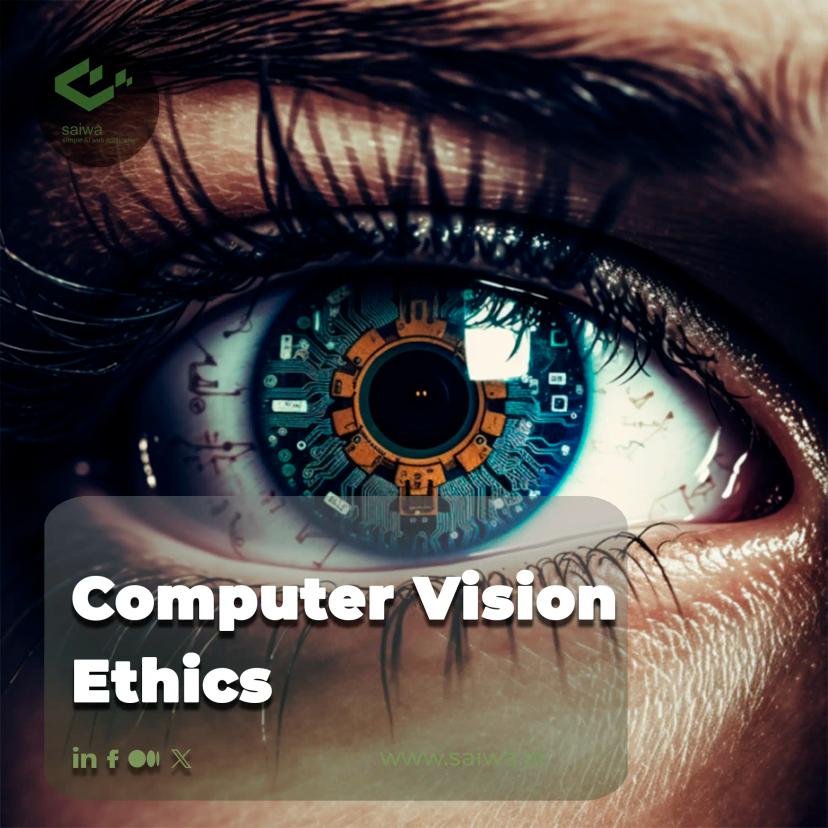
Computer Vision Ethics | Everything You Need to Know
The field of computer vision has advanced rapidly in recent years with innovations in deep learning and neural networks, enabling computers to identify, analyze, classify, and process images and videos. However, these powerful technologies also raise critical ethical questions about proper standards, controls, and oversight needed to guide responsible development and deployment. As computer vision increasingly permeates high-impact areas like surveillance, security, recruitment, credit decisions, autonomous vehicles, and more, the stakes around ethical use grow. This blog post examines key computer vision ethics considerations essential to the trustworthy and socially beneficial evolution of computer vision.
Ethical Considerations in Image Recognition
Image and facial recognition models rely on analyzing human biometric data sourced from images and video feeds. Training robust models requires mass datasets with personal information that can enable the identification of individuals without consent. This risks privacy violations or misuse if adequate data protection, anonymization, and governance are not established. Further, dataset biases could propagate unfair outputs. For instance, AI recognition has displayed higher error rates for women and minorities, indicative of skewed learning. Rigorous checks are vital to ensure the technology performs equitably across demographics. Those building recognition models also need safeguards and quality assurance steps against unlawful or rights-infringing uses of their work.
Privacy Concerns in Surveillance Technologies
Widespread video analytics and automated surveillance pose dangers of exacerbating harms to civil liberties. Capabilities for tracking individuals or groups, aggregating data on movements and activities, and flagging anomalies based on appearance or behavior patterns could strengthen state or commercial surveillance powers over citizens. Those implementing vision AI in surveillance must consider necessary controls like restricted retention periods for captured footage, consent requirements for enrollment, human oversight on automated decision triggers that impact people, and allowing lawful challenge to system outputs affecting individuals. Extensive documentation and transparency on intended use cases, data flows, and protection protocols also form crucial public trust elements.
