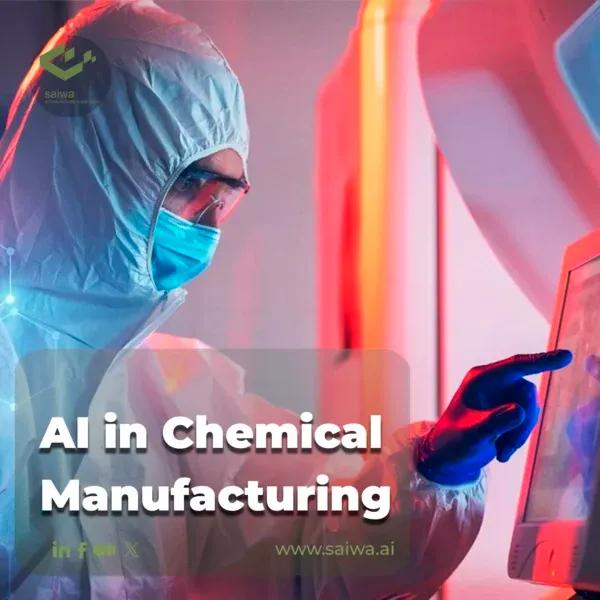
AI in Chemical Manufacturing | A Path to Quality and Safety
Artificial intelligence (AI) refers to advanced computer systems and algorithms that can perform tasks and make decisions comparable to human intelligence. AI includes techniques such as machine learning, deep learning, computer vision, natural language processing, robotics, and more. In recent years, the use of AI in the chemical manufacturing industry has grown significantly.
Chemical companies are using AI to optimize myriad aspects of their operations, from research to logistics. AI in chemical manufacturing enables improved process efficiency, quality control, predictive maintenance, and safety compliance, among other applications. It provides actionable insights from vast amounts of plant and enterprise data that exceed human analytical capabilities. As manufacturing becomes increasingly complex, AI is a critical tool for chemical companies to maintain competitive advantage.
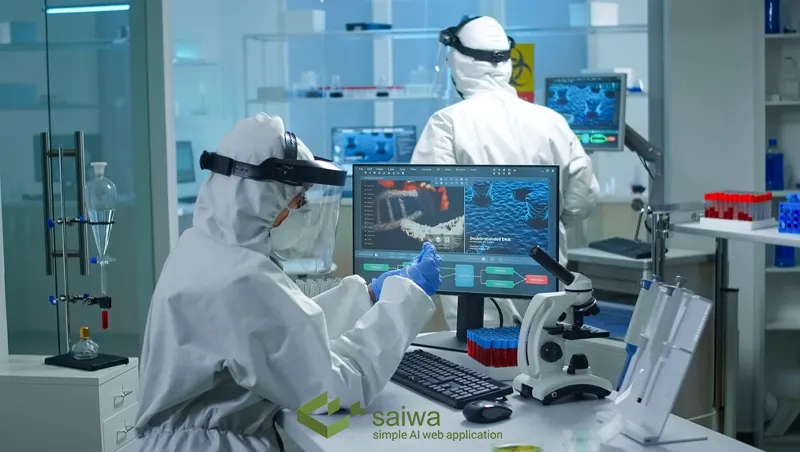
AI-Driven Safety and Compliance
AI is essential for hazard prevention and regulatory compliance in chemical plants. AI modeling identifies potential risks such as leaks, runaway reactions, and overpressure scenarios. Automated early warning systems provide time to prevent accidents. Process simulation supports safety testing. AI also tracks compliance with safety, health, environmental, and quality regulations. It monitors critical parameters and generates necessary reports. In addition, AI can optimize the design of inherently safer processes and suggest effective risk control measures.
AI and Sustainable Manufacturing
As stakeholders demand greener practices, AI is helping chemical manufacturers reduce their environmental impact. Energy management systems use AI to optimize energy consumption throughout production. This reduces costs and carbon emissions. AI also minimizes material waste by adjusting feedstock metering, recycling by-products, and scheduling maintenance. In R&D, AI facilitates the development of benign-by-design chemicals and sustainable processes through computational modeling. It also enables high-throughput screening to formulate greener products.
Read Also: Machine Learning in Manufacturing | Applications & Benefits
Enhanced R&D and Scientific Discovery
In chemical research, AI accelerates scientific discovery and product development through computational molecular design and automated laboratory experimentation. Physics-based simulations enable rapid in silico screening of millions of candidate molecules for desired properties without time-consuming synthesis. AI analysis of decades of historical publications provides knowledge discovery to guide new hypotheses. Autonomous lab robots controlled by AI algorithms also accelerate iterative testing of new materials and chemical formulations.
