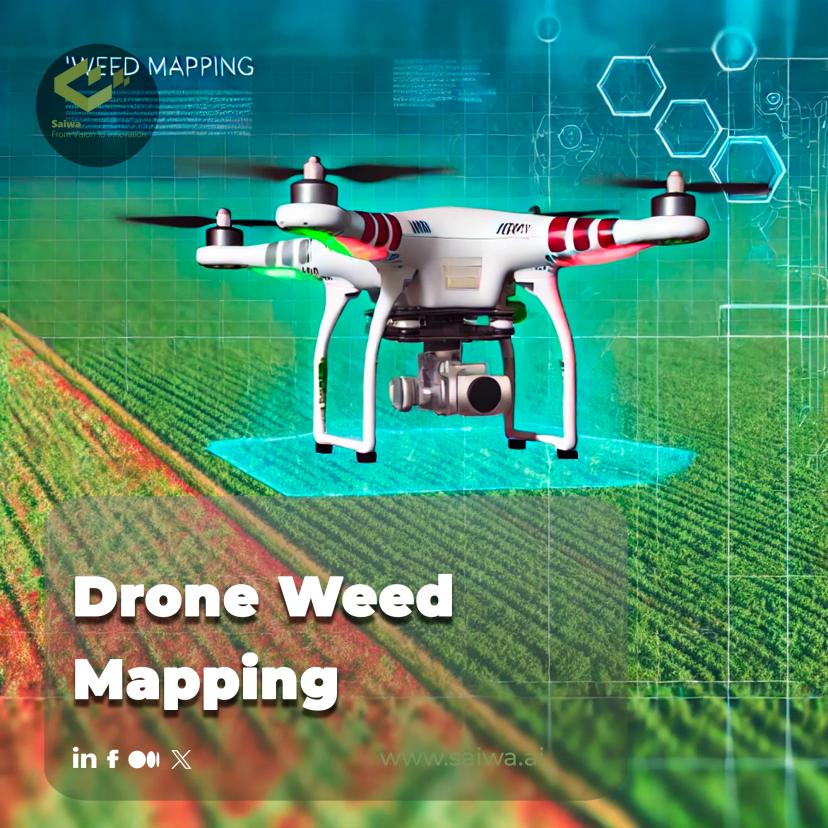
Drone Weed Mapping | A Game-Changer for Precision Agriculture
In the realm of modern agriculture, precision farming techniques are rapidly gaining traction for their ability to optimize resource utilization and enhance crop yields. Among these cutting-edge technologies, drone weed mapping has emerged as a game-changer, offering unprecedented accuracy and efficiency in identifying and managing weed infestations. This comprehensive article delves deep into the intricacies of drone weed mapping, exploring its mechanisms, applications, benefits, and future potential.
A principal contributor to the advancement of drone-based precision agriculture is Sairone, an integrated AI/ML drone platform developed by Saiwa. Sairone employs artificial intelligence and machine learning to enhance the efficiency of diverse agricultural procedures, including weed mapping. By integrating with a variety of drones, Sairone augments the capabilities of drone-based weed mapping systems through the application of sophisticated data analytics and predictive modeling. This enables more precise detection of weeds, the formulation of targeted treatment recommendations, and enhanced overall efficiency in the management of weed infestations.
This article will examine the fundamental principles, essential elements, and prospective developments of drone-based weed mapping, emphasizing both the prospective advantages and the prospective challenges inherent to the pursuit of more intelligent and sustainable agricultural practices.
What is Drone Weed Mapping?
As the term "drone weed mapping" implies, this process entails the utilization of unmanned aerial vehicles (UAVs), colloquially termed "drones," outfitted with sophisticated sensors and imaging technologies to generate detailed maps of weed distribution within agricultural fields. The resulting maps offer invaluable insights into weed density, species identification, and location-specific infestation levels, thereby enabling farmers to make informed decisions regarding the most appropriate weed control strategies.
The conventional methods employed for the mapping of weeds frequently entail manual scouting, a process that is inherently labor-intensive, time-consuming, and susceptible to human error. In contrast, drone weed mapping provides a rapid, automated, and data-driven approach that markedly enhances the precision and efficacy of weed detection and management.
Read Also: Leveraging Drones in Agriculture for a New Era of Data-Driven Farming
How does Drone Weed Mapping work?
