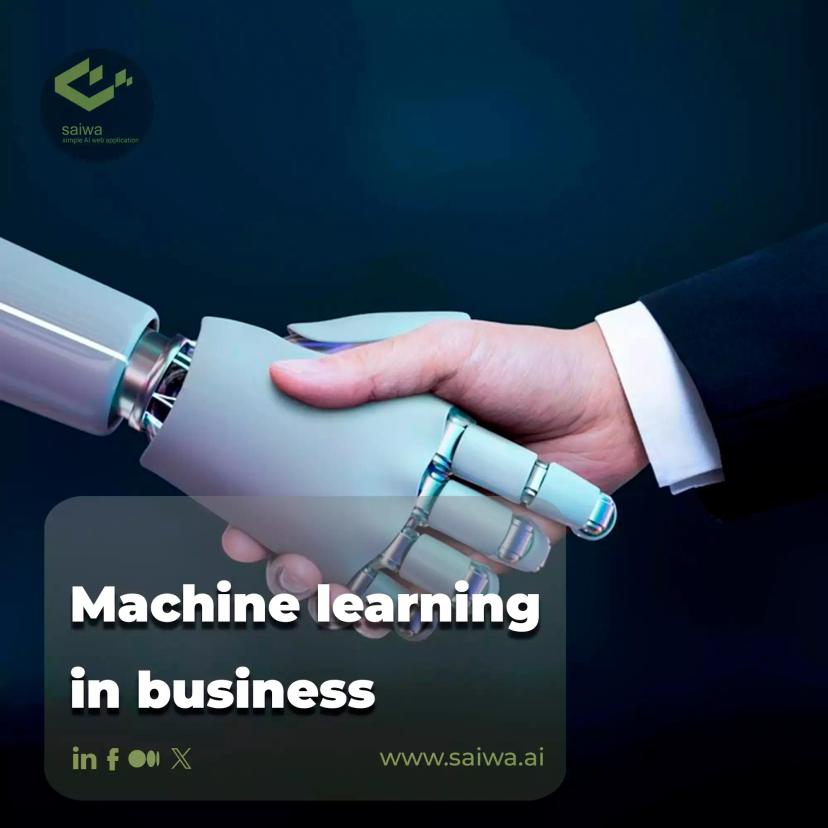
Machine learning in business| Advantages & Applications
With the rapid development of data science, organizations are increasingly focused on improving their operational efficiency through the use of modern technologies. Machine learning in business is a particular technology that enables organizations to efficiently derive insights from unstructured data. With very little programming, machine learning can be used to iteratively learn from a given data set to understand structures, actions, etc.
Machine learning in business processes is continuous and often growing, allowing companies to stay ahead of both organizational and customer demands. Furthermore, with all the major cloud providers offering ML platforms, it is easier than ever to develop or integrate ML into existing business processes.
A thorough overview of the most popular use cases and applications of machine learning in business is presented in the following article.
What is Machine Learning (ML)?
The science of programming computers to improve their performance by learning from data is known as machine learning, a subfield of artificial intelligence. It involves computers developing the skills they need to perform certain tasks without being explicitly programmed to do so.
This is done by using complex algorithms which teach the computer how to process the data. Each time the computer receives new data, its algorithms identify trends and make accurate predictions.
But that's not all. In addition to pattern recognition and prediction, which are often used in today's life and technology services, a computer can perform various other activities depending on the algorithms it is given. What sets machine learning algorithms apart from the competition is their practical correctness.
Read more: Unlock the power of Machine Learning as a Service (MLaaS)
Machine Learning Algorithms
It is important to understand all of the methods in which machine learning in business can operate to learn what it can be used for and how it works. Let's look at how machine learning and machine learning as a service operate through a combination of supervised, unsupervised, and reinforcement learning.
