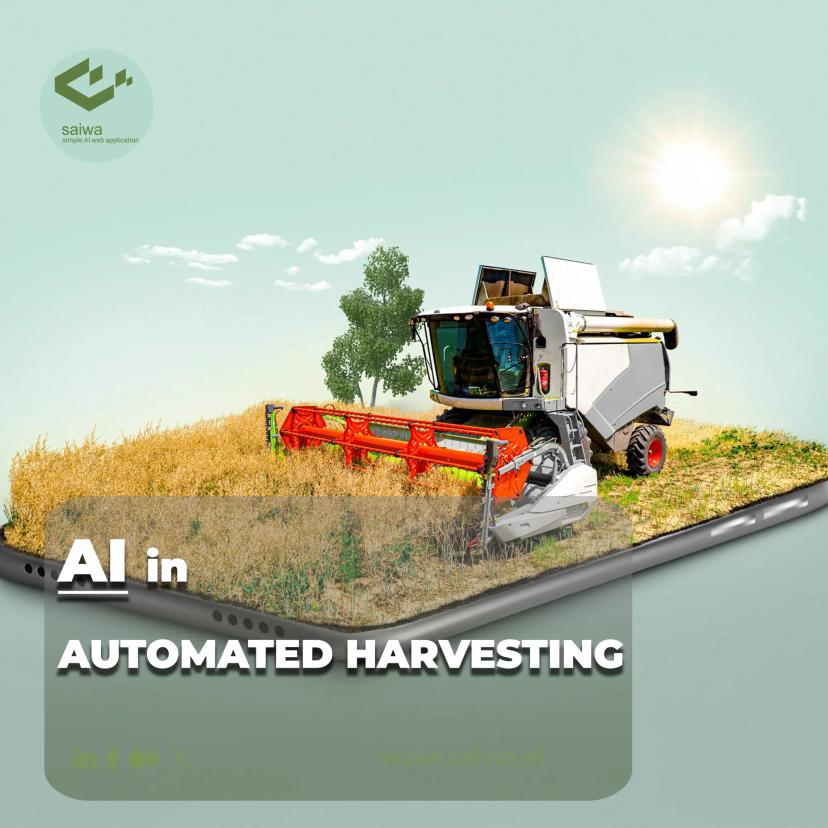
The Impact of AI in Automated Harvesting
Harvesting represents one of the most labor-intensive phases across agricultural operations. As labor shortages compound and farm sizes scale upwards globally, harvest automation processes offer invaluable efficiency improvements while addressing dwindling skilled worker availability. AI in automated harvesting provides capabilities surrounding computer vision, robotic motion control, and fleet orchestration that aim to replicate and even enhance the efficacies of human fruit pickers through sensors and algorithms.
When implemented astutely, AI-enabled autonomous crop harvesting solutions can deliver game-changing advancements over status quo manual methods including significantly boosting productivity per acre, ensuring the freshest and highest quality produce gets picked at ideal ripeness, while drastically cutting labor costs over manual harvest crews. This exploration will examine key harvest automation subsystems powering next-generation smart robotic harvesting.
Read Also: Practical Application & Future of AI in Agriculture
Computer Vision Systems for Harvest Automation
Sophisticated machine vision cameras and sensors play integral roles within harvest automation suited for specialty crops like tree fruits, citrus, and vegetables. Onboard cameras framed down crop rows continually scan plants assessing indicators of peak maturity including size dimensions, skin coloration shifts, and firmness.
Hyperspectral imaging detects exact spectra wavelength patterns across crops like apples or grapes to classify the level of sweetness and nutrient richness quantifying whether each fruit has achieved premium flavor suited for picking. Machine learning algorithms trained on sample crop image datasets programmatically tune sensitivity and specificity on these maturity triggers calibrated to specific apple strains for example being harvested to minimize premature picking while loss avoidance.
