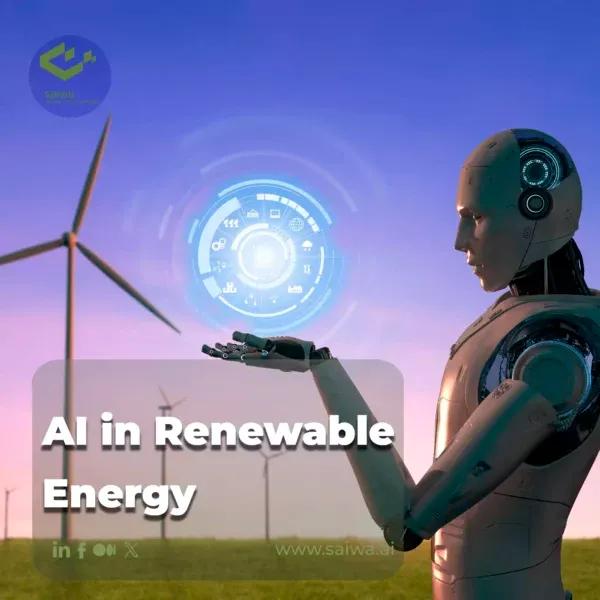
Powering Sustainability | AI in Renewable Energy Solutions
Renewable energy sources such as solar and wind are key to building sustainable, low-carbon energy systems. However, the inherent challenges of intermittency, distributed assets, and real-time balancing of supply and demand hinder the scalability of renewable energy. Artificial intelligence (AI) techniques hold great promise for overcoming these barriers and unlocking the full potential of renewable energy. This article explores applications of AI in renewable energy sector and reviews the opportunities and challenges involved.
What is Artificial Intelligence?
Artificial intelligence (AI) refers to computer systems or software that can perform tasks normally requiring human intelligence, such as visual perception, speech recognition, and decision-making. AI encompasses a variety of technologies, including machine learning as a service, deep learning as a service, natural language processing (NLP), robotics, and more. The common thread uses advanced algorithms and models that enable computers to mimic human-level cognition in specialized domains.
Key capabilities of AI include learning from data, adapting to new situations, understanding and responding to language, making predictions, optimizing processes, and automating tasks. When integrated into renewable energy systems, AI can provide invaluable optimization and automation to overcome integration challenges and unlock the sector's immense potential.
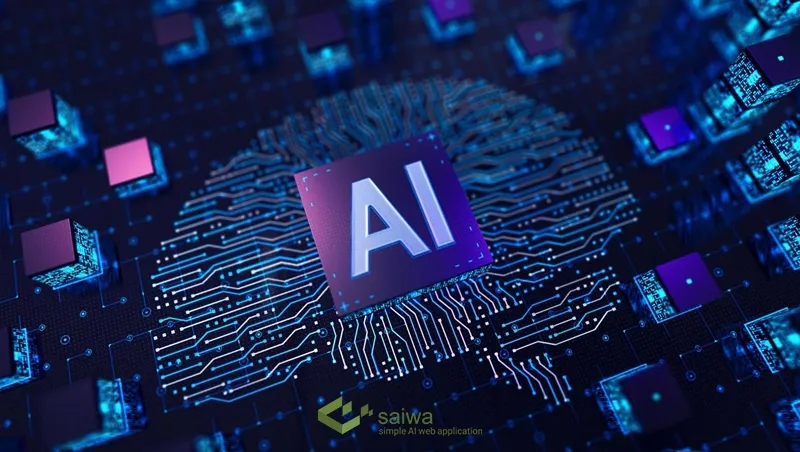
ML and AI in Renewable Energy Industry
Two branches of artificial intelligence taking a leading role in renewable energy are machine learning and deep learning. Both fall under the umbrella of AI but have some distinct capabilities contributing to the energy sector.
Machine learning refers to algorithms that can "learn" from data, improving at tasks like making predictions or identifying patterns without being explicitly programmed to do so. Machine learning analyzes training datasets to build models that can process new data to generate insights, make forecasts, or categorize information based on prior examples. For example, AI in renewable energy can extract insights from weather data to predict solar power production.
Deep learning is a sophisticated type of machine learning that uses multi-layer neural networks loosely inspired by the neuronal structure of the human brain. These neural networks can identify very complex relationships within massive, multidimensional datasets that may be overlooked by other techniques. This enables recognizing subtle anomalies or patterns for renewable applications like predictive maintenance.
Whereas machine learning relies on more structured data, deep learning can also process unstructured data like sensor time series, imagery, video footage, audio data, and text. Common machine-learning tasks include classification, regression, clustering, and anomaly detection. Deep learning excels at computer vision, complex forecasting, and natural language processing.
Together, supervised learning, unsupervised learning, reinforcement learning, and deep neural network techniques, which fall under the umbrella of machine learning, are driving extraordinary advances in AI in the renewable energy sector. Continued improvements in algorithms, computing power, and energy data availability promise to unlock further the potential of machine intelligence for renewable energy integration.
