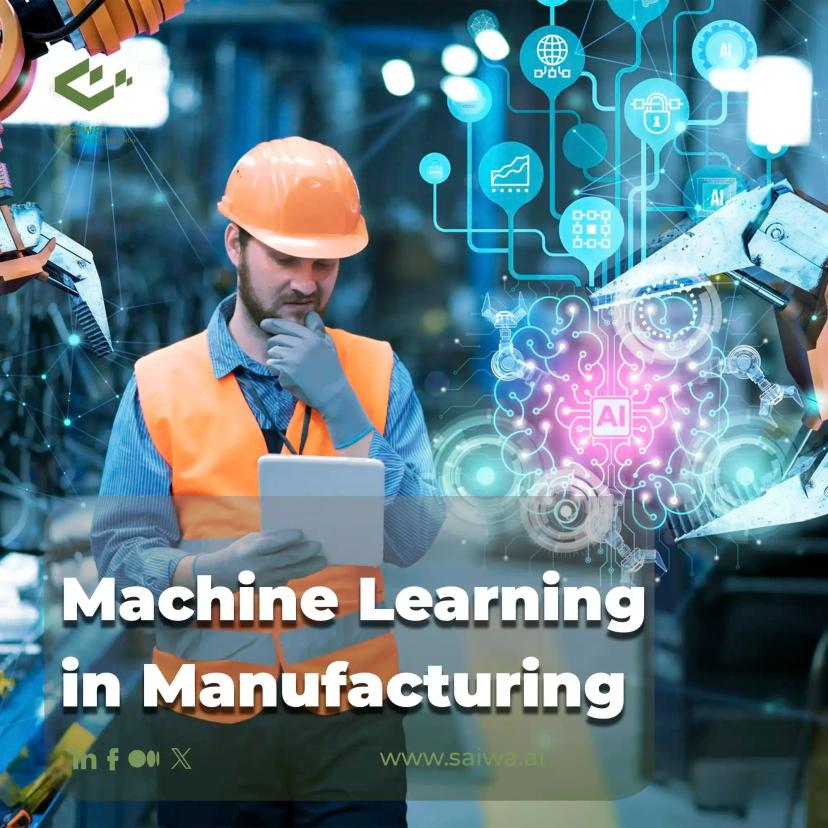
Machine Learning in Manufacturing | Applications & Benefits
In the ever-evolving landscape of technology, machine learning has emerged as a formidable force in various industries, including manufacturing. A branch of artificial intelligence, machine learning enables computer systems to learn and improve from experience without the need for explicit programming. Its transformative potential is now being realized in manufacturing, where it has the ability to optimize processes, increase productivity, and revolutionize decision-making. In this blog post, we will explore the fundamental concepts of machine learning, examine its specific applications in manufacturing, review its benefits to the manufacturing environment, and provide practical insights on how to embark on machine learning in manufacturing. Let's dive into an enlightening exploration of machine learning and its profound impact on the manufacturing industry.
What is Machine Learning?
Machine learning is a subfield of artificial intelligence (AI) that focuses on the development of algorithms and models that enable computer systems to learn and make predictions or decisions without being explicitly programmed. It involves using statistical techniques and data analysis to allow machines to learn and improve from experience automatically. Machine learning algorithms can analyze large amounts of data, find patterns and insights, and make predictions or decisions based on the data. Machine learning has many applications in different domains, such as medicine, finance, education, and entertainment. In this blog post, we will focus on how machine learning can be used in manufacturing, one of the most important sectors of the economy.
Read more: What Is Machine Learning as a Service?
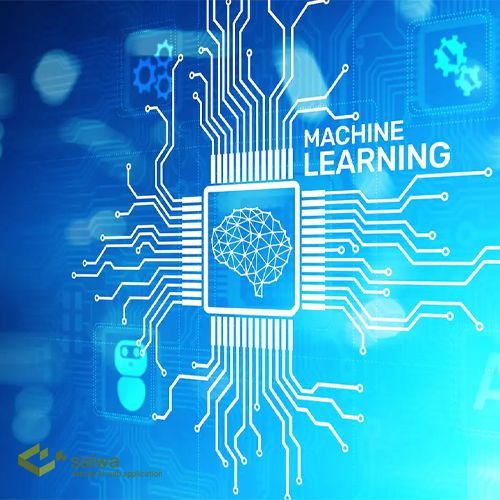
Machine learning in manufacturing?