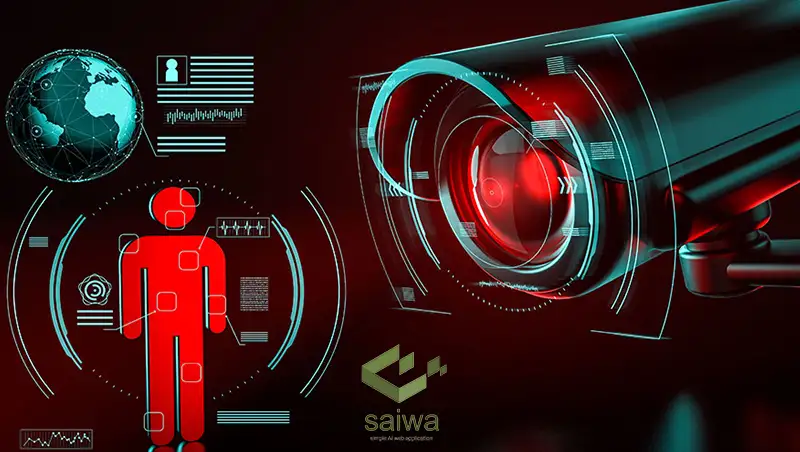
Transparency Needs
The transparency of computer vision ethics systems is crucial for ensuring ethical outcomes, yet many algorithms remain black boxes. Understanding how these systems work, auditing their design processes, and communicating transparently about limitations is vital.
Explainability of model features and decision criteria
With growing adoption in critical functions, computer vision systems need to incorporate explainability - illumination of the key model features and examples that inform classifications or predictions. This interpretability allows evaluation of fairness, reveals potential bias indicators that developers can remedy, assists human operators in decision loops to validate automated outputs, and would also helps address contested decisions or legality challenges through specifics on factors driving certain system behaviors.
Enabling scrutiny through audits and external quality checks
Independent algorithm audits and third-party testing mechanisms provide oversight to analyze model architectures, evaluate fairness metrics across gender, racial, and other cohorts, measure accuracy tradeoffs between groups, and gauge real-world performance across operational settings. Such rigorous inspection by those not involved in development flows allows models to meet quality thresholds before live deployment and catch issues missed during regular training evaluations.
Clarity around capabilities and limitations to users
Those adopting vision AI in products, services, or automated decisions must clarify intended use cases, explain model capabilities and limitations in ways accessible to end-users and stakeholders impacted, highlight contexts of potential inaccuracy or failure, and provide straightforward resolution channels. Setting proper expectations of functioning upfront and maintaining visibility builds understanding and trust in the technology.
Accountability Factors
Various stakeholders in computer vision models must be held accountable to ensure ethical practices, including developers, deployers, and policymakers. Responsibility starts with those building the algorithms, spans to those implementing the systems, and extends to policy bodies regulating the technology.
Monitoring model performance post-deployment
Responsible deployment demands proactive checks on computer vision ethics effectiveness through the operational lifetime via observation of system outputs after integration into products and environments. Continued data gathering on prediction quality, user feedback surveys, quantified user trust factors, and updated evaluations on diverse datasets allow those fielding AI to detect areas of declining performance from model drift or novel inputs that require developer intervention.
Channels for issue reporting and resolution
Timely redress of problems encountered assists user trust. Developers should enable direct pipelines for user reporting of computer vision failures, confusing behaviors, or perceived malfunctions. Some techniques like runtime confidence scores indicating uncertainty levels on classifications can also help human operators identify suspect outputs for further review. Clear protocols need to be created for triaging issues, diagnosing model weaknesses, patching deficiencies, and pushing updated versions.
Understanding liability by involved stakeholders
The nascent and fast-moving state of computer vision opens questions around legal or financial liability when failures occur in deployment - e.g. erroneous detection of threats, facial recognition false positives infringing on civil rights, and attribution of accidents involving autonomous vehicles relying on vision AI. Those building, integrating, and managing vision systems need clarity on which party bears ultimate accountability in case of regulatory non-compliance or disputes arising from improper functioning. As standards solidify, contracts denoting ownership of issues can balance responsibility across users, creators, and operational overseers.
