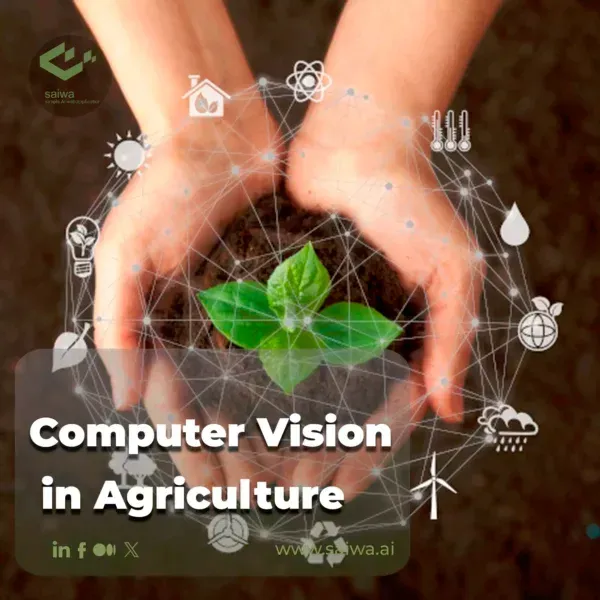
Agriculture is one of the most important industries in the world because it is our food source. As digital technologies are transforming different industries, agriculture is also being transformed by these technologies. Like other industries, agriculture faces various challenges, including climate change, labor shortages, disruptions caused by epidemics, and others. Digital technologies, including computer vision, can greatly help the agricultural sector overcome these challenges and achieve greater efficiency, flexibility, and sustainability.
In this article, we will discuss the applications of computer vision in agriculture. We will also explain the benefits and challenges of each.
Read Also: Practical Application & Future of AI in Agriculture
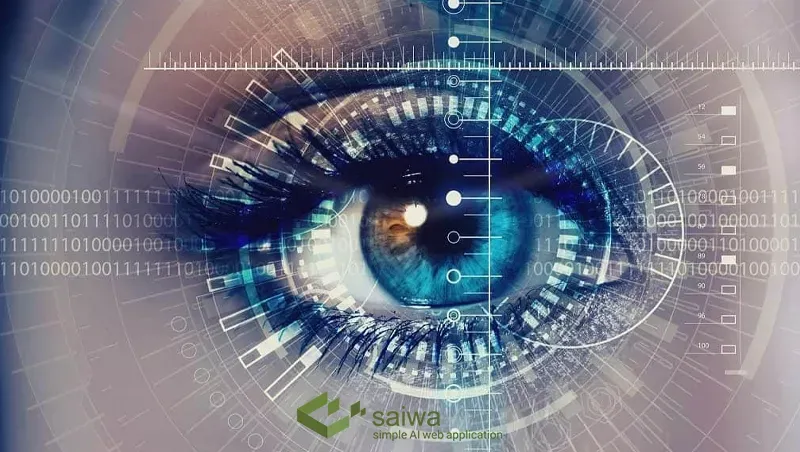
An overview of computer vision
Computer vision is a subset of artificial intelligence that gives machines the ability to think and interpret visual data like humans. Computer vision systems analyze a collection of images, snapshots, and other objects and can track, label, describe, predict, and evaluate specific objects in those images. Its purpose is to train artificial intelligence to go beyond simple automation processes, especially by integrating deep learning. Computer vision technology can include all cases by training artificial intelligence based on presence and pattern recognition. For example, when we see a scene, our eyes record the scene, where the objects are and whether they are moving; based on this, we can make predictions.
As everything is expanding, experts expect computer vision to develop and expand in the modern world and make changes.
How computer vision is helpful in agriculture
As mentioned in the previous section, computer vision is a subset of artificial intelligence that gives machines the ability to understand and interpret the visual world like humans. By combining computer vision techniques with remote cameras to capture images, non-contact and scalable sensing solutions are possible in agriculture.
Below is a list of some of the key applications of computer vision in agriculture. As technology advances, we can expect to see more cases in this area shortly.
