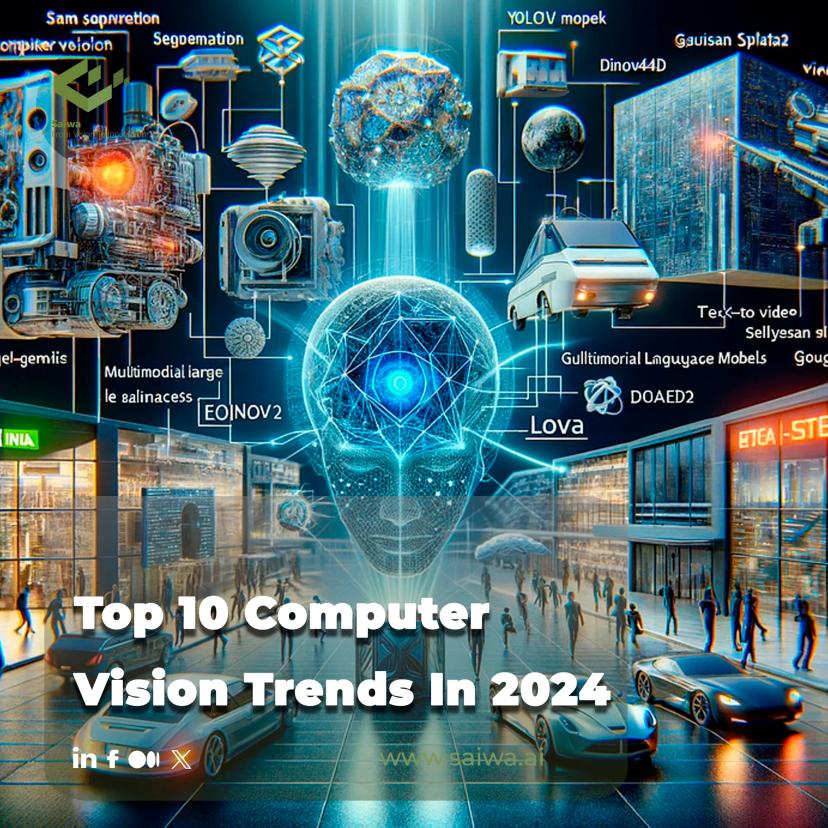
Top 10 Transformative Computer Vision Trends In 2024
The field of artificial intelligence (AI) is undergoing a period of rapid evolution, with significant advances being made in the capabilities of machines to perceive and understand their surroundings. One of the most transformative forces in this landscape is computer vision, which enables machines to "see" and interpret visual information in ways that were previously the domain of science fiction. At the forefront of these advancements is Saiwa, an AI tool that integrates a multitude of cutting-edge computer vision technologies, including, but not limited to, object detection, recognition, and segmentation. By leveraging these innovations, Saiwa is facilitating the transformation of industries and the evolution of human-computer interaction.
as we step into 2024, computer vision is positioned to drive substantial change across a range of domains, opening new avenues and enhancing the ways in which we engage with technology. This article examines the top 10 trends that are likely to influence the future of computer vision and their potential implications for our lives.
What is Computer Vision?
The objective of computer vision is to replicate the remarkable capabilities of human vision through the lens of artificial intelligence. It enables computers to "see" and make sense of images and videos, extracting meaningful information, recognizing patterns, and making informed decisions based on visual input. This intricate process involves a complex interplay of algorithms, mathematical models, and computational techniques designed to mimic the human visual system's ability to perceive depth, recognize objects, and understand scenes.
The process of computer vision typically involves several key steps:
Image Acquisition: Capturing visual data from the real world using various imaging devices such as cameras, sensors, or medical imaging equipment. This step involves converting the physical scene or object into a digital representation that can be processed by a computer.
Image Preprocessing: Enhancing the quality of the acquired images by reducing noise, adjusting brightness and contrast enhancement, and correcting for distortions. This step prepares the image for subsequent analysis by mitigating potential issues that could hinder accurate feature extraction.
