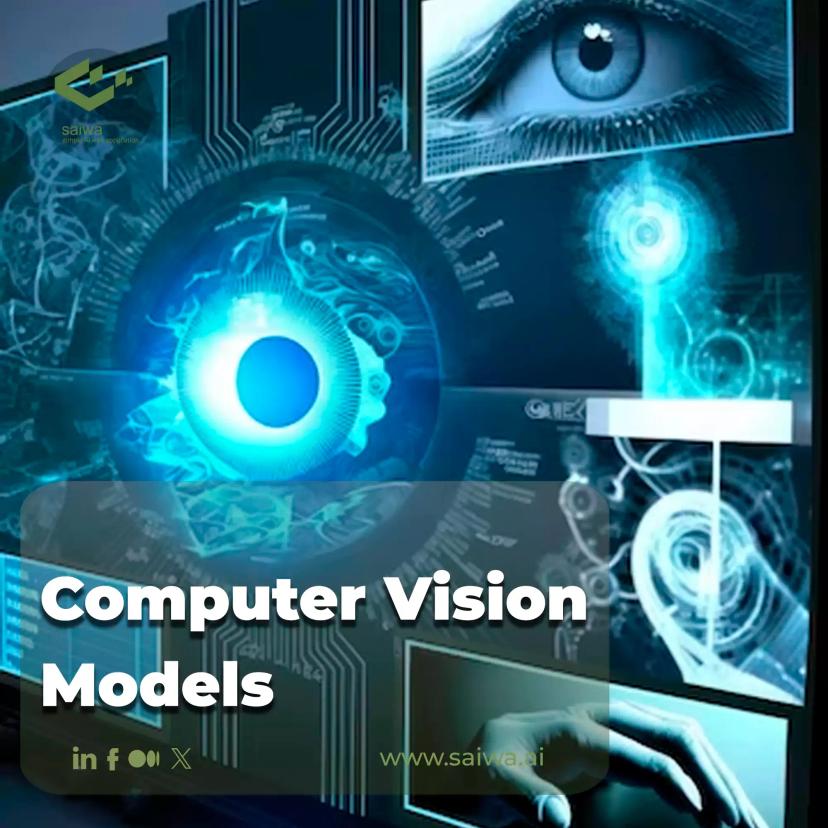
Explore Top Computer Vision Models | A Comprehensive Guide
Computer vision is an artificial intelligence branch that empowers computers to comprehend and interpret the visual world. It entails deploying algorithms and machine learning models to scrutinize and interpret visual data from various sources, including cameras.
Several computer vision models exist, including feature-based models, deep learning networks, and convolutional neural networks. These models can learn and recognize patterns and features in the visual environment. They can be trained on abundant quantities of labeled and image data. This article presents a detailed summary of computer vision models so that you acquire an in-depth comprehension of them.
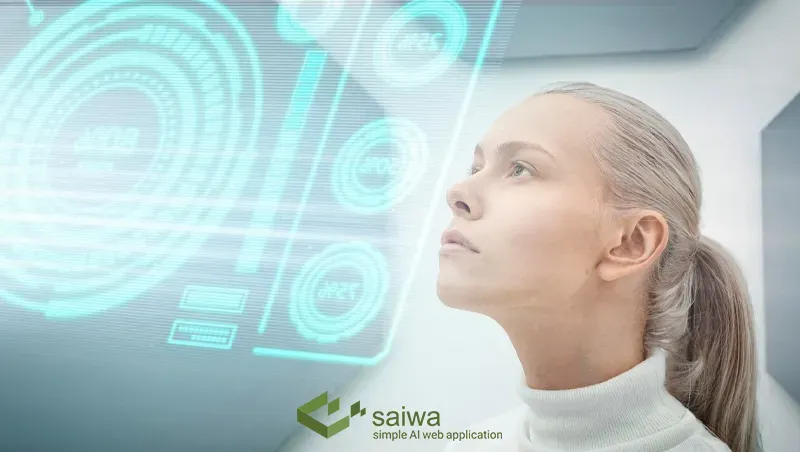
What are computer vision models?
Computer vision is a branch of computer science that studies how artificial intelligence algorithms can be used to teach machines how to see and interpret video and images. At the basis of this technological innovation is an architecture known as convolutional neural networks.
These neural networks use an image to analyze the colors at each pixel to decompose the image into a set of data, and then compare these data sets to known data for classification purposes.
