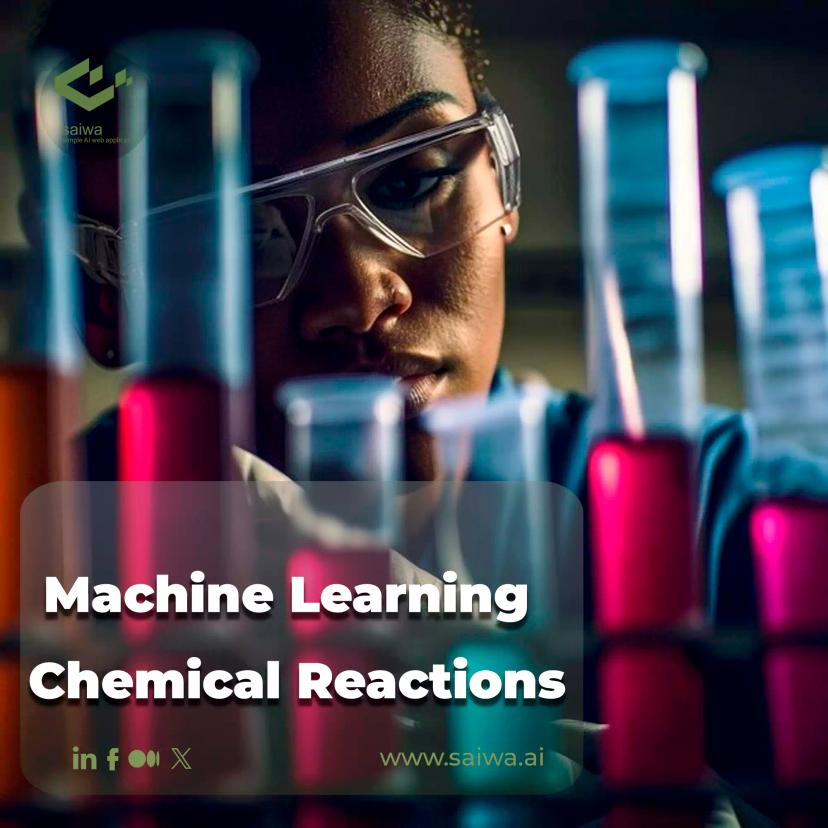
The Future of Chemistry | Machine Learning Chemical Reactions
Designing and optimizing chemical reactions is a complex, multifaceted problem. Chemists have traditionally relied on knowledge, experimentation, and trial-and-error approaches to design synthetic pathways and optimize reaction conditions such as temperature, solvent, and catalyst choice. This makes systematically exploring the vast chemical space to discover new materials tedious and expensive. However, recent advances in machine learning are automating chemical research by rapidly predicting reactions, properties, and optimal conditions from data. This blog post explores the applications of machine learning chemical reactions, methods, data sources, benefits, and challenges associated with integrating artificial intelligence into chemical research and development.
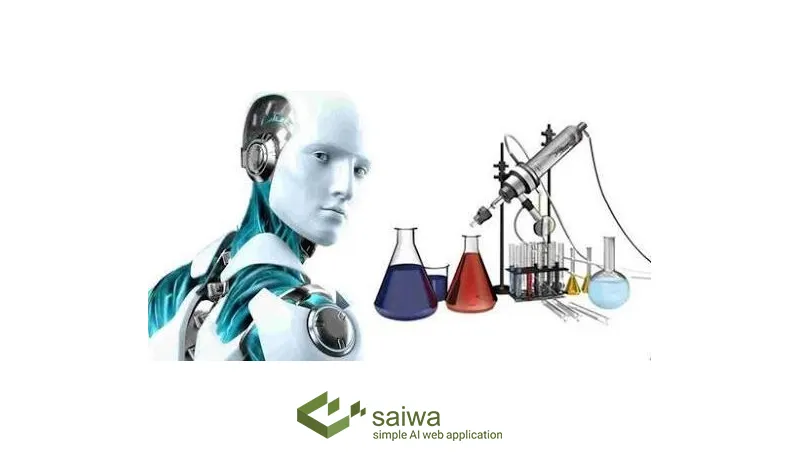
Machine Learning Methods for Chemical Research
Various machine learning techniques drive chemical AI:
Graph Neural Networks
Atoms and their connections are graphically represented, forming molecular structures where atoms serve as nodes and chemical bonds as connecting edges. Graph networks learn molecular embeddings that capture topological semantics and enable structure-based prediction. SchNet is a popular chemical graph network.
Meta-Learning Algorithms
The goal of meta-learning is to learn optimal learning rules from previous tasks so that models can quickly adapt to new tasks with few examples. This allows fast adaptation to new reaction types with limited experiments.
