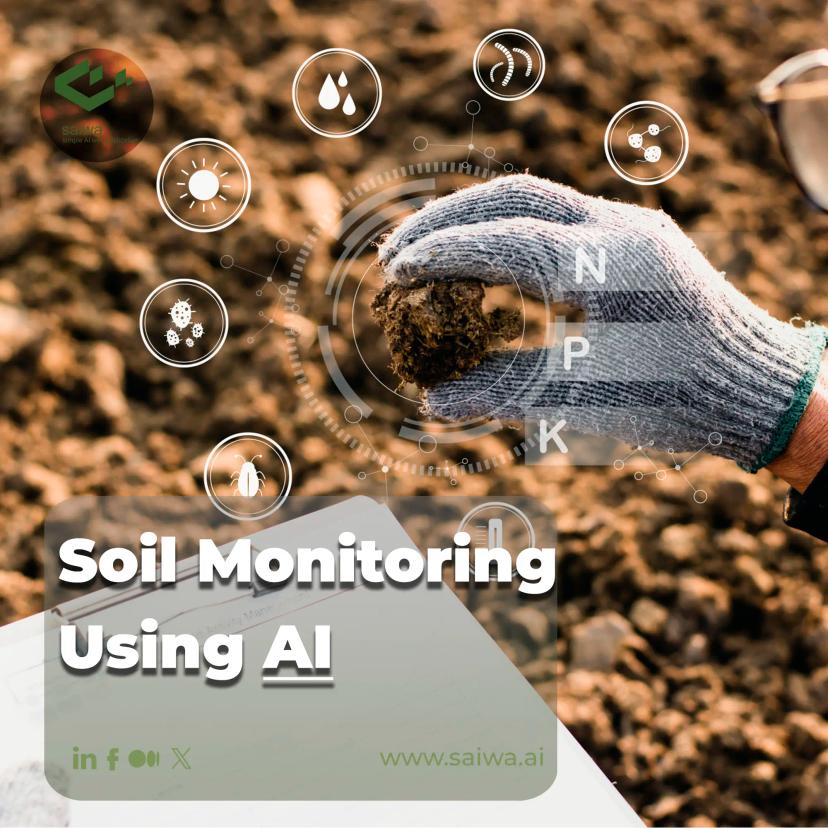
Revolutionizing Agriculture | Enhanced Soil Monitoring Using AI Insights
Soil health is critical to functional and productive agricultural systems. Regular monitoring of soil parameters is crucial for high-quality crops, minimal environmental damage, and supporting a dynamic underground microbial ecosystem. However, accurately measuring soil components is challenging due to considerable variability and complexity. Advanced artificial intelligence solutions can help solve this challenge.
Recent advancements in deep learning, spectral imaging, agricultural robotics, and predictive analytics can reveal novel approaches for accurately analyzing and interpreting soil properties in diverse environments. By integrating with real-time sensing data and satellite feeds, soil modeling powered by artificial intelligence enhances evaluation precision and offers practical insights for crucial decision-makers. This expedites the response to emerging soil challenges while devising sustainable land-use strategies for the long haul.
This blog post will provide a comprehensive overview of emerging soil monitoring using AI techniques. We survey innovative algorithms as well as sensor platforms and evaluation metrics tailor-made for soil ecology. The potential as well as challenges of applying AI to digitize soil systems are assessed. Overall, the convergence of hyperspectral data flows, unmanned vehicles, cloud analytics, and intelligent modeling promises a new era in mastering soil sustainability.
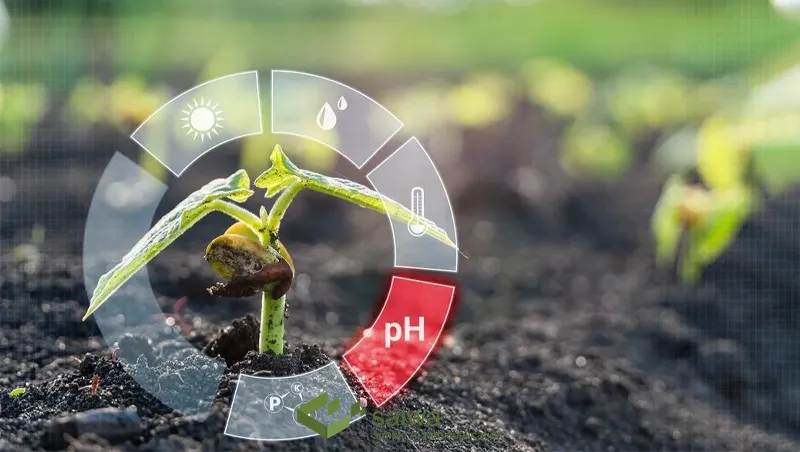
The importance of crop and soil monitoring using AI in agriculture
Artificial intelligence (AI) is an essential aspect of modern agriculture, offering farmers vital information about the health of their land and crops. For several reasons, including maximizing yields, preserving resources, and guaranteeing the long-term viability of agricultural methods, it is imperative to monitor crop health and soil conditions.
