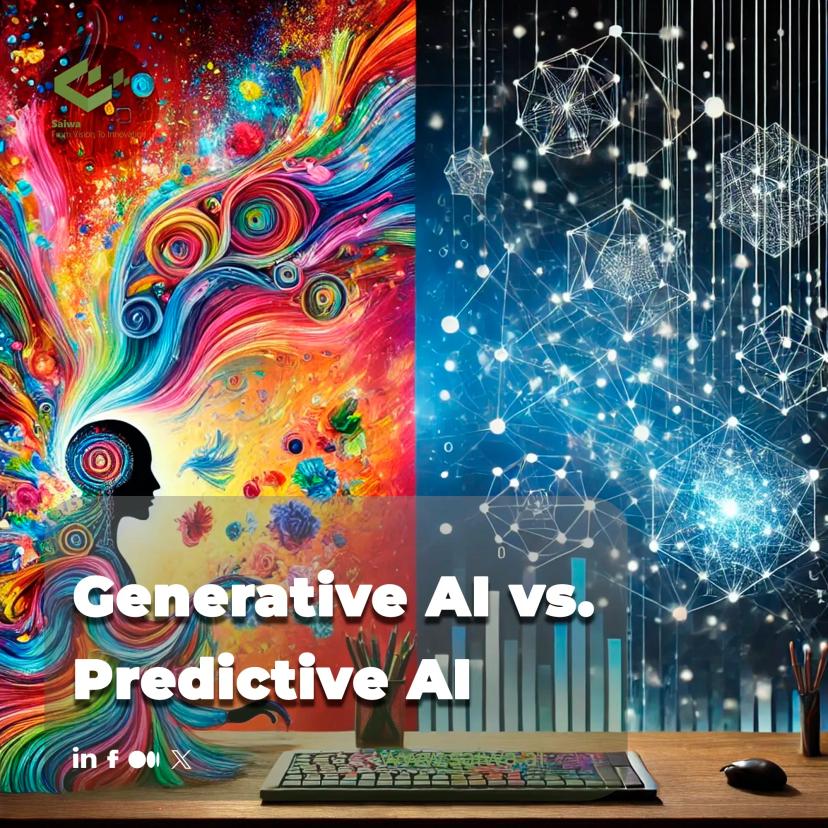
Generative AI vs. predictive AI | What's the difference?
Artificial intelligence (AI) has rapidly evolved, permeating various aspects of our lives and revolutionizing industries. Within this vast field, two prominent branches have emerged: generative AI and predictive AI. While both leverage the power of data and algorithms, their purposes, functionalities, and applications differ significantly.
Saiwa is an AI company that provides a service-oriented platform that delivers advanced artificial intelligence (AI) and machine learning (ML) solutions. Leveraging innovative technologies, Saiwa helps businesses improve efficiency, solve complex problems, and drive growth with customized AI and ML services. Their approach enables organizations to unlock the full potential of data and automation to achieve transformative results.
This article examines the intricacies of generative and predictive AI, exploring their underlying mechanisms, diverse applications, respective benefits, and the ethical considerations surrounding their use. By understanding the nuances of each approach, we can better appreciate their distinct roles in shaping the future of technology and their potential impact on society.
What is Generative AI?
Generative AI represents a class of artificial intelligence algorithms designed to create new, original content. Unlike traditional AI systems that primarily analyze existing data, generative AI models learn the underlying patterns and structures of the input data and then utilize this learned knowledge to generate novel outputs that resemble the training data.
These outputs can take various forms, including text, images, audio, code, and even 3D models. The defining characteristic of generative AI is its ability to produce something new, rather than simply classifying or predicting based on existing data.
How Does Generative AI Work?
Generative AI models typically employ deep learning architectures, particularly generative adversarial networks (GANs), variational autoencoders (VAEs), and transformer networks. GANs consist of two neural networks: a generator and a discriminator.
The generator creates new data instances, while the discriminator evaluates the authenticity of these generated instances, attempting to distinguish them from real data. Through an iterative training process, the generator improves its ability to create realistic data, while the discriminator becomes more adept at identifying fake data. VAEs, on the other hand, learn a compressed representation of the input data and then use this representation to generate new data samples.
Transformer networks, initially designed for natural language processing, have also proven effective in generating various forms of content, including text, images, and music.
