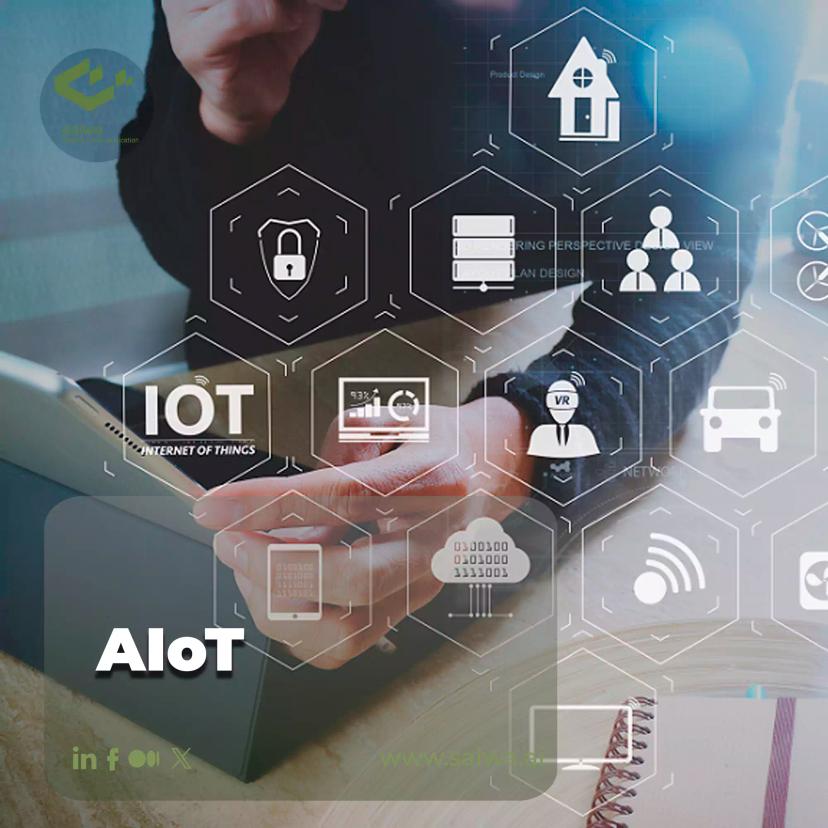
AIoT | The Emergence of Artificial Intelligence of Things
The Internet of Things (IoT) has connected billions of devices and objects to the digital world through embedded sensors and connectivity. However, IoT networks generate immense amounts of fragmented data that is difficult for organizations to analyze and act upon. Artificial intelligence (AI) provides techniques for recognizing patterns, interpreting insights, and automating complex decisions. Integrating AI into IoT ecosystems creates a new wave of technologies under the umbrella of AIoT. By combining data from connected things with intelligent algorithms, IoT systems can become smarter, more responsive, and capable of optimized autonomous operation.
By infusing artificial intelligence into smart devices and processes, AIoT has the potential to transform sectors ranging from manufacturing to agriculture. This paper provides a comprehensive reference on the technological foundations, capabilities, use cases, implementation challenges, and future trajectory of this emerging technology paradigm.
Read more: IOT machine learning | How do they work together?
What Is AI?
Artificial Intelligence refers to computer systems capable of performing tasks that normally require human intelligence, such as visual perception, speech recognition, and decision-making. AI uses advanced algorithms and statistical models to enable capabilities like machine learning and natural language processing.
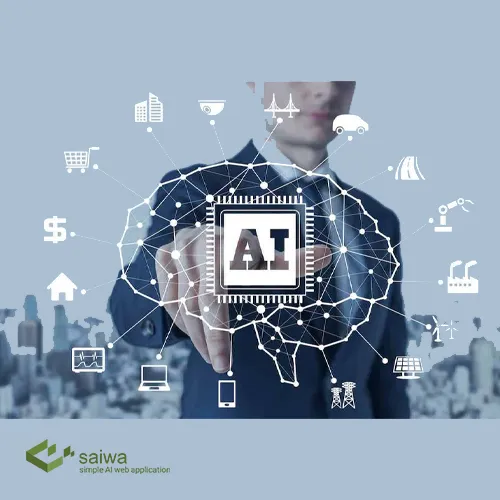
What is IoT?
