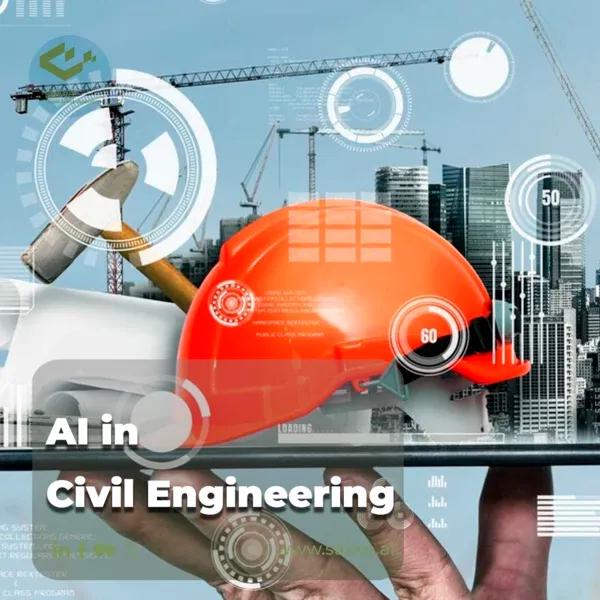
Unleashing AI in Civil Engineering| Building Tomorrow
Civil engineering undertakes complex projects to create and maintain critical infrastructure that supports human activity and economic progress. It involves coordinating numerous multifaceted technical tasks, integrating vast engineering data, modeling intricate structures across geographies, and maintaining robust operational systems. Artificial intelligence (AI) provides advanced algorithms to handle such elaborate challenges efficiently through automation, intelligence augmentation, and data-driven decision-making.
AI techniques are gaining rapid adoption across the civil engineering domain spanning structural design, analysis, construction planning, project controls, asset inspections, maintenance, and more. AI empowers engineers to optimize workflows, uncover insights from information, predict failures, achieve precision, simulate realistic models, and evaluate vast combinations based on machine learning. The integration of AI in civil engineering is poised to transform the future of civil infrastructure.
Evolution of AI in Civil Engineering
Some pioneering efforts included expert systems for construction planning in the 70s, neural networks for structural design in the 80s, case-based reasoning for cost estimation in the 90s, etc. While restricted by limited data and computing power, these niche applications provided glimpses into AI's potential.
Over the past decade, the convergence of big data and AI, advanced algorithms, and affordable computing has enabled AI breakthroughs. Civil engineering is now actively leveraging techniques like machine learning, computer vision, and natural language processing at scale to create value.
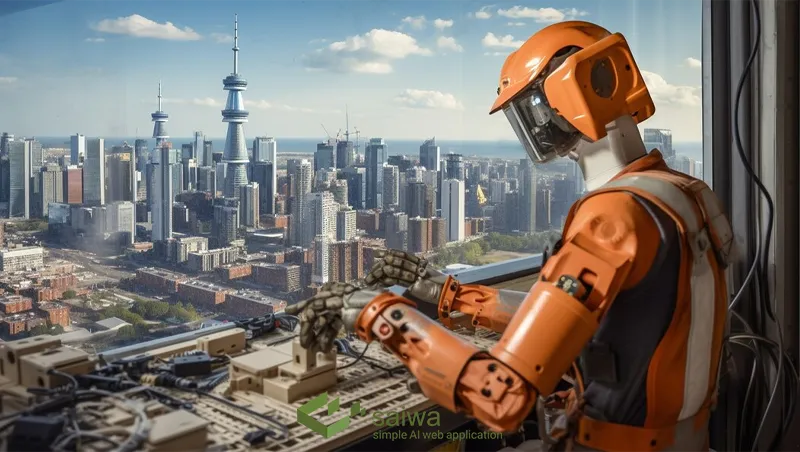
Key AI Techniques in Civil Engineering
The major categories of AI in civil engineering approaches for tackling complex analytical and design problems include:
Machine Learning
Statistical models and neural networks are capable of learning from data patterns to complete specified tasks like classification, prediction, optimization, computer vision, and natural language processing without explicit programming. Algorithms self-improve through continuous model training and validation.
