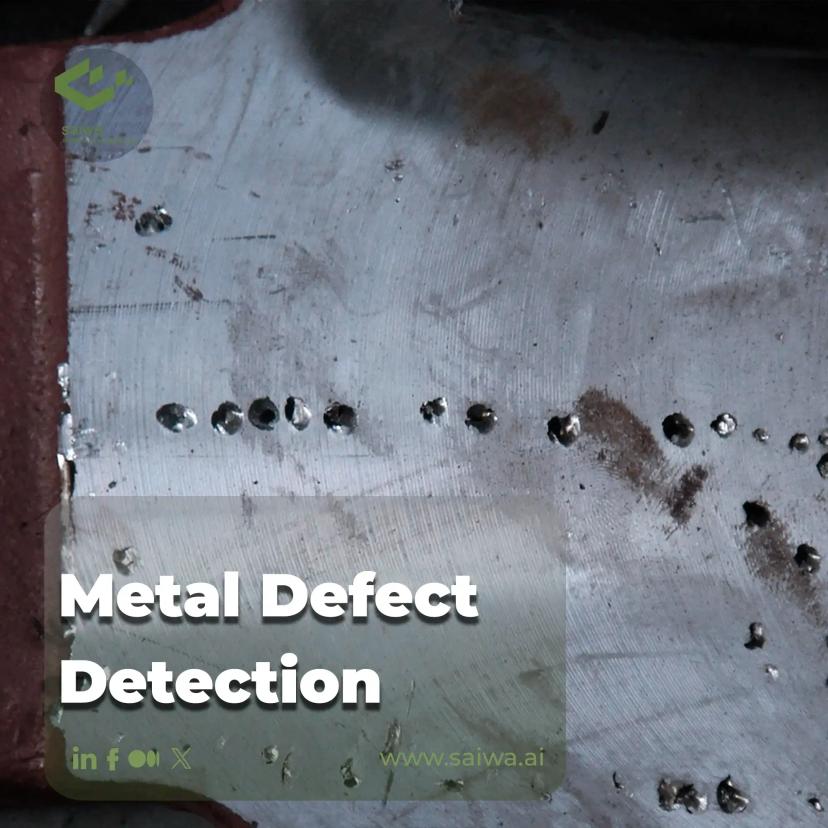
Metal Defect Detection | Enhancing Precision and Efficiency with AI
Metal flaw detection is critical to quality assurance in manufacturing and industrial processes. Even small defects in metal components can have catastrophic consequences, making employing reliable and efficient defect detection methods imperative. While effective, traditional inspection techniques often suffer from limitations such as labour-intensive and time-consuming processes. However, with the advent of artificial intelligence (AI) and deep learning, the metal defect detection landscape has undergone a transformative change. In this blog post, we will explore the different types of metal defects, traditional inspection methods, and the significant role of AI in revolutionizing defect detection. In addition, we will delve into popular deep learning models used in metal defect detection and address the challenges and applications of this cutting-edge technology.
Read Also: AI inspection for precision aluminum defect detection
Types of Metal Defects
Metals production encompasses various manufacturing steps, including casting, forming, machining, welding, and finishing. Throughout these complex processes, various defects can occur due to issues in raw materials, equipment wear, process parameter misconfigurations, or environmental factors. Typical metal surface anomalies include:
Cracks and Fractures: These are discontinuities in the metal structure that can significantly impact mechanical properties.
Inclusions: Inclusions are non-metallic particles or impurities embedded in the metal during manufacturing.
Porosity: Porosity refers to the presence of voids or air pockets in the metal, which can weaken the material and affect its integrity.
Weld Defects: Weld defects occur during welding and can include issues like incomplete fusion, porosity, and undercuts.
Surface Irregularities: Surface irregularities encompass scratches, pits, and dents that affect the appearance and functionality of the metal surface.
Even microscopic defects like these can initiate cracks or component failures. Therefore, reliable defect detection is imperative for metals where high integrity is required.