Machine learning has significantly advanced in various industries, and manufacturing is no exception! In the manufacturing sector, machine learning refers to applying these techniques to analyze and interpret large amounts of data generated during production. By leveraging machine learning algorithms, manufacturers can extract valuable insights and patterns from data, leading to improved decision-making, process optimization, and overall operational efficiency. Using machine learning in manufacturing can help manufacturers improve their efficiency, productivity, quality, safety, and profitability by using data-driven methods to solve complex problems and enhance decision-making.
Read Also : The Future of Chemistry | Machine Learning Chemical Reactions
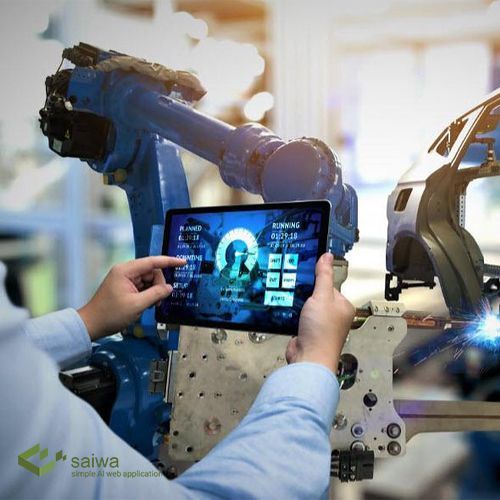
Applications of machine learning in manufacturing
Machine learning has found numerous applications in the manufacturing industry, revolutionizing traditional practices and enabling smarter, data-driven operations. Here are some key applications:
Quality Control and Defect Detection
Machine learning algorithms can analyze real-time data from sensors and cameras to identify defects in production lines. By detecting patterns and variations in product quality, identifying root causes and corrective actions, and automating quality checks, manufacturers can take proactive measures to minimize defects, maintain consistent quality standards, and improve customer satisfaction and loyalty.
Predictive Maintenance
Machine learning algorithms can analyze historical equipment data and identify patterns that indicate potential failures or maintenance needs, predict and prevent failures and malfunctions, and schedule preventive maintenance and repairs. Manufacturers can proactively schedule repairs or replacements by predicting when machines will likely need maintenance, reducing downtime and improving overall equipment effectiveness.
ML for Accelerated R&D
Product design and prototyping constitute a lengthy, costly R&D phase. Machine learning enables accelerated innovation:
Physics-based simulations can be optimized in an automated, parallelized manner using techniques like genetic algorithms and reinforcement learning to accelerate design exploration.
Generative design techniques leverage vast computations to create manufacturable, high-performing product designs meeting requirements.
Instead of physical prototyping, ML surrogate models rapid test virtual prototypes under varied simulated test conditions at a fraction of the time and cost.
Synthetic data generation techniques like generative adversarial networks amplify limited real-world training data to improve model accuracy and generalization.
By leveraging simulation, optimization and data synthesis powered by machine learning, manufacturers can significantly compress R&D timelines to boost innovation velocity.
