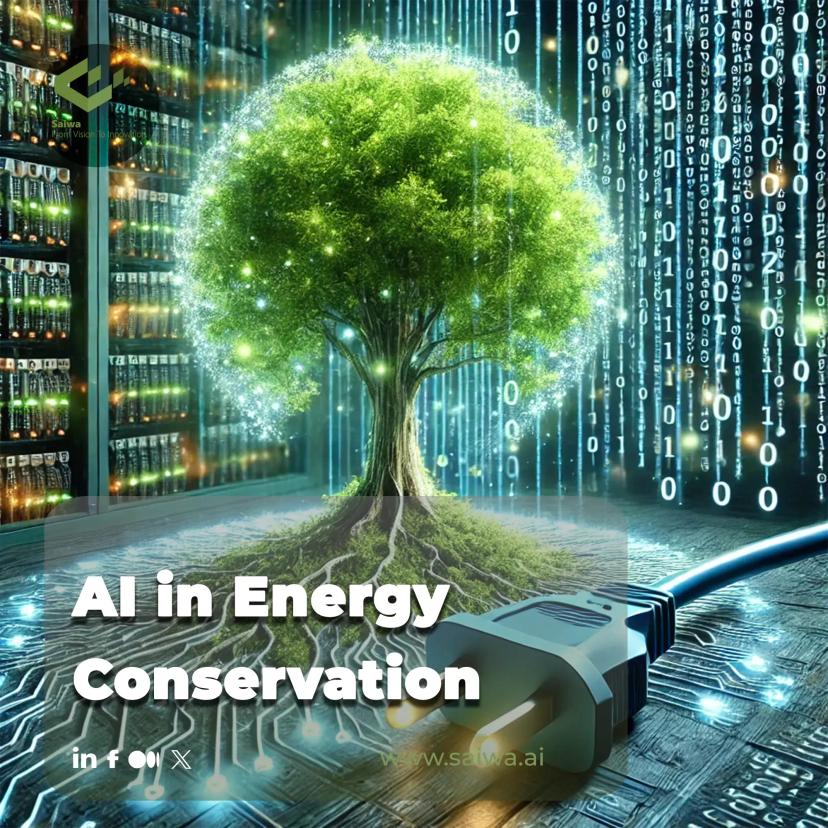
AI in Energy Conservation | Transforming the Future of Sustainable Innovation
The global energy landscape is undergoing a significant transformation, driven by the urgent need for sustainable practices and the increasing adoption of innovative technologies. Among these technologies, Artificial Intelligence (AI) has emerged as a powerful tool with the potential to revolutionize energy conservation and drive a more sustainable future. AI's ability to analyze vast amounts of data, identify patterns, and make predictions offers unprecedented opportunities to optimize energy consumption across various sectors, from individual buildings to entire power grids.
This article explores the multifaceted role of AI in energy conservation, examining its applications, benefits, challenges, and prospects in shaping a more sustainable energy future. We will delve into how AI is enhancing energy efficiency, optimizing renewable energy integration, and transforming the way we manage and consume energy across industries and smart cities.
Saiwa is an AI company that provides a service-oriented platform delivering advanced artificial intelligence (AI) and machine learning (ML) solutions. By leveraging innovative technologies, Saiwa empowers businesses to improve efficiency, address complex challenges, and achieve growth through customized AI and ML services. The company enables organizations to unlock the full potential of data and automation, and Saiwa can potentially play a crucial role in driving transformative results across industries, including energy conservation.
The article will also address the challenges and ethical considerations associated with AI adoption in the energy sector, including data privacy, security, and accessibility.
What is AI in Energy Conservation?
AI in energy conservation refers to the application of artificial intelligence techniques, including machine learning, deep learning, and computer vision, to optimize energy consumption and reduce waste. This involves using AI algorithms to analyze energy data, identify patterns of usage, and make predictions about future energy demand.
