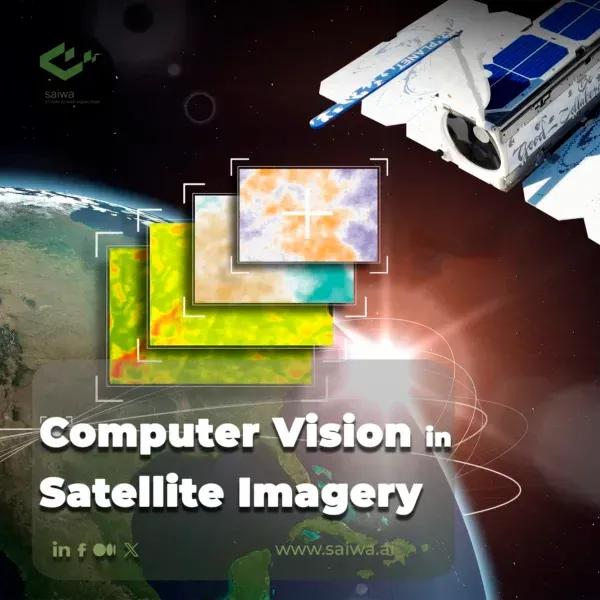
The Transformative Power of Computer Vision in Satellite Imagery
The exponential growth of satellite imagery in recent years has opened up new possibilities for analysis through computer vision techniques. Computer vision involves training computers to interpret and understand visual data such as digital images and videos. When applied to the vast amounts of satellite data being collected daily, computer vision unlocks the potential to rapidly extract actionable insights at scale.
This blog post explores the burgeoning role of computer vision in satellite imagery analyzing across diverse applications from mapping to surveying and monitoring environmental changes. It delves into computer vision techniques like convolutional neural networks that enable feature extraction and pattern recognition from overhead visual data. The importance of labeled training data and considerations like cloud cover challenges are also examined. Overall, the convergence of satellite technology and computer vision promises to transform fields ranging from urban planning to disaster response through near real-time geospatial intelligence.
What is Satellite Imagery
Satellite imagery refers to photographs of the Earth's surface captured from orbiting satellites. It provides an overhead perspective not possible from ground level, enabling a unique vantage point of our planet. Satellites use a variety of sensors to collect different types of image data:
Visible spectrum images show natural color representations similar to standard photography.
Multispectral images capture specific electromagnetic spectrum bands beyond human vision such as infrared.
Radar images use microwave signals to penetrate cloud cover and capture terrain and topography.
High-resolution satellites can capture detail down to one meter per pixel or less. With multiple global satellites in orbit, new images of nearly any location on Earth are captured at regular intervals, providing invaluable data for computer analysis.
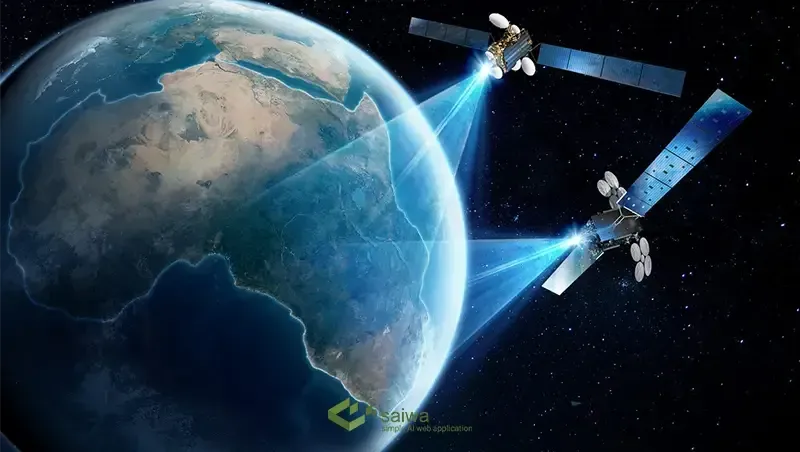
Computer Vision Satellite Image Analysis
The wealth of visual data acquired by Earth observation satellites is ripe for analysis through computer vision techniques. This involves processing the raw image pixels and applying algorithms to extract meaningful information. Key approaches include:
Image pre-processing
This prepares images for further analysis by transforming them into standardized formats and enhancing image quality through processes like pan-sharpening, noise reduction, and normalization.
Feature extraction
Algorithms detect distinct elements in images like edges, corners, contours, shapes, and textures that represent features of interest. This reduces raw pixels into informative feature data.
Object detection
Models identify and classify specific objects like buildings, roads, forests, and vehicles, while also localizing their position within images through bounding boxes.
Semantic segmentation
This assigns categorical labels to image pixels to segment and classify objects and regions in satellite images through color coding.
Deep learning models like convolutional neural networks (CNNs) have proven highly effective for computer vision satellite image analysis. By training CNNs on vast labeled datasets, they can learn to interpret overhead visual data for tasks like land cover mapping, object counting, and damage assessment.
Fusing satellite imagery with sensor data like hyperspectral, LiDAR, and RADAR through data integration techniques further enhances analysis capabilities for computer vision.