The Internet of Things, commonly called IoT, refers to the network of physical objects and devices embedded with sensors, software, and connectivity that enable them to connect and exchange data over the Internet. This includes everything from consumer products such as smartphones and appliances to industrial equipment such as manufacturing machinery. The connectivity and data exchange capabilities enable IoT devices to be monitored and controlled remotely, unlocking new functionality and insights.
By embedding Internet connectivity at the device level, everyday objects can become SMART, able to collect and analyze data in real-time and integrate seamlessly into digital systems. IoT represents an evolution of the Internet from connecting people to people (social media, email) and people to information (the web) to now connecting physical things to each other, to infrastructure, and to people. This technology shift is unlocking opportunities for remote control, data-driven services, autonomy, and distributed intelligence in a wide range of settings, from homes to factories to cities. The proliferation of low-cost sensors and processors is enabling the IoT concept to become a reality as more connected things are deployed across industries.
What is AI IoT?
AIoT stands for Artificial Intelligence of Things and refers to the combination of artificial intelligence technologies with the Internet of Things. It involves the integration of AI algorithms and connectivity into IoT devices and systems, enabling intelligent analytics and autonomous decision-making capabilities. In AIoT architectures, machine learning and AI models run directly on IoT gateways and devices rather than relying solely on cloud analytics. This enables real-time localized analytics, operation in remote locations with limited connectivity, enhanced security and privacy through on-device data processing, and reduced data transmission costs.
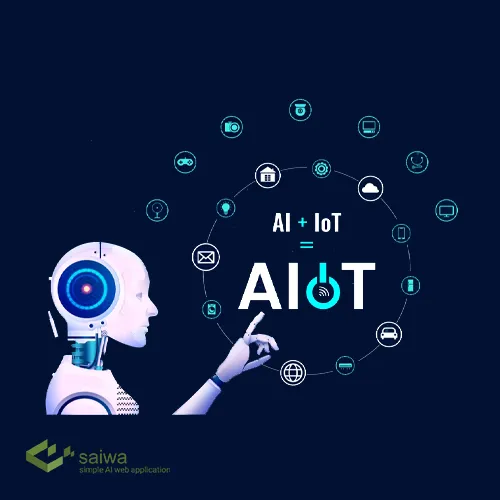
AIoT aims to make IoT networks smarter, more responsive, and capable of optimized autonomous operation by embedding intelligence at the edge. Key enablers of AIoT include advanced machine learning models, increased computing power, and 5G connectivity. By infusing AI into the IoT infrastructure, devices and machines can continuously learn, reason, and adapt to new data. The convergence of AI and IoT promises to revolutionize many industries and systems, including manufacturing, agriculture, smart cities, and healthcare.
Reasons for Integrating AIoT&AI
The Internet of Things (IoT) generates massive volumes of data from networked sensors and edge devices. Applying artificial intelligence algorithms to this data enables extracting valuable insights. AI can detect usage patterns for personalization, predict failures for proactive maintenance, and secure systems by identifying anomalies. With increasing edge hardware capabilities, AI models can also run locally to enable real-time automation instead of relying solely on the cloud. Integrating these technologies amplifies the value of collected data.
How Does Artificial Intelligence of Things Work?
Traditional IoT networks transmit sensor data to the cloud for processing and analysis. AIoT architectures allow artificial intelligence algorithms to run directly on IoT devices and gateways at the network's edge. There are several key ways this edge-based approach works:
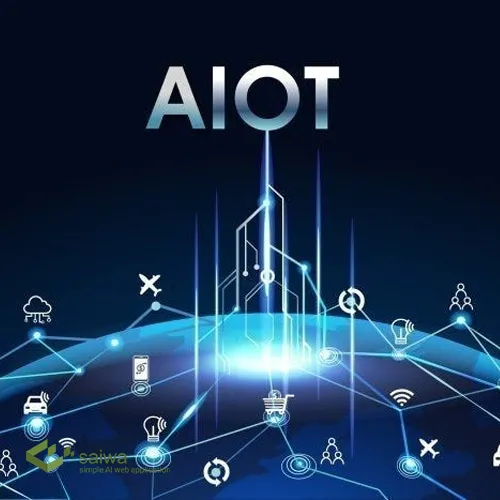
AI software like machine learning models is embedded into IoT devices and gateways, enabling them to process data and make decisions without constant cloud connectivity locally.
Devices preprocess and analyze data locally, only transmitting the most relevant data to the cloud, reducing bandwidth usage and costs.