Deep Learning
Advanced neural network architectures like convolutional and recurrent networks that contain multiple layers automatically learn hierarchical feature representations directly from input data like sensor measurements, videos, texts, and images to develop insights.
Reinforcement Learning
Agents interact dynamically with environments by testing actions to maximize a cumulative numerical reward signal indicative of solving a problem optimally instead of static input-output dataset mapping. Enables adaptive behavior.
Applications of AI in Civil Engineering
Major application areas where AI techniques are enhancing processes across civil engineering domains encompass:
Design and Project Planning
Generative design algorithms suggest design alternatives, automate repetitive drawing tasks, perform code compliance checks, and simulate constructability. Multi-objective optimization balances costs, safety factors, aesthetics, environmental impact, and other aspects in finalizing architectural and construction plans based on constraints. AI also creates project schedules balancing time, budget, and resource considerations through optimization.
Read Also: The Rise of AI in Architecture | Innovations and Applications
Construction Management
Monitoring hundreds of overlapping construction tasks against a dynamic baseline requires AI. Computer vision analyzes images to inspect progress and asset condition. Natural language processing extracts insights from textual reports. Predictive analytics identify schedule overruns and cost risks by considering historical data and simulations. Chatbots handle contractor queries.
Structural Analysis and Optimization
Analyzing intricate structures involves modeling thousands of load combinations to ensure integrity. Machine learning and neural networks enable accurate stress and strain simulation using sensor data. Topology optimization determines efficient shapes and internal structures. AI selects appropriate materials, dimensions, and members for optimal structural performance adhering to safety norms.
Geotechnical Engineering
Estimating suitable foundation dimensions and types for underlying soil conditions is key in construction. AI correlates soil properties from geophysical data to recommend appropriate foundations. Computer vision analyzes satellite imagery to create high-resolution terrain maps indicating subsurface composition, drainage patterns, etc. to guide design. AI optimizes drilling, sampling, and testing processes.
Genetic Algorithms
Heuristic global search techniques inspired by biological evolution mechanisms of reproduction, mutation, recombination, and selection are applied to arrive at near-optimal solutions faster for high dimensionality and complexity.
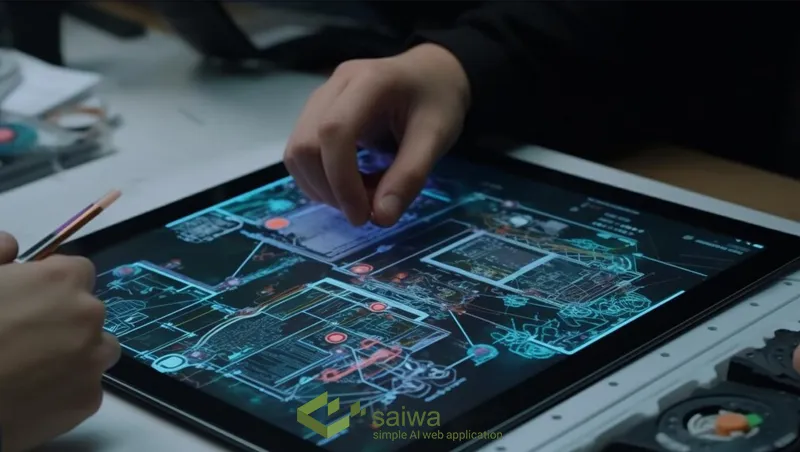
Real-World Case Studies
Here are a few examples highlighting the capabilities unlocked by AI in civil engineering needs:
AI-assisted Bridge Inspections
Computer vision algorithms are applied to detect concrete cracks, steel corrosion, and deformation across vast bridge infrastructure from drone and ground-level images more accurately than human visual scrutiny. This prioritizes maintenance actions. 3D scene analysis and positional tagging enrich functional deterioration assessments.
