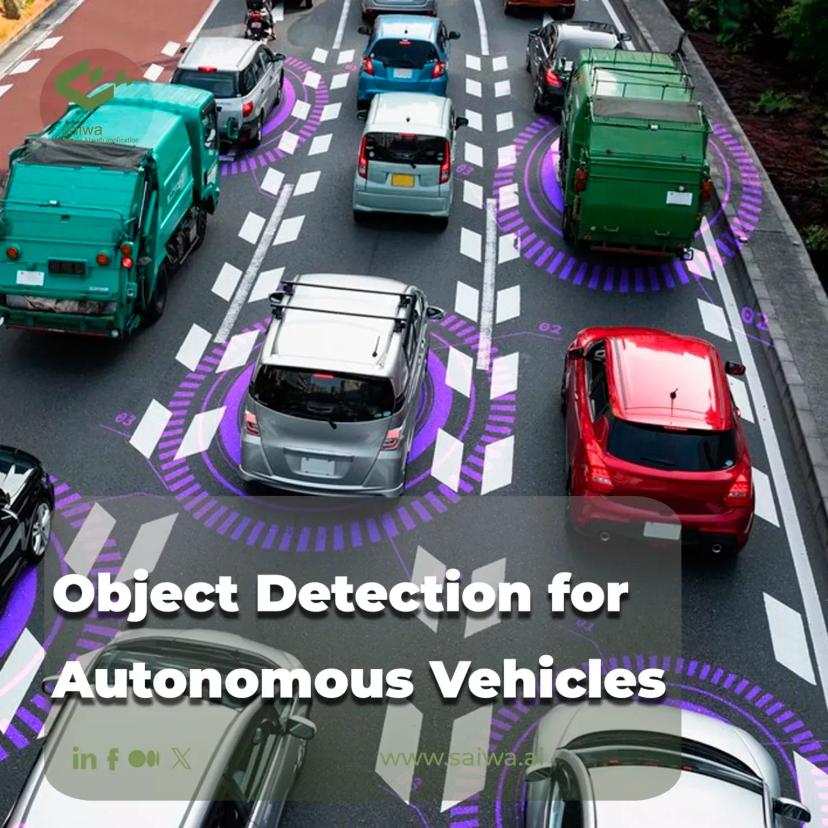
The Role of Object Detection for Autonomous Vehicles
With the emergence of AI in Self Driving Cars in recent years, a major shift has occurred in the automotive industry. At the heart of this transformation is Object detection. Object detection is an important part of computer vision that enables self-driving vehicles to understand and adapt to their environment. In this article, we will talk about Object detection for autonomous vehicles. There are several key elements in this area that we will discuss in detail.
What is object detection?
Object detection is the process of locating and identifying objects in images or videos, involving two main tasks: finding regions of interest that contain objects and assigning a label or class based on appearance and context. Various methods, such as deep learning, computer vision, and sensor fusion, are utilized in object detection to achieve high accuracy and speed. Consistent usage of metrics and units, unambiguous language, and precise word choice are crucial for effective object detection.
Read Also: Exploring The Real-World Applications of Object Detection
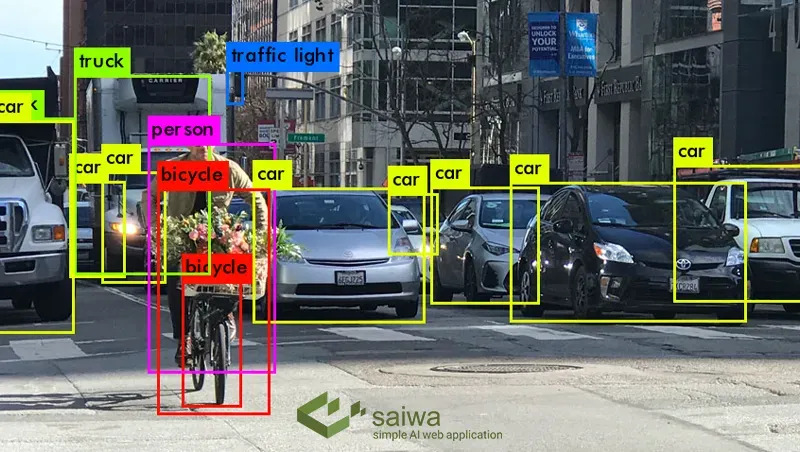
Why is object detection important for self-driving vehicles?