Challenges Facing Renewable Energy
While renewable energy offers benefits like lowered emissions, energy independence, and cost competitiveness, several challenges constrain adoption:
Intermittency of generation from weather-dependent sources like wind and solar poses difficulties in real-time grid balancing. Fluctuating supply must be continuously matched to variable demand to maintain grid stability.
Distributed and decentralized nature of assets like rooftop solar, small-scale wind, and batteries complicates coordination and optimization across numerous grid-edge points.
Limited storage capabilities make balancing instantaneous renewable supply and demand more pressing, given inability to buffer generated energy.
Forecasting uncertainties around renewable production based on weather, equipment performance, and other factors further hinder effective management.
Achieving equivalent reliability and resilience as conventional power poses engineering hurdles as renewables scale.
AI based energy solutions is poised to help overcome these obstacles in cost-effective and scalable ways.
How can the use of AI in the renewable energy sector?
AI can help tackle several major challenges faced by renewable energy:
Forecasting
Machine learning algorithms can analyze historical weather patterns, turbine sensor data, geospatial data, and other variables to generate much more accurate forecasts of expected renewable energy production across different time horizons. More precise forecasting enables grid operators and energy traders to better schedule dispatchable generation, charge storage assets, and balance electricity supply and demand to integrate higher shares of variable renewables.
Asset Optimization
AI systems can optimize the performance of distributed energy assets in real-time, maximizing renewable generation yields while minimizing equipment stresses and failure risks. This may involve modulating turbine blade pitch or solar inverter loading to respond to transient wind and solar conditions. AI control systems continuously tune operations for efficiency gains.
Predictive Maintenance
By applying anomaly detection algorithms to data streams from sensors on wind turbines, solar panels, batteries, and electrical infrastructure, AI can identify signs of deteriorating performance or impending equipment failures. This predictive capability allows maintenance to be proactively scheduled before problems cause failures and costly downtime.
Grid Management
As a greater portion of grid power comes from distributed renewables like rooftop solar and community wind turbines, AI coordination algorithms can optimize decentralized assets in real-time to balance local supply and demand while maintaining power quality and reliability. This grid balancing act across numerous small assets is extremely difficult without AI optimization.
Trading & Matching
Renewable forecasting enables generators to optimally bid into electricity markets while matching forecast supply with flexible user demands smoothes grid operations. Shifting flexible energy consumption like EV charging and appliance usage to align with renewable availability reduces curtailments.
Process Automation
AI techniques like robotic process automation use software bots to automate back-office processes involved in customer service, billing, asset registration, regulatory reporting, and other administrative tasks. This cuts costs and boosts productivity.
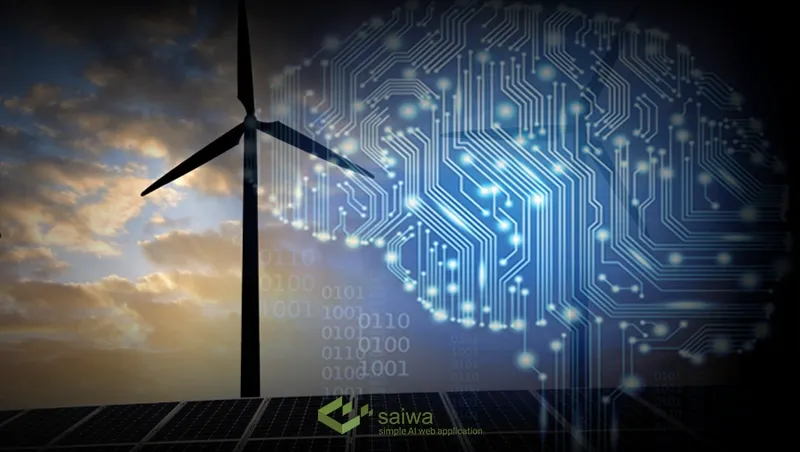
Impact of AI on Renewable Energy
Adopting AI can significantly advance renewable integration and adoption:
Increased renewable generation
By optimizing the performance and availability of assets like wind turbines and solar panels while improving predictive maintenance, AI solutions directly increase renewable energy generation volumes and capacity factors.
Reduced maintenance costs
The predictive capabilities of AI significantly reduce operation and maintenance costs by minimizing unplanned downtime, service needs, and field interventions through prognostics.
Greater grid stability
As the percentage of power from variable sources like wind and solar increases, AI helps maintain grid stability by coordinating and optimizing distributed assets in real-time to match supply with demand.
Lower energy costs
Efficiency gains from optimizing assets and trading renewable energy ultimately help lower consumer costs while increasing access to clean power.
Faster renewable adoption
By enabling solutions to challenges like intermittency and decentralized assets, AI helps accelerate the global transition and adoption of renewable energy.