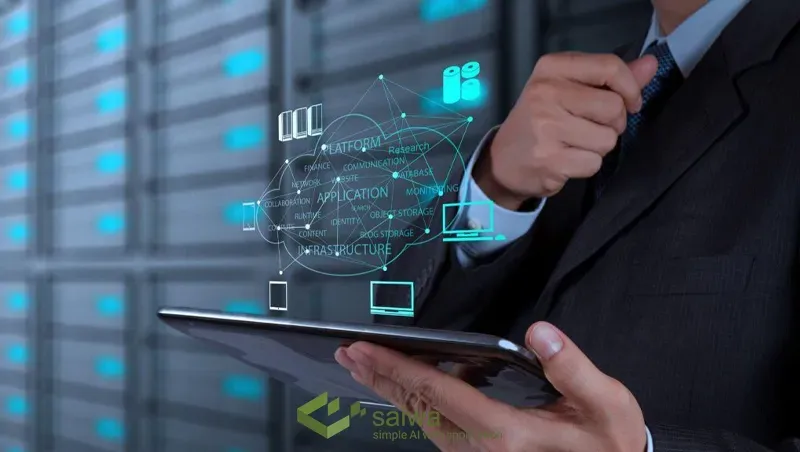
Supply Chain Optimization
Machine learning can optimize the supply chain by analyzing data from multiple sources, such as sales, inventory, and customer demand. Manufacturers can streamline supply chain processes by predicting demand patterns, optimizing inventory levels, identifying potential bottlenecks, and ensuring timely product delivery. For example, machine learning can be used to monitor and control production parameters, predict and prevent failures and defects, optimize the use of resources and energy, and improve coordination and collaboration between machines and humans.
Demand Forecasting
Manufacturers can use machine learning models to analyze historical sales data, market trends, and other relevant factors to predict future demand. As a result, they can optimize production schedules, manage inventory levels, track shipments and deliveries, and efficiently align resources to meet customer demand while minimizing costs, waste, and emissions.
Design
Another use of machine learning in manufacturing is to help manufacturers design better products and processes using data from previous designs, customer feedback, market trends, and simulations. Machine learning can be used to generate new product ideas, optimize product features and specifications, reduce design errors and costs, and speed time to market.
Improved Demand Forecasting with ML
Accurately predicting customer demand is crucial for efficient manufacturing planning and inventory management. Machine learning unlocks more intelligent demand forecasting capabilities:
Time series forecasting techniques like ARIMA and LSTM neural networks can uncover seasonal, cyclical and trend-based patterns from historical order data. This provides superior demand visibility.
Incorporating exogenous variables like past promotions, holidays, competitor actions, market trends etc. improves forecast accuracy. Feature engineering helps extract useful signals.
Multi-level forecasting can predict granular SKU-location combinations versus aggregate volumes only. This optimization of the demand-supply mismatch.
Simulation of multiple demand scenarios allows contingency planning. Tactics like clustering help segment products into forecastability profiles.
Overall, machine learning in manufacturing provides manufacturers with data-driven foresight to calibrate production schedules, workforce levels and inventory buffers minimizing over or under-supply risks.
Reduced Downtime Through ML
Unexpected downtime of machinery disrupts production targets and reduces asset lifetime. Machine learning enables reducing such inefficiencies:
Monitoring sensor data like vibration, temperature, etc., and applying anomaly detection algorithms helps detect equipment issues warranting intervention before failure.
Analysis of maintenance logs using techniques like sequence mining reveals common failure chains. Addressing early warning signs prevents shutdowns.
Predictive maintenance models forecast expected time-to-failure for components based on operational data. This enables optimally scheduling maintenance to minimize downtime.
Optimization algorithms can intelligently sequence changeovers, setups, and material handling to reduce wait times leveraging advanced analytics.
By leveraging machine learning in manufacturing on operational data, manufacturers can minimize disruptive downtime through predictive approaches.
Benefits of machine learning in a manufacturing environment
Machine learning can benefit manufacturers who adopt it in their operations. Some of the most important benefits are listed below:
1. Improved Efficiency and Productivity
Machine learning algorithms can identify inefficiencies in the manufacturing process and suggest improvements. By automating repetitive tasks, optimizing production schedules, and reducing downtime, manufacturers can enhance overall efficiency and productivity.
2. Cost Reduction
By optimizing processes, reducing scrap and rework, and improving supply chain management, machine learning can help manufacturers reduce costs and be able to satisfy their customers. Predictive maintenance can also prevent costly equipment failures and unplanned downtime.
3. Enhanced Quality Control
The algorithms of machine learning enable real-time monitoring and analysis of product quality, minimizing defects and ensuring consistent quality standards. This leads to higher customer satisfaction and reduced waste or rework.