The network then quickly ignores any known data sets that do not match as it searches for a classification. With each pass, the possibilities for what the image represents are reduced until the computer reaches a precise definition of what is in the image or video.
Various computer vision models can use this interpretive data to make decisions and automate tasks.
Read Also: Exploring Diverse Computer Vision Applications
Examples of Computer Vision Models and How Do They Work?
Self-driving cars are an excellent example of computer vision models. Equipped with cameras, they constantly scan the environment to detect nearby objects. The received information is then used to plan the vehicle's route and direction.
Computer vision models that utilize deep learning rely on iterative image analysis to continuously enhance their knowledge over time. Additionally, top-performing computer vision models are self-teaching, wherein the analysis output of the computer is enhanced as it is utilized more.
When developing a computer vision model, gather high-quality images that closely resemble what your system needs for precise analysis. These images must be of exceptional quality to ensure that your model functions as intended.
For instance, if you are designing a self-driving car system, the images you collect should depict cars, trash cans, caution cones, and stop signs, all of which function as landmarks on the road, just as they do in the real world.
As an example, when designing a system for reading and analyzing invoice documents, it is recommended to use authentic invoice images rather than prototypes or templates to ensure accurate results.
The next step is annotation. At this stage, you need to define what is in these images so that the device can associate these objects with these definitions and decide based on this interpretation.
To train your model effectively, use thousands of annotated images. A larger dataset enhances the model's accuracy and effectiveness in artificial intelligence applications.
The inclusion of high-quality and detailed images ensures the provision of as much information as possible in the system.
If you are not planning to create a computer vision model, this summary can provide insight into how the technology functions.
Read Also: Top Computer Vision Libraries
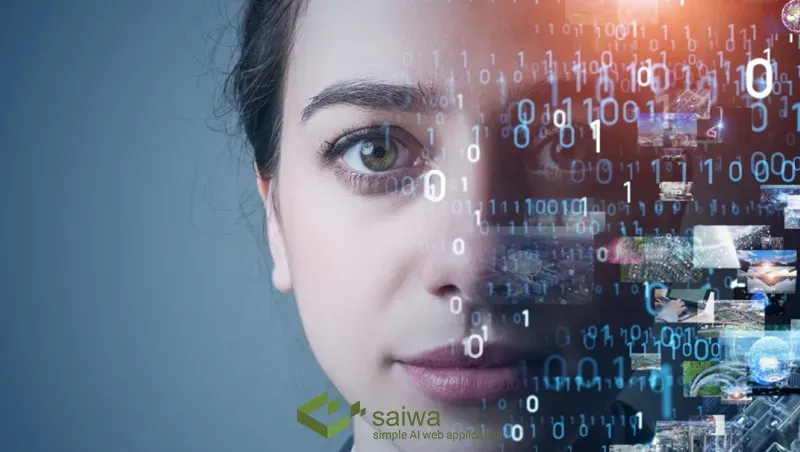
Types of computer vision models
Different types of computer vision models assist in answering inquiries concerning an image, such as identifying the objects within it, locating said objects, pinpointing key object features, and determining which pixels belong to each object. These questions are answered by developing various types of deep neural networks, which can then be utilized to tackle challenges such as counting cars in an image or identifying if an animal is sitting or standing. In this section, we overview some prevalent computer vision models and their applications.
It is important to note that computer vision model output typically includes a label and a confidence score, reflecting the probability of accurately labeling the object. Here are some different types of computer vision models.
Read Also: Vision Transformer | A Paradigm Shift in Computer Vision
Image Classification
Ai Image classification is a model that identifies the most significant object class in an image. In the field of computer vision, each class is referred to as a label. The model receives an image as input and outputs a label with the model's confidence level in that particular label compared to others. Also, it is important to note that for image classification tasks, DNN does not provide the object's location in the image. Therefore, use cases that require this information for tracking or counting objects necessitate the use of an object detection model, which is further elaborated below.
Object Detection
Object Detection DNNs are crucial for determining the location of objects. Intuitively, object location is key to inferring information from an image. They provide a set of coordinates (bounding box) that specify the area of an input image that contains the object, along with a label and a confidence value.
For example, we can draw traffic patterns by counting the number of vehicles on a highway. An application's functionality can be extended with a classification model supported by an object recognition model. For instance, one could import a portion of the image connected to the bounding box from the recognition model to the classification model. This way, the number of available trucks can be recognized in the image, surpassing other cars.
