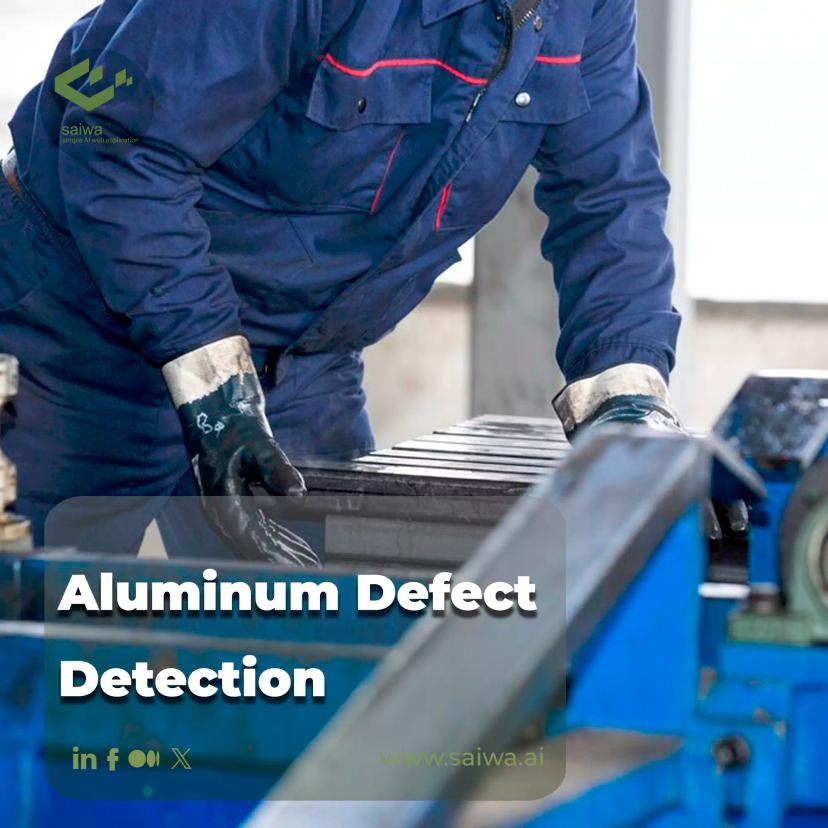
AI inspection for precision aluminum defect detection
Aluminum is one of the most widely used metals in automotive, aerospace, construction, consumer goods, and other industries due to its versatile properties like strength, durability, and lightweight nature. However, defects introduced during manufacturing can critically undermine aluminum's capabilities. Implementing robust quality control is imperative, but manual inspection is slow, inconsistent, and ineffective for spotting microscopic flaws. Fortunately, recent advances in artificial intelligence (AI) are automating surface aluminum defect detection, transforming quality assurance across the metals industry.
Read more: Metal Defect Detection | Enhancing Precision and Efficiency with AI
Aluminum Manufacturing Process
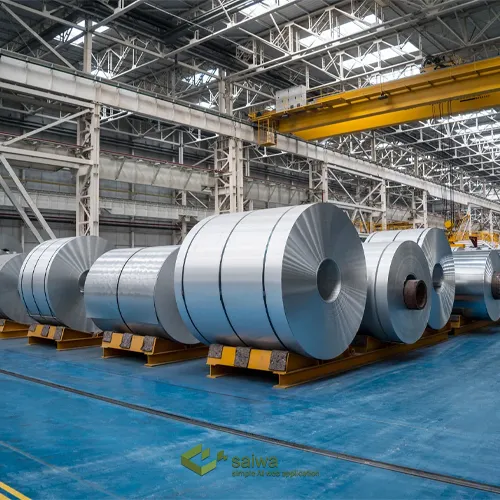
Aluminum fabrication involves the following key steps:
Bauxite Ore Refining: Mined bauxite ore is refined using the Bayer process to extract alumina. Impurities in the ore can result in compositional defects.
Alumina Smelting: Extracted alumina is smelted using the Hall-Héroult electrolytic process to produce molten aluminum. Contaminants from smelting can result in melt-related defects.
Molten Metal Treatment: The molten aluminum undergoes degassing, filtering, and fluxing to reduce dissolved hydrogen and solid impurities that cause defects.