First and foremost, farmers may recognize and promptly resolve problems affecting crop health thanks to crop and soil monitoring using AI. Utilizing cutting-edge technologies like computer vision, machine learning, and remote sensing, farmers can see early indicators of illness, stress, or food shortages in their crops. With this knowledge at hand, farmers may minimize risks and avoid possible crop losses by responding proactively, which will eventually boost yields and profitability.
Furthermore, timely and precise data on soil conditions and crop health enable farmers to manage resources with knowledge. Farmers may optimize inputs like water, fertilizers, and pesticides, cutting waste and limiting environmental effects, by knowing the unique requirements of their crops and land. As an example, farmers may use reliable irrigation and fertilization strategies suited to the requirements of their crops thanks to real-time assessments of soil moisture and nutrient levels offered by AI-powered soil sensors and Internet of Things devices.
Moreover, farmers can implement more sustainable farming methods thanks to crop and soil monitoring using AI. Farmers can enhance soil health and biodiversity by decreasing their need for chemical pesticides and fertilizers by optimizing resource utilization and limiting inputs. Furthermore, farmers can use AI-driven predictive modeling to assist them foresee environmental problems like pest outbreaks or droughts, enabling them to put adaptive tactics in place to reduce risks and preserve productivity.
Based on crop and soil monitoring using AI is vital for agriculture because it provides farmers the information and tools they need to maximize yields, preserve resources, and advance sustainability. Farmers may make intelligent choices that promote productivity, profitability, and environmental stewardship by employing AI technology to gather, analyze, and interpret data on crop health and soil conditions.
Read Also: Exploring the Future with AI-Based Farm Equipment
Data Collection Methodologies
The key to soil monitoring utilizing AI and any robust AI system is high-quality, well-structured data from the application environment. The monitoring process benefits from recent progress in remote sensing platforms and nearby on-site sensors. For instance, satellite platforms, such as NASA’s Soil Moisture Active Passive (SMAP) mission, offer frequent microwave data feeds to capture global soil moisture levels and conditions at 5-10 kilometer resolutions. Combining optical and infrared data enables the determination of local vegetation densities and their impact on soil cover. Additionally, high-resolution satellite imagery at 25-100 meter scale supports the mapping of regional soil parameters.
Historical datasets regarding soil types, management practices, and crop yields provide reliable baseline information for AI models. Government and academic soil surveys that detail numerous sample points and varying features are now available for use. When these are combined with other agricultural data layers, they result in rich digital soil counterparts that reflect the diverse range of soils found in the real world.
Static wireless sensor nodes and internet-of-things (IoT) devices are scattered across farms at the field level to sample hyperlocal moisture patterns, salinity fluctuations, and nutrient dynamics. Probes are also used to accurately characterize soil texture, water retention, aeration, and mineralization rates without disturbing soil structure. Additionally, robots and unmanned ground vehicles equipped with spectral analyzers provide on-the-go scanning with centimeter accuracy. Condition indicators such as soil respiration also indicate biological activity. The combined data fusion offers soil monitoring from multiple perspectives at minute resolutions.
AI Techniques for Soil Monitoring Using AI
This real-time influx of diverse soil data at global and hyperlocal scales calls for AI to efficiently interpret indicators, fuse datasets and infer conditions.
Visual Interpretation with Convolutional Neural Networks (CNN)
Convolutional neural networks (CNN) are able to classify high-resolution aerial soil images as well as scans from ground robots to specific typologies and flag visual patterns indicative of issues.
Object Detection for Soil Characteristics
Object detection models identify the coverage of vegetation, crop residue, gully erosion, and more from such images. Spectral signature models analyze elemental compound mixtures in a location using sensor time series or spectral camera data. Class activation map approaches on hyperspectral data pinpoint important soil bands.