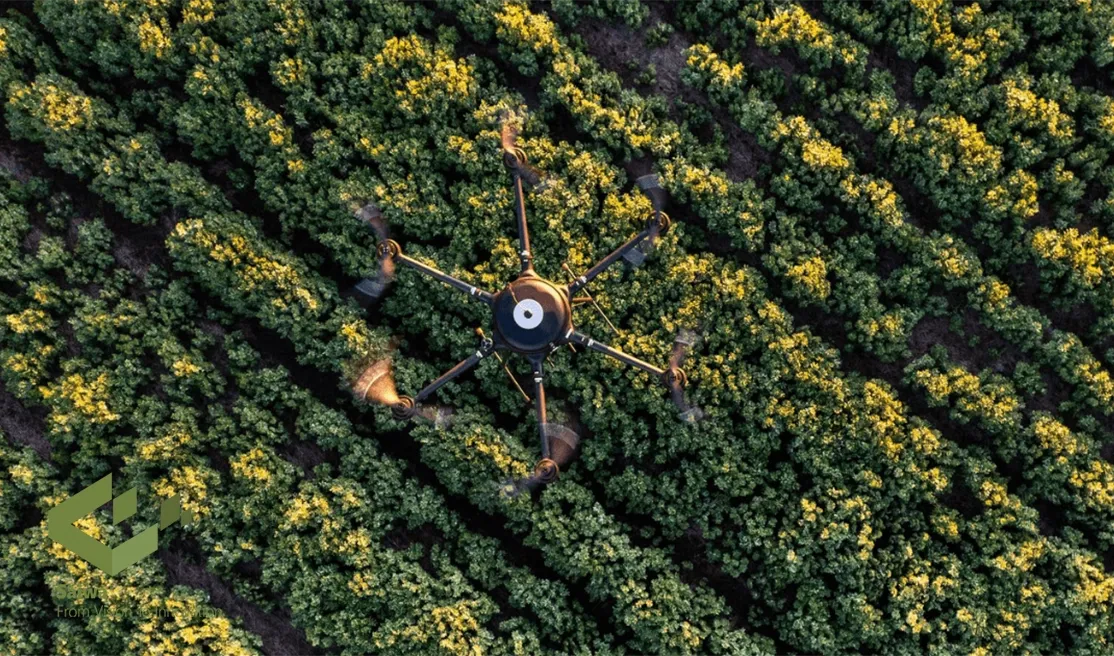
The process of drone weed mapping can be broadly categorized into two main stages: data acquisition and image processing and analysis.
Data Acquisition
Flight Planning: The first step involves meticulously planning the drone's flight path over the target field. This involves defining flight altitude, overlap between images, and flight speed to ensure comprehensive coverage and optimal image resolution.
Sensor Selection: The choice of sensors and imaging technologies depends on the specific requirements of the mapping project. Multispectral cameras, thermal imaging sensors, and LiDAR systems are commonly employed for weed mapping.
Data Collection: Once the flight plan is established and sensors are selected, the drone autonomously flies over the field, capturing high-resolution images or data points according to the predefined parameters. These images or data points form the raw data for subsequent analysis.
Image Processing and Analysis
Image Stitching: The individual images captured by the drone are stitched together using specialized software to create a single, seamless orthomosaic image of the entire field. This process corrects for distortions and ensures accurate spatial representation.
Weed Detection: Sophisticated algorithms and machine learning models are employed to analyze the processed images and identify the presence of weeds. These algorithms differentiate weeds from crops based on various factors such as color, texture, and shape.
Weed Classification: In many cases, the drone mapping system can go beyond mere detection and classify weeds into different species. This information is crucial for tailoring herbicide treatments and implementing targeted weed control measures.
Map Generation: The final output of the image processing and analysis stage is a detailed weed map that highlights weed-infested areas, often color-coded to represent weed density or species type. These maps provide farmers with a clear visualization of weed distribution within their fields.
Weed Detection Principles
The efficacy of drone-based weed mapping is contingent upon the capacity of the system to accurately differentiate between weeds and crops. The differentiation between weeds and crops is achieved through the utilisation of a range of weed detection principles, including:
Spectral Signature Analysis: Different plant species reflect and absorb light in unique ways, creating distinct spectral signatures. Multispectral cameras capture images across multiple wavelengths, allowing algorithms to analyze these spectral signatures and differentiate weeds from crops.
Texture Analysis: Weeds often exhibit different textures compared to crops. Image processing algorithms can identify these textural variations to distinguish weeds from the surrounding vegetation.
Shape and Size Analysis: Some weed species have characteristic shapes or sizes that set them apart from crops. Algorithms can be trained to recognize these morphological features for accurate weed detection.
Machine Learning: Advanced machine learning models are trained on vast datasets of labeled images, enabling them to learn the subtle differences between weeds and crops and improve their detection accuracy over time.