Real-time localization of analysis close to sensors and endpoints allows for quicker responsiveness.
Edge-located processing enhances privacy and security since data is not transmitted to external cloud systems.
AI-enabled gateways aggregate and analyze data from multiple nearby devices before selectively sending insights to the cloud.
Hybrid systems take advantage of both edge AI for localized real-time needs and cloud AI for deeper analysis.
Virtual digital twins mirroring real-world systems can test AI optimization strategies by simulating IoT data and environmental conditions.
Closed loop actuators allow networks to take actions that then produce new data, enabling adaptive improvements of AI models.
Software-defined networking provides intelligent network automation based on AI analysis of network traffic patterns and demands.
Distributed intelligence divides processing between the cloud, gateways, and devices, with each level contributing analytics.
By embedding intelligence at the edge, AI IoT architectures allow for responsive and automated functionality while reducing costs, bandwidth usage, latency, privacy risks, and dependency on external cloud connectivity. The fusion of AI and IoT promises to make systems far more intelligent, autonomous, and efficient.
Edge-based AIoT
Edge-based AIoT refers to architectures where artificial intelligence processing occurs primarily on local embedded devices and gateways rather than relying on cloud computing resources. Key aspects of edge-based AI IoT:
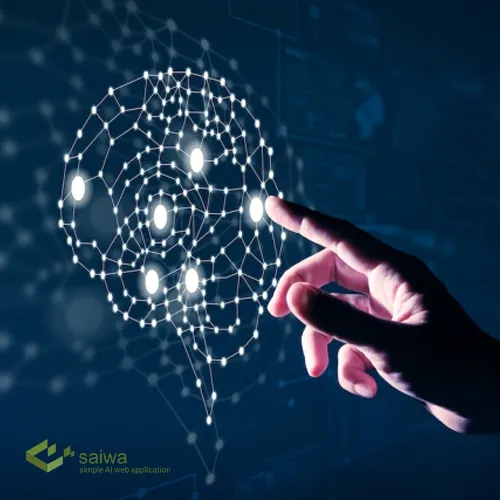
Machine learning models are trained in the cloud, then optimized and deployed to run on IoT gateways or end devices like sensors and robots. This reduces reliance on constant connectivity to the cloud by allowing onboard intelligence.
Real-time processing close to endpoints provides very low latency analytics and response.
Data preprocessing on the device reduces transmission costs and bandwidth usage by filtering out non-essential data.
Privacy and security are enhanced by avoiding sending all raw data to the cloud.
Autonomous operation is possible in remote locations with limited connectivity.
Smart gateways aggregate data from numerous nearby devices and run ML inference to produce insights.
AutoML techniques simplify deploying ML models directly into IoT applications and embedded firmware.
Specialized chips and neural network accelerators optimize the performance of on-device ML.
Digital twins can simulate locally to test AI strategies before real-world implementation.
Allows for closed-loop control flows, enabling local adaptive automation.
5G, WiFi 6, and mesh networks provide high bandwidth connectivity for dense edge networks.
Applications include self-driving vehicles, smart factories, automated oil rigs, and remote farms.
While edge Artificial Intelligence of Things removes cloud dependency, hybrid approaches take advantage of both cloud and edge capabilities. But running AI predominantly at the edge provides localization, speed, privacy, resilience, and autonomy.
AIoT&AI to Enhance IoT Capabilities
AI augments several facets of IoT systems:
Predictive Maintenance: Historical telemetry from appliance sensors trains AI models to forecast imminent failures based on early symptoms like vibration increases. This allows proactively servicing equipment before disruption.
Anomaly Detection: By analyzing expected normal sensor data patterns, machine learning models identify outliers indicative of potential cyber intrusions or device malfunctions. Rapid alerts facilitate incident response.
Personalization: Aggregating user behavior data allows AI agents to recognize habits and preferences to customize IoT services like smart home automation routines and notifications.
Cybersecurity: AIoT&AI algorithms help secure IoT devices which often lack native security features. Continuously updated attack pattern recognition defends against emerging threats.
Read Also: Artificial Intelligence in Smart Home | The Future Is Now
Applications and Examples of AIoT
Integrating AI into IoT networks and edge devices enables a broad range of intelligent applications across industries:
Manufacturing
Let’s review some of the manufacturing applications of AIoT:
Predictive maintenance: AI analyzes sensor data to forecast equipment failures and required repairs.