Feature Extraction: Identifying and extracting salient features from the preprocessed images, such as edges, corners, textures, and shapes. These features serve as building blocks for higher-level image understanding. This step involves identifying the most informative aspects of the image, reducing its complexity while preserving essential information.
Object Detection and Recognition: Employing basic machine learning algorithms to identify and classify objects within images or videos. This could involve recognizing faces and Object Detection identifying different species of plants, or detecting anomalies in medical scans. This step often involves training models on large datasets to enable them to accurately recognize specific objects or patterns.
Scene Understanding: Interpreting the overall context and relationships between objects within a scene. This might involve understanding the layout of a room, recognizing human actions, or analyzing traffic patterns. This step goes beyond individual object recognition to interpret the scene holistically, inferring relationships and interactions between objects.
Why Does Computer Vision Matter?
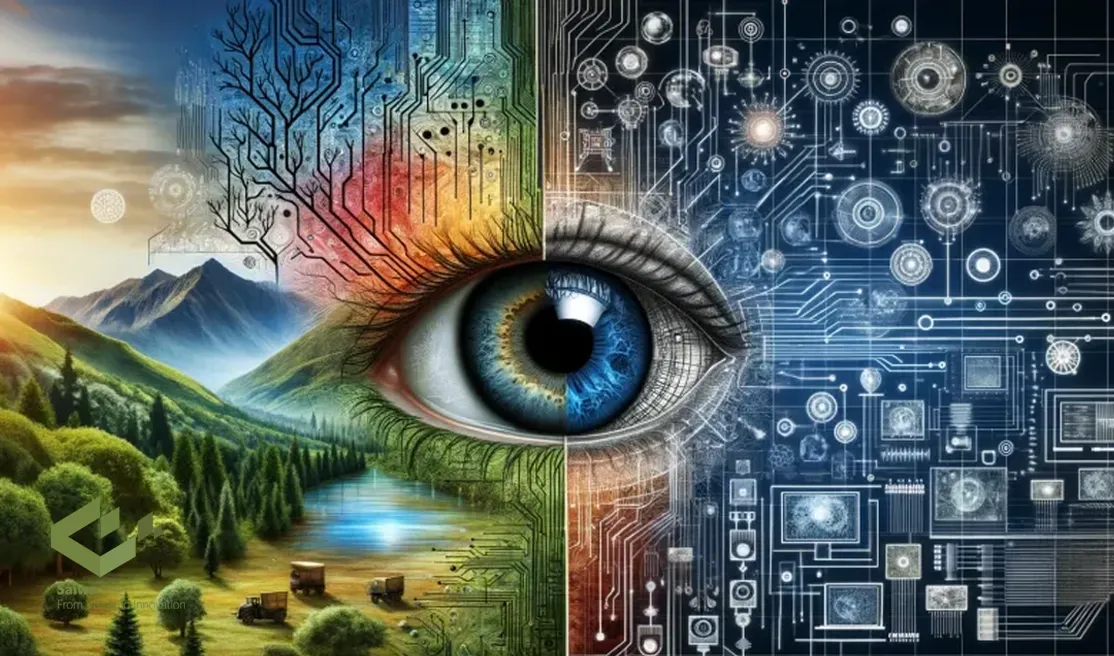
Computer vision is not merely a technological marvel; it has profound implications for virtually every aspect of our lives. The automation of tasks that previously required human vision, coupled with the capacity to uncover insights within vast troves of visual data, positions computer vision as a transformative force across industries.
Automation and Efficiency
Computer vision streamlines processes and boosts efficiency by automating tasks previously performed by humans. This includes applications like quality control in manufacturing, where computer vision systems can inspect products for defects with greater speed and accuracy than human inspectors. In healthcare, robotic surgery systems guided by computer vision enable surgeons to perform minimally invasive procedures with enhanced precision and dexterity. And in transportation, autonomous navigation systems rely on computer vision to perceive their surroundings, making real-time decisions to navigate safely and efficiently.
