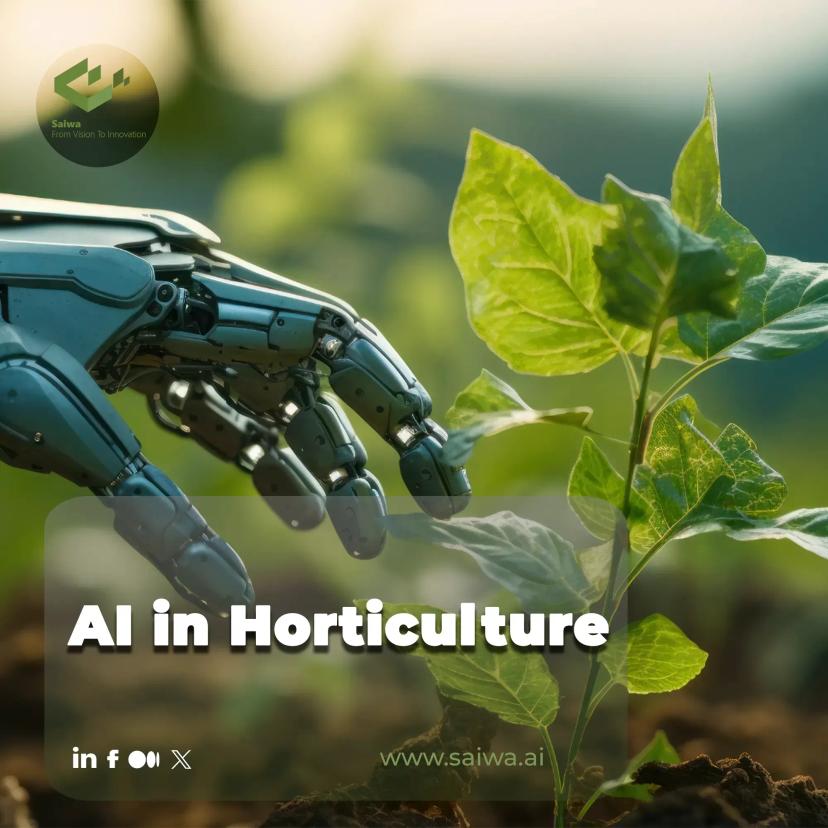
AI in Horticulture | Transforming Plant Cultivation and Sustainability
Horticulture, the cultivation of plants and landscapes, requires knowledge of plant biology, soil science, and environmental management. As the world's population grows and climate challenges intensify, there is an urgent need for more sustainable and efficient agricultural practices. Artificial Intelligence (AI) is emerging as a powerful tool to address these challenges.
By leveraging machine learning, robotics, and computer vision, AI can transform horticulture by increasing crop yields, improving sustainability, and optimizing resource use. AI-driven technologies can automate tasks, predict outcomes, and make data-driven decisions, reshaping the way horticulture is practiced. The integration of AI in horticulture offers breakthrough solutions to the industry's most pressing issues.
Sairone, an AI and machine learning platform developed by Saiwa, plays an important role in advancing AI applications in horticulture, agriculture, greenhouses, and ecological fields. Sairone can be integrated with various drones to optimize horticultural operations.
The platform's capabilities, such as invasive species detection, plant counting, nitrogen content estimation, and crop detection, directly contribute to the precision agriculture and sustainability goals of AI in horticulture. By providing actionable insights based on real-time data, Sairone helps improve crop management, resource utilization, and overall operational efficiency, in line with the transformative potential of AI in the sector.
This article examines how artificial intelligence is revolutionizing horticulture, from automating tasks and increasing efficiency to improving sustainability and crop quality. By exploring its diverse applications, this discussion highlights the transformative potential of AI in horticulture for the future of horticultural practices.
The Importance of AI in Horticulture
The global population is projected to reach nearly 10 billion by 2050, placing increasing demands on food production systems. Horticulture plays a crucial role in meeting this demand by providing a diverse range of fruits, vegetables, and ornamental plants. However, traditional horticultural practices often face limitations in terms of efficiency, resource utilization, and scalability.
AI offers the potential to overcome these limitations by enabling data-driven decision-making, automating labor-intensive tasks, and optimizing resource allocation. By enhancing productivity and sustainability, AI can contribute significantly to ensuring food security and meeting the growing global demand for horticultural products.