Generative AI Applications
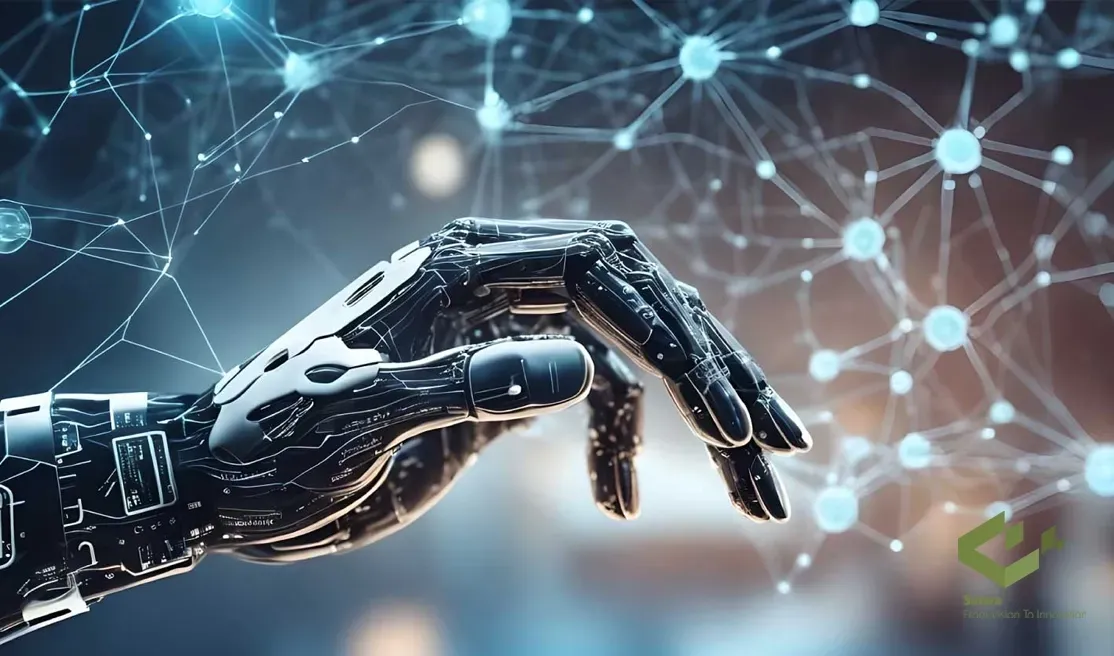
The applications of generative AI are diverse and rapidly expanding:
Creative Content Generation
Generative AI can create original artwork, music, writing, and other forms of creative content, pushing the boundaries of artistic expression and enabling new forms of creative exploration. This includes generating realistic images, composing unique musical pieces, writing different kinds of creative text formats, and even scripting screenplays. These capabilities empower artists and creators with new tools and inspiration, fostering innovative forms of artistic expression and potentially automating tedious creative tasks.
Design and Engineering
Generative design tools leverage AI to generate design options based on specified constraints and objectives, accelerating the design process and exploring innovative solutions. By inputting parameters such as materials, weight, and performance requirements, engineers can utilize generative AI to explore a vast array of design possibilities, optimizing for specific criteria and discovering novel solutions that might be overlooked by traditional design methods. This is particularly useful in fields like architecture, automotive design, and aerospace engineering.
Drug Discovery and Development
Generative AI can be used to design new molecules with desired properties, accelerating the drug discovery process and potentially leading to the development of more effective treatments.
By modeling molecular structures and predicting their interactions with biological targets, generative AI can identify promising drug candidates, significantly reducing the time and cost associated with traditional drug discovery methods.
AI in drug discovery has the potential to revolutionize pharmaceutical research and accelerate the development of life-saving medications.
Personalized Experiences
Generative AI can personalize user experiences in various domains, such as e-commerce, entertainment, and education, by tailoring content and recommendations to individual preferences.
This includes generating personalized product recommendations, creating customized learning paths, and even generating personalized news feeds and social media content. By understanding individual user preferences, generative AI can enhance user satisfaction and engagement, creating more relevant and meaningful experiences.
Data Augmentation
Generative AI can be used to augment existing datasets with synthetic data, improving the performance of machine learning models, particularly in scenarios with limited training data.
When real-world data is scarce or expensive to collect, generative models can create synthetic data that mimics the characteristics of the real data, effectively increasing the size of the training dataset and improving the accuracy and robustness of machine learning models. This is especially valuable in fields like medical imaging and computer vision.