Geospatial Applications of Computer Vision in Satellite Imagery
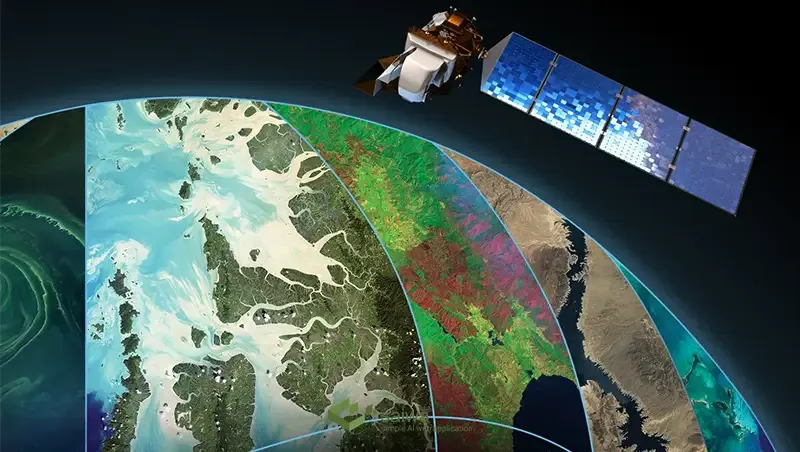
The ability to rapidly process volumes of overhead imagery with computer vision creates immense opportunities across diverse geospatial applications:
Land cover and land use mapping
Multi-temporal analysis of satellite images enables tracking changes in vegetation, water bodies, urbanization, agriculture, and other land use over time. This supports applications like urban planning, disaster response, and environmental monitoring.
Infrastructure mapping
Roads, buildings, bridges, and other critical infrastructure elements can be automatically detected from techniques of computer vision in satellite imagery. This is invaluable for mapping in remote or rapidly evolving areas.
Cartography
Computer vision significantly accelerates traditional cartography processes of satellite image analysis and feature identification for map creation and updating.
Surveying and cadaster
Highly precise measurements and 3D models of any terrain can be extracted from satellite data through photogrammetry methods, aiding surveying tasks. Property boundaries for land administration can also be mapped.
Traffic monitoring
Vehicle movements, road usage patterns, and congestion can be assessed by analyzing time-series satellite images of road networks. This supports intelligent transportation initiatives.
Disaster response
Computer vision satellite imagery enables rapid analysis of disaster-affected areas to reveal the extent of damage and prioritize response. Change detection from pre and post-disaster images is invaluable.
Agriculture monitoring
Detection of crop types, health assessment, and yield prediction is possible by applying techniques of computer vision in agriculture land satellite imagery.
The wide-ranging use cases demonstrate the advantages of scalable overhead visual data analysis through computer vision for actionable geospatial intelligence.
Read Also: Satellite Monitoring in Agriculture | Advancing Sustainable Farming
The role of data labeling tools
A major challenge in training models of computer vision in satellite imagery is the need for vast amounts of labeled data covering the immense diversity of objects and landscapes visible from space. Manually labeling sufficient satellite images to teach algorithms to interpret overhead visual data is impractical. This is where data labeling tools become crucial to generate tagged datasets.
Modern data labeling platforms provide user-friendly interfaces for domain experts to rapidly label geospatial features in satellite images. This includes drawing bounding boxes around objects like buildings, tracing outlines of land parcels, and assigning classification tags to segments through semantic segmentation. Advanced platforms offer capabilities like distributed labeling workflows to coordinate work by multiple annotators and auto-labeling through machine learning to expedite the process.
The richly annotated satellite imagery datasets produced by data labeling tools serve as input to train supervised computer vision models. Performance metrics during training provide feedback to refine the labeling process for optimal model development.
With ready access to well-labeled satellite image training data produced efficiently through such platforms, developers can operationalize highly accurate computer vision models for geospatial applications much faster. These tools help overcome a key bottleneck in harnessing the vast troves of available but unlabeled satellite imagery.
Challenges of Training Computer Vision Models on Satellite Imagery
While satellite imagery provides abundant visual data to train computer vision models, it also poses some unique challenges:
Perspective distortion: Overhead viewpoints produce skewed perspectives of objects compared to natural human vision. This can impact model performance.
Variability: Satellites capture imagery under diverse conditions (time of day, season, weather etc.) leading to high visual variability of the same location.
Mixed pixel effect: With lower-resolution imagery, single pixels may contain multiple ground objects. This creates ambiguity.
Cloud occlusion: Clouds frequently obscure parts of images, limiting model training.
By utilizing techniques like data augmentation, transfer learning from labeled open source datasets, and simulated training data, the challenges of computer vision in satellite imagery can be mitigated to develop robust computer vision models. Ongoing improvements in sensor resolution and revisit frequency also promise to ease issues like mixed pixels and cloud occlusion over time.
Conclusion
The integration of computer vision in satellite imagery technology promises to revolutionize the extraction of geospatial intelligence from overhead imagery. Through advanced algorithms trained on massive labeled datasets, computers can now interpret complex visual patterns in satellite images at unprecedented speed and scale. While challenges exist, computer vision offers a new paradigm for rapidly monitoring changes, mapping features, assessing damage, and gaining insights from satellite visual data across countless applications.
Realizing the full potential of computer vision on satellite imagery will require cross-disciplinary collaboration between data scientists, geospatial experts, and subject matter specialists. As sensor and computer vision technologies continue advancing in tandem, near real-time geospatial awareness at a global scale is on the horizon. Just as GPS transformed navigation, this new wave of AI-driven satellite image analytics could soon provide a digital "sixth sense" to augment our understanding of the physical world. With such knowledge, society will be empowered to address challenges like climate change, food security, disaster response and environmental sustainability with greater foresight than ever before possible.