annotator
It is a web-based image annotation and tagging tool available under the MIT license. It was created by Justin Brooks to aid in the training of keypoint, object, and object localization models.
Here we describe the features of this image annotation tool:
Dataset Labeling: This tool gives you the ability to label data using freeform curves, key points, and polygons. It provides the ability to track object instances and label image segments. Enables labeling of objects with isolated visible parts in an instance.
COCO Format: The tool saves and exports notes in COCO format to detect objects on a large scale.
Selection and Annotation- Using semi-trained models, this tool provides picture annotation.
Security: This tool gives you the ability to use an authentication system to secure your data.
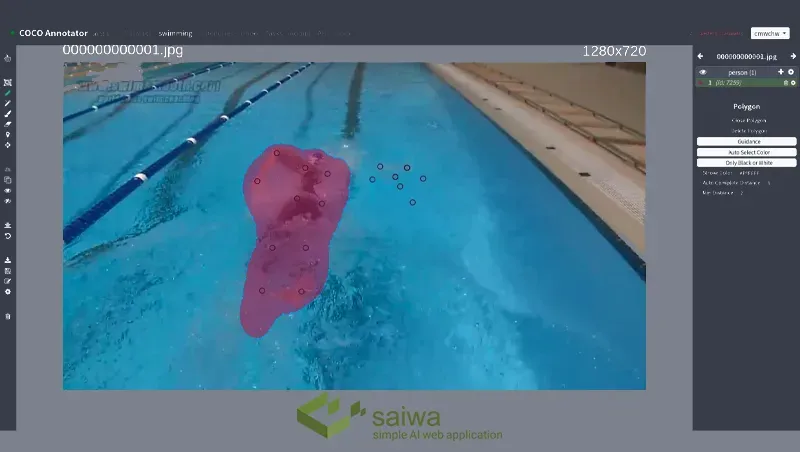
Encord Annotate
It is an automated annotation platform for AI-assisted image annotation, video annotation, and dataset management. It's the best option for teams looking for automated, semi-automated, or AI-assisted image and video annotation.
Key advantages and features of this tool are:
Annotations that are focused on use cases, such as SAR-specific features for geographical data and native DICOM & NIfTI annotations for medical imaging.
Support for annotation types- bounding boxes, polygons, polylines, image segmentation, key points, custom primitive objects and others.
Integrated data tagging services
Identify edge cases and gaps in your training data and generate augmented data to improve label quality
Easy collaboration, annotation management and QA workflow- to track annotator performance and increase label quality.
Online Image Annotation: Enhancing Computer Vision Through Precision
Labeling or tagging images with metadata, which contains important details about the objects, features, or patterns within the visual data is referred to as image annotation. In the field of computer vision, this exacting task is essential to the ability of machines to understand and interpret visual content accurately. Specifically, online image annotation has become a collaborative and dynamic method that is changing how we create annotated datasets for machine learning model training.
The significance of image annotation in computer vision cannot be overstated. As computer vision algorithms strive to mimic human visual understanding, annotated images serve as the bridge between raw visual data and algorithmic comprehension. In essence, annotations act as guideposts, teaching algorithms to recognize objects, identify boundaries, and understand contextual relationships within images. By leveraging online tools and platforms dedicated to online image annotation, the process becomes more streamlined, allowing for efficient collaboration among annotators and the rapid creation of large, diverse datasets.
The demand for accurately annotated datasets arises across various applications in computer vision. Object detection, facial recognition, and semantic segmentation are just a few examples where online image annotation plays a critical role. Through precise labeling, algorithms can learn to identify and differentiate between objects, making them capable of making informed decisions in real-world scenarios.
Moreover, the collaborative nature of online image annotation fosters a dynamic workflow. Multiple annotators can work simultaneously, enhancing productivity and contributing to the creation of high-quality datasets. This collaborative approach aligns with the evolving landscape of computer vision, where human-in-the-loop annotation becomes integral. By combining the expertise of human annotators with machine learning algorithms, the annotation process becomes not only more efficient but also more nuanced.
Online image annotation stands out as a vital activity that shapes the capabilities of artificial intelligence as we traverse the intricate landscape of computer vision. It also opens the door for novel applications that depend on precise visual data interpretation, thereby advancing the development of sophisticated machine learning models. Online image annotation continues to be a key component in the rapidly developing field of computer vision, propelling advancement and enabling the development of intelligent visual systems.
FAQ
Why do we need image annotation?
Image annotation is mostly used to recognize objects and boundaries and to segment images such as the meaning or understanding of the whole image. For each of these applications, training, validating and testing a machine learning model requires a significant amount of data to achieve the desired result.
How does image annotation work?
Image annotation is the act of labeling images for training artificial intelligence and machine learning models. It usually involves human annotators using image annotation tools to label images or tag related information.
Why are annotation tools important?
Data annotation tools can help manage the quality control and validation process. Ideally, the QC tool is built into the annotation process itself. For example, real-time feedback and the initiation of problem tracking at the time of annotation are important.
What is the difference between image tagging and image annotation?
Annotating data is a prerequisite for training machine learning models while labeling aims to find meaningful features in a dataset. Annotation makes it easier to identify relevant material, and labeling helps identify patterns to train algorithms.
What is the most important part of annotation?
As you annotate, you should note the author’s main points, changes in the message or point of view of the text, key areas of focus, and your thoughts as you read.