Autonomous vehicles rely on object detection to navigate safely and effectively in complex and dynamic environments. Object detection enables self-driving vehicles to detect and track other vehicles, pedestrians, cyclists, traffic signs, lines, and obstacles.
By recognizing objects and their characteristics such as size, shape, color, distance, and speed, self-driving vehicles can plan their route, avoid collisions with objects, and obey traffic laws.
Technical aspects of autonomous vehicles
Self-driving vehicles rely on various sensors such as cameras, radar, and ultrasonic sensors to detect objects in their environment, and these sensors work together to create a 360-degree view of the vehicle's surroundings, which is then processed by object detection software. In order for vehicles to detect objects in their environment, engineers must pay attention to the selection of the necessary controllers and sensors.
Another important technical aspect of object detection for autonomous vehicles is the algorithm used for object detection. Various algorithms are used, including deep learning algorithms such as convolutional neural networks and recurrent neural networks. These algorithms are trained on large datasets of labeled images to learn how to identify, recognize, and classify objects in different environments.
Neural Networks in Object Detection
Neural networks play the most important role in adaptive Object detection for autonomous vehicles. Neural networks are a machine learning algorithm for Object detection that is inspired by the human brain to recognize patterns in visual and audio data. These networks consist of interconnected nodes that work together to process and analyze data.
In autonomous vehicles, neural networks analyze data collected by vehicle sensors and identify objects in the environment. Feature extraction identifies the key features of sensor data that are relevant to detecting the presence of an object in a given environment. These features include edges, corners, and observed changes that can be extracted by the camera during visual interpretation of the frame.
Classification is the final stage in the process of Object detection, a stage where the trained algorithm compares the features of the desired area using a neural network classifier and outputs the output into different recognizable classes such as cars, and pedestrians. It divides pedestrians and road signs.
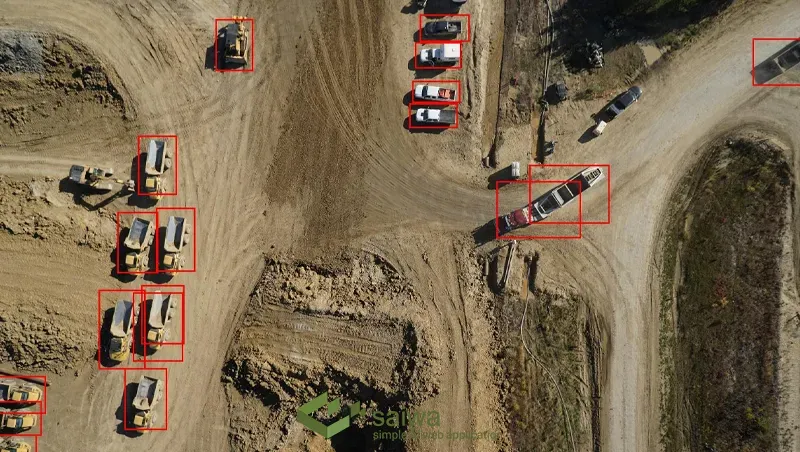
Object detection for autonomous vehicles:
In this section, we will discuss the key elements of this topic:
Trade-off speed and accuracy
Achieving a balance between speed and accuracy for real-time object detection is important in autonomous driving scenarios. Deep learning algorithms should be optimized for efficiency without sacrificing accuracy. Innovations in model architectures and optimizations in inference processes help to minimize latency and ensure reliable object detection.
Recognition of traffic signs
Accurate traffic sign recognition is very important for the safe operation of self-driving vehicles. Deep learning models, especially convolutional neural networks, are excellent at decoding complex visual information embedded in various traffic signs.
This skill gives these models the ability to accurately identify and interpret traffic signals and act as an important component for autonomous systems' informed decision-making processes.