Transfer Learning
Leverage knowledge gained from specific reaction mechanisms and transfer that understanding to accelerate learning of related new reactions. Reuse of functions reduces retraining.
Active Learning
Instead of random experiments, active learning techniques strategically select informative experiments that are expected to rapidly increase the model's knowledge. This provides data efficiency.
In addition, unsupervised learning and generative modeling allow the use of unlabeled data, while reinforcement learning optimizes sequential experiments. Hybrid AI systems show promise by combining statistical and mechanistic models. Overall, machine learning provides the predictive power to guide chemical discovery.
Read Also: Active learning in machine learning | Use Cases and Frameworks
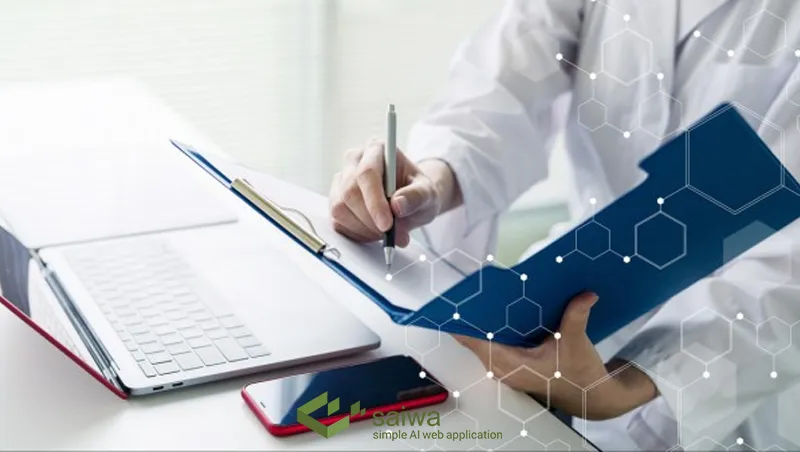
Types of Algorithms for machine learning chemical reactions
A wide array of machine learning algorithms is employed in the domain of chemistry. These algorithms are tailored to address specific aspects of chemical reactions, ranging from reaction classification to property prediction. The adaptability of these algorithms makes them indispensable tools for chemists and researchers.
Supervised Learning
Supervised learning algorithms are pivotal in reaction classification and property prediction. Chemists utilize these models to identify reaction types, predict product properties, and optimize reaction conditions. An example of their application is the prediction of chemical compound toxicity, streamlining drug development and safety testing processes.
Unsupervised Learning
Unsupervised learning is primarily used for clustering and data exploration in chemical reactions. These algorithms uncover hidden patterns and relationships in large data sets. In chemoinformatics, unsupervised learning helps group chemical compounds with similar structures, facilitating drug discovery and materials science.
Reinforcement Learning
Reinforcement learning, a subset of machine learning, plays a significant role in optimizing chemical reactions. Chemists employ these models to improve reaction conditions and predict reaction pathways. By providing automated decision-making capabilities, reinforcement learning is at the forefront of reaction optimization and sustainable chemistry.
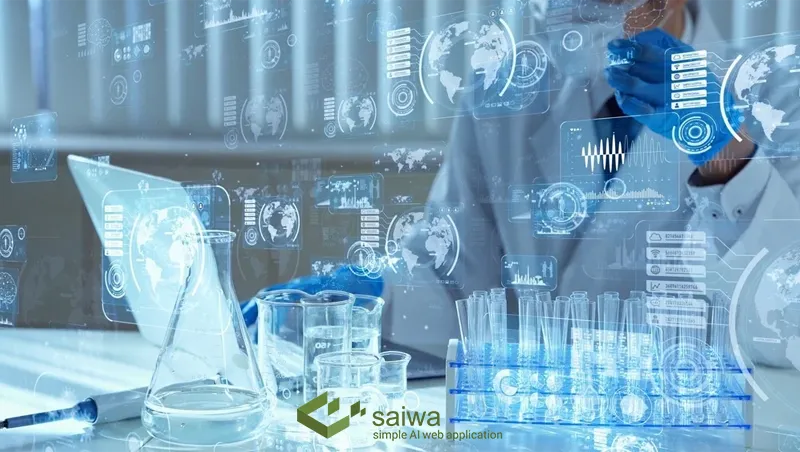
Applications of Machine Learning in Chemistry
Machine learning is advancing chemical research across multiple fronts:
Read Also: AI in Chemical Manufacturing | A Path to Quality and Safety
Reaction Prediction
Forward reaction prediction involves using machine learning models to predict the major expected products when given a set of reactants and reagents. This aids discovery by avoiding exhaustive enumeration of combinations. Neural graph learning algorithms excel at learning molecular representations to predict synthesis outcomes.