Drug Discovery and Materials Science
In pharmaceuticals and materials science, AI is accelerating research and development. By sifting through massive amounts of data, AI algorithms identify promising compounds and materials for further investigation. This accelerates the discovery process, leading to breakthroughs in drug development and materials science.
Read Also: The Growing Role of AI in Drug Discovery
Computational Chemistry
Computational chemistry, a field that involves complex simulations and molecular modeling, benefits significantly from AI. AI-driven models can analyze molecular interactions and predict chemical reactions with a high degree of accuracy. This accelerates research and innovation in the chemical field.
ROI Strategies for AI in Chemical Industry
AI in the chemical industry is a powerful tool, but its success hinges on a clear financial picture. Here's how to calculate the return on investment (ROI) for AI projects:
Quantify Gains
Focus on measurable improvements like increased yield, reduced downtime through AI-powered predictive maintenance, and lower energy consumption. Translate these into dollar values over a defined period to establish a baseline ROI for your AI in chemical industry project.
Value the Intangibles
AI's impact goes beyond immediate cost savings. Consider the improved product quality, faster innovation cycles, and enhanced safety measures enabled by AI in the chemical industry. While assigning a direct monetary value can be tricky, estimate the potential market advantage or cost avoidance associated with these benefits.
Factor in All Costs
Be transparent about the upfront costs of implementing AI in chemical industry. This includes hardware, software, data management, and acquiring AI expertise. Factor in ongoing maintenance and potential model retraining for a complete picture of your AI in the chemical industry investment.
Long-Term ROI Perspective
AI is a long-term investment. While initial benefits from AI in chemical industry may take time to materialize, the cumulative impact on efficiency, innovation, and brand reputation can lead to significant value creation over the years.
By employing these ROI calculation strategies, chemical companies can make informed decisions about AI investments. Considering both tangible and intangible benefits alongside costs ensures they unlock the true potential of AI in the chemical industry and gain a competitive edge.
Predictive Maintenance Averts Equipment Failures
Unplanned downtime inflicts heavy losses, making preventative maintenance critical. AI in chemical manufacturing apnest solutions leverage AI techniques like:
Deploying sensors across equipment to collect real-time performance data, including temperature, vibration, pressure, and other indicators of health.
Aggregating historical sensor data to analyze patterns and derive predictive failure models using machine learning algorithms.
Monitoring key indicators from predictive models in real-time to accurately anticipate failures before occurrence based on learned precursor patterns.
Triggering proactive maintenance like component replacements ahead of predicted failures to avoid unplanned downtime.
Continuously refining predictive models with new data, enhancing accuracy over time.
By closely monitoring equipment health, chemical manufacturers can pivot from reactive to predictive maintenance through AI, averting costly downtime.
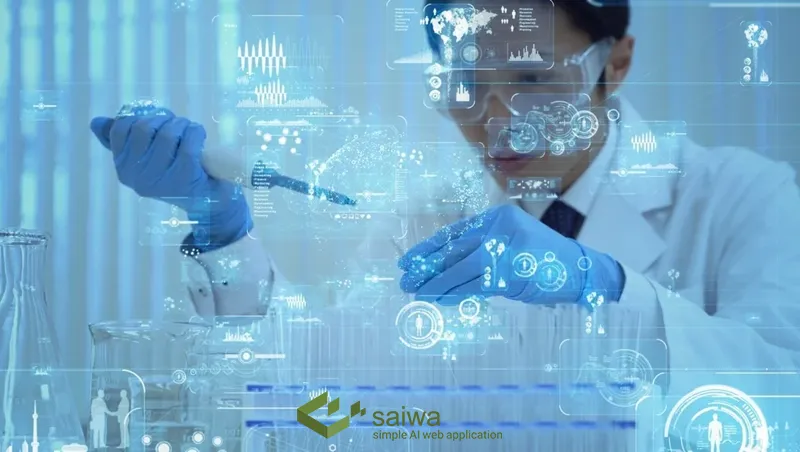
Workforce Augmentation and Training
For human workers, AI provides a wide range of assistance and skill enhancement. Intelligent wearables monitor worker fatigue, exposure, and health to prevent overwork and identify risks. Immersive virtual reality simulations create realistic environments for operator training. Collaborative robots perform dangerous tasks to keep workers safe. Expert systems provide on-demand assistance for following procedures, troubleshooting problems, and accessing technical documentation. These applications aim to maximize human capabilities while minimizing workplace hazards.