Applications of computer vision in agriculture
Agriculture, livestock, and farming all benefit from computer vision techniques. When agriculture, as the oldest profession known to man, is combined with the new frontiers of computer vision and machine learning, it is poised for many advancements. The adaptation of deep learning and machine learning applications in agriculture will be transformative, making processes more efficient, accurate, and automated, and bringing us closer to the future.
With the help of computer vision systems and the large amount of data they provide, farmers can make the most informed decisions and provide faster solutions. There are many applications of computer vision in agriculture, and we look at some of them in this section:
Harvesting with machines
It can be said that the benefits of agriculture with the help of computer vision in agriculture are many, that is, the principles and methods of agricultural automation can be different. Machines are an efficient and time-saving way to harvest crops. Specialized robots equipped with object recognition and deep learning technologies can harvest fruits and vegetables autonomously.
Currently, farmers can classify and identify their products through computer vision systems, and specialized machine learning models can check and identify the condition of the product. By using computer vision in agriculture, the work process becomes faster and the need for manual work is reduced. This technology can also be very useful during harvest seasons, as fruits and vegetables perish if left unattended.
Of course, it should be kept in mind that fruit recognition is one of the most difficult tasks in agricultural automation. These complications are due to unpredictable variables in the process that can distort the results, such as inconsistent light, poor visibility, the presence of a lot of foliage, inconsistency in the shape of the fruit or vegetable, and others.
Self-driving tractors
Self-driving tractors are becoming a huge success in agriculture. Relying on computer vision, they have a complete 360-degree view of their surroundings. Using self-driving tractors, time-consuming tasks such as harvesting and removing crops can be done faster and more sustainably. These tractors need the help of neural network algorithms to analyze the data captured by their cameras and later use it to prove their algorithms and improve performance.
With the help of computerization, tractors can tag crops, giving farmers the ability to monitor plant changes while tracking the necessary trends to increase crop yields.
Of course, you should keep in mind that self-driving tractors are a big investment, but you should be careful about the legal aspects of buying them. Since self-driving tractors operate in public areas, there is a risk of damaging or even harming the soil and plants. There is transmission to humans and animals.
Read More: Find the Impact of AI in Self Driving Cars with Our Guide
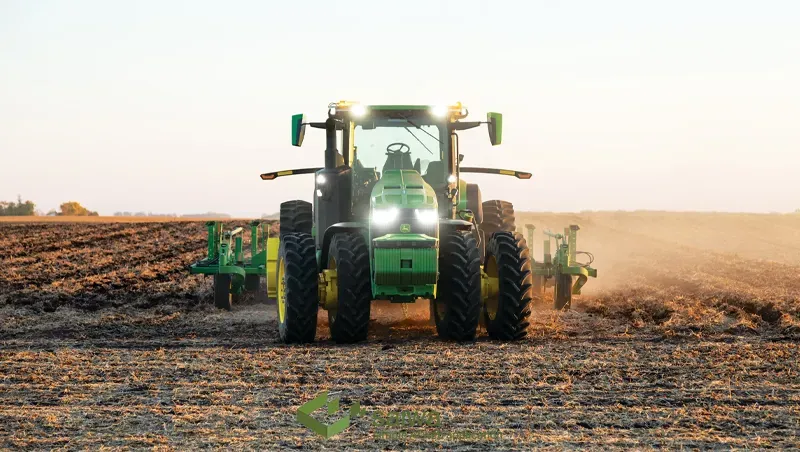
Grading and sorting
Assessing characteristics such as color, size, and defects in crops is a task that agricultural workers perform to ensure the quality of their harvest. They also evaluate the needs of a particular product. Traditional human grading and sorting is an intensive and time-consuming process. Larger farms require more workers and growers to sort and grade hundreds of thousands of crops each day.
In this situation, computer vision in agriculture is introduced to innovate the process through scanners equipped with image classification technology. Using artificial intelligence and computer vision algorithms, farm workers receive real-time monitoring of crop growth and satellite images of their conditions.
Of course, while early technologies and new prototypes are showing promise, they still have some shortcomings that need to be addressed. For example, scanning the product through a 2D image can lead to inconsistencies. Also, the biological diversity between fruits and vegetables should be considered for yield.