If it is necessary to differentiate the pixels that pertain to the identified object from those in the remaining part of the image, segmentation will be required, and we will elaborate on this further.
Image segmentation
As previously mentioned in the different types of computer vision models, certain tasks require a precise understanding of an image's shape. This involves creating a boundary at the pixel level for each object, which is achieved through image segmentation. DNNs classify every pixel in the image based on object type in semantic segmentation, or individual objects in instance segmentation.
Note: Semantic segmentation is commonly used for virtual backgrounds in teleconferencing software, where it distinguishes pixels that belong to a person from those that do not, and separates them from the background.
We can use image segmentation to identify the pixels belonging to each object in an image. However, determining the relative position of objects in the image, such as locating a person's hand or a car's headlights and bumper, requires information about specific areas of the object. However, determining the relative position of objects in the image, such as locating a person's hand or a car's headlights and bumper, requires information about specific areas of the object. To achieve this, we need to track object markers. We will discuss this model in detail later.
Read Also: The difference between computer vision and image processing
Object Landmark Detection
Object landmark detection involves labeling key points within images to capture important features of an object. One possible model for our work is the pose estimation model, which identifies key points on the body, including the body, shoulders, and elbows.
It is worth noting that a helpful app utilizing these key points is available to ensure proper form during exercise and athletics.
Computer Vision Models in Aviation
Computer vision models and capabilities is improving air travel safety and efficiency by enabling machines to understand digital images. The applications are very broad and include recognition and detection of airplanes, airport security and management, plane identification, autonomous flying of drones, and aerial robotics.
Aircraft Inspection and Maintenance
The usage of computer vision models and capabilities in aircraft inspection and maintenance promotes normal aircraft operations. Technicians are leveraging this technology for detecting problems and recognizing the damages of patterns that are difficult to see with naked eye. This has the potential to improve safety and reduce accidents.
Additionally, computer vision models and capabilities can save time and decrease labor costs for manual inspection. Technicians and engineers can inspect cracks or other damage, or use this technology for landing gear inspection.
Intelligent Baggage Handling
For baggage handling in airports, computer vision models and capabilities are able to read labels automatically to recognize their trolleys and locations. Computer vision systems equipped with cameras can scan tags and connect them by using the airline’s database. Another application of visual deep learning improves airport ground operations by locating vehicles like baggage tugs and carts, leading to more efficient baggage handling and cart management.
Face Recognition at Airports
Computer vision models and capabilities in recognition systems are implemented at airports for passenger identification. These systems utilize AI algorithms to compare a passenger's facial features with a database of pre-existing images. The detection and recognition process is often accomplished using conventional IP cameras. The application of this technology facilitates a more efficient boarding procedure and bolsters security by confirming passenger identities and, potentially, identifying individuals of interest.
Read More: AI in Aviation | Driving Efficiency and Safety in the Skies
The best computer vision models
No two computer vision models can be considered equal, as each algorithm possesses unique features that suit specific application requirements. Consequently, the best computer vision software for your organization may not align with those of another entity, searching for the optimal model subjective.
Nevertheless, the best computer vision platforms share fundamental characteristics. For instance, the process of training a deep learning machine can be arduous and time-consuming. This is why the top computer vision models are pre-trained, saving valuable time and resources.
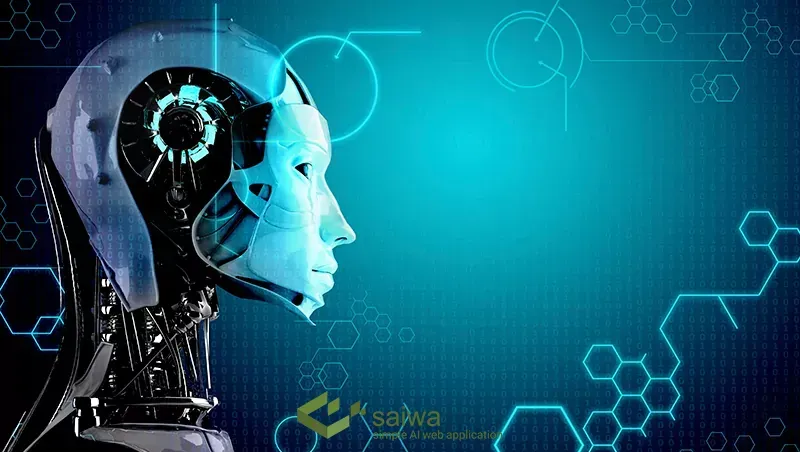
Generative Adversarial Networks (GANs) for Computer Vision
Generative Adversarial Networks (GANs) have emerged as a powerful class of deep learning models that have revolutionized various types of computer vision tasks. GANs operate on the principle of training two neural networks simultaneously: a generator network and a discriminator network, which engage in an adversarial game.
