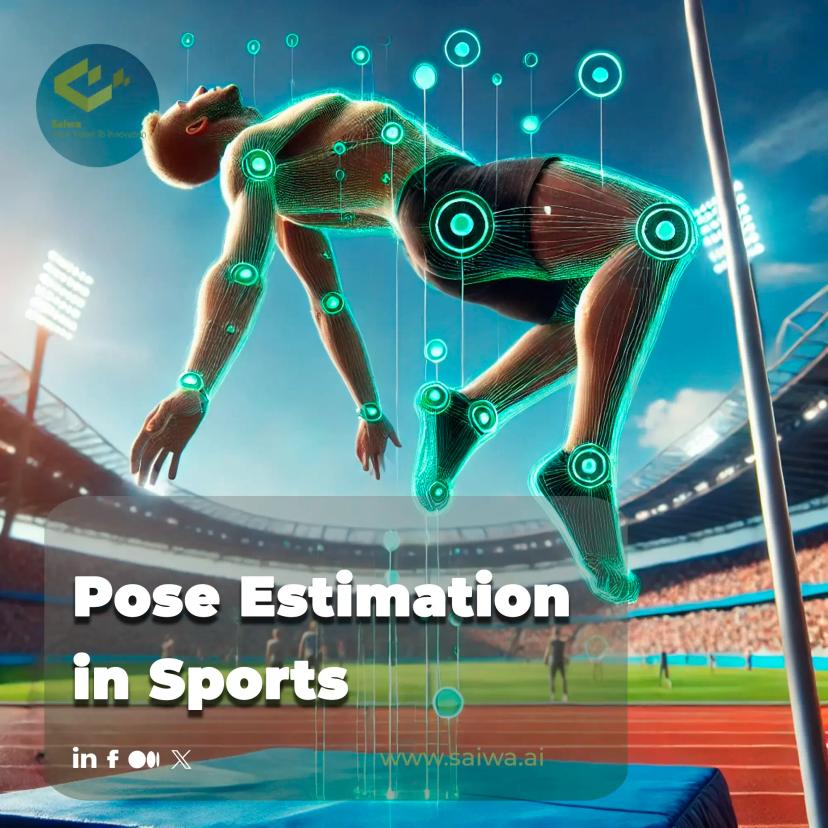
Pose Estimation in Sports | Enhancing Performance
Human pose estimation is revolutionizing the sports industry by providing an accurate digital representation of athletes in action. This innovative computer vision technique accurately maps key body joints, creating a virtual "skeleton" reflecting the biomechanical dynamics from images or video.
Identifying critical points such as elbows and knees provides a detailed analysis of posture and movement. Its versatility spans multiple fields and enhances sports by turning subjective observations into quantifiable data.
This technology optimizes athletic performance and training programs, plays a critical role in injury prevention, and advances our understanding of human biomechanics for peak performance.
Saiwa, an AI company, offers a range of solutions through its products, Saiwa's product "Fraime" provides a comprehensive pose estimation service using advanced AI for accurate human motion analysis in sports, healthcare, and more.
This service utilizes both bottom-up and top-down methods, detecting individual key points to analyze specific poses, making it ideal for various environments. In sports, the Fraime Pose Estimation service enhances training, improves performance and prevents injuries by providing in-depth insight into the body dynamics of athletes. By accurately capturing and analyzing motion, Fraime ensures customized and precise support, revolutionizing the way athletic performance is monitored and optimized.
This article examines the extensive capabilities of pose estimation technology within the sporting context, as exemplified by Saiwa's Fraime. It considers how athletes and coaches use this advanced tool to enhance training techniques, optimize performance, and significantly reduce the risk of injuries.
Furthermore, it assesses the broader impacts of this technology on sports science, demonstrating how data-driven insights are reshaping our understanding of human motion and athletic potential.