Regularity Landscape
The regulatory landscape around computer vision ethics remains complex and fragmented across global jurisdictions. While some countries are starting to develop AI regulations, specifics pertaining to computer vision are still emerging.
Geographical legislation on the use of facial recognition
Many jurisdictions now recognize risks around consent, privacy, mass surveillance, and profiling from the unfettered use of facial analysis technologies. Rules constrain certain applications - the state of Washington limits user access to preserve fairness and freedom while the European Union prohibits most uses in public spaces. China represents an outlier with widespread video analytics deployment nationally, although even this approach has faced criticism from ethical perspectives. Globally, the extent of legislative coverage remains uneven across geographies. However early efforts signal priority for targeted governance given societal impacts.
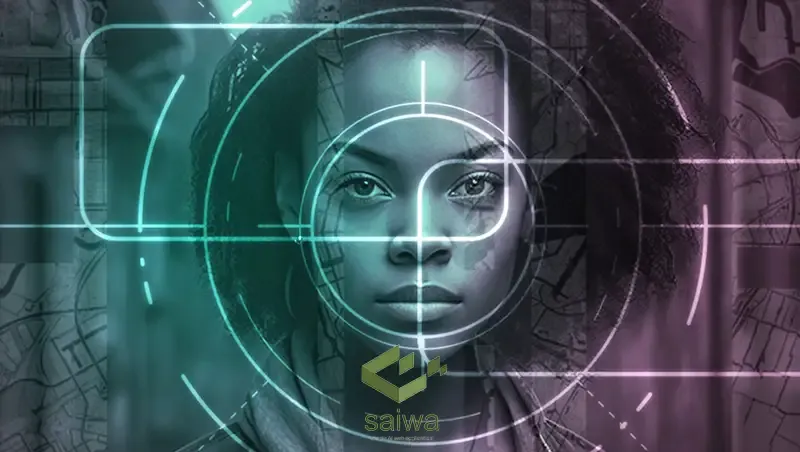
Law enforcement usage restrictions and rights
Police employment for investigation generates concerns of overreach or compromise of rights. Some measures introduced include requirements for court approvals before deploying facial search, guarantees on due process, warrants specifying scope, duration, and data use provisions, mandatory accuracy disclosure when used as evidence, and allowing legal objection to machine recognition outputs affecting individuals. Constraints aim to balance public safety needs with considerations of fairness and avoiding disproportionate impact on vulnerable groups.
Certification requirements linked to computer vision verticals
Domain specific implementations in areas like healthcare call for custom controls given technology influence on critical outcomes. Global MOUs between health software providers to only market-approved, tested tools meeting privacy, efficacy, and safety standards indicate attempts at self-regulation in sensitive machine vision contexts. Industry-specific ethical charters can propagate norms until government policy plays fuller catchup. Embedding such sector-specific principles guides those innovating tools for that profession and steers creation away from unethical paths early rather than relying solely on downstream policy to curb harm.
Conclusion
Rapid advances in computer vision unlock promising computer vision applications but simultaneously pose ethical challenges around privacy, accountability, transparency, bias, and consent management given the automation of impactful decisions. Technical progress without corresponding progress on governance jeopardizes socially acceptable outcomes. Those involved need clearly defined duties - whether technologists building responsibly, commercial providers guaranteeing rights protections, or governments shaping policy balancing innovation with the public interest.
Movement on multiple fronts - via voluntary standards, consumer pressures, industry norms, and legislative oversight - can help guide the highest utilities of computer vision ethics while curtailing risks of unchecked adoption. With so much at stake, proactive parallel priority towards ethics forms critical insurance for problems that hard censoring interventions later may struggle to undo once technologies disseminate widely. The still emergent nature of computer vision today offers the opportunity to positively influence trajectories towards responsible innovation by co-evolving solutions to complex questions that have no universally accepted playbooks yet.