Key AI Technologies in Horticulture
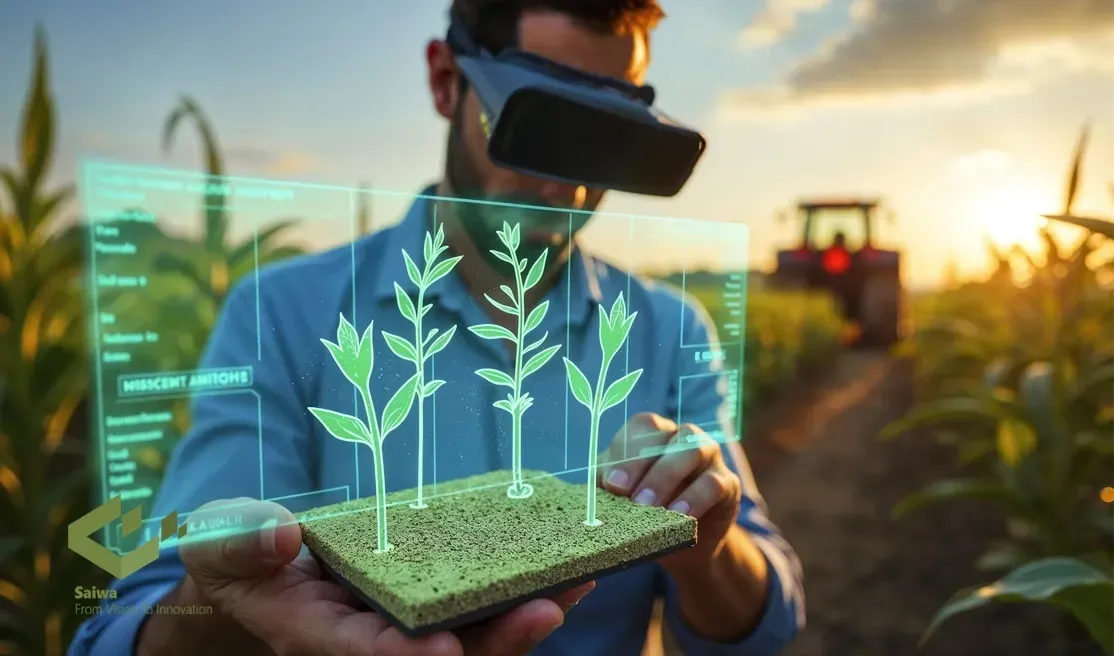
Several key AI technologies are driving the transformation of horticultural practices:
Machine Learning (ML)
Machine learning algorithms, a subset of AI, enable computers to learn from data without explicit programming. In horticulture, ML can be used to analyze vast datasets of environmental parameters, plant characteristics, and historical yields to develop predictive models for crop management, disease detection, and yield forecasting.
Different ML techniques, such as supervised learning, unsupervised learning, and reinforcement learning, are employed depending on the specific application and the nature of the available data. For example, supervised learning can be used to train models to classify plant diseases based on images, while unsupervised learning can be used to identify patterns in environmental data to optimize irrigation schedules.
Reinforcement learning can be utilized to train robots for autonomous navigation and harvesting tasks. The choice of ML technique depends on the specific problem being addressed and the characteristics of the available data. The increasing availability of large datasets and powerful computing resources is driving the rapid adoption of ML in horticulture.
Computer Vision and Image Recognition
Computer vision, another crucial AI technology, enables computers to "see" and interpret images, mimicking human vision. In horticulture, computer vision is used to analyze images of plants and their surroundings to identify diseases, pests, and nutrient deficiencies.
Image processing algorithms can be trained to recognize specific patterns and features associated with different plant conditions, enabling automated diagnosis and targeted interventions. These algorithms can analyze images from various sources, including drones, smartphones, and specialized cameras.
Computer vision can also be used to assess fruit ripeness, estimate crop yields, and monitor plant growth. Advances in deep learning, a subfield of ML, have significantly improved the accuracy and efficiency of computer vision algorithms for horticultural applications. The integration of computer vision with robotics enables automated tasks such as targeted spraying and selective harvesting.
Robotics and Automated Systems
Robotics and automation are transforming labor-intensive tasks in horticulture, such as planting, harvesting, and spraying. AI-powered robots can navigate autonomously through fields and greenhouses, performing tasks with precision and efficiency.
Automated systems can also monitor environmental conditions and adjust irrigation and fertilization schedules based on real-time data. Robotic systems can be equipped with various sensors and actuators to perform a wide range of tasks, including planting seeds, pruning plants, and applying pesticides. Automated systems can also control environmental factors such as temperature, humidity, and light intensity in greenhouses.
