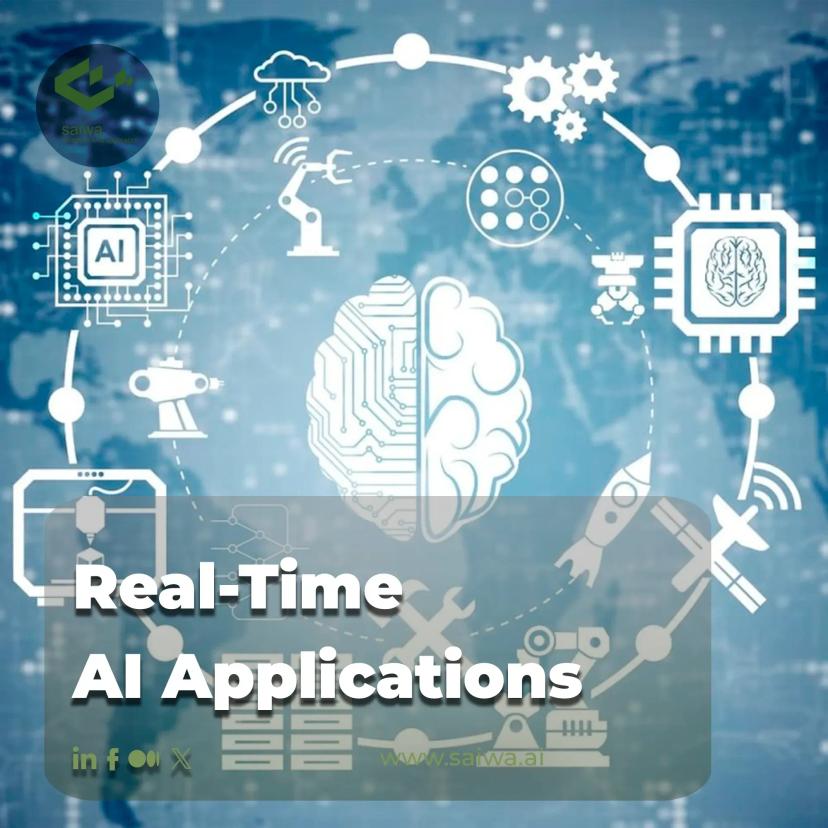
The Rise of Real-Time AI Applications
Artificial intelligence is moving beyond traditional big data batch processing to real-time, ultra-low-latency intelligent systems. As computing power grows exponentially, AI models can now ingest and analyze continuous data streams in milliseconds or less. This enables responsive applications from self-driving cars to algorithmic trading.
Real-time AI applications offer tangible benefits across industries but present new technical, ethical, and security challenges compared to offline modeling. In this article, we provide an overview of real-time AI approaches, impactful use cases, and a look at future opportunities and risks.
Core Technical Approaches
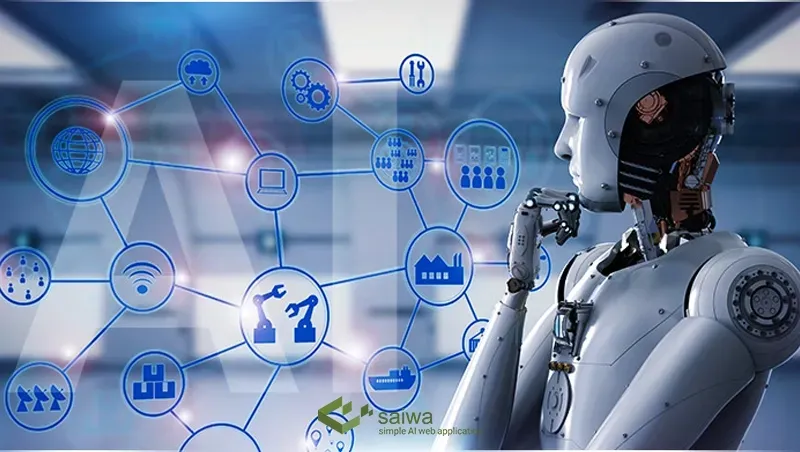
Achieving real-time AI requires optimized model architectures, specialized hardware, and efficient frameworks:
Lightweight neural networks trade some accuracy for much faster inference times, which is critical for applications like video analysis or augmented reality.
Model compression techniques like quantization and pruning reduce computational requirements without heavily impacting performance.
Edge computing distributes processing to local devices rather than the cloud, avoiding network latency. Smart sensors can run inference at the data source.
Some companies are developing dedicated AI chips delivering order-of-magnitude speedups in neural network training and inference.
Frameworks like TensorFlow Lite and PyTorch Mobile enable fast yet flexible deployment of models to edge devices with limited resources.
These items help address the dual challenges of maximizing prediction speed while minimizing latency in real-time AI applications.
Read Also: Best AI Tools for Developers in 2024
Real-Time AI Applications Use Cases
Real-time AI applications unlock transformative applications across domains:
AI in Pathology
The first real-time AI application we reviewed is the application of AI in pathology. The field of digital pathology is being transformed by artificial intelligence. AI-powered image analysis systems can quickly scan tissue slides and cell morphology in real time to classify cancer subtypes, detect abnormalities, and identify regions of concern for pathologists to review.