Enhanced Decision-Making
By extracting valuable insights from visual data, computer vision empowers businesses and organizations to make more informed decisions. This ranges from optimizing supply chains based on real-time inventory analysis gleaned from camera feeds to personalizing customer experiences through targeted advertising informed by visual cues and preferences. Computer vision enables businesses to leverage the wealth of information embedded within visual data, unlocking new avenues for optimization and growth.
Improved Safety and Security
Computer vision plays a crucial role in enhancing safety and security in various domains. This encompasses applications like facial recognition for access control, providing an additional layer of security for sensitive facilities. Surveillance systems equipped with computer vision can detect suspicious activities and aid in crime prevention. And in the realm of transportation, driver assistance systems utilize computer vision to alert drivers of potential hazards, helping to prevent accidents and save lives.
Accessibility and Inclusion
Computer vision has the potential to bridge gaps and promote accessibility for individuals with disabilities. This includes applications like assistive technologies for visually impaired individuals, enabling them to navigate their surroundings and interact with digital content more easily. Real-time language translation powered by computer vision facilitates communication across language barriers, fostering greater understanding and inclusion.
Read More: Exploring Diverse Computer Vision Applications
Computer Vision Trends for 2024
As computer vision continues its rapid evolution, several key trends have emerged that are likely to shape the technological landscape in 2024 and beyond.
Generative AI
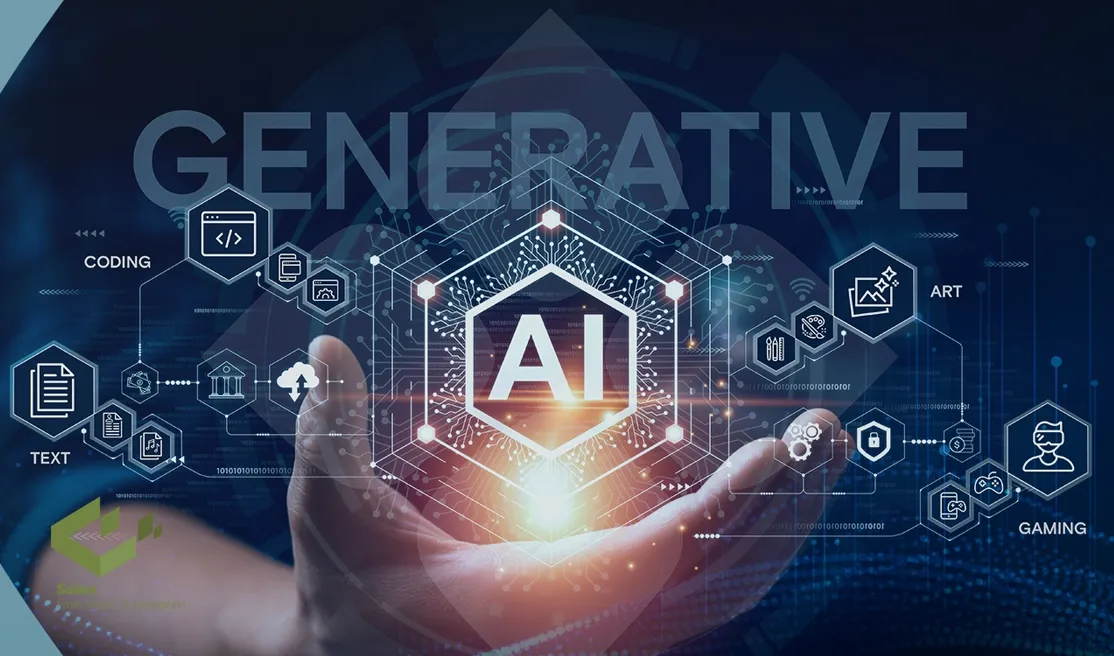
Generative AI, a burgeoning field within artificial intelligence, focuses on enabling machines to create new content, such as images, videos, text, and even music. When applied to computer vision, generative AI unlocks exciting possibilities for image synthesis, editing, and enhancement.
Realistic Image Generation: Generative AI models, like Generative Adversarial Networks (GANs), possess the remarkable ability to generate highly realistic images that are often indistinguishable from real photographs, blurring the lines between artificiality and reality. This has significant implications for various industries, including entertainment, advertising, and design, where the demand for high-quality, synthetic imagery is ever-increasing.