Reduced emissions
Increased renewable penetration and displacement of fossil fuel generation driven by AI solutions directly contribute to decarbonization objectives.
New revenue opportunities
Leveraging data to provide value-added services like granular forecasting analytics and predictive maintenance creates new revenue streams.
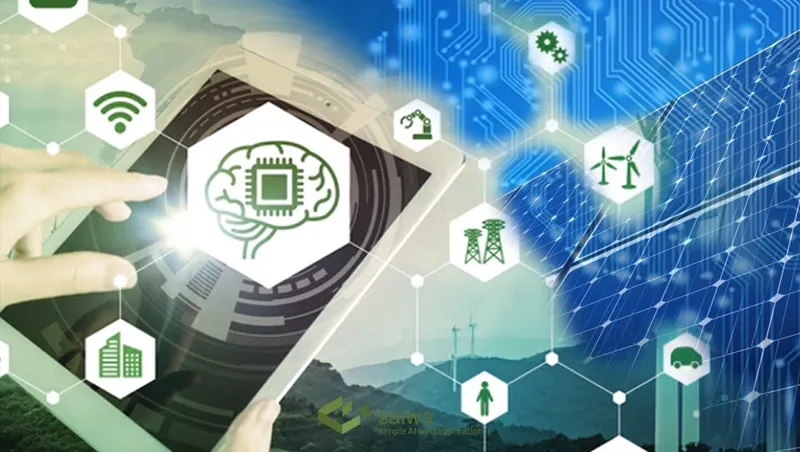
Challenges and Limitations of AI in Renewable Energy
While impactful. Harnessing AI faces hurdles:
Data availability
Large volumes of high-quality, cleaned, aggregated data over long periods are essential for training robust AI models. Such data is often scarce. Privacy also hinders data sharing.
Legacy infrastructure
Integrating modern AI software and hardware into aging, rigid infrastructure not designed for analytics presents compatibility challenges requiring complex customization.
Cybersecurity
Growing reliance on data connections, visibility software, and autonomous algorithms introduces new cybersecurity vulnerabilities and attack surfaces.
Cost barriers
Despite enormous potential value, tight budgets may deter investing upfront in costly talent, infrastructure, and months-long AI programs with long-term payoffs.
Explainability
The "black box" nature of complex machine learning models hampers trust, auditing, and oversight. The lack of model explainability poses risks.
Skill gaps
Most energy companies lack the unique mix of in-house data science, software development, and energy systems expertise required for AI success. Knowledge gaps slow progress.
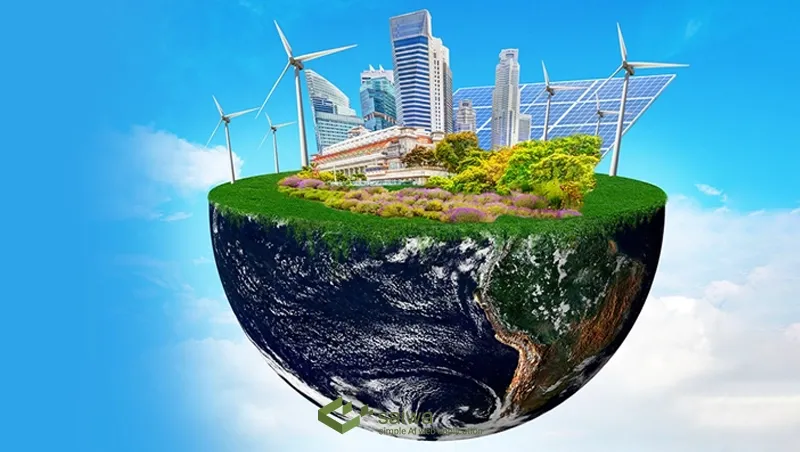
Real World Case Studies for AI based energy solutions
Real-world examples demonstrate AI in renewable energy contributions:
Vestas uses AI to create digital twins of wind turbines to simulate performance factors and optimize turbine design. The models help boost energy output.
Google employs deep neural networks for ultra-short term solar forecasting of Photovoltaic production at minute intervals to optimize electricity bidding and trading.
GE Renewable Energy improves wind turbine productivity by 10-20% using AI models that minimize downtime through predictive maintenance and anomaly detection.
WattTime developed an AI platform helping households automatically shift flexible electricity usage to times of abundant renewable supply, lowering emissions.
Conclusion
AI in Renewable Energy is a potentially transformative technology for overcoming barriers to large-scale renewable energy integration. Advanced AI algorithms offer capabilities such as improved forecasting, optimized infrastructure, predictive maintenance, automated processes, and real-time supply-demand matching, unlocking renewable energy's vast potential. However, effectively addressing challenges related to data, legacy systems, cybersecurity, costs, and expertise is crucial for organizations to strategically harness AI in Renewable Energy and maximize its contributions to the global transition toward a sustainable energy system.