Sensors and Imaging Technologies
A variety of sensors and imaging technologies are employed in drone weed mapping, each offering unique capabilities and advantages:
Thermal Imaging Sensors
Heat Signature Detection: Thermal cameras detect infrared radiation emitted by objects, allowing them to create images based on temperature differences. Weeds often exhibit different temperature profiles compared to crops, particularly during specific growth stages or times of day.
Stress Detection: Thermal imaging can also reveal signs of stress in plants, such as water stress or disease, which can manifest as temperature variations. This information can be valuable for identifying weed-infested areas that might require attention.
Read More: Introduction to Drone Thermal Inspection
LiDAR Technology
3D Point Cloud Generation: LiDAR (Light Detection and Ranging) systems emit laser pulses and measure the time it takes for the reflected light to return, creating highly accurate 3D point clouds of the scanned environment.
Canopy Height Mapping: LiDAR data can be used to generate detailed canopy height maps, revealing variations in plant height. This information can be useful for identifying weeds that are taller than the surrounding crops.
Biomass Estimation: LiDAR-derived data can also be used to estimate plant biomass, providing insights into crop health and potential yield. This information can be helpful for assessing the impact of weeds on crop growth.
Read More: Drone LiDAR Mapping | The Future of Data Acquisition
Multispectral Cameras
Spectral Band Analysis: Multispectral cameras capture images across multiple specific wavelengths, including those beyond the visible spectrum. This allows for the analysis of spectral signatures, which can differentiate weeds from crops based on their unique light reflectance properties.
Vegetation Indices Calculation: Multispectral data enables the calculation of various vegetation indices, such as the Normalized Difference Vegetation Index (NDVI), which provide insights into plant health and chlorophyll content. These indices can highlight areas of stress or low vigor, potentially indicating weed infestations.
Hyperspectral Cameras
Detailed Spectral Information: Hyperspectral cameras capture images across hundreds of narrow spectral bands, providing a wealth of detailed spectral information. This allows for highly accurate weed detection and classification, even at early growth stages.
Weed Stress Detection: Hyperspectral imaging can detect subtle changes in plant physiology, such as those caused by herbicide resistance or nutrient deficiencies. This information can be invaluable for tailoring weed management strategies.
Software Solutions for Drone Weed Mapping
The considerable quantity of data produced by drone-based weed mapping necessitates the utilisation of sophisticated software solutions for the processing, analysis, and interpretation of said data. A number of software packages and platforms have been developed with the specific aim of meeting the requirements of drone-based weed management.
Image Processing Software
Orthomosaic Generation: Software like Pix4D, Agisoft Metashape, and DroneDeploy specialize in stitching together individual drone images to create seamless orthomosaic images of entire fields. These orthomosaics provide a geographically accurate base map for further analysis.
Geometric Correction: Image processing software corrects for distortions and inaccuracies in drone images caused by factors like camera tilt, terrain variations, and lens distortion. This ensures accurate spatial representation and reliable measurements.
Cloud-Based Platforms for Data Management
Data Storage and Access: Cloud-based platforms like FieldView, Granular, and Climate FieldView offer secure and scalable storage solutions for the large datasets generated by drone mapping. These platforms allow farmers to access their data from anywhere with an internet connection.
Collaboration and Sharing: Cloud platforms facilitate collaboration among stakeholders, allowing farmers to share their data with agronomists, crop consultants, or other trusted advisors for insights and recommendations.
Data Visualization and Reporting: These platforms provide user-friendly interfaces for visualizing data, generating reports, and creating maps that highlight key insights. This enables farmers to easily interpret the data and make informed decisions.
Weed Detection and Mapping Software
Automated Weed Identification: Specialized software solutions like Taranis, PrecisionHawk, and Terraview utilize advanced algorithms and machine learning models to automatically detect and map weeds in drone imagery.
Weed Species Classification: Some software packages can classify weeds into different species, providing valuable information for targeted herbicide selection and application.
Prescription Map Generation: Based on the detected weed distribution and density, software can generate prescription maps that guide variable-rate herbicide applications, optimizing weed control while minimizing chemical usage.