AI can also be used to control energy systems in real-time, adjusting settings and optimizing performance based on current conditions and predicted needs. This can lead to significant improvements in energy efficiency and a reduction in greenhouse gas emissions. AI-powered energy management systems can be applied across various sectors, including buildings, transportation, industry, and the power grid itself.
The Role of AI in Enhancing Energy Efficiency
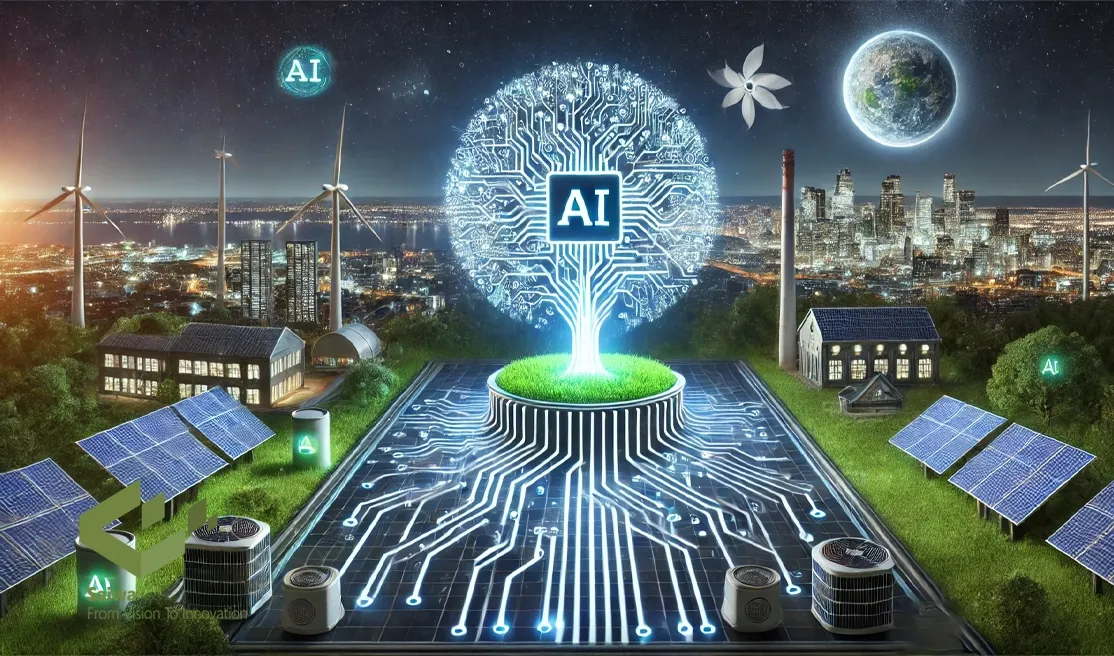
AI plays a crucial role in enhancing energy efficiency by providing intelligent control and optimization capabilities. Traditional energy management systems often rely on static rules and manual adjustments, which can be inefficient and ineffective in responding to dynamic changes in energy demand.
AI-powered systems, on the other hand, can learn from historical data and adapt to changing conditions in real-time. This allows for more precise control over energy consumption, minimizing waste and maximizing efficiency. AI can also identify hidden patterns and anomalies in energy usage, providing insights that can be used to further optimize energy consumption and identify potential areas for improvement.
AI in Energy Conservation for Buildings and Infrastructure
Buildings and infrastructure represent a significant portion of global energy consumption. AI can transform these spaces into intelligent, energy-efficient environments. Smart building management systems powered by AI can optimize heating, ventilation, and air conditioning (HVAC) systems, lighting, and other energy-consuming equipment based on occupancy patterns, weather conditions, and real-time energy prices.
AI can also predict equipment failures and optimize maintenance schedules, further reducing energy waste and extending the lifespan of building systems. By integrating AI into building design and operation, we can significantly reduce the energy footprint of buildings and infrastructure, contributing to a more sustainable built environment.