Automated Construction Site Monitoring
Panoramic cameras, aerial images from drones, and 360-degree photos captured at regular intervals are analyzed by computer vision software to track actual progress against construction schedules. NLP parses logs to check work completion. ML models forecast final cost and completion dates for early interventions.
Machine Learning for Tunnel Structure Analysis
Laser scans, ground-penetrating radar, and physical sensing provide extensive raw measurements of existing tunnel conditions. Deep neural networks integrate this multi-modal sensory data to detect leaks, model structural stability, evaluate rehabilitation needs, and design supportive structures optimized for surrounding soil/rock conditions using simulation training.
Deep Learning for Smart Traffic Control
Video streams processed by computer vision fuel deep reinforcement learning algorithms to optimize complex traffic light timing schedules based on real-time lane-specific vehicle flows and pedestrian movement to reduce congestion at intersections. Traffic patterns are forecast using historical trends to plan schedules.
Benefits of AI in Civil Engineering
Applying AI in civil engineering domains is creating profound value in various ways:
Greater efficiency
Automating repetitive and routine analytical tasks significantly improves individual productivity and project team velocity. Generative design shortcuts lengthy drawing and modeling steps.
Superior designs
Multi-criteria optimization produces infrastructure plans meeting specialized performance, safety, and sustainability needs surpassing human capabilities.
Enhanced quality
Continuous computer vision monitoring across project stages minimizes defects requiring rework through early detection and correction thereby improving construction quality.
Reliable inspection
Automated AI analysis assists engineers in inspecting vast numbers of roads, bridges, tunnels, dams, and buildings to accurately estimate rehabilitation needs and prioritize maintenance, especially across aging infrastructure.
Improved sustainability
Optimized structural and geotechnical designs avoid over-engineering and reduce material costs and environmental impact while preserving resilience and longevity.
Better predictability
Analysis of historical trends and real-time project data enables reliable forecasts of delays, accidents, budget overruns, and resource needs for preventive planning.
Informed decision making
AI extracts insights from exponentially increasing engineering data sources that are impossible to fully assimilate manually guiding superior judgments.
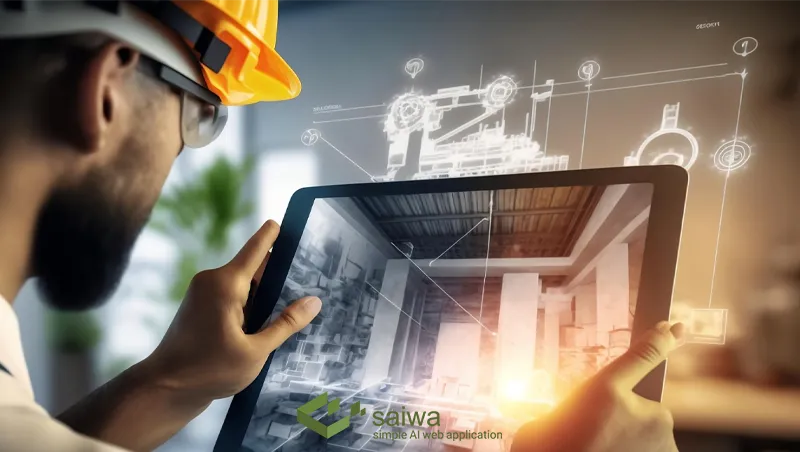
Conclusion
Civil infrastructure forms the backbone of economic functions making the role of civil engineers pivotal. Integrating AI techniques like machine learning, computer vision, and neural networks facilitates tackling complex analytical challenges, accelerates design cycles, assists critical decision-making, and introduces intelligent automation. AI in civil engineering adoption is modernizing engineering practices to improve quality, safety, and sustainability across planning, building, and managing civil structures.
While AI capabilities continue evolving, civil engineering companies need sound data management, tool strategy, and pilot testing before large-scale commitment. Formulating specialized AI models using available sensing data and domain expertise promises to amplify engineering capabilities extraordinarily. The future will see a broad proliferation of AI transforming construction flows and asset maintenance through complementing human ingenuity, augmenting complex analysis, and enabling data-driven actions in civil engineering.