Temporal Dynamics Tracking with Recurrent Neural Networks (RNN)
Recurrent neural networks (RNNs) monitor temporal changes in moisture, salinity, and nutrient levels in fields using delayed sequences, uncovering critical seasonal effects for agriculture. RNNs also predict future variations based on climate data. In contrast, graph neural networks (GNNs) analyze the intricate connections and interactions among soil structure, crop biology, and added amendments that affect quality.
Variational autoencoders capture essential variability through compressed representations. When combined with physicochemical modeling, deep networks, even black-box ones, produce soil insights with generalizability.
Outlier Detection Algorithms
Outlier detection algorithms detect unusual multivariate patterns in soil data, indicating contamination events or ecosystem disruptions. Natural language processing models extract indicators from text reports and literature linking soil biome state changes to above-ground phenomena. Reinforcement learning investigates best practices for fertilizers, pesticides, and irrigation, tailored to highly specific field areas. Overall, AI-powered soil monitoring enables both analytical scoring and contextual decision support.
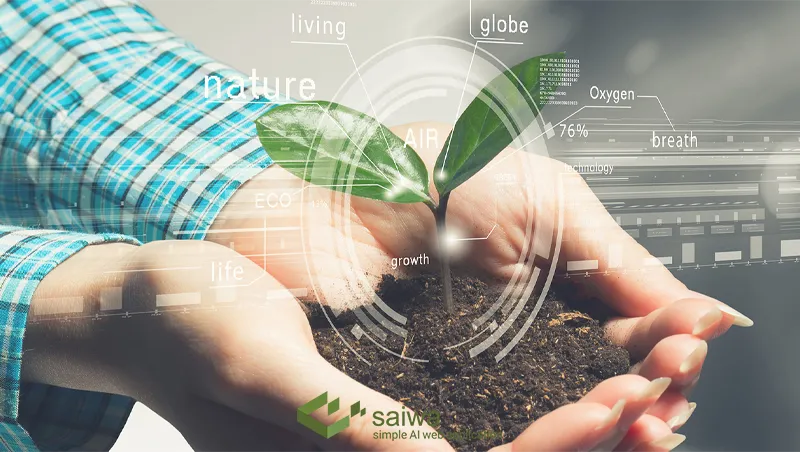
Internet of Things (IoT) and Sensor Networks for Crop Monitoring Using AI
The Internet of Things (IoT) plays a vital role in crop monitoring using AI by enabling the collection of real-time data from various sensors deployed in agricultural fields. These sensors can measure a wide range of parameters, including soil moisture, temperature, humidity, and nutrient levels, providing valuable insights into crop health and growth conditions.
IoT sensor networks consist of numerous nodes scattered throughout the field, each equipped with various sensors and wireless communication capabilities. These nodes continuously transmit data to a central gateway or cloud platform, where it is processed and analyzed using AI algorithms to detect patterns, identify potential issues, and make informed decisions. The integration of IoT and crop monitoring using AI enables farmers to monitor their crops remotely and receive real-time alerts and notifications regarding any deviations from optimal conditions. This allows for timely interventions, such as adjusting irrigation schedules, applying fertilizers, or implementing pest control measures, ultimately leading to improved crop yields and resource efficiency.
Unmanned Aerial Vehicles (UAVs) and Drone Technology in Crop Monitoring Using AI
Unmanned Aerial Vehicles (UAVs), commonly known as drones, have emerged as a powerful tool for crop monitoring using AI. These aerial platforms can capture high-resolution imagery and gather data over large areas quickly and efficiently, providing a comprehensive view of crop health and field conditions. Drones equipped with specialized cameras, such as multispectral or hyperspectral sensors, can detect subtle changes in crop health and identify areas affected by stress, disease, or nutrient deficiencies. This data can be analyzed using AI algorithms to generate detailed vegetation indices, enabling early detection and targeted interventions.
Additionally, crop monitoring using AI and drone technology can be combined with other technologies, such as LiDAR (Light Detection and Ranging), to create 3D models of crop canopies and estimate biomass and yield potential. This information can be used for more accurate yield forecasting and optimizing resource allocation throughout the growing season.
Evaluation Metrics and Benchmarks
Validating soil monitoring through AI model effectiveness, particularly in real-world field deployments, is crucial. Multivariate goodness-of-fit metrics like R-squared are the standard for assessing model alignment with ground truth. Classification accuracy, F1 scores, and Recall indicate the model's proficiency with soil typing. However, due to constraints in sample collection and labeling challenges, constructing reliable training and test datasets presents challenges. Thus, utilizing semi-supervised, self-supervised, and sim-to-real techniques can boost model robustness even in the face of limited data availability.
