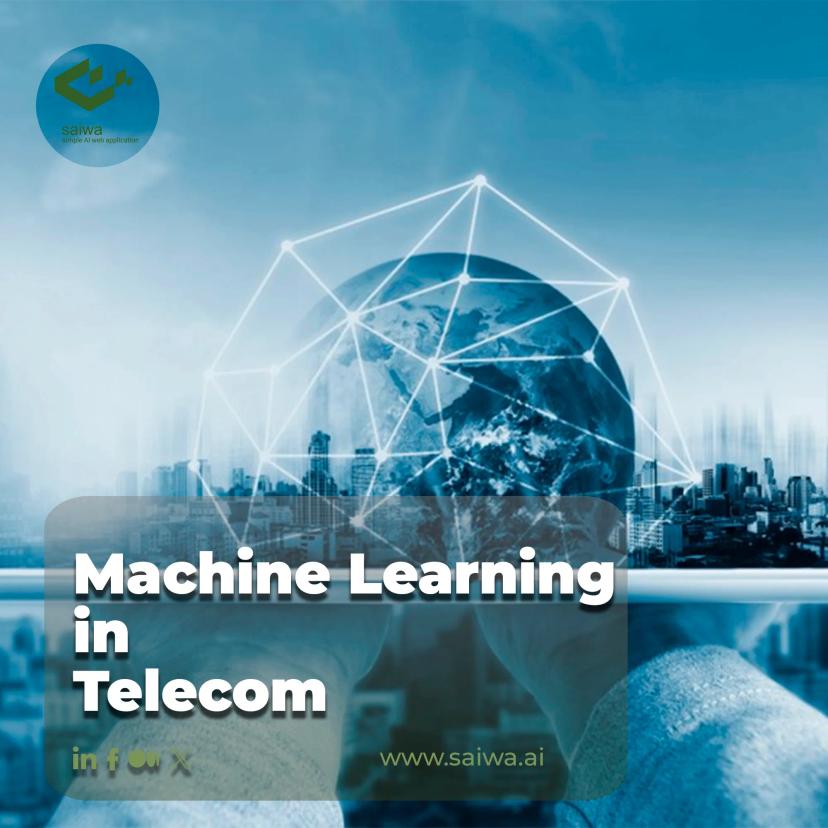
Machine Learning in Telecom | From Data to Insights
The telecommunications industry generates massive amounts of data daily from multiple sources including phone calls, texts, internet activity, and device interactions. Telecom companies have huge amounts of customer data that hold valuable insights about usage patterns, service quality, network operations, and market trends. Machine learning provides algorithms and statistical models to automatically analyze this data, identify correlations, make predictions, and recommend actions without needing explicit programming.
Telecom service providers are adopting machine learning across business functions from customer experience to network optimization. By uncovering insights from data that is already available within their systems using ML techniques, carriers can reduce costs, risks, and complexities while accelerating innovation and revenue growth. As 5G deployments intensify data traffic, applying machine learning in telecom will become imperative to evolve and adapt systems to dynamic demands.
What is Machine Learning?
Machine learning trains computational models to learn from data patterns and make decisions or predictions without relying on rules-based programming. The algorithms keep improving their analysis and output accuracy as they process more real-world data. Machine learning methods can be classified into three main categories:
Supervised learning
Supervised learning feeds labeled data like matched input and output pairs into models during training so that they can learn the mappings between them. These trained models can then be applied to new unseen data to predict the correct outputs. Classification and regression techniques fall under supervised learning.
Unsupervised learning
Unsupervised learning relies on algorithms to identify inherent structures, relationships, and groupings within unlabeled input data on their own without any accompanying trainer output values. Clustering data into distinct categories based on discovered similarities is an example unsupervised learning task.
Reinforcement learning
Reinforcement learning incentivizes desired behaviors of machine learning models through a system of rewards and penalties without needing labeled input/output pairs. Maximizing long-term rewards is the objective during training. Game-playing bots leverage reinforcement learning to enhance strategies.