4. Data-Driven Decision Making
With machine learning in manufacturing, manufacturers are able to leverage vast amounts of data and extract actionable insights. By making data-driven decisions, manufacturers can improve process efficiency, identify opportunities for innovation, and stay ahead of the competition.
5. Improved safety:
Machine learning can help manufacturers protect their workers, machines, and environment by identifying and mitigating risks and hazards.
6. Workforce Augmentation Through ML
While automation displaces certain manufacturing roles, machine learning also aims to augment human capabilities:
Intelligent tutoring systems powered by machine learning can deliver personalized training tailored to each operator's strengths and weaknesses based on interactions, minimizing skill gaps.
In semi-automated production lines, robots handle hazardous and injury-prone material handling while operators focus on quality control and customization.
Computer vision techniques enable real-time guidance to assembly line workers on optimally performing manual operations increasing consistency.
Exoskeletons and prosthetics embedded with machine learning improve mobility and minimize risk of strain injuries for production floor staff through responsive assistive motion.
Integrating human oversight and judgment with machine learning automation creates an ideal symbiotic partnership between workers and technology.
How to get started with machine learning
Machine learning is not a one-size-fits-all solution for every manufacturer. Each manufacturer has different needs, goals, challenges, and opportunities that require different approaches and solutions. Getting started with machine learning in the manufacturing industry requires a systematic approach. Here are some steps to consider:
1. Identify Business Goals:
Determine the specific goals you want to achieve through machine learning, such as improving quality control or optimizing production processes.
2. Data Collection:
Gather relevant data from various sources within your manufacturing environment. This can include production data, sensor data, maintenance records, and customer feedback.
3. Data Preprocessing:
Clean and preprocess the collected data to ensure its quality and suitability for machine learning algorithms. This can involve data cleaning, normalization, and feature engineering.
4. Model Selection:
Choose the appropriate machine learning algorithms based on your specific objectives and data characteristics. This can include supervised learning, unsupervised learning, or reinforcement learning algorithms.
5. Model Training and Evaluation:
Train the selected machine learning models using the prepared data and evaluate their performance. This involves splitting the data into training and testing sets, tuning model parameters, and assessing the models' accuracy and predictive capabilities.
6. Implementation and Deployment:
Integrate the trained machine learning models into your manufacturing processes and systems. Monitor their performance and make necessary adjustments as needed.
7. Data Security and Privacy:
When implementing machine learning in manufacturing, it's essential to prioritize data security and privacy. Ensure that sensitive data is appropriately protected, and have relevant regulations and standards. Accomplish measures such as encryption, access controls, and data anonymization to safeguard information.
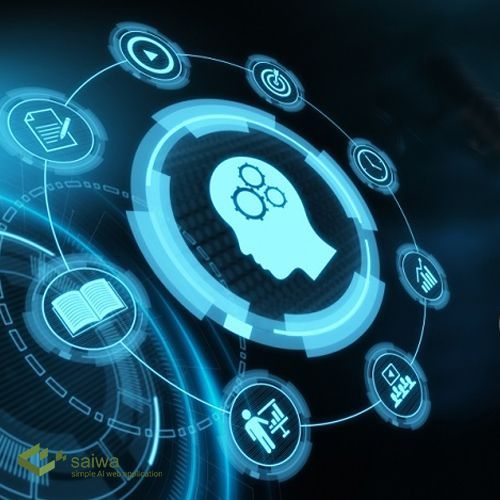
8. Continuous Learning and Improvement:
Machine learning is an iterative process. Encourage a culture of continuous learning and improvement within your organization. Regularly update and retrain your models with new data to adapt to evolving manufacturing conditions and maintain optimal performance.
By following these steps, manufacturers can embark on their machine learning journey and leverage its potential to drive innovation, efficiency, and competitiveness in the manufacturing industry.
Conclusion
Machine learning transforms the manufacturing industry by enabling data-driven decisions, optimizing processes, and improving overall efficiency and productivity. By applying machine learning techniques to manufacturing, manufacturers can gain valuable insights from their data, improve product quality, reduce costs, and stay competitive in an ever-evolving marketplace.