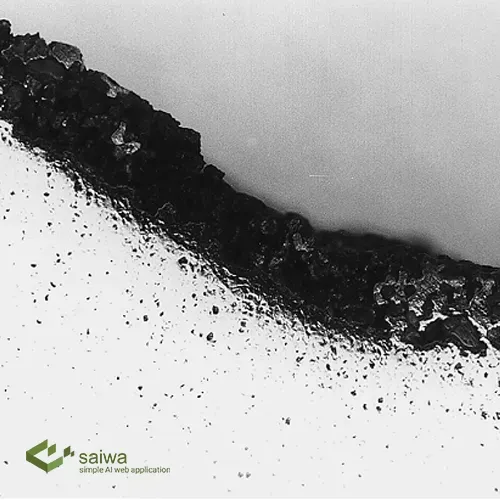
Traditional Methods of Metal Defect Detection
Before the emergence of AI, manufacturers relied on traditional inspection techniques to identify metal defects. Some of the common methods include:
Visual Inspection: Skilled inspectors visually examine metal components for any visible defects. While this method is subjective and dependent on human expertise, it remains widely used for preliminary defect identification.
Magnetic Particle Inspection: This technique involves applying magnetic particles to the metal surface, revealing defects through magnetic field changes.
Ultrasonic Testing: Ultrasonic waves are transmitted through the metal, and the reflected waves are analyzed to detect defects beneath the surface.
X-ray Inspection: X-ray imaging can reveal internal defects in metal components, offering a non-destructive evaluation method.
While these methods have been effective, they have limitations regarding speed, scalability, and sensitivity to small defects. AI-powered defect detection promises to overcome these limitations and usher in a new era of precision and efficiency.
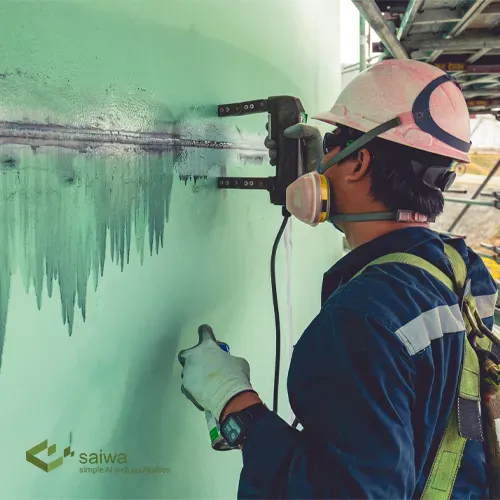
AI Role in Metal Defect Detection
The integration of AI-powered defect detection systems offers numerous advantages that significantly enhance the efficiency, accuracy, and cost-effectiveness of the defect detection process.
Let’s delve deeper into the key aspects of AI's role in metal defect detection:
Automation and Speed
One of the primary advantages of AI in defect detection is automation. AI-powered systems can rapidly process vast amounts of data and images, reducing the need for labor-intensive manual inspections. This automation saves time and enables manufacturers to achieve faster inspection rates, enhancing overall productivity and efficiency in the manufacturing process.
High Accuracy and Precision
AI algorithms, particularly Deep Learning models, are capable of detecting even minute defects with a high degree of accuracy. These models can learn from vast datasets of labeled images, allowing them to discern subtle patterns and anomalies that might be challenging for human inspectors to identify. The result is a defect detection process that is more reliable and less prone to human error.
Real-Time Monitoring and Quality Control
AI-powered defect detection systems enable real-time monitoring of the manufacturing process. As metal components are produced, they can be inspected in real-time, allowing for immediate action in case of defects. This real-time monitoring ensures that faulty products are detected early, reducing waste and preventing defective items from reaching the market.
Adaptability and Scalability
AI models are highly adaptable and can be trained to detect various types of defects in different metal components. As new defect types are discovered or manufacturing processes evolve, the AI models can be retrained to accommodate these changes. This adaptability makes AI-powered defect detection systems versatile and suitable for a wide range of applications in different industries.
Continuous Learning and Improvement
AI models can continuously learn from new data, improving their defect detection capabilities over time. As more labeled images become available, the AI model becomes more refined and accurate, providing manufacturers with ongoing enhancements in defect detection performance.
Defect Localization and Segmentation
Deep Learning models, such as U-Net, excel in defect localization and segmentation. They can precisely identify the boundaries of defects within metal components, allowing for accurate defect characterization. This level of detail is essential in understanding the nature and severity of defects, enabling manufacturers to take appropriate corrective measures.
Reduced False Positives and Negatives
Traditional defect detection methods may suffer from false positives (identifying non-defective components as defective) or false negatives (failing to detect defects). AI-powered models can significantly reduce these errors, leading to more reliable defect detection results and minimizing unnecessary rejections or faulty products.