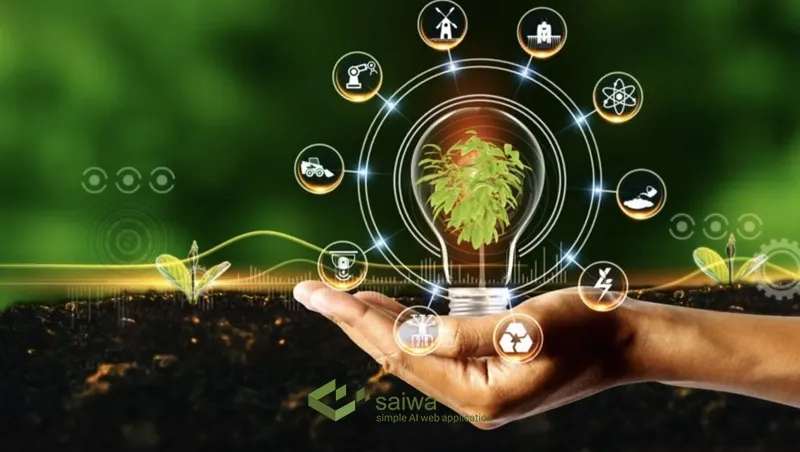
Read Also: An Over View Applications of Computer Vision in Agriculture
Obstruction Avoidance and Navigation
The same vision sensing also provides vital obstruction avoidance functionality allowing autonomous platforms to navigate fields safely without human assistance. 3D terrain maps localize bodies of water, and irrigation infrastructure above ground, and yield supporting structures to plot efficient routes avoiding collisions through narrow planting gaps.
With multiple robotic harvest automation operating as a fleet simultaneously, vision systems facilitate vehicle-to-vehicle positional awareness preventing conflicts. Integrated lidar sensors give proximity alerts to any foreign objects detected posing collision threats to trigger proximity alarms.
Quality Inspection and Sorting in Harvest Automation
Post-picking, inline quality inspection takes place by comparing each newly plucked item against ideal specimens with produce sorted accordingly. Minor defect identification ensures only premium grade produce moves forward minimizing unnecessary supply loss due to largely cosmetic damage. Robust onboard compute resources make real-time quality and maturity decisions without reliance on cloud connectivity.
Robotic Manipulator Arms
Specialized lightweight robotic arms mounted on autonomous harvester prototypes perform the crucial picking duties once crops slated for harvest are identified by the platforms’ computer vision scanning arrays. Gripper appendages get custom-engineered for the fruits gathered based on size, shape, and delicate skin sensitivity.
Pressure sensors determine the minimum grasping forces required to hold harvested materials steady through movements without causing bruising or penetration. Feedback camera guidance aids inappropriately picking trajectory motions tailored to that crop varietal.
Advanced Gripper Materials and Adaptive Design
For fruits like strawberries, polyurethane conformal foam lining gripper contact points adapt to delicate forms avoiding damage driven by rigid metal components. Adjustable tension settings prevent dropped materials from excessive compression or piercing skin integrity. Gripping multiple items per cycle boosts per-plant yield harvesting efficacies over single fruit grasp limitations common with emerging harvest robots.
Efficiency Boost through Multiple-Item Grasping
For collected items not passing onboard quality inspections, grippers reroute identified produce accordingly into alternate holding bins tagging reject materials for special handling compared to containers gathering premium output. Specialized anti-shock absorbing bin constructions prevent impact bruising over transport to sorting facilities. Some prototypes implement gentle shaking to allow reorientation of loaded items improving hauling density.
Supply Chain Transportation and Storage
Post-harvest, maintaining food safety and quality becomes crucial. AI-driven telematics sensors monitor product environments during transit, triggering alerts when adverse conditions threaten shelf life. Connected analytics detect patterns correlated with higher levels of waste, enabling optimized transport protocols. Routing systems consider weather data, choosing routes less prone to delays, and ensuring perishables reach their destinations promptly.
Warehousing periods are enhanced with continuous air quality readings, allowing proactive climate control adjustments to preserve harvested materials. Advanced scanning technology ensures storage containers meet cleanliness requirements, preventing contamination. The result is a seamless and secure transition from harvest to distribution.