How Pose Estimation Works
Pose estimation algorithms typically employ deep learning models, particularly convolutional neural networks (CNNs), to analyze images or videos and identify key body joints. These models are trained on vast datasets of annotated images, learning to recognize patterns and relationships between different body parts. The process can be broadly categorized into two main approaches:
Top-Down Approach
This approach first detects individuals within the image or video using object detection techniques, typically also based on deep learning models. Once individuals are localized, a separate pose estimation model is applied to each detected person to estimate the location of their body joints. It's particularly effective in scenarios with multiple individuals or complex backgrounds, as it isolates each person before performing pose estimation. However, this two-stage process can be computationally more expensive than the bottom-up approach.
Bottom-Up Approach
This approach first detects all body parts (e.g., elbows, knees, wrists) in the image or video independently. After detecting all potential body parts, the algorithm then uses association techniques, often involving graph-based methods, to group these parts together and associate them with individual people.
It can be more efficient than the top-down approach, especially in crowded scenes, as it avoids the individual person detection step. However, it can be more challenging to accurately associate body parts in cases of significant overlap or occlusion.
Once the body joints are identified, the algorithm connects them to create a skeletal representation of the pose. This skeletal representation can then be used for various applications, such as motion capture, activity recognition, and biomechanical analysis. The accuracy and robustness of pose estimation algorithms are constantly improving, driven by advancements in deep learning and the availability of larger and more diverse datasets.
Technological Components in Sports Pose Estimation
Several key technologies contribute to the effectiveness of pose estimation in sports:
Spatiotemporal Transformers
Spatiotemporal transformers are advanced deep learning models designed to process both spatial and temporal information simultaneously. This is crucial for analyzing video sequences of athletic performance, as it allows the model to understand not only the position of body joints in each frame but also how those positions change over time.
By capturing these dynamic relationships between body joints, spatiotemporal transformers provide a more comprehensive understanding of movement patterns and biomechanics. Their ability to attend to both spatial and temporal features allows them to capture long-range dependencies and contextual information, leading to improved accuracy in pose estimation, especially in complex and dynamic movements common in sports.
Temporal Convolutional Networks (TCN)
Temporal Convolutional Networks (TCNs) are specialized neural networks specifically designed to process sequential data, making them well-suited for analyzing time-series data like the sequence of frames in a sports video. TCNs excel at capturing temporal dependencies and patterns in movement, allowing them to accurately track the trajectory of body joints over time and understand how movement unfolds.
This is crucial for understanding the dynamics of athletic movements and identifying subtle variations in technique that might be missed by analyzing individual frames in isolation. Their ability to handle long sequences of data makes them particularly effective in analyzing extended sports actions and identifying key phases within a performance, such as the different stages of a golf swing or a swimming stroke.
Graph Convolutional Networks (GCN)
Graph Convolutional Networks (GCNs) offer a unique approach to pose estimation by representing the human body as a graph. In this representation, the body joints are treated as nodes in the graph, and the bones connecting the joints are represented as edges. This structure allows GCNs to effectively capture the relationships and dependencies between different body parts, reflecting the interconnected nature of human movement.
In sports pose estimation, GCNs can leverage this graph representation to learn complex interactions between joints and improve the accuracy of pose predictions, especially in situations where some joints might be occluded or difficult to detect. They are particularly effective in handling variations in body shape and size, as the graph structure provides a flexible and adaptable representation of the human skeleton detection .
The Benefits of Human Pose Estimation for Sports
The application of human pose estimation in sports offers numerous benefits:
Objective Performance Analysis
Pose estimation provides a powerful tool for objective performance analysis by capturing quantitative data on an athlete's movement. This data includes precise measurements of joint angles, velocities, and accelerations, providing a detailed and granular view of biomechanics.
This objective analysis goes beyond subjective visual assessments, offering concrete metrics to identify strengths and weaknesses in technique. By tracking these metrics over time, coaches and athletes can monitor progress, evaluate the effectiveness of training interventions, and compare performance against benchmarks or other athletes, fostering data-driven decision-making in training and competition.
Personalized Training Programs
Pose estimation enables the creation of truly personalized training programs tailored to the unique needs and biomechanics of individual athletes. By analyzing pose data, coaches can gain insights into an athlete's specific movement patterns, identifying areas for improvement and potential biomechanical deficiencies.