Casting: The purified molten aluminum is cast into molds or ingots. Defects like air pockets, shrinkage cavities, and cracks can arise during solidification.
Rolling: Cast ingots are rolled under heat and pressure to produce aluminum sheets, plates, and foils. This can introduce surface flaws.
Extrusion: Aluminum billets are pushed through a die to create complex extruded profiles. Seams and die lines may form.
Forging: Applying localized pressure forges aluminum into final shapes. Improper techniques cause cracks and poor surface finish.
Each stage can potentially introduce defects like pits, cracks, metal inclusions, and surface roughness if optimal process parameters are not maintained. Real-time AI inspection provides crucial feedback to optimize these processes.
The Importance of Aluminum Defects
Identifying aluminum defect detections like cracks, pits, inclusions, and surface roughness during aluminum production is crucial for:
Preventing defective aluminum from progressing to downstream fabrication processes
Reducing scrap and minimizing substantial material wastage
Ensuring final product quality, reliability, and safety
Avoiding expensive manufacturing line shutdowns and maintenance from undetected defects
Optimizing process parameters like temperature, pressure, and impurity levels based on defect feedback
Overall, effective inline defect detection via techniques like automated AI-based vision enables proactive control of aluminum quality.
Traditional Methods for Aluminum Defect Detection
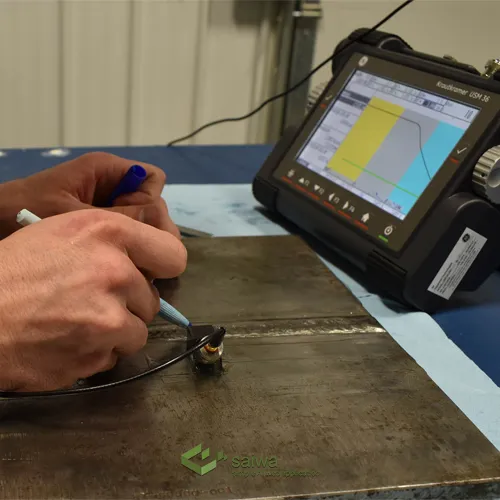
Conventional aluminum quality inspection relies on techniques such as:
Visual Inspection: Manual examination of surfaces for visible flaws. Slow, inconsistent, and ineffective for small defects.
Liquid Penetrant Inspection: Applying indicator fluids to highlight surface discontinuities. Time-consuming post-processing.
Ultrasonic Testing: High-frequency sound waves identify subsurface defects. Requires coupling and expert interpretation.
X-ray Radiography: X-ray imaging reveals internal defects. Low throughput and high equipment costs.
While providing value, these methods are too slow, labor-intensive, or expensive for high-volume inline manufacturing quality control.
The Role of AI in Revolutionizing Defect Detection
Modern computer vision and deep learning technologies allow automating visual surface inspection for faster and more consistent aluminum defect detection:
Deep neural networks reliably recognize microscopic defects missed by human inspectors.
Continuous inline inspection prevents defective material from progressing to downstream processes.
Scalable deployment across multiple production lines and facilities.
Reduces wastage and manual labor costs. Enables proactive improvements.
Processes high-resolution images unaffected by lighting, texture, and orientation changes.
Overall, AI-based automation makes aluminum defect detection cheaper, faster, and more reliable.
AI Techniques for Aluminum Surface Defect Detection
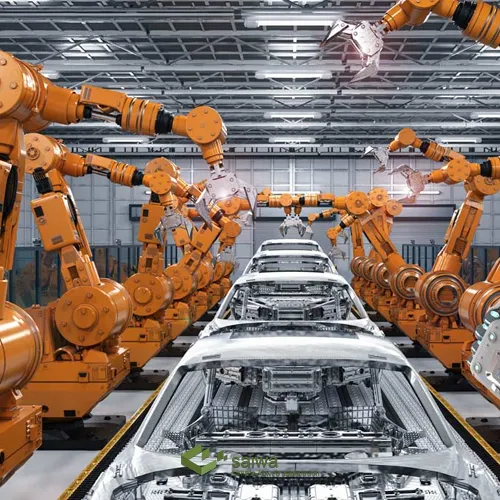
A variety of AI techniques are being applied for automated visual inspection and aluminum defect detection:
Image Classification Models
Image classification models like convolutional neural networks (CNNs) are commonly used where the goal is binary detection - labeling an aluminum surface image as either defective or normal. The CNN takes images as input and outputs a probability of defect. By training on labeled examples of normal and defective surfaces, CNN learns to recognize textural patterns indicative of flaws.