Emerging Trends and Applications
Ongoing advances in AI will continue to transform chemical manufacturing. Distributed AI edge devices will enable real-time optimization across extensive plant networks. Genome editing of industrial microorganisms will create customized biofactories guided by AI modeling. Blockchain and the Internet of Things will improve supply chain visibility. As computing power increases, end-to-end AI integration will emerge - from materials to molecules to manufacturing. The training of the next generation of chemists will also be supported by AI-driven platforms. Responsible development and adoption of these emerging capabilities will drive the industry forward.
Monitoring Manufacturing Lines and Quality Control
AI in chemical manufacturing apnest solutions also employs computer vision and other sensing technology for real-time monitoring:
High resolution cameras positioned along production lines to track products using image analysis as they move through manufacturing stages.
AI models trained to detect anomalies like contamination, color variation, and other defects from product images for instant correction.
Integrations allowing automated equipment shutdown or divert valves if models detect critical faults.
Historical data aggregation to determine trends and root causes of recurring quality deviations.
AI-powered intelligent monitoring becomes the omnipresent eyes along the line, ensuring consistently high, safe product quality levels.
Applications of AI in Chemical Manufacturing
AI provides significant value in optimizing chemical manufacturing processes such as reaction systems, separations, material handling, and instrumentation. Physics-based AI models can simulate processes for efficient design and prototyping. Online AI optimization adjusts variables such as temperature and pressure to improve yield and quality. Computer vision enables automated visual inspection of chemical products and packaging.
For quality control, AI statistical process control uses massive production data to quickly detect deviations from normal operations. Predictive maintenance uses AI anomaly detection on sensor data to predict equipment failures and prescribe maintenance. This minimizes downtime and prevents supply disruptions. In warehousing and logistics, AI optimizes inventory levels, shipment sizes, and delivery routes to keep the supply chain running smoothly.
Read Also: The Future of Chemistry | Machine Learning Chemical Reactions
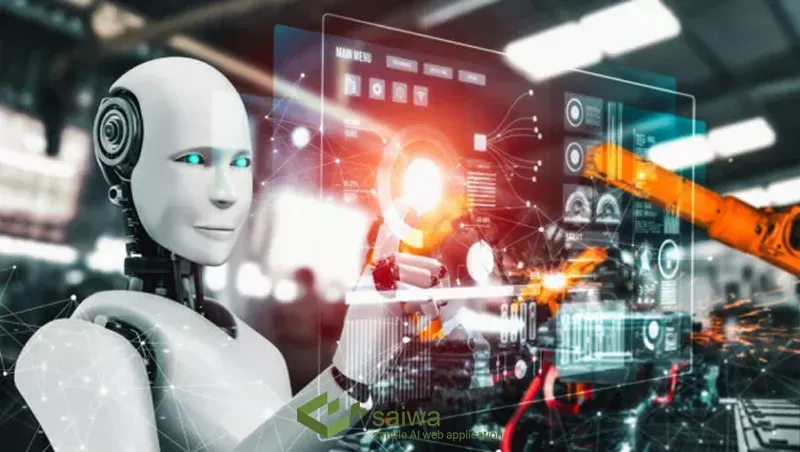
Challenges and Concerns in AI Implementation
As many benefits as AI can bring to chemical manufacturing, there are some challenges that need to be considered.
Data Security and Privacy
One of the major concerns in implementing AI in chemical manufacturing is data security and privacy. Chemical companies deal with sensitive information, and safeguarding this data is paramount. Robust cybersecurity measures must be in place to protect against data breaches and cyber-attacks.
Workforce Adaptation
The introduction of AI into chemical manufacturing requires a workforce that can adapt to and work alongside AI technologies. This necessitates training and upskilling employees to operate and maintain AI systems. It's crucial to prepare the workforce for the changes AI brings to the industry.
Future Trends and Innovations
The future of chemical manufacturing is increasingly autonomous, thanks to AI. Autonomous factories can operate 24/7, significantly improving efficiency and productivity. AI-powered machines can perform complex tasks with minimal human intervention, resulting in enhanced safety and consistency.
Quantum Computing and AI
The synergy between quantum computing and AI has the potential to revolutionize chemical manufacturing. Quantum computers can perform complex calculations much faster than classical computers. Combined with AI, they can solve complicated chemical problems, leading to more efficient and innovative processes.
Conclusion
In summary, artificial intelligence is playing an integral role in the evolution of smarter, safer, and more efficient chemical manufacturing. AI in chemical manufacturing enables the understanding and optimization of incredibly complex chemical processes. Its use continues to grow as computational capabilities improve alongside advances in sensor instrumentation and data analytics.
While AI cannot fully replace human ingenuity, when applied judiciously, it can dramatically augment human capabilities to drive innovation. With a thoughtful approach, manufacturers can leverage AI to improve productivity, sustainability, and their competitive advantage.