Principles of Generative Adversarial Networks
Principles of Generative Adversarial Networks The generator network is responsible for creating synthetic data, such as images or videos, while the discriminator network aims to distinguish between real and generated data. Through this iterative process, the generator learns to produce increasingly realistic and high-quality data, while the discriminator becomes better at distinguishing real from fake data.
Applications in Image Generation and Enhancement
Applications in Image Generation and Enhancement GANs have demonstrated remarkable success in various types of computer vision applications, particularly in image generation and enhancement tasks. They can be used to generate photorealistic images from scratch, enabling applications in fields like computer-aided design, virtual reality, and media production.
Style Transfer and Domain Adaptation
Style Transfer and Domain Adaptation Additionally, GANs are widely used for style transfer and domain adaptation tasks. Style transfer involves transferring the artistic style of one image onto another, allowing for creative applications in digital art and media editing. Domain adaptation, on the other hand, enables the translation of data from one domain to another, facilitating using types of computer vision models in diverse environments and scenarios.
Deployment of computer vision models
When deploying computer vision models, organizations should consider best practices such as edge deployment. This means deploying models close to where the data originates, eliminating the need for a long and complex computer vision pipeline that can lead to data loss or corruption. Thorough and regular testing and monitoring of the computer vision model are also crucial for successful project implementation.
When deploying computer vision models, it's crucial to consider MLOps for successful management. MLOps combines machine learning and continuous improvement processes that are associated with DevOps. By following a series of steps, your organization can ensure the smooth launch of any machine learning product.
Specific Applications of Computer Vision Models in Image Processing Domains
Computer vision models are revolutionizing various image processing domains. They act as powerful tools for extracting useful information from images, automating tasks, and driving innovation. Here, we explore a few specific applications:
Medical Imaging Analysis
Computer vision models in image processing are transforming medical diagnosis. By analyzing X-rays, MRIs, and CT scans, these models can detect abnormalities, classify diseases, and even predict patient outcomes. This translates to faster and more accurate diagnoses, providing earlier intervention and improved patient care.
Industrial Defect Detection
On factory production lines, computer vision models in image processing ensure product quality by surface defect detection. They can inspect products for defects like cracks, scratches, or misalignments with high precision. This real-time analysis helps identify and remove faulty products early in the production process, minimizing waste and improving overall production efficiency.
Read Also: AI in Mineral Exploration | Transforming the Future of Resource Discovery
Satellite Image Processing
Computer vision models in image processing play a crucial role in processing satellite imagery. They can classify land cover types, monitor deforestation, and even detect changes in weather patterns. This information is valuable for environmental monitoring, resource management, and disaster response efforts.
Autonomous Vehicles
Self-driving cars rely on computer vision models in image processing. These models analyze camera feeds to detect and track objects like pedestrians, vehicles, and traffic signs. This real-time understanding of the environment is essential for safe and reliable autonomous navigation.
Facial Recognition
Used in security and authentication, computer vision models can identify and verify individuals based on facial features. Applications range from unlocking smartphones to advanced surveillance systems. Among the various types of computer vision, facial recognition stands out for its ability to support personalized marketing by identifying returning customers in retail environments.
Retail Analytics
Retail stores employ computer vision models for customer behavior analysis, shelf management, and checkout automation. These applications help enhance customer experience and optimize store operations. Advanced systems are also capable of forecasting inventory requirements by analyzing sales trends in real time.
Optical Character Recognition (OCR)
Computer vision models facilitate the extracting and digitizing of text from images or scanned documents. OCR is widely used in automating data entry, processing invoices, and digitizing historical archives. As one of the core types of computer vision, OCR integration into mobile apps allows users to translate text in real time simply by pointing their camera at it.