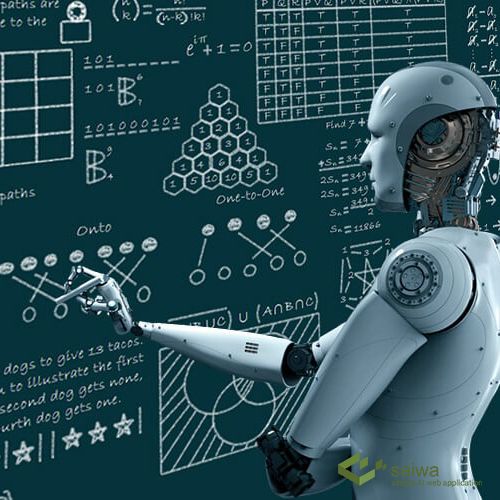
Supervised Learning
To train Machine Learning in business models, supervised learning utilizes data that has already been labeled or tagged. In such a framework, humans initially label the data before sending it to computers so they can figure out what it is. Through this process, the computer learns how to automatically recognize related data from fresh datasets. As a consequence, supervised learning enables organizations to address saleable problems like sorting spam from your email.
Supervised learning collects data from a previous experience or generates data from that occurrence. It helps with a range of real-world computing problems and assists with optimizing performance requirements based on prior experience.
Unsupervised Learning
Unsupervised learning refers to the process by which machine learning in a business algorithm learns from data without external supervision. The algorithm must operate on the information without any supervision, and the unsupervised models can be trained on the unlabeled dataset, which is neither classified nor categorized. In unsupervised learning, the model searches the vast amount of data for meaningful insights, rather than producing a predetermined result. These are used to address the problems of association and clustering.
Reinforcement Learning
By putting AI into a game-like scenario, reinforcement learning teaches computer models to make decisions. In reinforcement learning, the algorithm gains knowledge by making mistakes and using the feedback from those mistakes. The use of rewards and punishments to signal desired and undesired behavior.
The ML model learns from its own experience to maximize the accuracy of its output by receiving positive and negative feedback on its behavior through rewards and punishments. The agent receives no oversight. In reinforcement learning, the Q-Learning algorithm is utilized.
Advantages of Machine Learning in Business
Machine learning in business has moved from the realm of science fiction to become a critical element of today's businesses, with the widespread adoption of machine learning technology by organizations in virtually every industry. With machine learning being used in logistics, manufacturing, hospitality, travel, tourism, energy, and utilities, numerous use cases are emerging across all industries.
Real-time chatbot agents
Chatbots are becoming more human-like in their speech and more adept at predicting and fulfilling user needs. Chatbots have the potential to become even more interesting and useful when machine learning and natural language processing (NLP) through AI are added. Digital assistants such as Siri, Google Assistant, and Amazon's Alexa are powered by machine learning algorithms, and new platforms for customer service and interaction could eventually use this technology instead of traditional chatbots.
Promote reliable medical diagnostics and predictions
Thanks to machine learning in healthcare, it is now possible to identify high-risk patients, diagnose them, provide the best treatment, and anticipate readmissions. The main sources of these insights are symptoms and data from anonymized medical records. Without unnecessary medication, patient recovery can be accelerated. The medical sector can improve patient health thanks to ML.
Market analysis and Client segmentation
Businesses can use machine learning software's customer segmentation and predictive inventory management capabilities to set prices while offering the right products and services to the right customers at the right time. According to Adnan Masood, chief architect at UST Global and a specialist in artificial intelligence and machine learning, retailers use machine learning in business to predict what goods will sell best in their locations based on seasonal considerations and the area's demographics and other data points.
Fraud detection
Machine learning is an effective way to detect fraud since it can quickly identify irregularities and spot trends. Financial institutions in this industry have been using machine learning for years.
This is how it works: Machine learning can be used to understand a customer's typical behaviors, such as when and where they use their credit card. By analyzing the data in milliseconds, machine learning can use this and other data sets to quickly distinguish between transactions that fall within expected norms and those that may be potentially fraudulent.
ReadMore: Role of Machine Learning in Education and its Benefits
Applications of Machine Learning in Business
In many areas, such as marketing and advertising, customer support, and product development, machine learning can be used to streamline your workflows and processes. Here are some of its most popular applications.
Anticipating customer purchase behavior
To predict customer behavior and buying trends, businesses can use machine learning and data extraction. The algorithms help to show the best deals to specific customers based on their browsing and purchasing history.