Applications in Precision Agriculture
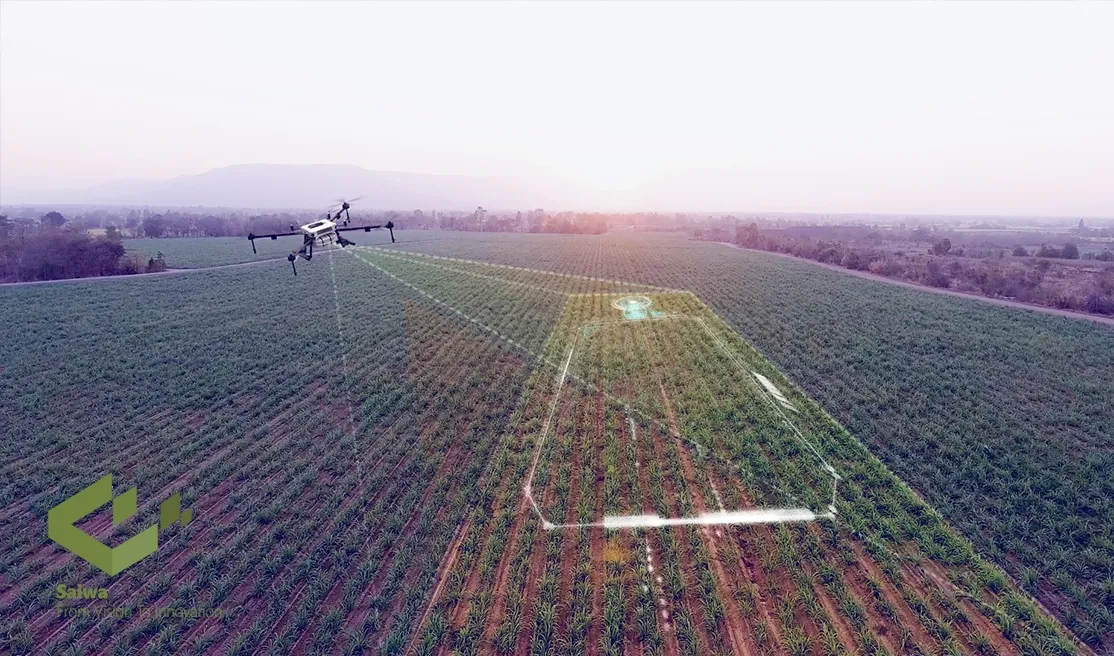
The application of drone-based weed mapping has the potential to revolutionize precision agriculture, offering a transformative approach to weed management and crop production optimization for farmers.
Crop Health Monitoring
Early Weed Detection: Drones can detect weeds at their early growth stages, when they are most vulnerable to control measures and before they significantly impact crop yield.
Stress Detection and Diagnosis: Multispectral and hyperspectral imaging can reveal subtle signs of stress in crops caused by factors like nutrient deficiencies, pests, or diseases. Early detection of these issues allows for timely interventions.
Yield Prediction and Analysis
Estimating Yield Potential: By analyzing crop health and vigor throughout the growing season, drone-derived data can be used to predict yield potential with greater accuracy.
Identifying Yield-Limiting Factors: Drone mapping can pinpoint areas within fields that are underperforming, allowing farmers to investigate potential causes such as weed pressure, soil compaction, or nutrient imbalances.
Variable-Rate Herbicide Application
Precision Weed Control: Drone-generated weed maps enable the creation of prescription maps that guide variable-rate herbicide applications. This means applying herbicides only where and when needed, minimizing chemical usage, reducing costs, and minimizing environmental impact.
Herbicide Resistance Management: By targeting herbicide applications precisely, farmers can help slow the development of herbicide resistance in weed populations.
Crop Scouting and Management
Efficient Scouting: Drones can cover vast areas quickly, significantly reducing the time and labor required for manual crop scouting.
Targeted Interventions: Drone mapping allows farmers to focus their scouting efforts on specific areas identified as potentially problematic, optimizing resource allocation.
Read More: Revolutionizing Agriculture with UAV Precision Agriculture
Benefits of Using Drones in Weed Mapping
The adoption of drone technology for weed mapping offers numerous advantages over traditional methods:
Increased Efficiency and Speed: Drones can cover large areas much faster than manual scouting, enabling timely weed detection and management.
Enhanced Accuracy and Precision: Drone-based sensors and imaging technologies provide high-resolution data, leading to more accurate weed maps and targeted interventions.
Reduced Labor Costs: Automation through drones reduces the need for manual labor, lowering labor costs associated with scouting and weed control.
Improved Crop Yields: Timely and precise weed management leads to healthier crops and higher yields.
Environmental Sustainability: Reduced herbicide usage minimizes the environmental impact of weed control practices.
Data-Driven Decision-Making: Drone-generated data provides farmers with valuable insights to make informed decisions regarding weed management strategies.
Conclusion
The advent of drone weed mapping is transforming the manner in which farmers address the issue of weeds, inaugurating a new era of precision agriculture that is defined by efficiency, accuracy, and sustainability. By providing detailed insights into weed distribution and crop health, drone technology empowers farmers to make data-driven decisions, optimize resource utilization, and enhance crop yields while minimizing their environmental footprint. As drone technology continues to advance and become more accessible, it is anticipated that its role in precision agriculture will continue to expand, thereby paving the way for a more sustainable and productive future for the agricultural industry.