Companies are offering clinical-grade deep learning tools that can diagnose breast cancer metastases and prostate cancer in seconds based on digitized pathology slides. This is made possible by convolutional neural networks optimized specifically for cellular images, which can run in real-time thanks to model compression techniques. AI-based diagnostics enable faster, higher throughput screening and more consistent cancer diagnosis.
However, human expertise remains essential for final review and monitoring. Key challenges in deploying these systems include curating robust labeled training data across diverse patient populations, quantifying model uncertainty, and integrating with standard clinical laboratory workflows. However, the future potential to augment pathologists and improve patient outcomes through real-time AI pathology analysis is immense.
Read More: The Transformative Impact of AI in Pathology and Patient Care
AI in Self-Driving Cars
Safe autonomous vehicle navigation relies heavily on real-time sensor fusion and environmental perception algorithms. Ultra-low latency object detection, semantic segmentation, depth estimation, and prediction are required to understand dynamic surroundings and navigate at high speeds. Companies like Waymo, Cruise, and Pony.ai use specialized onboard chips to run optimized deep learning models like YOLOv3 that can identify vehicles, pedestrians, traffic signs, and lane markings within milliseconds based on continuous camera, LIDAR, RADAR, sonar, and GPS inputs.
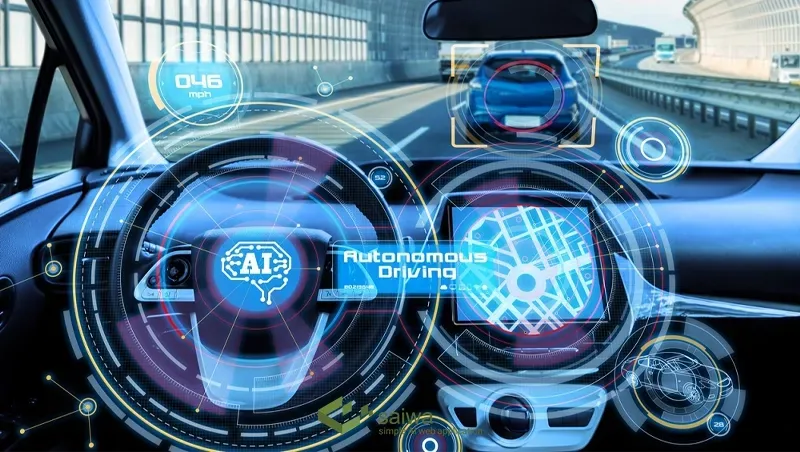
This enables self-driving cars to interpret complex urban scenes like crowded pedestrian zones or construction sites on the fly. Software development kits enable tuning deep neural networks specifically for embeddable automotive deployment. Key challenges include handling corner cases outside of training distributions, building redundancy for safety, and establishing public trust in autonomous real-time decision-making. But refined real-time AI perception lays the algorithmic foundation for scalable autonomous transportation.
Read more: Find the Impact of AI in Self Driving Cars with our guide
AI in Ecology
Ecologists are pioneering the use of real-time AI applications and techniques deployed on drones, camera traps, and remote sensors to monitor ecosystems and wildlife continuously. Embedded deep learning algorithms can detect poachers, track species movement, model population health, and survey habitat threats with minimal latency.
This allows conservation teams to respond rapidly to emergencies like injured animals, illegal activity, fires, or sudden weather shifts. Microsoft Research, WWF, and conservation technology startups are exploring these real-time AI approaches to boost ecosystem resilience.
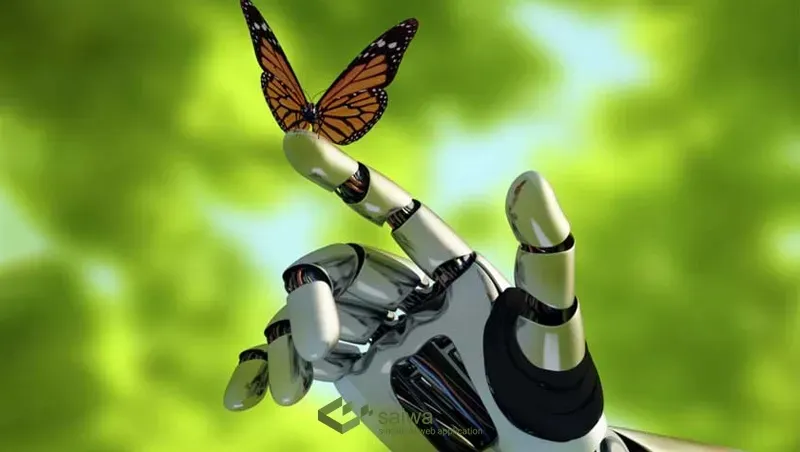
Processing data streams onboard avoids reliance on connectivity in remote field areas and reduce transmission needs by filtering only salient events. However, model robustness across geographic and seasonal variation, appropriate confidence calibration, and long-term maintenance of semi-autonomous systems remain challenges to address. Overall, the future is bright for real-time AI to help conserve biodiversity if developed responsibly and in collaboration with conservation domain experts.