Image-to-Image Translation: Computer vision models have achieved a new level of sophistication, enabling them to effectively translate images from one domain to another. This transformative capability allows for tasks such as converting simple sketches into photorealistic images or magically transforming daytime photos into captivating nighttime scenes, opening up a world of possibilities in areas like image editing and artistic expression.
Image Super-Resolution: AI is pushing the boundaries of image enhancement, enabling the generation of high-resolution images from their low-resolution counterparts. This breakthrough has far-reaching applications in fields like medical imaging, where enhancing the details in medical scans can aid in diagnosis; satellite imagery analysis, where sharper images reveal finer details of our planet; and forensic science, where enhancing the resolution of blurry images can provide crucial evidence.
Read Also: Top AI Trends in 2024 | Future Of AI
Multimodal AI
Traditionally, AI models have excelled at processing a single modality of data, such as text or images. However, the real world is inherently multimodal, with information often conveyed through a combination of text, images, sounds, and even sensory data. Multimodal AI aims to bridge this gap by developing models capable of processing and understanding information from multiple modalities simultaneously.
Enhanced Image Captioning: By seamlessly combining image understanding with the nuances of natural language processing, multimodal AI enables more accurate and contextually rich image captioning. This means that images can be described in greater detail, capturing subtle nuances and emotions that might be missed by unimodal models, making them more accessible and informative.
Visual Question Answering: Multimodal AI models have attained a level of visual and linguistic understanding that allows them to answer open-ended questions about images. This remarkable capability demonstrates a deeper comprehension of both the visual content and the subtle nuances of human language, paving the way for more natural and intuitive interactions with machines.
Cross-Modal Retrieval: Multimodal AI breaks down the barriers between different modalities, facilitating the search for information across text, images, and more. This means users could search for images using descriptive text phrases or vice versa, making information retrieval more flexible and intuitive.
Computer Vision in Healthcare
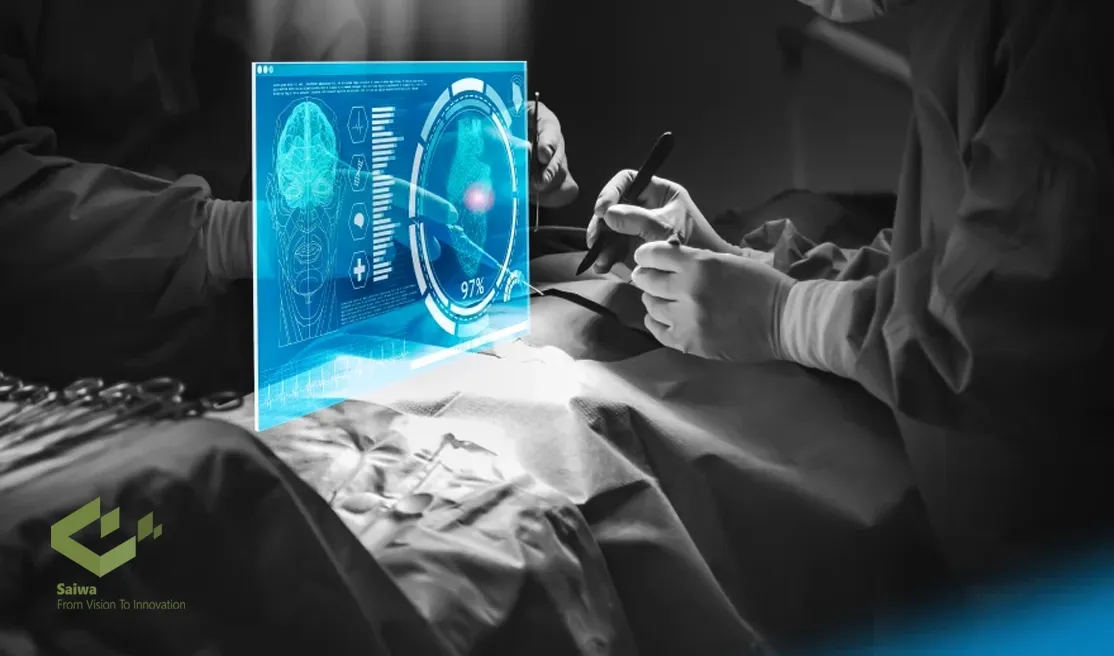
The healthcare industry is undergoing a profound transformation fueled by the integration of artificial intelligence, with computer vision playing a pivotal role in enhancing diagnostics, treatment planning, and patient care.