Sports Analytics
In the sports industry, computer vision models analyze player movements, track ball trajectories, and evaluate team strategies. This helps coaches and analysts make data-driven decisions. Among the many types of computer vision applications, those in sports are particularly valuable for broadcasters providing real-time insights and enhancing the viewing experience with augmented overlays.
The Role of Computer Vision Models in Agriculture
Computer vision models are offering unprecedented insights and automation across the entire farming process. From planting to harvesting, these sophisticated systems are transforming food production, making it more efficient, sustainable, and productive.
Crop Monitoring and Health Assessment: Computer vision models analyze images captured by drones, satellites, or ground-based cameras to assess crop health. They can detect early signs of disease, nutrient deficiencies, or pest infestations, often before they are visible to the unassisted eye. This allows for timely interventions, reducing crop loss and optimizing resource allocation.
Precision Farming: Computer vision models enable precision agriculture by mapping fields and identifying variations in soil composition, moisture levels, and crop growth. This information helps farmers to target inputs such as fertilizers and water precisely where they are needed, minimizing waste and environmental impact.
Automated Harvesting: Computer vision in agriculture is also used in robotic harvesting systems. These robots have the ability to identify ripe fruits and vegetables, selectively picking them without damaging the produce. This automates the harvesting process, reducing labor costs and ensuring consistent quality.
Yield Prediction: By analyzing images of crops throughout the growing season, computer vision models can predict yields with greater accuracy. By using this information, farmers can make informed decisions about marketing and resource planning.
Weed Detection and Control: Computer vision models can identify crops and weeds, enabling targeted weed control. This reduces the need for broad-spectrum herbicides, minimizing environmental impact and promoting sustainable farming practices.
The Impact of Computer Vision Satellite Imagery on Global Challenges
The integration of computer vision with satellite imagery is transforming the manner in which our planet is observed and understood, offering revolutionary tools to address environmental, agricultural, and societal challenges. By analyzing vast datasets from satellites, this technology provides insights that were once unimaginable.
Environmental Protection: The use of computer vision satellite imagery facilitates the precise monitoring of deforestation, desertification, and biodiversity changes. This supports conservation efforts by identifying vulnerable ecosystems and allowing stakeholders to implement effective strategies to protect natural habitats.
Disaster Preparedness and Response: The integration of satellite data with computer vision techniques helps predict and mitigate risks associated with natural disasters like floods, wildfires, and hurricanes. By providing real-time analysis of affected areas, computer vision satellite imagery ensures faster and more efficient disaster response, ultimately saving lives and resources.
Advancements in Agriculture: Computer vision satellite imagery is a valuable tool for farmers and agricultural organizations, facilitating the monitoring of crop health, the detection of pest infestations, and the optimization of irrigation systems. These insights improve food security by maximizing yields while minimizing environmental impact.
Urban Development: Urban planners use this technology to track infrastructure growth, assess land use, and manage population density. This data-driven approach helps design cities that are not only more sustainable but also better equipped to meet the needs of growing urban populations.
Climate Change Research: Satellite imagery powered by computer vision provides critical data on global warming indicators, such as rising sea levels and melting ice caps. These insights drive international efforts to combat climate change and promote sustainability.
The Role of Hybrid Models in Computer Vision
Hybrid models in computer vision combine the strengths of different methodologies to enhance performance and accuracy. These models leverage the capabilities of traditional image processing techniques alongside modern machine learning approaches. Understanding the types of computer vision models is crucial for developing efficient systems. Here’s a breakdown of hybrid models and their components:
Traditional Methods: These include edge detection, feature extraction, and classical machine learning algorithms. They provide foundational techniques that can be integrated into hybrid systems.
Deep Learning Techniques: Neural networks, especially convolutional neural networks (CNNs), are pivotal in modern computer vision. By incorporating deep learning, hybrid models can learn complex patterns from large datasets.
Feature Fusion: Hybrid models often utilize feature fusion techniques to combine traditional features with those learned by neural networks. This enhances the model's ability to interpret images more effectively.
Ensemble Approaches: Some hybrid models implement ensemble techniques where multiple models of various types of computer vision models are combined. This can lead to improved robustness and performance in tasks like object detection and classification.
Applications: Hybrid models are particularly beneficial in applications requiring high accuracy, such as medical image analysis and autonomous driving, where understanding the nuances of image data is critical.
By exploring the various types of computer vision models, developers can create more robust solutions that meet specific challenges in the field. Understanding these combinations allows for innovative approaches in computer vision applications.