Online learning systems update models dynamically as new labeled samples stream in. It is important to specify acceptable model uncertainty bounds and confidence intervals. Ultimately, agricultural success is determined by crop yields, farmer costs, and sustainability indices, so tying AI outputs to these tangible metrics is crucial. Economic value differentiation identifies the best-performing systems. As datasets expand through international soil data sharing and with the expansion of testing to new geographies, model benchmarks will enhance.
Decision Support Systems and Operationalization
To enable precision agriculture, AI-powered soil monitoring must provide decision support for stakeholders through user-friendly interfaces. Farmers can use apps that identify micronutrient deficiencies in specific areas and suggest the corresponding fertilizers for maximum yield. Agricultural advisors can integrate soil state forecasts with weather outlooks on dashboards to plan sustainable crop rotations. Carbon credit investors and sustainability certification agencies can measure farming emission offsets and sequestration capabilities through monitored soil organic content.
Soil scientists can trace mineralization and salinization risks at a regional level to plan amendments and counter degradation. Cross-referencing pollution data with production levels can highlight regulatory infractions that require investigation by authorities. AI-assisted soil diagnostics can also assist in identifying high-yield cultivation sites. Operationalizing intelligent analytic systems necessitates standardizing the collection of sensor data, establishing protocols for updating field-level models and integrating them seamlessly into current farm equipment and legacy systems.
Challenges and Future Outlook
Despite the immense promise in augmenting soil monitoring using AI, realizing the full potential entails overcoming key challenges. Most prominently, compiling massive labeled soil sample datasets across diverse agroecological zones for training universal models is extremely resource-intensive. Thankfully, international open data access initiatives and digital soil mapping collaboration help alleviate this.
Enhancing Scientific Receptiveness
Explaining the logic and uncertainty of Blackbox models can enhance scientific receptiveness. Innovative techniques are needed to bridge gaps between simulator-synthetic data and physical reality and to manage the sparsity of sensor data.
On-Farm Deployment Challenges
Deploying AI-based decision tools on farms requires upgrading computing infrastructure and strengthening cybersecurity. Coordinating regulatory data evaluations and creating technology skill development pathways for users increases adoption. However, the practical benefits of round-the-clock intelligent diagnostics and data fusion for evidence-based soil conservation ensure that AI will become an integral part of modern agronomy.
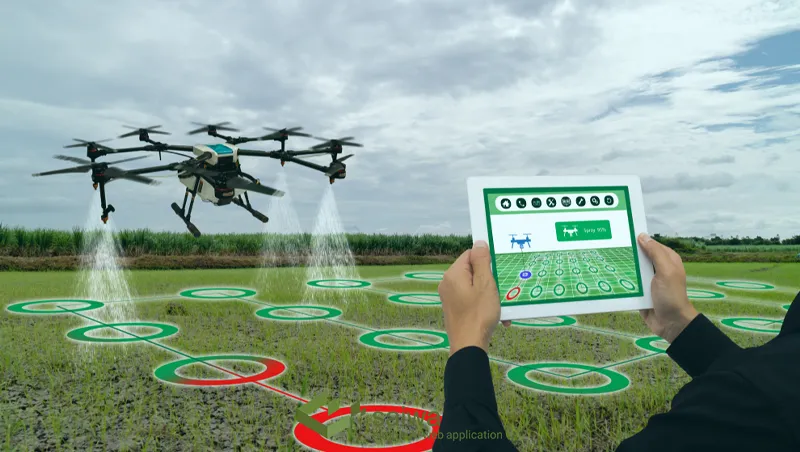
Conclusion
From modeling soil biota on the nanoscale to observing landscape-wide changes from space, AI plays a pivotal role in soil monitoring across different scales. For the first time, AI datasets enable rapid analysis of vast, heterogeneous, and temporal soil monitoring. By integrating satellite readings, proximal soil physics measurements, and chemical indicators with weather and crop data in data-driven models, AI powers the next Green Revolution with a focus on soil health. Science-backed soil management plans are enabled to an unprecedented degree, generating actionable analytics. The advent of data-intensive, sustainable smart farming systems worldwide is marked by the application of tremendous data science toolkits to unravel soil systems.