Retrosynthesis Analysis
Retrosynthesis prediction takes a target end molecule and plans optimal synthetic routes mapping backward from readily available starting materials. Tree search algorithms coupled with reaction rules help assemble pathways efficiently. This automates the identification of viable routes.
Catalyst Discovery
Finding optimal catalysts to improve reaction efficiency and selectivity poses combinatorial complexity. Machine learning models trained on quantum chemistry simulations, catalyst molecular descriptors, and experimental data enable faster screening and optimization.
Solvent Selection
Choosing suitable solvents enhances selectivity and yield. Machine learning on solvent attributes like polarity helps select optimal solvents. Reinforcement learning allows dynamic tuning of solvent batches. Reduced solvent waste benefits sustainability.
Reaction Condition Optimization
Varying factors like temperature, pressure, concentration, etc. change reaction kinetics and yield. Bayesian optimization algorithms coupled with surrogate models intelligently select experiments to rapidly optimize conditions with minimal trials.
Machine learning amplifies chemists’ expertise to explore chemical space faster.
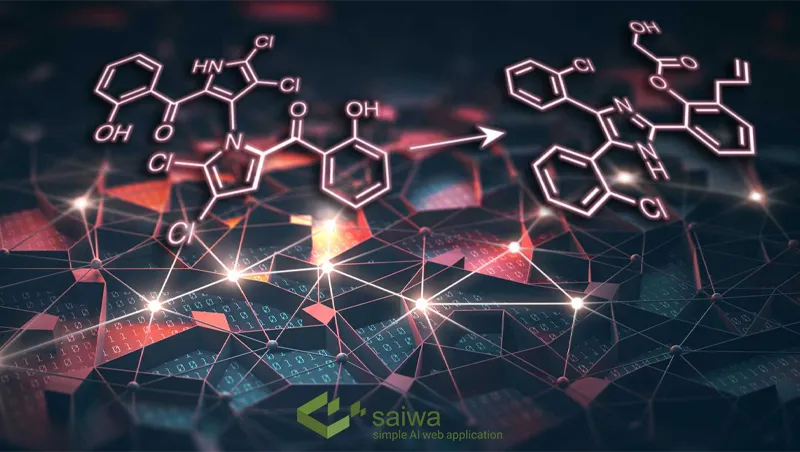
Available Data for Training ML Models
Useful data sources for training models of machine learning chemical reactions include:
Published Reaction Records
Journals like Science publish verified reaction datasets containing reactants, reagents, conditions, yields, etc. Patent records also provide validated reaction workflows. These provide labeled training examples.
Quantum Chemistry Simulations
Methods like density functional theory provide physicochemical insights like reaction energies, transition states, and molecular properties for training data.
Text Mining Reactions from Literature
Powerful NLP techniques extract reaction information like chemical graphs and properties from unstructured text in publications to accumulate big data.
Experimental Data
Laboratory experiments provide well-controlled data on reaction outcomes, kinetics, and conditions. Sharing this data enables collaborative modeling.
While data scarcity is a challenge, combining public data with private experimental results generated in the field enables customized ML solutions.
Accelerating Chemical R&D Using Machine Learning
Thoughtfully developed machine learning in chemical reaction systems confers multiple advantages to research programs:
Faster Lead Discovery
Navigating huge molecular search spaces to identify candidate drug molecules or materials can be exponentially faster using learned predictive models versus ad hoc screening.
Lower Development Costs
Optimally recommending reagents, conditions, and synthetic routes minimizes expensive failed experiments. Model-guided experimentation provides data efficiency.
Safer Chemical Design
Models can predict toxicity early in the design process and suggest modifications to improve safety. This avoids downstream problems.
Democratized Access
Cloud-based machine learning platforms enable even small labs to benefit from advanced algorithms without requiring extensive in-house computing resources.
Machine learning increases research and development productivity, reduces costs, and mitigates risks in bringing new chemicals and materials to market.
Conclusion
Recent advances in machine learning are transforming tedious reaction optimization and molecular discovery processes into rapid, automated workflows. Powerful AI techniques can learn from chemical data to accurately predict synthetic pathways, properties, optimal conditions, and undiscovered structures.
Integrating chemical reaction machine learning into experimental and computational chemistry frees researchers from restrictive trial-and-error searches to unleash creativity. The future of chemical innovation will increasingly leverage artificial intelligence to unlock new materials, drugs, and compounds that benefit humanity. However, focused human oversight and wisdom must remain essential to ensure ethical, responsible progress. Overall, chemistry is poised for a machine learning revolution.