Benefits of Generative AI
Generative AI offers several advantages:
Enhanced Creativity
Generative AI can augment human creativity by providing new tools and inspiration for artistic expression and design. It allows artists to explore new styles and mediums, experiment with different creative approaches, and overcome creative blocks.
By automating repetitive tasks, generative AI frees up artists to focus on higher-level creative aspects, pushing the boundaries of artistic expression and fostering innovation in creative fields. It can also be used to create personalized art, music, and literature tailored to individual preferences.
Automation and Efficiency
Generative AI can automate tasks that previously required significant human effort, such as content creation and design generation, improving efficiency and productivity. This automation can significantly reduce production time and costs, allowing businesses to create more content in less time.
Automated content creation can be particularly beneficial for tasks like generating marketing copy, product descriptions, and social media posts, freeing up human resources for more strategic initiatives.
Personalization
Generative AI enables personalized experiences by tailoring content and recommendations to individual preferences, enhancing user satisfaction and engagement.
This can lead to more effective marketing campaigns, personalized learning experiences, and customized product recommendations. By understanding individual user needs and preferences, generative AI can create more relevant and engaging experiences, fostering stronger customer relationships and improving user retention.
Innovation
Generative AI can drive innovation by exploring new design spaces and generating novel solutions to complex problems. By generating a wide range of design options, generative AI can help identify innovative solutions that might be overlooked by traditional methods.
This capability can lead to breakthroughs in fields like engineering, architecture, and materials science, pushing the boundaries of what's possible and driving technological advancements. This can also lead to the creation of entirely new products and services, disrupting existing markets and creating new economic opportunities.
What is Predictive AI?
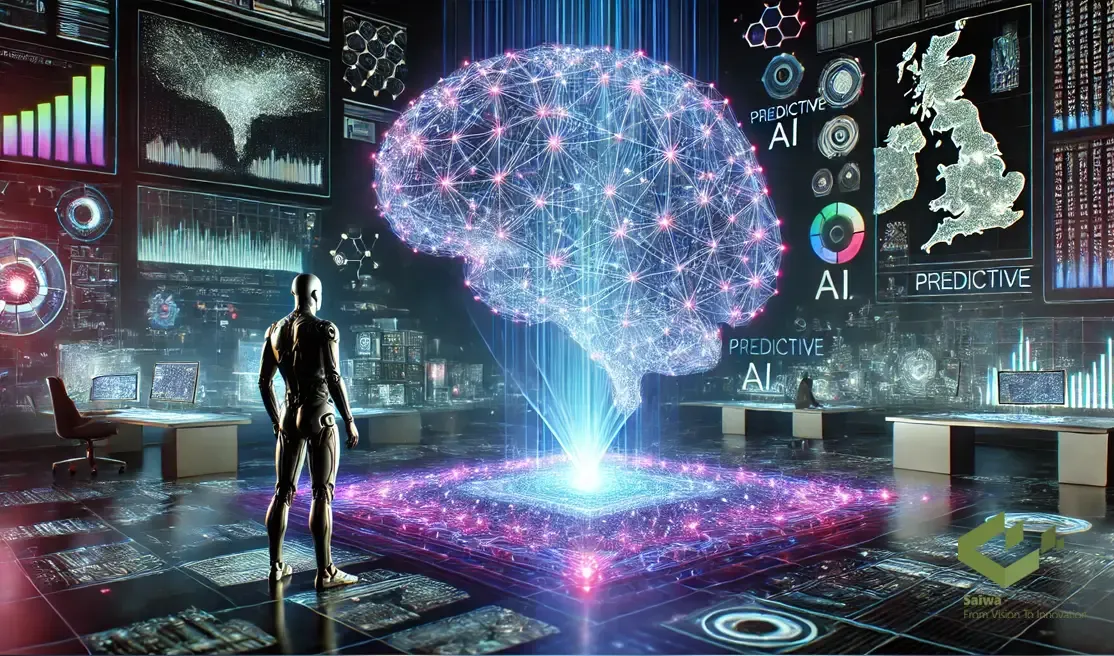
Predictive AI focuses on forecasting future outcomes based on historical data and statistical modeling. These models analyze past patterns and trends to identify relationships between variables and then use these relationships to predict future events or behaviors. Predictive AI is concerned with anticipating what is likely to happen, rather than creating something new.