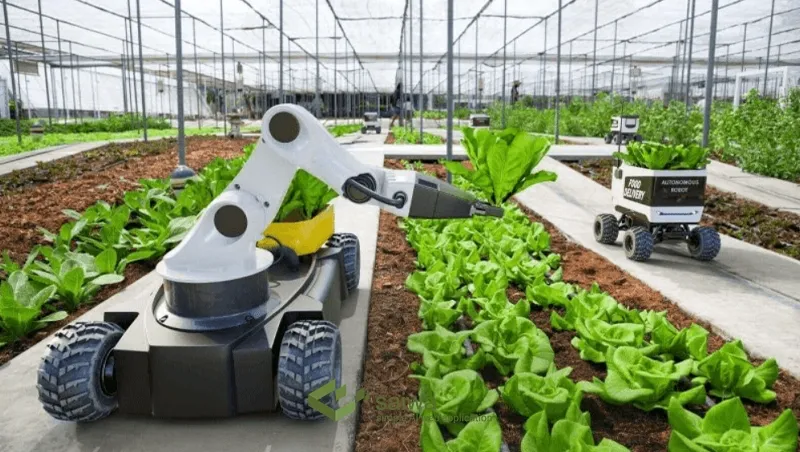
Read Also: A Deep Dive Into AI in Agricultural Supply Chain
Fleet Coordination
Managing groups of independent autonomous picking robots across expansive orchards and fields introduces added complexity requiring orchestration software to direct vehicles efficiently. Harvest automation mission planners ingest readings for ground terrain accessibility, obstruction hazards noted from central data lakes, and crop maturity indicators from associated computer vision to assign prioritized sequences guiding the next locations each platform gets dispatched to for optimal fruit retrieval.
Dynamic Harvest Planning with Real-Time Ripeness Sensing
Updated ripeness sensing helps fine-tune collection plans daily as maturation shifts dictate which crop rows take immediate precedence for picking runs versus moving down pre-defined grid patterns oblivious of actual field conditions.
Optimizing Fleet Performance and Scalability
Continuous status monitoring tracks percentage completion across all harvester assets with overtime alerts flagging underperformers signaling potential maintenance to avoid prolonged downtime and schedule gaps. As fleets scale over successive seasons, simulations modeling yield variability histories and predicting future output volumes determine minimum projected fleet sizes required to complete tight seasonal harvest windows in the critical window ensuring produce gets picked at ideal states before spoiling Still Have Unused Capacity.
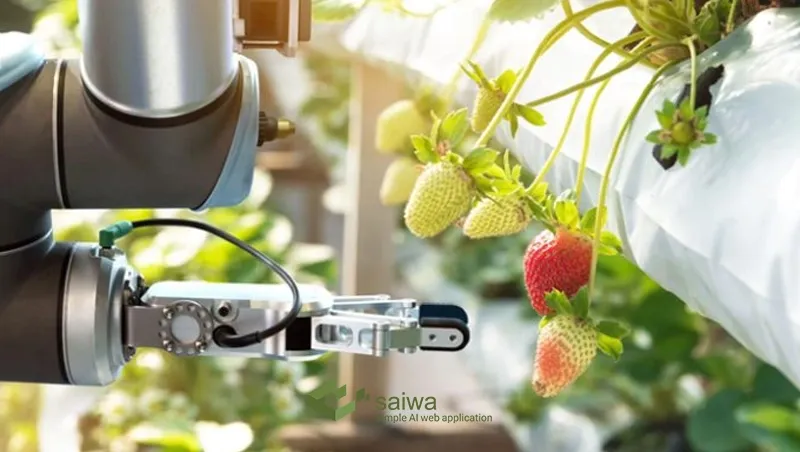
Challenges and Future Prospects
While AI in automated harvesting presents transformative possibilities, it is not without challenges. Technological limitations, scalability concerns, and the need for continued innovation are among the obstacles to widespread adoption. Addressing these challenges is crucial for realizing the full potential of AI in agriculture.
However, the future prospects are promising. Advancements in AI in automated harvesting technology, coupled with ongoing research and development, hold the key to overcoming existing limitations. The agricultural sector can anticipate increased productivity, sustainability, and economic benefits as AI applications in harvest automation evolve.
Conclusion
Incorporating AI in automated harvesting processes holds monumental potential to overcome severe industry-wide labor shortages while bolstering supply volumes to meet rising food scarcity concerns globally. Machine vision gives autonomous crop-picking robots heightened maturity assessment accuracy and safety unmatched by unassisted alternatives while manipulator arms replicate human grasp-and-pick actions with precision.
Coordinating entire smart harvest automation equipment crews ultimately helps farm enterprises maximize yields and freshness. By embracing cutting-edge mechatronics powered by artificial intelligence to elevate picking operations, the world's crops stand a stronger chance of making it safely from fields onto family dinner tables.