AI in Renewable Energy Integration
Integrating renewable energy sources, such as solar and wind power, into the existing power grid presents significant challenges due to the intermittent and unpredictable nature of these resources. AI in Renewable Energy can play a crucial role in addressing these challenges by accurately forecasting renewable energy generation, optimizing energy storage, and balancing supply and demand in real-time.
AI-powered grid management systems can predict fluctuations in renewable energy generation and adjust the dispatch of other power sources accordingly, ensuring grid stability and reliability. AI can also optimize the charging and discharging of energy storage systems, maximizing the utilization of renewable energy and minimizing reliance on fossil fuels.
AI for Solar and Wind Energy Optimization
AI can optimize the performance of solar and wind energy systems by predicting solar irradiance and wind speeds, optimizing the positioning and orientation of solar panels and wind turbines, and predicting equipment failures.
By analyzing weather data and historical performance data, AI algorithms can accurately forecast solar and wind power generation, allowing for better integration into the grid and more efficient energy management. AI can also optimize the design and operation of solar and wind farms, maximizing energy capture and minimizing downtime.
The Impact of AI in Energy Conservation: Case Studies and Results
Numerous case studies demonstrate the significant impact of AI in energy conservation across various sectors. For example, Google has implemented AI-powered cooling systems in its data centers, reducing energy consumption by up to 40%. Similarly, AI-powered building management systems have been shown to reduce energy consumption in commercial buildings by 10-20%.
These real-world examples highlight the tangible benefits of AI in achieving significant energy savings and reducing greenhouse gas emissions.
AI in Energy Optimization Across Industries
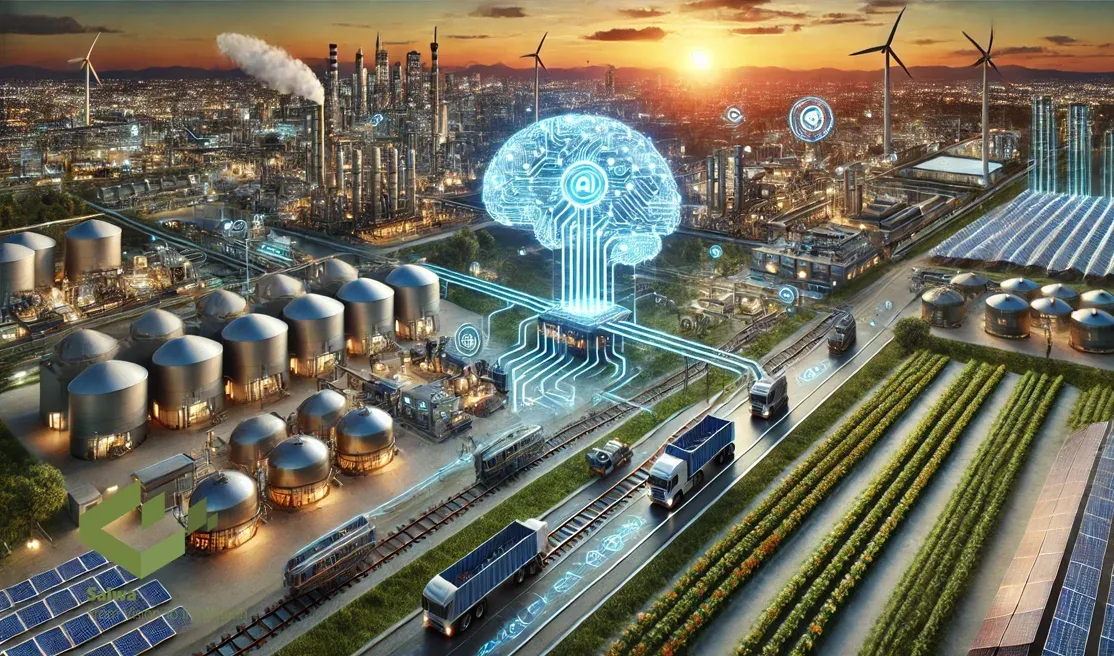
Beyond buildings and infrastructure, AI is transforming energy optimization across various industries, including manufacturing, transportation, and agriculture. In manufacturing, AI can optimize production processes by analyzing real-time data from machinery and sensors to identify inefficiencies and adjust operations dynamically, reducing energy consumption in industrial equipment and minimizing waste.