Medical Image Analysis: Computer vision algorithms have emerged as invaluable tools in medical image analysis, excelling at analyzing complex medical images like X-rays, CT scans, and MRIs. These algorithms assist radiologists in detecting subtle anomalies, classifying tumors with higher accuracy, and assessing treatment responses more effectively, ultimately leading to faster and more accurate diagnoses.
Automated Disease Diagnosis: AI-powered systems are being developed to assist medical professionals in diagnosing diseases based on medical images. This has the potential to significantly improve diagnostic accuracy, especially in cases where human interpretation can be subjective, and enable earlier detection of diseases, leading to more favorable patient outcomes.
Robotic Surgery: Computer vision is playing a crucial role in advancing the field of robotic surgery, guiding robotic surgical systems with enhanced precision, dexterity, and control. This technology empowers surgeons to perform minimally invasive procedures with greater accuracy, reducing patient trauma and improving surgical outcomes.
Read More: Transformative Potential of Computer Vision in Healthcare
Edge Computing and Lightweight Architectures
Traditionally, computer vision tasks have relied heavily on powerful cloud-based servers to process vast amounts of data. However, the rise of edge computing is shifting this paradigm by bringing computation closer to the data source, enabling real-time processing and reducing latency.
On-Device Processing: The development of lightweight computer vision models is enabling on-device processing, where these models can run directly on devices like smartphones, cameras, and drones. This eliminates the need to rely on cloud connectivity for image analysis, enabling real-time decision-making and opening up new possibilities for applications in remote areas or situations where low latency is critical.
Reduced Latency: Edge computing minimizes the time delay associated with transferring data to the cloud for processing. This is crucial for applications that require instantaneous responses, such as autonomous vehicles that need to make split-second decisions to avoid obstacles or industrial automation systems that require real-time feedback.
Enhanced Privacy: By processing data locally on devices, edge computing reduces the need to transmit sensitive information to the cloud. This enhances data privacy and security, addressing concerns about data breaches and unauthorized access, which is particularly important in industries dealing with sensitive personal information.
Autonomous Vehicles
Self-driving cars represent one of the most anticipated applications of artificial intelligence, with computer vision serving as their eyes on the road, enabling them to perceive their surroundings, make navigational decisions, and avoid obstacles.
Object Detection and Tracking: Computer vision algorithms are the foundation of autonomous navigation, enabling self-driving vehicles to detect and track objects in their vicinity, including pedestrians, other vehicles, and traffic signals. This real-time object recognition is crucial for ensuring safe navigation and avoiding collisions.
Lane Keeping and Lane Changing: Computer vision systems play a critical role in keeping autonomous vehicles safely within their designated lanes. By analyzing lane markings and monitoring surrounding traffic, these systems assist vehicles in maintaining their lane position and performing safe lane changes, contributing to the overall safety and efficiency of autonomous driving.
Traffic Sign Recognition: Autonomous vehicles rely on computer vision to accurately recognize and interpret traffic signs, ensuring compliance with traffic regulations. This ability to understand and respond to traffic signs is essential for navigating roads safely and legally.