Read more: AI in Ecology | Expanding Ecology’s Vision with AI
AI in Agriculture
Precision agriculture seeks to provide crops with customized care and resources tailored to local conditions. Real-time computer vision analysis on imagery feeds from aerial drones, tractors, and stationary cameras allows monitoring of crop health variability within fields. Embedded deep neural networks enable low-latency disease and pest detection, triggering targeted alerts for preventative treatment instead of delayed reactions once the damage is visible.
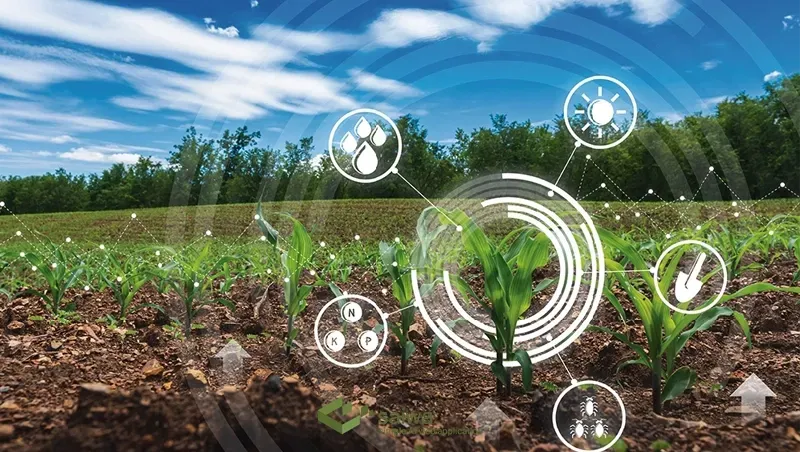
Some companies are commercializing such technologies, combining multiple visual indicators over time, including leaf discoloration, spots, and terrain changes to improve alert accuracy. This finer-grained temporally rich perspective empowers more timely interventions like selective spraying or harvests. However, challenges remain in handling environmental dynamism, explainability, and economically scaling real-time precision agriculture to meet global food security needs. Further, multidisciplinary collaboration between agronomists and AI experts will be key to overcoming these hurdles.
Read More: AI in Agriculture | Practical Application & Future of AI in Agriculture
AI in Sports
Sports analytics are being transformed by real-time computer vision AI systems. Very low latency is critical to render animations, stats, and predictions for broadcasting and maintaining game flow. Data streams are processed instantly using techniques like Kalman filters to smooth trajectories and neural networks to classify granular player actions. Some other companies are commercializing these capabilities across many professional leagues and sports.
Key bottlenecks remain in camera resolution, computation power, and balancing model accuracy alongside speed. As adoption expands, regulatory debates continue around maintaining competitive fairness and integrity, given the power of automated analytics. However, refined real-time sports AI seems poised to become an increasingly ubiquitous part of both competitive play and fan experiences.
Read More: Practical applications of AI in Sports that you need to know
AI in Healthcare
In the world of various real-time AI applications, wearable medical sensors integrated with edge AI allow real-time monitoring of vital signs, falls, tremors, and health emergencies, alerting caregivers of symptoms early. If you are interested in some more information about AI in healthcare, you can click here.
AI in Finance
High-frequency trading firms apply real-time AI to massive data streams to exploit fleeting arbitrage opportunities in microseconds. Latency reduction is critical, and driving the adoption of specialized prediction chips is used to maximize speed advantages.
Read More: Best Machine Learning Applications in Finance
AI in Security
Video analytics for real-time perimeter monitoring, intruder detection, and remote surveillance rely on efficient deep-learning pipelines optimized to run on edge devices with minimal network latency.
Read More: What is AI in security, and How does it work?
AI in Fraud Detection
Financial transactions occur in milliseconds, demanding real-time fraud analysis. AI systems ingest account activity data streams to spot anomalous usage patterns and block illegal transactions before they are completed.
Plant Counting Using AI
Real-time crop monitoring drones fused with computer vision algorithms can enumerate and map plants across fields. Low-latency segmentation and object detection enable precise plant counts to inform agricultural decisions without delays of manual surveys. Startups like Hummingbird are commercializing such technologies.
Read More: The Surprising Value of Plant Counting
AI for Space Exploration
AI for Space Exploration is transforming missions through autonomous rover navigation, real-time data analysis for exoplanet detection, and spacecraft health monitoring. It enables independent and efficient operations, overcoming communication delays and enhancing scientific discovery, despite challenges in harsh space environments.
Conclusion
Real-time AI applications enable intelligent systems that interact with and respond to the world dynamically. However, developers must consider if split-second autonomous decisions are always appropriate or if some oversight should be maintained. Real-time AI Applications could lead to more nimble organizations, smarter cities, and preventative medicine if responsibly leveraged responsibly. The path forward lies in sustained technology innovation combined with continuous societal engagement.