Cost-Effectiveness
While implementing AI-powered defect detection systems may require an initial investment, the long-term cost savings are substantial. By reducing manual labor, minimizing defective products, and optimizing production processes, manufacturers can achieve significant cost-effectiveness and better utilize resources.
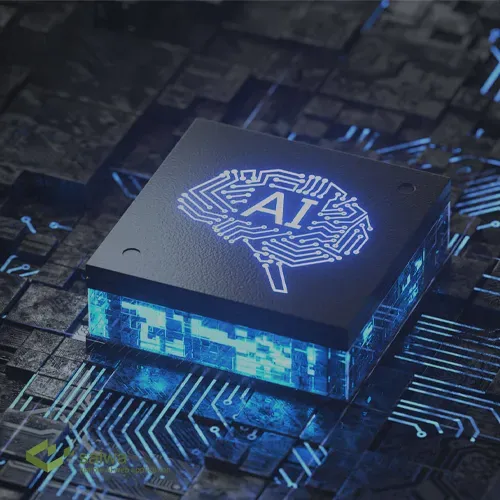
Deep Learning Models for Metal Defect Detection
Deep Learning models have demonstrated remarkable success in various computer vision tasks, including defect detection in metal components. Some popular Deep Learning models used in metal defect detection are:
R-CNN: R-CNN is a two-stage object detection model that localizes objects in an image and then classifies them. It can effectively detect and classify different types of metal defects.
U-Net: U-Net is a convolutional neural network architecture designed for semantic segmentation tasks. It can precisely segment and identify defects in metal images, enabling accurate defect localization.
One-Class Classification (OCC): OCC is a specialized technique for anomaly detection, where the model is trained on normal (defect-free) samples. It can effectively identify deviations from the normal pattern, making it suitable for defect detection.
Saiwa's platform integrates several deep learning models and fuses their strengths to provide a comprehensive solution for metal defect detection. By leveraging the capabilities of CNNs (Detectron2 and Yolov5), Saiwa enables industries to identify defects with precision and improve operational efficiency. The convergence of these models within the Saiwa framework reflects a quantum leap in pursuing flawless quality assurance in metal production processes.
Challenges in Metal Defect Detection Using AI
While AI has shown immense promise in metal defect detection, several challenges need to be addressed:
Limited Labeled Data Available:
Training Deep Learning models requires large labeled datasets, which can be scarce for specific defect types.
Lighting and Environmental Conditions:
In real-world manufacturing environments, lighting and environmental conditions can impact the quality of image data, making defect detection more challenging.
Applications of Metal Defect Detection in Industries
The integration of AI-powered defect detection systems has found applications in various industries, including:
Manufacturing: AI-driven defect detection ensures that metal components meet stringent quality standards, reducing production costs and enhancing product reliability.
Aerospace: The aerospace industry relies on defect-free components to ensure the safety and performance of aircraft.
Automotive: Defect detection in automotive components ensures vehicle safety and reduces the likelihood of recalls.
Construction: AI enables the early detection of defects in construction materials, preventing potential hazards in infrastructure.
Outlook
Automated visual surface inspection of metals enabled by machine learning and deep learning has enormous potential for enhancing manufacturing quality and reliability. But further advances are needed in areas like:
Novel defect detection: Most methods rely on models trained only on available labeled defects. Detecting new defect types will require semi-supervised and active learning approaches.
Explainability: Interpretability of model outputs is key for engineers to trust and act upon AI-based defect detections.
Multi-modal sensor fusion: Combining imaging with other sensor data provides additional insights into material anomalies.
Model uncertainty: Systems that quantify their own predictive confidence allow more targeted human inspector involvement.
Simulation-based training: As labeled defect data is scarce, rendered synthetic training data can improve model robustness.
With progress on these research frontiers, AI-powered metal surface defect detection will continue improving and opening new manufacturing possibilities.
Conclusion
While traditional inspection methods have been effective, integrating AI and deep learning has revolutionized defect detection, improving accuracy and efficiency. Through automation, real-time monitoring, and high accuracy, AI-powered defect detection systems offer manufacturers the ability to deliver defect-free products, enhancing customer satisfaction and brand reputation. With modern machine learning and deep learning techniques, such as convolutional neural networks, these systems can match or exceed the capabilities of human inspectors. As AI advances, the future of metal defect detection holds immense potential to reshape industries and drive innovation to new heights.