Read More: The Role of Object Detection for Autonomous Vehicles
Detecting Deep Fakes
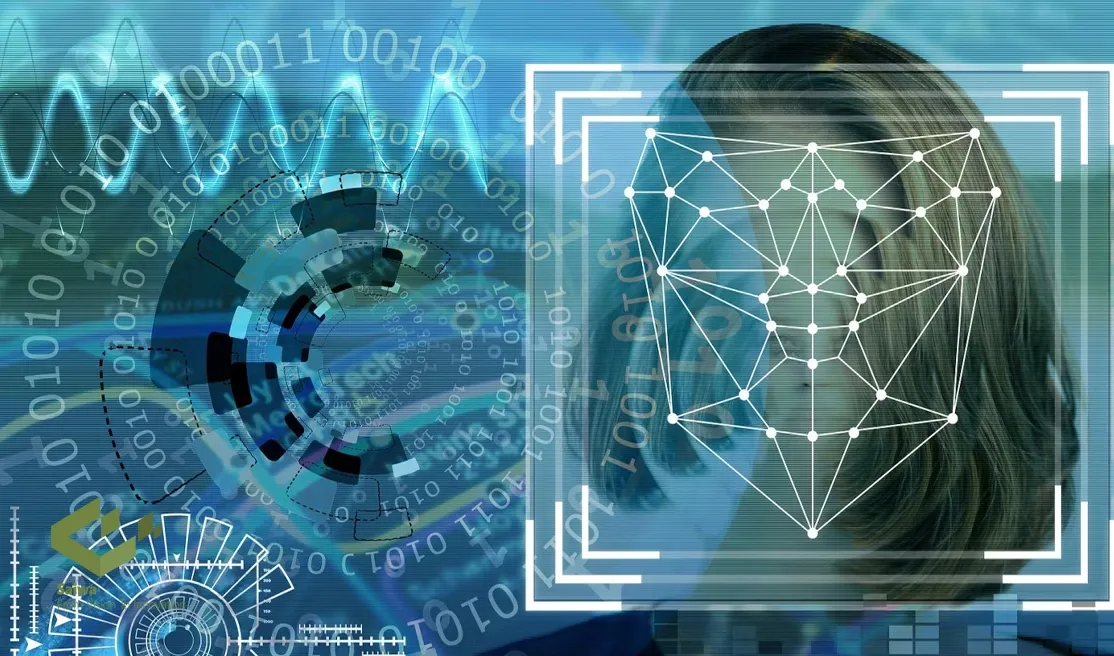
The proliferation of deep learning techniques has given rise to "deep fakes," highly realistic but fabricated videos or images that can be used to spread misinformation, manipulate public opinion, or damage reputations. Detecting these deep fakes is crucial for combating their malicious use.
Artifact Detection: Deep fake detection algorithms are being developed to identify the subtle artifacts or inconsistencies that are often introduced during the deep fake generation process. These artifacts can include unnatural blinking patterns, inconsistencies in lighting and shadows, or subtle distortions in facial movements, providing clues that can help distinguish real footage from fabricated content.
Behavioral Analysis: Analyzing the behavior and mannerisms of individuals in videos is another approach to deep fake detection. Deep fakes might exhibit unnatural facial expressions, body language, or inconsistencies in speech patterns that can betray their artificial nature.
Provenance Verification: Tracking the origin and history of images and videos is crucial for verifying their authenticity. By establishing a clear chain of custody and identifying any potential points of manipulation, provenance verification can help expose deep fakes and other forms of content forgery.
Augmented Reality
Augmented reality (AR) overlays digital information in the real world, enhancing our perception and interaction with our surroundings. Computer vision plays a crucial role in enabling AR experiences by understanding the environment and tracking the user's position.
Object Recognition and Tracking: AR applications rely on computer vision to recognize and track objects in the real world, enabling the seamless integration of digital content with the physical environment. For instance, AR furniture shopping apps allow users to visualize how furniture would look in their homes by overlaying 3D models onto live camera feeds, providing a realistic preview before making a purchase.
Simultaneous Localization and Mapping (SLAM): SLAM techniques empower AR devices to map their surroundings and track their own position within the environment, creating immersive and interactive experiences. This technology allows AR applications to understand the geometry and spatial relationships of the physical world, enhancing the realism and interactivity of AR experiences.