Comparison of Cloud-Based and On-Device Computer Vision Models
The choice between cloud-based and on-device computer vision models depends on the specific application, performance requirements, and available resources. The following comparison illustrates the relative merits of each approach:
Cloud-Based Models
These models operate on remote servers and rely on internet connectivity.
Cloud-based systems offer the advantage of scalability, allowing them to handle massive datasets and complex computational tasks, including training deep-learning models.
Regular updates and access to extensive resources improve the efficiency of these computer vision models.
However, data transmission to the cloud can introduce latency, rendering them unsuitable for real-time applications. Privacy concerns may also arise when handling sensitive information.
On-Device Models
On-device models run locally on edge hardware like smartphones, IoT devices, and embedded systems.
They offer low-latency processing, essential for real-time applications like robotics, AR, and autonomous systems.
These computer vision models ensure greater privacy since data doesn’t leave the device.
However, on-device models are often constrained by hardware limitations, which requires the use of lightweight or optimized architectures.
Key Considerations
Performance: Cloud-based solutions excel in tasks requiring heavy computation. On-device models prioritize speed and responsiveness.
Connectivity: Cloud-based models require internet access, whereas on-device systems function offline.
Data Privacy: On-device solutions reduce privacy risks compared to cloud alternatives.
Future Trends and Advancements in Computer Vision Deep Learning Models
The field of computer vision deep learning models is rapidly evolving, driven by advances in computational power, algorithm development, and the availability of larger and more diverse datasets. One emerging trend is the integration of computer vision models with other AI technologies, such as natural language processing and speech recognition, enabling multimodal analysis and more sophisticated applications. Another area of focus is the development of more efficient and lightweight computer vision deep learning models that can be deployed on edge devices and embedded systems. This would enable real-time processing and decision-making without relying on cloud-based infrastructure, leading to faster response times and improved privacy and security.
Read Also: Top 10 Transformative Computer Vision Trends In 2024
Can Computer Vision Models Learn from Each other?
Traditionally, training computer vision models have been a data-hungry endeavor. These models require massive datasets meticulously labeled with object descriptions. But what if, instead of solely relying on human-curated data, computer vision models could learn from each other?
This intriguing concept, known as collaborative learning, is gaining traction in the world of AI. Imagine a scenario where models trained on different datasets, say one focused on identifying cars and another on recognizing pedestrians, could share their knowledge. The car model might identify an object with wheels and a windshield, while the pedestrian model recognizes a bipedal figure. Through collaboration, these models could refine their understanding, potentially leading to improved accuracy and a broader range of object recognition capabilities.
Collaborative learning offers several potential benefits for computer vision models. Firstly, it could significantly reduce our reliance on vast, labeled datasets. This is a time-consuming and expensive process, and collaborative learning could make training computer vision models more efficient and accessible. Secondly, it holds promise for creating "generalist" computer vision models capable of handling diverse tasks. Imagine a model that, by learning from various specialized computer vision models, can not only recognize a car but also understand its interaction with pedestrians, leading to safer autonomous vehicles.
However, challenges remain. Developing effective communication protocols between computer vision models with different architectures and training backgrounds is crucial. Additionally, ensuring the quality and security of the knowledge being shared is paramount to avoid perpetuating biases or introducing errors in computer vision models.
Explainable AI in Computer Vision Models
Computer vision models have experienced rapid advancement and causing a wide range of applications from autonomous vehicles to medical image analysis. Yet, their intricate decision-making processes often remain shrouded in mystery, posing challenges to trust and accountability. This is where Explainable AI (XAI) emerges as a critical tool.
XAI tries to illuminate the black box of computer vision models, providing insights into the factors influencing their outputs. Such transparency is paramount in sectors like healthcare, where understanding the rationale behind a model's disease diagnosis is crucial for both medical professionals and patients. Similarly, in the realm of autonomous vehicles, comprehending the decision-making process of a model can significantly enhance safety and reliability.
Beyond trust and accountability, XAI plays a pivotal role in addressing bias in computer vision models. These models are trained on extensive datasets that may inadvertently contain biases. By shedding light on the model's decision-making process, XAI facilitates the identification and mitigation of these biases. As computer vision models continue to evolve in complexity, the demand for explainability will only intensify.
Ultimately, XAI is instrumental in bridging the gap between humans and complex computer vision models, fostering trust, transparency, and accountability.