The development of robust and reliable robotic systems is crucial for addressing labor shortages and improving the efficiency of horticultural operations. The integration of AI with robotics enables more sophisticated and adaptive automation, further enhancing the productivity and sustainability of horticultural practices.
Read Also: The Impact of AI in Automated Harvesting
Natural Language Processing (NLP)
Natural Language Processing (NLP) enables computers to understand and process human language. In horticulture, NLP can be used to analyze text data from various sources, such as research papers, farmer forums, and social media, to extract valuable insights about crop management practices, disease outbreaks, and market trends.
This information can be used to improve decision-making and enhance the effectiveness of horticultural practices. NLP can also be used to develop chatbots and virtual assistants that can provide farmers with real-time advice and support.
By analyzing text data from diverse sources, NLP can help identify emerging trends and best practices in horticulture. NLP can also be used to translate information between different languages, facilitating knowledge sharing and collaboration among researchers and practitioners worldwide. The increasing availability of digital text data and the development of sophisticated NLP algorithms are expanding the potential applications of NLP in horticulture.
Internet of Things (IoT) and Sensor Networks
The Internet of Things (IoT) refers to the network of interconnected devices that can collect and exchange data. In horticulture, IoT sensors can monitor various environmental parameters, such as temperature, humidity, soil moisture, and light intensity, providing real-time data for crop management and decision-making.
Sensor networks enable continuous monitoring of plant health and environmental conditions, facilitating timely interventions and optimizing resource utilization. IoT sensors can be deployed in fields, greenhouses, and even on individual plants to collect detailed data about their environment and physiological status.
This data can be transmitted wirelessly to a central hub for analysis and decision-making. IoT networks enable remote monitoring and control of horticultural operations, improving efficiency and reducing labor costs.
The integration of IoT with AI algorithms enables more sophisticated data analysis and automated decision-making, further enhancing the precision and effectiveness of horticultural practices. The decreasing cost and increasing availability of IoT sensors are driving the rapid adoption of IoT technology in horticulture.
AI Applications in Horticulture
AI is being applied across various aspects of horticulture, transforming traditional practices and enabling new capabilities:
Crop Monitoring and Management
AI-powered systems can monitor crop growth and development, providing real-time insights into plant health, nutrient status, and water requirements. This information enables growers to make informed decisions about irrigation, fertilization, and pest control, optimizing resource utilization and maximizing crop yields.
These systems utilize various data sources, including sensor data, weather information, and historical records, to provide comprehensive insights into crop conditions. AI algorithms can analyze this data to identify potential problems, such as nutrient deficiencies or water stress, and recommend appropriate interventions.
By continuously monitoring crop health and environmental conditions, AI-powered systems enable proactive and targeted management practices, leading to improved crop yields and reduced resource consumption. Furthermore, AI can optimize the timing and application of fertilizers and pesticides, minimizing environmental impact and maximizing the effectiveness of these inputs. These advancements contribute to a more sustainable and efficient approach to crop production.
Disease and Pest Detection
AI algorithms can analyze images of plants to detect early signs of diseases and pests, enabling timely interventions and preventing widespread outbreaks. Early detection is crucial for effective disease and pest management, minimizing crop losses and reducing the need for chemical treatments.
These algorithms can be trained to recognize specific visual patterns and anomalies associated with different diseases and pests, often before they are visible to the naked eye. This early detection allows for targeted application of treatments, minimizing the use of broad-spectrum pesticides and reducing the risk of resistance development.
AI-powered disease and pest detection systems can be integrated with drones or robotic platforms for automated scouting and monitoring of large fields, further enhancing efficiency and reducing labor costs. Moreover, AI can analyze environmental data, such as temperature and humidity, to predict the likelihood of disease outbreaks, enabling proactive preventative measures.
Precision Agriculture
AI enables precision agriculture by providing site-specific recommendations for irrigation, fertilization, and other crop management practices. By tailoring inputs to the specific needs of each plant or zone within a field, precision agriculture optimizes resource utilization and minimizes environmental impact.
AI algorithms analyze data from various sources, including soil sensors, GPS systems, and aerial imagery, to create detailed maps of field variability. This information allows growers to apply inputs, such as water and fertilizer, only where and when they are needed, reducing waste and minimizing environmental impact.
Precision agriculture techniques enabled by AI can also optimize planting density, row spacing, and other agronomic practices to maximize yield potential. Furthermore, AI can be used to develop variable-rate application systems that automatically adjust the amount of inputs applied based on real-time data and site-specific conditions.