AI in Predictive Maintenance allows for proactive interventions that prevent costly downtime and extend the lifespan of equipment, further contributing to energy savings.
In transportation, AI can optimize routing and logistics by analyzing traffic patterns and predicting congestion, improving fuel efficiency, and reducing emissions. Furthermore, AI is enabling the development of autonomous vehicles, which have the potential to further optimize traffic flow and reduce energy consumption in the transportation sector.
In agriculture, AI can optimize irrigation systems by analyzing soil moisture levels and weather data, delivering water precisely when and where it is needed, reducing water and energy consumption in farming operations. AI in agriculture can also be used to monitor crop health and predict yields, enabling more efficient use of resources and improving overall agricultural productivity.
Smart Cities and AI-Driven Sustainability
AI is playing a key role in the development of smart cities, enabling more efficient and sustainable urban environments. AI-powered systems can optimize traffic flow by analyzing real-time traffic data and adjusting traffic light timings dynamically, reducing congestion and minimizing fuel consumption.
AI can also manage waste disposal by optimizing collection routes and predicting waste generation patterns, improving efficiency and reducing the environmental impact of waste management. Furthermore, AI can monitor air quality by analyzing data from sensors deployed throughout the city, providing real-time insights into pollution levels and enabling proactive measures to improve air quality and public health.
By integrating AI into various aspects of city operations, from transportation and waste management to environmental monitoring and public safety, we can create smarter, more efficient, and more sustainable urban centers that enhance the quality of life for all citizens.
Challenges and Considerations in AI for Energy Conservation
Despite the numerous benefits, there are also challenges and considerations associated with implementing AI for energy conservation.
Energy Consumption of AI Models
Training and running complex AI models, especially deep learning models with millions or even billions of parameters, can require significant computational resources and energy. The computational demands translate directly into substantial energy consumption, particularly in large-scale deployments.
This raises concerns about the overall environmental impact of using AI for energy conservation, as the energy used to train and run the AI models could potentially offset some of the energy savings achieved. Therefore, it is important to consider the energy footprint of AI itself and develop more energy-efficient algorithms and hardware, including specialized AI chips and more efficient training methods.
Research into more sustainable AI practices, including algorithmic efficiency and optimized hardware utilization, is crucial for minimizing the environmental impact of AI-driven energy conservation efforts.
Data Privacy and Security Concerns
AI systems rely on access to large amounts of data, including granular energy consumption data from smart meters, building management systems, and other sources. This raises concerns about data privacy and security.
The data collected may contain sensitive information about individuals' energy usage patterns, which could be exploited for malicious purposes if not adequately protected. Protecting sensitive energy data from unauthorized access and misuse is crucial. This requires implementing robust security measures, including data encryption, access control, and intrusion detection systems.
Furthermore, adhering to data privacy regulations and best practices is essential for maintaining public trust and ensuring responsible use of energy data. Developing privacy-preserving AI techniques, such as federated learning, can further enhance data protection while still enabling effective AI-driven energy management.
Financial and Accessibility Barriers
Implementing AI-powered energy management systems can require significant upfront investment in hardware, software, and expertise. This can be a barrier for some organizations, particularly smaller businesses and non-profit organizations, as well as for individuals looking to implement smart home energy management systems.
The cost of AI technology, including the development of customized AI models and the integration with existing systems, can be prohibitive for those with limited resources. Making AI technology more accessible and affordable is essential for widespread adoption. This could involve developing open-source AI tools and platforms, providing financial incentives for AI adoption, and promoting knowledge sharing and collaboration within the energy sector.