Read Also: Unlock the power of Machine Learning as a Service (MLaaS)
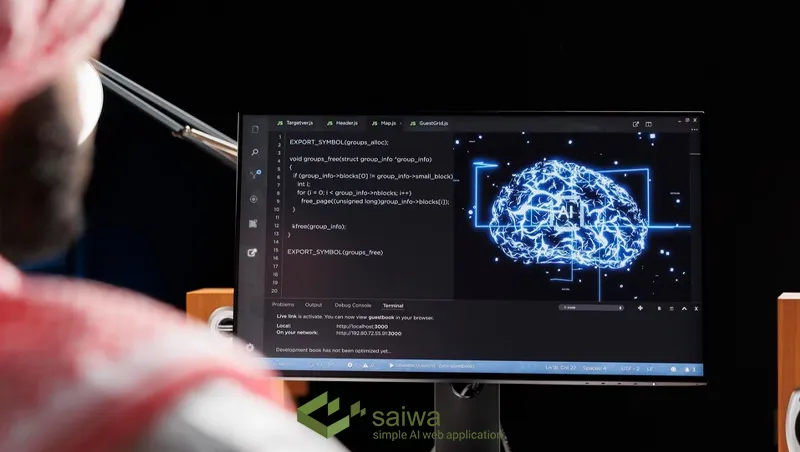
Machine Learning in the Telecom Industry
Rapid technology advancements and cutthroat competition have significantly disrupted the telecom landscape. Carriers need to swiftly analyze market shifts, optimize investments, enhance quality-of-service, retain customers, and diversify revenue streams. The insights required to drive these mission-critical decisions can be obtained by applying machine learning to tap into the wealth of data generated across telecom networks and systems every second.
ML offers telecom providers actionable intelligence to serve customers better, accelerate innovation, automate processes, and improve business performance. Telecom organizations are utilizing machine learning across various functions:
Marketing: Segment consumers, predict churn, target offers, and personalized pricing
Customer Service: Sentiment analysis, intelligent chatbots, cognitive engagement
Network Operations: Traffic forecasting, predictive maintenance, anomaly detection
Fraud Management: Unusual usage patterns, suspicious activity indicators
New Products: Demand simulation, viral behavior modeling, adoption forecasting
As 5G networks expand globally, they will trigger an exponential increase in connected devices and data traffic. Handling the scale and complexity these expansions entail will necessitate intelligent and automated decision making which machine learning solutions can aptly deliver.
Applications of Machine Learning in Telecom
Here are some specific application areas where machine learning algorithms are helping telecom service providers derive transformative business value from data:
Churn Prediction
Supervised classification models predict the likelihood of customers canceling subscriptions based on usage patterns, billing, network quality, and service interactions. This use of machine learning in telecom allows proactive retention efforts.
Recommendation Systems
Collaborative filtering and content-based filtering algorithms personalize suggestions to enhance customer experience across channels based on behavioral data. This grows revenue opportunities.
Text Analytics
Machine learning performs sentiment analysis on unstructured customer feedback and call transcripts to identify pain points and evaluate service quality. Dashboards highlight areas for improvement.
Network Optimization
Algorithms analyze infrastructure performance data across locations to forecast demand, allocate resources, route traffic, flag anomalies, and prevent outages. This reduces costs.
Fraud Management
Unsupervised anomaly detection identifies suspicious usage spikes, unusual activity combinations, and improbable sequences to minimize telecom fraud. This improves security.
Marketing Analytics
Machine learning segments customers determines attribution and propensity models, optimizes pricing, and measures campaign effectiveness to sharpen targeted advertising.
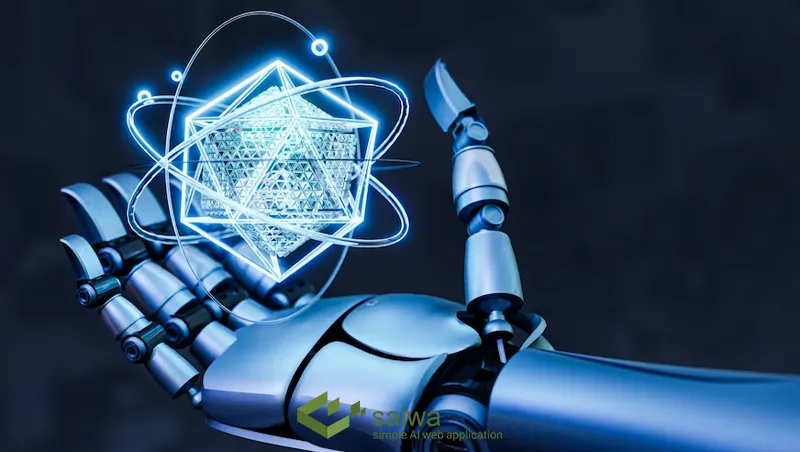
Machine Learning in Telecom Operations
Machine learning in telecom is transforming telco operations by enabling intelligent automation at scale. ML tackles the most complex and data-intensive operations use cases that previously required extensive human intervention:
Network Planning
Prior network expansions relied solely on expert estimations. Now ML evaluates billions of spatial-temporal data points about call traffic, device mobility, demographic trends, and application usage to provide granular, optimized decisions on where to upgrade, densify, and invest in infrastructure.