How Predictive AI works
Predictive AI models employ various machine learning algorithms, including regression, classification, and time series analysis. Regression models predict continuous values, such as sales figures or stock prices.
Classification models predict categorical values, such as customer churn or disease diagnosis. Time series analysis models analyze data collected over time to identify trends and patterns and then use these patterns to forecast future values. The choice of algorithm depends on the specific problem and the nature of the data.
Predictive AI Applications
Predictive AI finds applications in numerous fields:
Business Analytics
Predictive AI can forecast sales, customer behavior, and market trends, enabling businesses to make informed decisions and optimize their strategies. By analyzing historical sales data, customer demographics, and market trends, predictive models can identify potential growth opportunities, optimize pricing strategies, and improve inventory management.
This data-driven approach allows businesses to anticipate market changes and adapt their strategies proactively, gaining a competitive edge and maximizing profitability. Predictive AI can also be used to personalize marketing campaigns and improve customer targeting.
Financial Modeling
Predictive AI can predict stock prices, assess credit risk, and detect fraudulent activities, improving financial decision-making and risk management. By analyzing market data, economic indicators, and company financials, predictive models can provide insights into future stock performance, helping investors make informed investment decisions.
Predictive AI can also be used to assess the creditworthiness of borrowers, reducing the risk of loan defaults. Furthermore, it can detect patterns indicative of fraudulent activities, protecting financial institutions and consumers from financial losses.
Healthcare
Predictive AI can predict patient outcomes, diagnose diseases, and personalize treatment plans, improving healthcare delivery and patient care. By analyzing patient medical history, genetic information, and lifestyle factors, predictive models can identify individuals at risk of developing certain diseases, enabling early intervention and preventative care.
Predictive AI can also assist in diagnosing diseases by analyzing medical images and identifying patterns indicative of specific conditions. Furthermore, it can personalize treatment plans by considering individual patient characteristics and predicting treatment response.
Weather Forecasting
Predictive AI models analyze weather data to forecast future weather conditions, improving the accuracy and timeliness of weather predictions. By incorporating vast amounts of data from various sources, including satellites, weather stations, and radar systems, predictive models can generate more accurate and detailed weather forecasts.
These improved forecasts can help individuals and organizations make informed decisions regarding travel, agriculture, and disaster preparedness, minimizing the impact of adverse weather events. They also contribute to better climate modeling and understanding long-term weather patterns.
Risk Management
Predictive AI can assess and predict various types of risks, such as credit risk, insurance risk, and operational risk, enabling organizations to mitigate potential losses. By analyzing historical data and identifying patterns associated with past incidents, predictive models can assess the likelihood and potential impact of future risks. This information allows organizations to implement proactive risk mitigation strategies, reducing the probability of adverse events and minimizing their financial and operational impact. This can include optimizing resource allocation, improving safety protocols, and developing contingency plans.
Benefits of Predictive AI
Predictive AI offers several benefits:
Improved Decision-Making
Predictive AI provides data-driven insights that inform decision-making, leading to more effective and efficient outcomes. By analyzing historical data and identifying trends, predictive models can provide valuable insights that help organizations make better decisions across various domains.
This can include optimizing resource allocation, improving strategic planning, and making more informed investment decisions. Predictive AI removes the guesswork and allows for data-backed decisions, leading to better outcomes.
Risk Mitigation
Predictive AI can identify and assess potential risks, enabling organizations to take proactive measures to mitigate those risks. By analyzing data and identifying patterns associated with past incidents, predictive models can assess the likelihood and potential impact of future risks.
This allows organizations to implement preventative measures and reduce the probability of negative outcomes, minimizing financial losses and operational disruptions. Early identification of potential risks allows for timely intervention and more effective risk management.
Enhanced Efficiency
Predictive AI can automate tasks and optimize processes, improving efficiency and productivity. By automating repetitive tasks and optimizing workflows, predictive AI can free up human resources to focus on more strategic activities.
This can lead to increased productivity, reduced operational costs, and improved overall efficiency. Automation can also reduce human error and improve the speed and accuracy of various processes.
Personalization
Predictive AI can personalize experiences by tailoring recommendations and services to individual needs and preferences. By analyzing user data and understanding individual preferences, predictive AI can provide personalized recommendations for products, services, and content.
This can enhance customer satisfaction, improve user engagement, and increase sales. Personalization creates more relevant and tailored experiences, leading to increased customer loyalty and improved business outcomes.