Reducing the cost and complexity of implementing AI solutions will democratize access to this transformative technology and accelerate its adoption for energy conservation.
The Future of AI in Energy Conservation
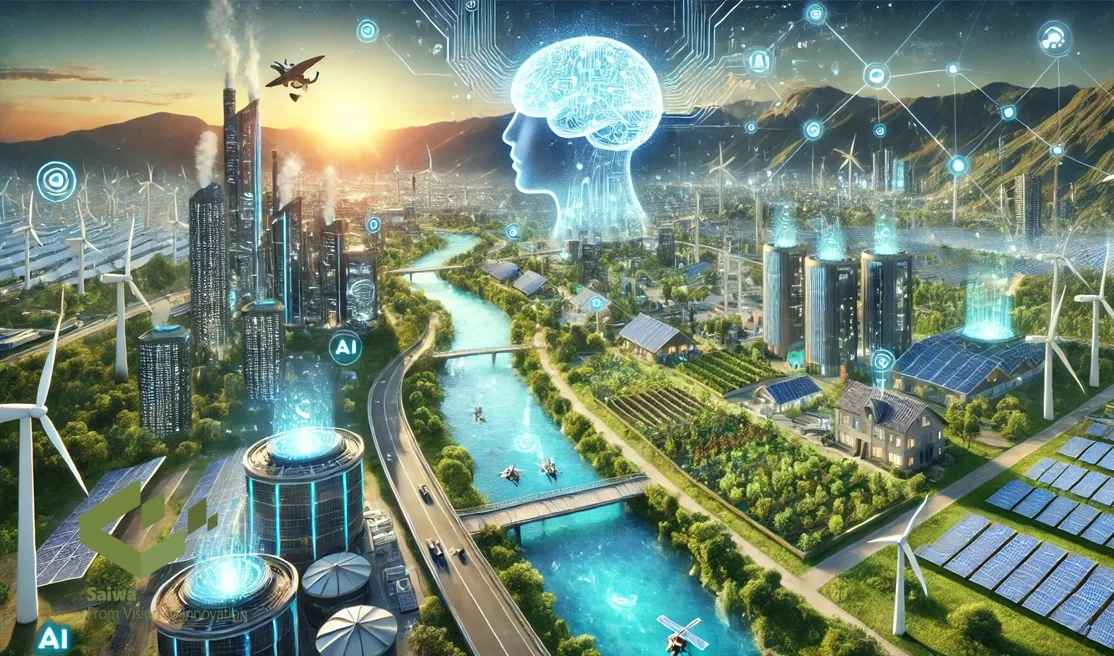
The future of AI in energy conservation is exceptionally promising, driven by rapid advancements in AI algorithms and the growing availability of data from smart grids and IoT devices. We can anticipate increasingly sophisticated AI models, leveraging techniques like reinforcement learning and evolutionary computation, to optimize energy consumption across all sectors.
This includes more accurate predictions of energy demand, smarter energy storage and distribution, and personalized energy management solutions tailored to individual needs. The convergence of AI with technologies like blockchain and edge computing will further enhance security and decentralization in energy systems.
Widespread adoption of AI-powered solutions will lead to significant reductions in energy consumption and greenhouse gas emissions, while also facilitating the seamless integration of renewable energy sources. Explainable AI (XAI) will be crucial for fostering trust and transparency, and a greater emphasis on human-AI collaboration will empower informed decision-making in the quest for a sustainable energy future.
Final Thought
The journey towards a sustainable energy future is a complex and multifaceted undertaking, and AI stands as a powerful ally in this critical endeavor. While the technology itself holds immense promise, its true potential lies in our collective ability to harness it responsibly and equitably. By acknowledging the challenges, embracing innovation, and prioritizing collaboration, we can ensure that AI's transformative power drives meaningful and lasting change in the way we produce, manage, and consume energy.
The future of energy conservation is not just about technological advancement; it's about building a more sustainable and equitable world, powered by intelligent and responsible innovation.