Predictive Maintenance
Machine learning in telecom models is trained on asset health indicators including signal quality metrics, firmware versions, temperatures, and outage histories to determine failure probabilities for towers, switches, and transmission equipment. This permits just-in-time maintenance.
Anomaly Detection
By baseline modeling normal network performance, machine learning algorithms can pinpoint abnormalities like traffic congestion or element misconfigurations from massive volumes of performance data and identify the root causes for rapid remediation.
Automated Assurance
Bots leverage image recognition and natural language processing to parse thousands of daily alarm notifications and trouble tickets. They auto-diagnose issues, implement fixes, or auto-escalate complex problems to appropriate teams. This transforms assurance functions.
Benefits of Using Machine Learning in Telecom
Applying ML to mine value from the extensive data continuously generated in running large-scale telecom networks and serving millions of subscribers is delivering manifold benefits:
Higher efficiency: Automating manual tasks improves productivity and reduces costs
Enhanced agility: Faster adaption to usage variations and market changes
Greater reliability: Minimizing network failures through predictive models
Improved quality: ML recommendations enhance and personalize customer service
New revenues: Demand-based tailored offerings and pricing maximize customer lifetime value
Lower churn: Customized retention campaigns proactively engage customers
Optimized investments: Data-driven infrastructure capacity planning reduces overprovisioning
Proactive security: Early fraud detection minimizes financial risks
The practical, proven impact machine learning is already achieving for telecommunications indicates even broader transformations as 5G further accelerates data expansion.
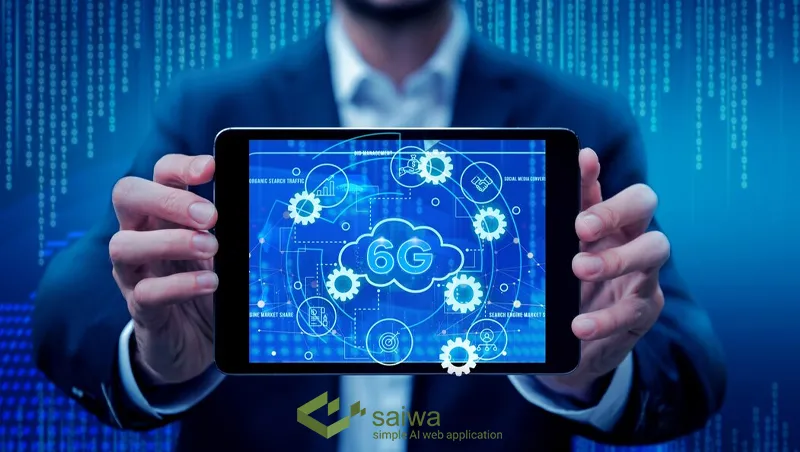
Conclusion
The exponential increase in data traffic and connected devices with 5G requires telecom carriers to incorporate machine learning across functions to effectively harness valuable insights at scale for strategic advantage. Machine learning in telecom facilitates leveraging the network and customer knowledge locked within petabyte-sized databases to drive intelligent, informed decisions automatically without human bottlenecks.
By deploying machine learning to uncover correlations, patterns, and trends that would be impossible to manually analyze, telecom operators can boost efficiency, productivity, and speed to unlock innovation and profitability. Processing billions of data points daily for accurate forecasting and risk reductions requires intelligent algorithms.
With growing industry competition and technology disruptions, the need for rapid adaptation accentuates the benefits of machine learning for telecommunications. ML delivers data-driven agility and innovation essential for succeeding in the 5G era through actionable intelligence that transforms business operations, enhances customer engagement, and creates new revenue opportunities.