Quality control: Computer vision inspects products on assembly lines for defects.
Inventory management: AI tracks inventory levels and optimizes supply chains.
Intelligent robotics: Robots with embedded AI adapt to changing environments and tasks.
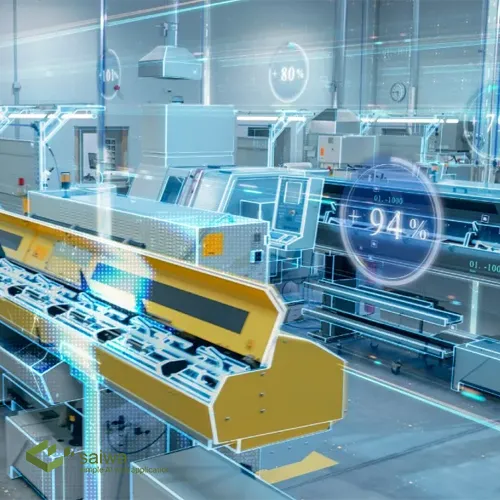
Transportation
Some Artificial Intelligence of Things examples in transportation are:
Self-driving cars: AI analyzes data from cameras and sensors for navigation and obstacle avoidance.
Traffic optimization: IoT devices collect traffic data for AI to optimize traffic light timing and routes.
Fleet management: AI optimizes delivery routes, vehicle usage, and cargo loading.
Agriculture
Agriculture is one of the key fields that need to become more optimized to reduce waste and labor.
Crop monitoring: Cameras and drones with computer vision track crop growth and health.
Soil optimization: AI analyzes moisture, nutrition, and environment data to improve yields.
Weather forecasting: Local weather data powers AI models to predict outcomes like rainfall.
Livestock tracking: IoT wearable tags and biometrics track animal health and behaviors.
Energy
Energy is another vital domain that furnishes the force required for every aspect of modern life, from illuminating our homes to fueling industries and advancing technology.
Renewables optimization: AI forecasts production and demand to efficiently balance the grid.
Predictive maintenance: Monitoring data from infrastructure equipment feeds AI to predict failures.
Usage forecasting: AI anticipates peaks and troughs in energy needs for grid stability.
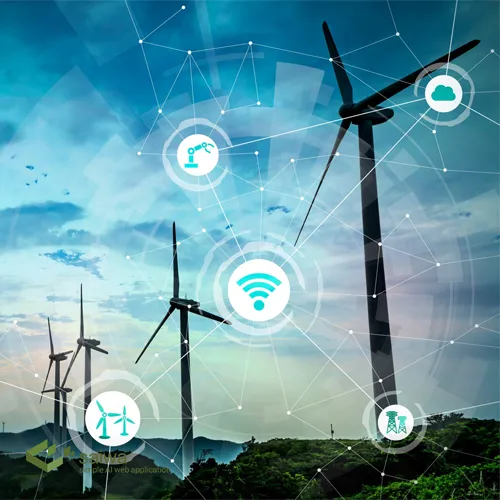
Retail
AIoT brings forth a revolution by enhancing customer experiences, optimizing inventory management, and facilitating personalized marketing strategies.
Automated checkout: Computer vision powers cashier-less stores by tracking products.
Personalized promotions: AI tailors promotions and recommendations based on customer data.
Demand forecasting: Predict expected sales volumes for inventory planning.
These examples highlight the transformative potential of infusing artificial intelligence into IoT ecosystems across every industry and domain. Intelligent edge devices and systems will reshape business operations, automation strategies, and personalized services.
Read More: IoT in Retail Industry | Examples & Use Cases
IoT to Empower AI Model Training
While generating useful data, IoT ecosystems also provide infrastructure for pragmatic AI training:
Distributed sensors allow aggregating expansive training data from numerous real-world environments. This data diversity improves model robustness and generalizability.
Continual data gathering enables online learning to adapt models after deployment. This maintains accuracy despite changing device usage patterns over product lifetimes.
Studying behavioral data at scale reveals macro trends and niche cases alike to enhance personalization. User privacy must be preserved with aggregation and other safeguards during analysis.
Testing model performance across the distributed deployment provides evidentiary validation before releasing updates. Canary deployments confirms improvements statistically.
What are the benefits and challenges of AIoT?
In this section, we look into how Artificial Intelligence of Things can make everyone's lives so much easier. It is obvious that this new technology comes from different challenges as well.