Yield Prediction and Crop Forecasting
AI algorithms can analyze historical yield data, weather patterns, and other relevant factors to predict future crop yields. Accurate yield predictions enable growers to make informed decisions about planting, harvesting, and marketing, optimizing their operations and minimizing risks.
These algorithms can identify complex relationships between various factors that influence crop yields, such as temperature, rainfall, soil conditions, and pest pressure. By incorporating real-time data and predictive models, AI can provide dynamic yield forecasts that are updated throughout the growing season.
Accurate yield predictions enable growers to optimize their harvesting and logistics operations, minimizing post-harvest losses and maximizing profitability. Furthermore, yield forecasts can inform marketing decisions, allowing growers to negotiate better prices and secure contracts based on anticipated production. This data-driven approach to crop management helps mitigate risks associated with market fluctuations and unpredictable weather events.
Robotic Harvesting and Automation
AI-powered robots are being developed to automate harvesting tasks, particularly for labor-intensive crops such as fruits and vegetables. Robotic harvesting can address labor shortages, reduce costs, and improve the efficiency of harvesting operations. These robots utilize computer vision and machine learning to identify ripe produce, navigate through fields, and delicately pick and handle fruits and vegetables without causing damage.
Robotic harvesting systems can operate continuously, increasing harvest efficiency and reducing the time required to bring produce to market. Furthermore, robotic harvesting can reduce reliance on manual labor, addressing labor shortages and reducing labor costs.
The development of robust and adaptable robotic harvesting systems is crucial for improving the productivity and sustainability of horticultural operations, particularly for high-value crops with demanding harvesting requirements.
Automation Systems in Irrigation
AI can optimize irrigation systems by adjusting watering schedules based on real-time data about soil moisture, weather conditions, and plant water requirements. Automated irrigation systems conserve water, reduce labor costs, and improve crop yields by ensuring that plants receive the optimal amount of water at the right time.
AI algorithms can integrate data from various sources, including soil moisture sensors, weather forecasts, and plant growth models, to determine the precise water needs of crops. Automated irrigation systems can then adjust watering schedules dynamically, delivering water only when and where it is needed.
This precise control of irrigation reduces water waste, minimizes energy consumption, and optimizes crop growth. Furthermore, AI-powered irrigation systems can detect leaks and malfunctions, preventing water loss and ensuring the efficient operation of irrigation infrastructure. By automating irrigation management, AI contributes to more sustainable and resource-efficient horticultural practices.
Grading of Fruits
AI-powered systems can automate the grading of fruits based on size, shape, color, and other quality parameters. Automated grading improves efficiency, reduces labor costs, and ensures consistent quality standards for horticultural products.
These systems utilize computer vision and machine learning algorithms to analyze images of fruits and classify them based on predefined quality standards. Automated grading systems can operate at high speeds, significantly increasing throughput compared to manual grading methods.
By ensuring consistent quality standards, automated grading enhances the marketability of horticultural products and reduces post-harvest losses. Furthermore, AI-powered grading systems can collect data about fruit quality, providing valuable insights into crop performance and enabling data-driven decisions about orchard management.
This automated approach to grading improves efficiency, reduces labor costs, and enhances the overall quality and value of horticultural products.
AI Drone-Based Technology in Horticulture
Drones equipped with advanced cameras and sensors are rapidly transforming horticultural practices by providing a powerful platform for data acquisition and analysis. These aerial platforms can capture high-resolution images and collect various types of data about crops and their surroundings, enabling a wide range of applications for precision agriculture and crop management.
AI algorithms play a crucial role in analyzing the data collected by drones, extracting valuable insights that can inform decision-making and optimize horticultural operations.
Benefits of AI in Horticulture
The adoption of AI in horticulture offers numerous benefits:
Improved Efficiency and Reduced Costs
AI automates labor-intensive tasks, optimizes resource utilization, and reduces operational costs, enhancing the overall efficiency of horticultural operations. By automating tasks such as planting, harvesting, and spraying, AI reduces the need for manual labor, addressing labor shortages and lowering labor costs.
AI-powered systems can optimize irrigation and fertilization schedules, minimizing water and nutrient waste, leading to cost savings and reduced environmental impact. Furthermore, AI can analyze market trends and optimize pricing strategies, maximizing profitability for growers.
Enhanced Crop Quality and Yield
AI enables precise crop management, early disease detection, and optimized resource allocation, leading to improved crop quality and increased yields. AI-powered systems can monitor plant health and detect early signs of disease or stress, enabling timely interventions and preventing widespread damage.