What’s the difference between generative AI and predictive AI?
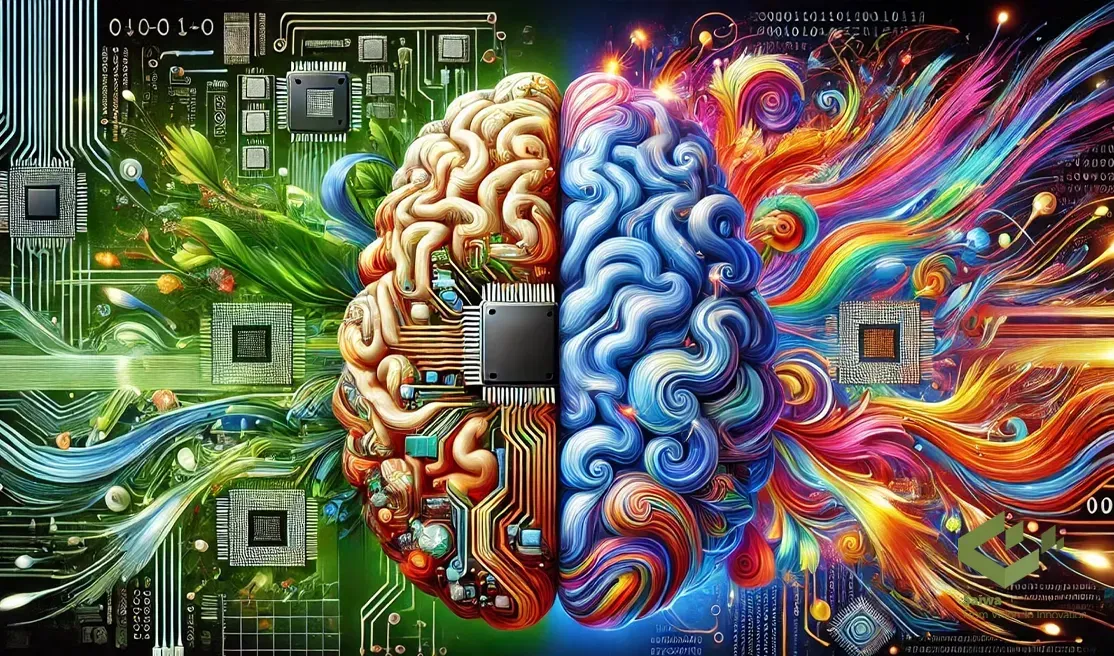
While both generative and predictive AI leverage data and algorithms, their core functionalities and applications differ significantly.
Purpose
Generative AI: Creates new, original content. This involves learning the underlying patterns and structure of input data to generate novel outputs resembling the training data. It's not about analyzing existing data but creating something entirely new, whether it's text, images, music, or code. The focus is on generating creative and innovative outputs.
Predictive AI: Forecasts future outcomes. This involves analyzing historical data to identify trends and patterns, then using these patterns to predict future events or behaviors. The goal is to anticipate what is likely to happen, focusing on accuracy and reliability in forecasting future values or categories.
Output
Generative AI: Generates novel data instances (text, images, audio, etc.). These outputs are unique and original, although they are based on the patterns learned from the training data. The generated outputs can be used for various purposes, such as creating art, generating realistic images, or composing music.
Predictive AI: Predicts future values or categories. The output is a prediction or forecast, often a numerical value or a classification label. For example, predicting customer churn (yes/no), forecasting sales figures, or predicting the likelihood of a disease.
Applications
Generative AI: Creative content generation, design, drug discovery, personalized experiences. It can be used to create art, music, and literature, design new products and optimize existing ones, discover new drugs and materials, and personalize user experiences in various domains like e-commerce and entertainment.
Predictive AI: Business analytics, financial modeling, healthcare, weather forecasting, risk management. It's used to analyze business data, predict financial markets, improve healthcare diagnostics and treatment, forecast weather conditions, and assess and mitigate various types of risks.
Algorithm Focus
Generative AI: GANs, VAEs, transformer networks. These algorithms are specifically designed for generating new data instances. GANs use a generator and discriminator network in a competitive process, VAEs learn compressed data representations, and transformers excel at generating sequential data like text and music.
Predictive AI: Regression, classification, time series analysis. These algorithms are focused on predicting future values or categories based on historical data. Regression predicts continuous values, classification predicts discrete categories, and time series analysis focuses on data collected over time.