Attention Mechanisms in Computer Vision Models
Attention mechanisms have significantly improved the performance of computer vision deep learning models by allowing them to focus on the most relevant parts of an image when making predictions. This concept, originally popularized in natural language processing (NLP), has become important in developing more accurate and efficient computer vision models. By mimicking human-like visual attention, these mechanisms enable the models to prioritize crucial features, ensuring better understanding and interpretation of visual data.
Enhanced Focus
Traditional computer vision deep learning models process the entire image uniformly, which can be computationally expensive and less effective, especially when dealing with high-resolution photos or complex scenes. Attention mechanisms enable these models to allocate more computational resources to the most critical regions of an image, such as the edges, textures, or objects of interest, enhancing performance without a proportional increase in computational costs. This approach not only speeds up the processing but also allows the model to be more precise in its predictions.
Improved Feature Extraction
In computer vision deep learning models, attention mechanisms help improve feature extraction by weighting the importance of each pixel or region in an image. This selective focus allows the models to learn more nuanced patterns, leading to better object detection, segmentation, and classification results. For instance, in tasks like facial recognition, attention mechanisms can help the model focus on key facial features such as eyes, nose, and mouth, improving accuracy even in challenging conditions like low light or partial occlusion.
Applications in Complex Scenarios
Attention mechanisms are particularly beneficial in complex visual tasks where multiple objects or overlapping features are present. By guiding computer vision deep learning models to prioritize relevant visual information, they achieve higher accuracy in scenarios like medical imaging, autonomous driving, and facial recognition. In autonomous vehicles, for example, attention-based models can more accurately identify pedestrians, other vehicles, and traffic signs amidst cluttered backgrounds, ensuring safer and more reliable decision-making processes.
Challenges in Developing Computer Vision Models
The development of computer vision models presents numerous challenges, each of which has the potential to impact the efficiency and effectiveness of the models. It is therefore essential that these issues are addressed if the field of computer vision is to advance and if practical, scalable applications are to be achieved.
Data Quality and Annotation
One of the most significant challenges in computer vision is the acquisition of high-quality datasets. Many types of computer vision tasks, such as object detection or semantic segmentation, require meticulously labeled datasets. This process is labor-intensive, expensive, and often limited by the availability of diverse, unbiased data.
Computational Complexity
The training of computer vision models requires the use of advanced hardware, including high-performance GPUs and extensive memory. These requirements often restrict accessibility for smaller organizations or projects with limited budgets. Furthermore, the scaling of models for large datasets can significantly increase the computational load, making model optimization a critical factor in efficient development.
Model Generalization and Domain Adaptation
It is a common challenge for models to generalize across diverse environments. Factors such as changing lighting, different camera angles, or noise can significantly reduce performance. This limitation is especially critical for applications like autonomous driving, where adaptability is essential.
Scalability and Real-Time Processing
Scaling models for large-scale deployment or real-time applications introduces a number of additional challenges. Latency, data throughput, and infrastructure demands make consistent performance difficult to achieve, particularly in dynamic environments. To overcome these issues, cloud and edge computing solutions are often required to balance performance with speed and scalability.
Ethical Considerations and Privacy Concerns in Computer Vision Deep Learning Models
The widespread deployment of computer vision deep learning models raises important computer vision ethics and privacy concerns. These models can potentially be used for mass surveillance, invasions of privacy, and the unauthorized collection and exploitation of personal data, including biometric information. There are also concerns about the potential for algorithmic bias and discrimination in computer vision deep learning models. If the training data is skewed or lacks diversity, the models may perpetuate societal biases or exhibit unfair behavior toward certain groups or individuals.
To address these concerns, it is crucial to establish robust ethical frameworks, data privacy regulations, and governance mechanisms for the responsible development and deployment of computer vision deep learning models. This includes ensuring transparency, accountability, and the protection of individual rights and civil liberties.
Read Also: MLOps platform | A shortcut to the data-driven way
Conclusion
As mentioned in this article, one of the most challenging and important areas of innovation in artificial intelligence is computer vision. Although machines have always been good at processing data and performing advanced calculations, image, and video processing are completely different processes. When humans look at an image, complex functions give their brains the ability to assign labels and definitions to each object in the image and to interpret what the image shows. It is very difficult for a computer to achieve this level of intelligence, but developments are being made in this direction.