Benefits of AIoT:
Here are the benefits of applying Artificial Intelligence of Things in use.
Increased automation: AI enables systems to analyze data and take adaptive actions without human intervention automatically. This boosts efficiency.
Personalization: AI allows for customized experiences by tailoring system behavior and recommendations to individual users.
Enhanced predictive capabilities: AI techniques like predictive analytics and predictive maintenance enable proactive interventions.
Improved operational efficiency: AI can optimize workflows, uncover cost savings, reduce waste, and tighten supply chains.
New intelligence applications: AIoT expands the capabilities of IoT systems to include computer vision, voice interfaces, intelligent assistants, etc.
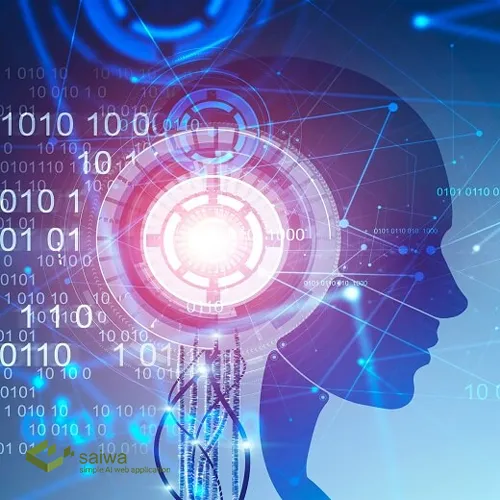
Challenges with AIoT:
The challenges of Artificial Intelligence of Things as a new tech are:
Data quality: Insufficient, incomplete, or biased data reduces the accuracy of AI algorithms and models.
Data scarcity: Lack of quality training data limits the development of AI models tailored for specialized applications.
Algorithmic bias: Models can inherit and amplify biases present in their training data.
Edge hardware constraints: Limited memory, power, and processing of edge devices restricts AI model complexity.
Security risks: Broader attack surfaces are introduced by extensive edge nodes and devices.
Lack of skills: Most organizations lack specialized skills to develop, deploy, and maintain complex Artificial Intelligence of Things systems.
High costs: Significant investments are required for hardware, network build-out, and software development.
Addressing these challenges through technological improvements and implementation of best practices will further accelerate the adoption of Artificial Intelligence of Things across industries.
What is the future of AIoT?
By integrating artificial intelligence, IoT creates the most intelligent system. The goal is for these systems to make accurate judgments without the need for human intervention. Digital transformation and the collaboration between artificial intelligence and the Internet of Things have the potential to exploit unknown customer values in several industrial sectors, including edge analytics, self-driving vehicles, personal fitness, remote healthcare, precision agriculture, retail It has intelligent sales, predictive maintenance and industrial automation.
Popular and emerging AIoT trends include:
Edge computing: This technology focuses on processing data close to its source rather than relying on centralized cloud servers, offering benefits such as reduced latency, increased efficiency, and reduced network congestion.
Collective intelligence: Collective intelligence includes the coordinated behavior of decentralized and self-organizing systems. Inspired by natural groups such as bees or ants, this technology can be used to optimize the performance of IoT devices.
5G technology: One of the biggest innovations in AIoT is the introduction of 5G. This feature is designed to enable faster transfer of large data files in IoT devices through higher bandwidth and lower latency.
Operational efficiency: AIoT can help solve existing operational problems, such as the costs associated with effective human capital management or the complexity of supply chains and delivery models.
Computer Vision: The goal of computer vision is for machines to understand and interpret visual information collected from the real environment. This capability can analyze video streams from cameras, detect objects, and identify anomalies in AIoT applications, enabling real-time automation, monitoring, and optimization. Computer vision is revolutionizing the industrial sector by enabling companies to improve operational efficiency, put in place quality control procedures, improve preventive maintenance practices and prioritize worker safety measures.
Conclusion
The integration of artificial intelligence into IoT networks through edge devices and hybrid cloud architectures promises to transform future smart systems by enabling intelligent automation, dynamic optimization, predictive insights, and data-driven decision-making. Realizing the full benefits will require careful attention to implementing best practices, evolving supporting technologies, and ensuring ethical application. Multidisciplinary collaboration, combining AI software with IoT systems engineering, will drive innovation in applying AIoT to solve complex problems in nearly every area of life and work.