Increasing customer satisfaction
In order to find hidden patterns and related group items, machine learning in business algorithms assists in analyzing a customer's purchasing history while comparing it to a large inventory of products. Product suggestions are based on this information.
An examination of the financial situation
Machine learning in business is used in finance for portfolio management, algorithmic trading, loan underwriting, and fraud detection. Future applications of machine learning in banking include chatbots and other conversational interfaces for security, customer service, and sentiment analysis.
Enhancing cybersecurity
Cybersecurity may also be enhanced by machine learning. Based on user data, the algorithms are capable of quickly identifying the most likely vulnerabilities as well as potential malware and spyware programs.
Read more : What Is The AI Impact on Society?
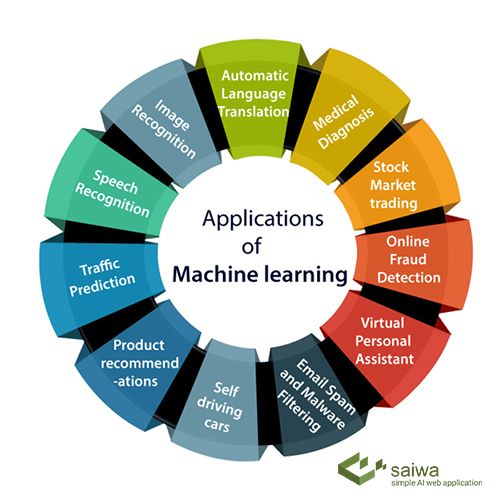
Importance of Machine Learning in Business
Traditional data analysis models are based on trial and experimentation. When it comes to solving complex problems involving large data sets, these approaches are no longer useful or practical.
Machine learning can now perform certain tasks faster and more accurately than humans using traditional models. It has also made it possible to analyze huge amounts of data at breakneck speed to provide answers and solutions instantly.
The fact that machine learning does not require human intervention is its main advantage and contribution to real-world applications. By saving so much time, people can devote their time and energy to more productive work.
Read more: 10 Machine Learning Companies You Need To Know
Future of Machine Learning in Business
Here we'll discuss 2 trends that researchers predict will develop over the next few decades. They are all the result of ongoing battles and recent developments in the market.
The future of machine learning could be shaped by quantum computing
One development that has the potential to improve machine learning capabilities is quantum computing. Faster data processing is made possible by quantum computing, which enables the execution of simultaneous multi-state processes. Quantum machine learning can improve data processing and produce deeper insights. Businesses can take advantage of this improved performance to achieve better results than they could with traditional machine learning techniques.
There isn't a quantum computer ready for commercial use yet. However, some major IT companies are making investments, and the emergence of quantum machine learning is not far off.
The entire model development process is simplified with AutoML
Using machine learning algorithms to perform tasks in the real world is called automated machine learning, or AutoML. With AutoML, anyone or any organization can use sophisticated machine learning models and techniques without having to be an expert in the field.
Thanks to AutoML, machine learning can be used by a wider audience, which suggests that it can change the technological environment. For example, a data scientist can use AutoML to quickly identify the algorithms they can apply or to determine if any algorithms have been overlooked.
Machine learning is quickly becoming a fundamental technology that is being organically embraced across all business sectors to solve difficult business challenges and increase an organization's efficiency and scalability.
Despite the challenges associated with ML adoption, organizations are willing to undertake this time-consuming and relatively expensive process because it offers real and significant advantages over more traditional analytical techniques.
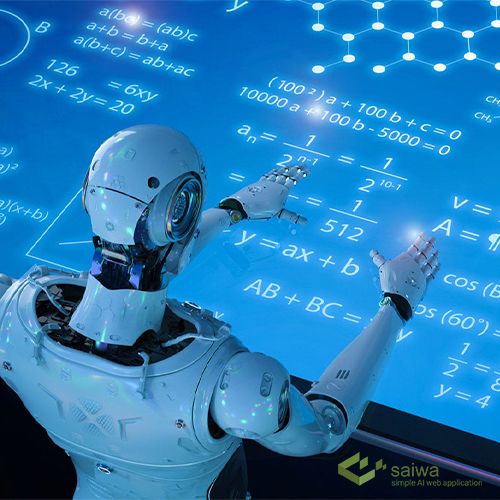