This capability ensures a high level of safety and compliance with traffic regulations, which is necessary to successfully integrate autonomous vehicles into diverse and dynamic road environments.
Fusion technology and sensor radar
Object detection for autonomous vehicles is more than visual data. Radar sensors contribute to the capabilities of self-driving cars by providing additional information about their surroundings.
The combination of sensors that combine the data of cameras and radars provides a more comprehensive and complete awareness of the environment and leads to increased safety and reliability.
Vehicle and pedestrian detection
Ensuring the safety of pedestrians and identifying vehicles on the roads is one of the important and necessary priorities for self-driving vehicles. Deep learning models are better at accurately identifying pedestrians and vehicles using diverse datasets.
This skill gives autonomous systems the ability to navigate complex urban environments with greater precision and increase overall safety. Using the power of neural networks and comprehensive training data, these models enable fast and reliable identification of dynamic elements in real-time and create a strong foundation for the seamless integration of autonomous vehicles in the modern transportation landscape.
Obstacle detection and safety of autonomous vehicles
Detecting obstacles in real time is necessary to ensure the safety of vehicles and their passengers. Deep learning algorithms are excellent at recognizing various obstacles, including stationary objects and dynamic elements, which prompt adaptive responses to ever-changing road conditions.
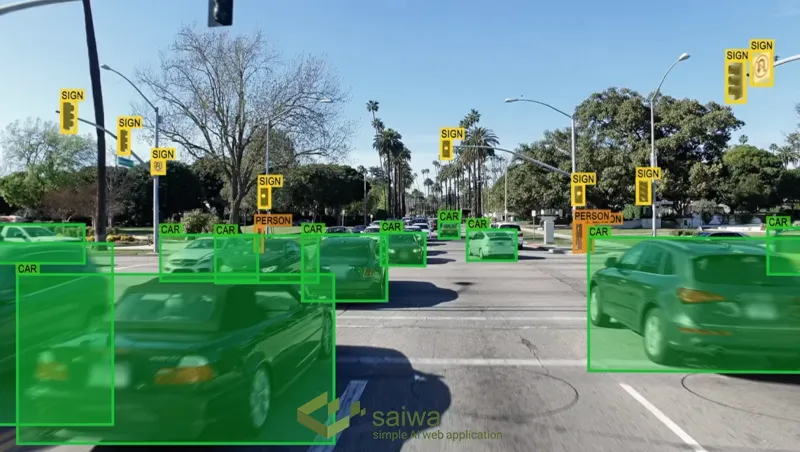
Self-Driving Hybrid Cars
Combining self-driving technology with hybrid vehicles is an important step toward sustainable and effective transportation. Object detection technologies play an important role in improving the performance of self-driving hybrid vehicles and ultimately reduce fuel consumption and greenhouse gas emissions.
By seamlessly detecting and responding to the dynamic environment, these technologies optimize energy consumption and promote environmentally friendly driving habits.
In addition to enhancing the driving experience, this synergy between self-driving capabilities and hybrid performance underscores the important link between advanced technology and environmental responsibility, creating a more sustainable future for the automotive industry.
Read Also: Benefits and Challenges of Ai-Powered Greenhouses
What are the challenges of Object detection for autonomous vehicles?
Object detection for autonomous vehicles is a complex task because it involves several challenges such as variety, size, complexity, diversity, and uncertainty. Objects can vary in appearance, posture, orientation, brightness, occlusion, and background. They also have different sizes and distances from the camera, which affects their clarity and visibility. In addition, objects can have multiple parts, shapes, and textures, making them difficult to recognize and classify. Objects can belong to different categories, subsets, and examples, which require large and diverse datasets for training and testing. Finally, objects can have ambiguous or crowded labels, which affect the reliability and accuracy of detection.
Conclusion
Object detection is crucial for self-driving cars to comprehend their environment and drive safely. Although advancements in technology have greatly improved object detection in terms of speed and accuracy, challenges still exist. Continuous research is being conducted in this field, and advancements have led to the actualization of self-driving cars compared to previous years. It is expected that we will reach the most ideal conditions in the near future.