This information informs the design of targeted exercises and drills that address specific muscle groups, movement patterns, or weaknesses. This individualized approach maximizes training efficiency and effectiveness, optimizing performance gains by focusing on areas where the athlete needs the most attention.
Remote Coaching and Monitoring
Pose estimation facilitates remote coaching and monitoring, bridging the geographical gap between athletes and coaches. Athletes can record their training sessions, and the captured pose data can be shared with coaches for remote analysis and feedback.
This allows coaches to provide guidance and support even when they are not physically present, expanding access to expert coaching for athletes in remote locations or those with limited resources. This remote monitoring capability democratizes access to high-quality coaching and fosters ongoing communication between athletes and coaches.
Automated Performance Assessment
Pose estimation enables automated assessment of athletic performance, streamlining the evaluation process and reducing reliance on manual analysis and subjective judgments. Automated systems can process pose data from videos, extracting key performance metrics and providing objective evaluations.
This automation not only saves time and resources but also ensures consistency and objectivity in performance assessments. Furthermore, automated assessment facilitates large-scale performance analysis, such as evaluating athletes in talent identification programs or conducting population-level studies of athletic biomechanics.
Applications of Human Pose Estimation in Sports
Performance Analysis: Quantifying Athletic Movement
Pose estimation provides a powerful tool for analyzing athletic performance across a wide range of sports. By tracking the movement of body joints, coaches and athletes can gain detailed insights into technique, biomechanics, and efficiency of movement.
This data can be used to identify areas for improvement, optimize training strategies, and gain a competitive edge. For example, in golf, pose estimation can analyze the swing mechanics, identifying subtle flaws that may be impacting performance. In swimming, it can track the body position and stroke efficiency, helping swimmers refine their technique and reduce drag.
Injury Prevention: Identifying Risk Factors
Pose estimation can play a crucial role in injury prevention by identifying biomechanical risk factors and movement patterns that may predispose athletes to injury. By analyzing the movement of joints and limbs, the technology can detect imbalances, asymmetries, and improper loading patterns that may increase the risk of strains, sprains, and other injuries.
This information can be used to develop preventative exercises and modify training programs to reduce the likelihood of injury. For example, in running, pose estimation can analyze gait patterns and identify potential risk factors for knee injuries.
Training Optimization: Tailoring Programs for Peak Performance
Pose estimation allows for the development of personalized training programs tailored to the specific needs and goals of individual athletes. By analyzing an athlete's movement patterns and identifying areas for improvement, coaches can design targeted exercises and drills that address specific weaknesses and optimize performance.
This data-driven approach to training can lead to more efficient and effective training sessions, maximizing the athlete's potential. For instance, in basketball, pose estimation can analyze shooting techniques and provide feedback on shot mechanics, helping players improve their accuracy and consistency.
The Future of Human Pose Estimation in Sports
The future of pose estimation in sports is bright, with ongoing advancements in deep learning and computer vision promising even more sophisticated and powerful applications.
Real-time pose estimation, integrated with wearable sensors and virtual reality technologies, will enable immersive training experiences and personalized feedback. The development of more robust and accurate algorithms will further enhance the ability to analyze complex movements and provide deeper insights into athletic performance.
We can expect to see wider adoption of pose estimation technology across various sports, from amateur to professional levels, transforming the way athletes train, compete, and recover.
Conclusion
Human pose estimation is revolutionizing sports, providing an unprecedented ability to analyze and enhance athletic performance. By accurately capturing and interpreting human movement, this technology empowers athletes, coaches, and scientists with objective data and actionable insights.
From refining techniques and optimizing training to preventing injuries and automating assessments, pose estimation offers a powerful toolkit for achieving peak performance. With continued advancements in deep learning and computer vision, the future holds even greater potential for personalized training, real-time feedback, and a deeper understanding of the complexities of human motion in sports. From amateur to professional levels, pose estimation is transforming how we train, compete, and interact with the world of sports.