CNNs provide easy integration and can be trained to identify different defect categories like cracks, pits, scratches, etc. Data augmentation and test-time flipping/rotation improve robustness. However, CNNs do not provide defect localization within images.
Segmentation Models
Semantic segmentation models like U-Net are useful when more comprehensive localization of defective regions is needed. These models classify each pixel in the image as a defect or no defect. This highlights irregularities in the form of heatmaps and enables assessing defect shape and area precisely.
However, segmentation models cannot differentiate between multiple defect types in one image. They also struggle with small defects like micro-cracks. Segmentation is computationally expensive for industrial inline inspection.
Object Detection Models
Object detection models like YOLO (You Only Look Once) are ideal for localizing multiple individual defects in images with bounding boxes while categorizing the defect types in the aluminum defect detection field. This enables reliable defect counting, sizing, and retrieval.
However, training object detectors require more granular defect annotation. Ensembling object detection with segmentation can improve performance. Test augmentations like added Gaussian noise enhance robustness against production environment variability.
Ensemble Models
Given their complementary strengths, ensemble models combining CNN classifiers, object detectors, and semantic segmentation networks can improve overall precision and minimize the limitations of individual models. This provides multi-faceted defect analysis.
A thorough evaluation of curated test sets is essential to assess model performance before deploying for inline quality control. No single technique provides a silver bullet, and hybrid AI is ideal.
Automated Defect Detection Using Image Processing
Effective AI-based automated aluminum defect detection involves:
High-resolution imaging of aluminum surfaces using cameras and lighting optimizations.
Deep neural network architectures like convolutional neural networks trained on labeled defect datasets.
Models recognizing defective patterns and localizing flaws using segmentation and bounding boxes.
High-performance computing infrastructure for real-time inline inference.
Verification, dynamic model updates, and ensemble approaches for robustness.
User-friendly analytics dashboards providing actionable defect insights.
By proactively harnessing AI automation, aluminum producers can achieve new heights of manufacturing quality, efficiency, and consistency.
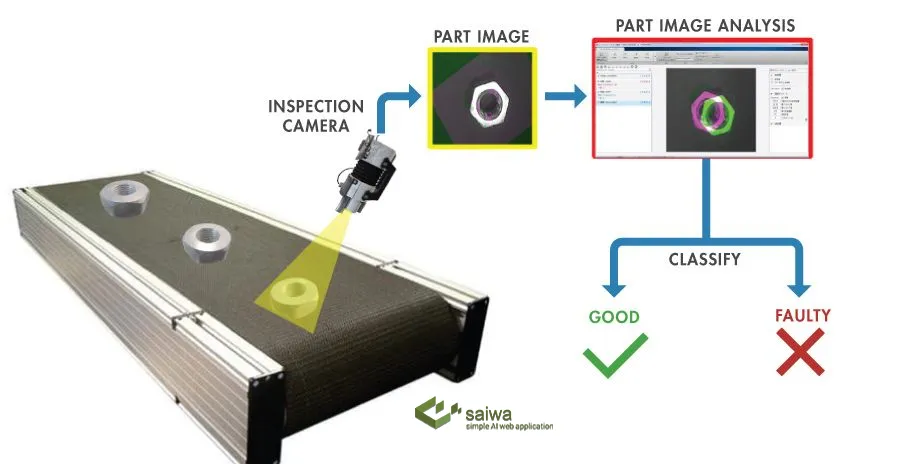
Conclusion
Detecting surface and subsurface defects is imperative for aluminum quality assurance during manufacturing. While traditional methods in aluminum defect detection have limitations, modern AI and computer vision enable fast, accurate, and automated inline defect inspection. AI promises to revolutionize archaic quality practices through intelligent and proactive solutions that minimize wastage and transform manufacturing.