Decision Support
Generative AI: Supports creative exploration and design innovation. By generating multiple design options and exploring new possibilities, generative AI helps designers and engineers think outside the box and come up with innovative solutions.
Predictive AI: Supports data-driven decision-making and risk mitigation. By providing insights into future trends and potential risks, predictive AI helps businesses and organizations make informed decisions, optimize strategies, and mitigate potential losses. It provides a basis for proactive and data-informed decision-making.
Key Similarities Between Generative AI and Predictive AI
Despite their differences, generative and predictive AI share some commonalities:
Data Dependence
Both rely on large datasets for training and learning. The quality and quantity of the data significantly impact the performance of both types of AI. Larger datasets generally lead to more accurate and robust models, allowing the AI to learn more complex patterns and relationships.
Data preprocessing and cleaning are essential for both generative and predictive AI to ensure the data is accurate and consistent. The effectiveness of both approaches is directly tied to the availability and quality of relevant data.
Algorithmic Foundation
Both utilize machine learning algorithms, albeit different types. Both rely on algorithms to learn from data and generate outputs. While generative AI uses algorithms like GANs, VAEs, and transformers to create new content, predictive AI uses algorithms like regression, classification, and time series analysis to forecast future outcomes.
Both approaches often leverage deep learning techniques, relying on neural networks with multiple layers to process and learn from complex data.
Potential for Impact
Both have the potential to transform industries and reshape various aspects of our lives. Generative AI can revolutionize creative industries, automate design processes, and personalize user experiences. Predictive AI can improve decision-making in various sectors, optimize resource allocation, and mitigate risks.
Both technologies are rapidly evolving and have the potential to drive significant societal and economic changes, impacting how we work, live, and interact with the world around us. They also present similar challenges regarding ethical considerations and responsible development.
Generative AI and Predictive AI: Ethical Considerations
The increasing adoption of generative and predictive AI raises important ethical considerations:
Bias and Fairness
AI models can inherit and amplify biases present in the training data, leading to unfair or discriminatory outcomes. If the training data reflects existing societal biases, the AI model may perpetuate and even exacerbate these biases in its outputs.
This can lead to discriminatory outcomes in areas such as loan applications, hiring processes, and even criminal justice. Mitigating bias requires careful attention to data collection, preprocessing, and model evaluation, as well as ongoing monitoring for unintended discriminatory effects. Addressing bias is crucial for ensuring fairness and equity in AI applications.
Privacy and Security
The use of personal data in AI models raises concerns about privacy and data security. Generative and predictive AI models often require access to large amounts of data, including sensitive personal information. Protecting this data from unauthorized access and misuse is crucial. Data anonymization and encryption techniques can help mitigate privacy risks, but careful consideration must be given to the potential for re-identification and data breaches. Robust security measures and responsible data governance are essential for maintaining public trust and protecting individual privacy.
Transparency and Explainability
The lack of transparency in some AI models makes it difficult to understand how they arrive at their decisions, raising concerns about accountability and trust. Complex AI models, particularly deep learning models, can be "black boxes," making it challenging to interpret their decision-making processes.
This lack of transparency can make it difficult to identify and correct errors, assess fairness, and build trust in the system. Developing explainable AI (XAI) techniques is crucial for addressing these concerns and ensuring that AI systems are accountable and transparent.
Job Displacement
The automation capabilities of AI raise concerns about potential job displacement in various sectors. As AI-powered systems become more capable of performing tasks previously done by humans, there is a risk of job displacement in certain industries.
While AI can also create new job opportunities, it is essential to address the potential negative impacts on employment and develop strategies for retraining and supporting workers who may be affected by automation.
This includes investing in education and training programs to prepare the workforce for the changing job market and exploring policies such as universal basic income to mitigate the potential economic consequences of job displacement.
Conclusion
Generative and predictive AI represent two distinct yet powerful branches of artificial intelligence. Generative AI focuses on creating new content, while predictive AI focuses on forecasting future outcomes.
Both approaches offer unique benefits and have the potential to revolutionize various industries. However, it is crucial to address the ethical considerations surrounding their deployment to ensure responsible and beneficial use of these transformative technologies.
As AI continues to evolve, understanding the nuances of generative and predictive AI will become increasingly important for navigating the complex landscape of artificial intelligence and harnessing its full potential for the betterment of society.