Facial Recognition and Tracking: The popular AR filters and lenses used on social media platforms rely heavily on facial recognition and tracking to overlay digital effects onto users' faces in real time. These filters can enhance facial features, add virtual accessories, or create playful distortions, showcasing the entertaining and engaging aspects of AR technology.
Read More: Computer Vision in Augmented Reality
Satellite Computer Vision
The increasing availability of high-resolution satellite imagery has opened up new frontiers for computer vision applications, enabling us to monitor and understand our planet on a global scale.
Environmental Monitoring: Satellite computer vision provides invaluable data for environmental monitoring, allowing us to track deforestation rates, monitor the melting of glaciers, and assess the impact of natural disasters. This data is crucial for understanding the effects of climate change, managing natural resources, and developing effective conservation strategies.
Urban Planning and Development: Analyzing satellite imagery helps urban planners monitor urban sprawl, optimize transportation networks, and plan for sustainable city development. By understanding how cities are growing and changing, planners can make more informed decisions about infrastructure, housing, and environmental protection.
Agricultural Monitoring: Satellite imagery analysis has become an indispensable tool for modern agriculture, enabling farmers to monitor crop health, optimize irrigation strategies, and estimate yields with greater accuracy. This data-driven approach to agriculture helps improve productivity, conserve resources, and ensure food security.
3D Computer Vision
While traditional computer vision has primarily focused on 2D images, 3D computer vision is gaining momentum, enabling machines to perceive depth and understand the three-dimensional structure of objects and scenes.
Object Reconstruction: 3D computer vision techniques have revolutionized object reconstruction, allowing for the creation of highly detailed 3D models from 2D images or videos. This technology has applications in various fields, including robotics, where robots can use 3D models to interact with objects more effectively; virtual reality, where realistic 3D environments enhance immersion; and industrial design, where 3D models facilitate product prototyping and visualization.
Depth Sensing and Mapping: Sensors like LiDAR and structured light cameras are essential for capturing depth information, enabling the creation of accurate 3D maps of environments. These maps are crucial for applications like autonomous navigation, where vehicles need to understand the three-dimensional structure of their surroundings, and augmented reality, where virtual objects can be placed realistically in the real world.
Gesture Recognition: 3D computer vision significantly enhances gesture recognition capabilities, allowing for more natural and intuitive human-computer interaction. By understanding the depth and movement of hands and fingers, computers can interpret gestures with greater accuracy, enabling more natural and expressive ways to control devices and interact with virtual environments.
Ethical Computer Vision
As with any transformative technology, it's imperative to address the ethical implications of computer vision to ensure its responsible development and deployment.
Bias and Fairness: Computer vision models are susceptible to inheriting biases present in the data they are trained on. If training datasets are not representative of diverse populations, the resulting models may perpetuate existing societal biases, leading to unfair or discriminatory outcomes. Addressing bias in training datasets and developing algorithms that promote fairness are crucial for mitigating these risks.
Privacy Concerns: Computer vision applications often involve the collection and analysis of personal data, raising concerns about privacy violations. The use of facial recognition technology, in particular, has raised concerns about mass surveillance and the erosion of privacy. Implementing robust data protection measures, ensuring transparency in data usage, and obtaining informed consent are paramount for addressing these ethical concerns.
Job Displacement: As computer vision automates tasks previously performed by humans, it's essential to address potential job displacement and ensure a smooth transition for workers in affected industries. Governments, educational institutions, and businesses need to collaborate on retraining programs and create new job opportunities to mitigate the negative impacts of automation on the workforce.
Conclusion
The field of computer vision, which is undergoing a period of rapid advancement, has the potential to profoundly impact our world in numerous ways. From revolutionizing healthcare to propelling the advancement of autonomous vehicles, the applications of this technology are as diverse as they are transformative.
As we navigate the frontiers of this technological revolution, it is incumbent upon us to harness the power of computer vision responsibly, ensuring that its benefits are accessible to all members of humanity while mitigating potential risks. By fostering collaboration between researchers, policymakers, and industry leaders, we can collectively shape a future where computer vision empowers us to address some of the world's most pressing challenges and create a more equitable, efficient, and sustainable future for all.