By optimizing irrigation, fertilization, and other crop management practices, AI ensures that plants receive the optimal nutrients and water they need to thrive, resulting in higher yields and improved quality. Furthermore, AI can be used to develop new crop varieties with enhanced traits, such as disease resistance and improved nutritional content.
Sustainability and Resource Conservation
AI optimizes water and fertilizer usage, reduces pesticide application, and minimizes environmental impact, promoting sustainable horticultural practices. AI-powered irrigation systems can adjust watering schedules based on real-time data about soil moisture and plant water requirements, minimizing water waste and reducing energy consumption.
AI can also optimize fertilizer application, ensuring that plants receive the precise nutrients they need, minimizing nutrient runoff and reducing environmental pollution. By enabling early disease detection and targeted interventions, AI reduces the need for broad-spectrum pesticides, minimizing the impact on beneficial insects and promoting biodiversity.
Data-Driven Decision Making
AI provides growers with access to real-time data and analytics, enabling data-driven decision-making and enhancing the effectiveness of crop management strategies. AI-powered systems can collect and analyze data from various sources, including sensors, weather stations, and historical records, providing growers with a comprehensive view of their operations.
This data can be used to identify trends, predict potential problems, and make informed decisions about planting, irrigation, fertilization, and pest control. By leveraging the power of data and analytics, AI empowers growers to optimize their operations, improve crop yields, and enhance the sustainability of their practices.
Challenges in Implementing AI in Horticulture
Despite the numerous benefits, several challenges hinder the widespread adoption of AI in horticulture:
Data Acquisition and Quality
Training AI algorithms requires large, high-quality datasets, which can be challenging to acquire in horticulture due to the variability of environmental conditions and plant characteristics. Collecting data from diverse sources, such as sensors, drones, and manual observations, can be time-consuming and expensive.
Data quality can be affected by factors such as sensor calibration, data entry errors, and inconsistencies in data collection protocols. Ensuring data accuracy and reliability is crucial for training effective AI models and ensuring the reliability of AI-driven insights. Standardizing data collection methods and developing robust data validation procedures are essential for addressing these challenges.
Cost of Implementation
Implementing AI-powered systems can require significant upfront investments in hardware, software, and training, which can be a barrier for smaller growers. The cost of sensors, drones, and other data acquisition equipment can be substantial.
Developing and deploying AI algorithms requires specialized software and computing resources, which can also be expensive. Furthermore, training personnel to operate and maintain AI systems requires investment in training programs and ongoing support. Exploring cloud-based solutions and collaborative initiatives can help reduce costs and make AI technology more accessible to smaller growers.
Lack of Expertise
Developing and implementing AI solutions requires specialized expertise, which may be lacking in the horticultural sector. Expertise in areas such as machine learning, computer vision, and data analytics is essential for developing effective AI applications.
Finding and retaining skilled personnel with these expertise can be a challenge for horticultural businesses. Bridging this skills gap through training programs, partnerships with research institutions, and knowledge-sharing initiatives is crucial for fostering wider adoption of AI in horticulture.
Integration with Existing Systems
Integrating AI systems with existing farm management software and hardware can be complex and require significant technical expertise. Data formats and communication protocols may vary across different systems, creating compatibility issues. Integrating AI systems seamlessly into existing workflows requires careful planning and technical expertise. Developing standardized data exchange formats and APIs (Application Programming Interfaces) can facilitate integration and interoperability between different systems.
Data Security and Privacy
The increasing use of data in horticulture raises concerns about data security and privacy, which need to be addressed to ensure responsible data management. Protecting sensitive data from unauthorized access and cyber threats is crucial. Implementing robust data security measures, such as encryption and access control, is essential. Furthermore, ensuring compliance with data privacy regulations and establishing transparent data governance frameworks are necessary for building trust and fostering responsible use of data in horticulture.
Conclusion
AI is poised to revolutionize horticulture, offering innovative solutions to address critical challenges and unlock new opportunities for sustainable and efficient plant production. From crop monitoring and disease detection to robotic harvesting and precision agriculture, AI is transforming every aspect of horticulture. While challenges remain in its widespread adoption, the benefits of AI are undeniable, and its continued development and integration will be crucial for the future of horticulture, ensuring food security and meeting the growing global demand for horticultural products.
As AI technologies continue to advance and become more accessible, their adoption in horticulture will undoubtedly accelerate, leading to a more sustainable, efficient, and productive future for the industry. Furthermore, ongoing research and development efforts are focusing on addressing the challenges associated with data acquisition, computational limitations, and ethical considerations, paving the way for even greater integration of AI in horticulture.