Vertical farming
Vertical farming is transforming traditional agriculture by growing crops vertically indoors, increasing crop production in a small space instead of relying solely on land, machinery, and water. Vertical farming improves performance and space by using computer vision to monitor a plant's life cycle through its cameras and sensors.
Vertical farming can control temperature, gases, and other things by maximizing the use of agricultural technologies. It can also reduce water consumption and labor costs, ensure more sustainable production, and use energy more efficiently.
Because vertical farming is done in indoor environments, it does not allow insects to enter and will affect the pollination process. Farmers should keep such things in mind and do pollination manually. It should also be said that vertical farming depends on technology, which means that the smallest problem can affect the entire process.
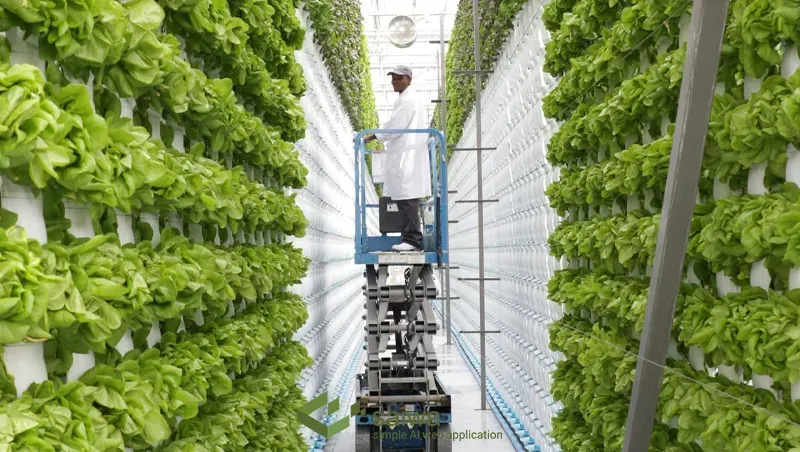
Aerial imaging and scanning
In recent years, drones have been widely used for tasks such as remote sensing and fire detection. Aerial imaging and scanning using computer vision technology aims to create what is known as agricultural vision. The purpose of using drones is to provide accurate data for agriculture.
The goal of precision agriculture is to improve environmental sustainability, yield estimation, and efficiency while reducing the number of traditional inputs required to grow crops, including land, water, fertilizer, and others. This is possible through accurate imaging and mapping to evaluate each area based on the imaging results.
Drones equipped with extensively trained computer vision models are an essential element of smart agriculture for processes such as vegetation classification and segmentation, crop counting, yield monitoring and prediction, crop mapping, weed identification, and disease and nutrient deficiency diagnosis. These drones allow people in the agricultural sector to remotely control agricultural products.
Of course, it is important to note that there are still limitations to computer vision in aerial agriculture. These limitations include subtle differences in the condition of plants over time, such as different plants going through different stages throughout the year and at different times. The technology needs to be error-free in its deep learning capabilities to assess these spatial changes without compromising accuracy.
Read More: AI-Based Drone Operation| AI in Drones use cases
How Computer Vision Farming Combats Pests and Disease
Traditionally, spotting pests and diseases in agricultural fields relied on manual scouting, a time-consuming and often inaccurate process. However, computer vision farming offers a revolutionary solution. By leveraging cameras, drones, and powerful algorithms, computer vision farming can automatically monitor vast fields, identifying signs of trouble long before the naked eye can.
This early detection is crucial. By catching infestations or diseases in their nascent stages, farmers can take targeted action, minimizing damage and preserving yield. Computer vision in agriculture can identify specific pests and diseases based on subtle visual cues in leaf color, texture, or growth patterns. This level of detail allows for a more precise response, compared to broad-spectrum treatments that can harm beneficial insects and disrupt ecological balance.
Furthermore, computer vision farming can track the spread of pests and diseases across fields over time. This real-time data allows farmers to predict outbreaks and implement preventative measures in unaffected areas. With its potential for comprehensive monitoring, early detection, and targeted intervention, computer vision farming is a powerful tool for sustainable pest and disease management.
Read More: Plant Disease Identification | Leveraging Technology for Early Detection and Effective Management
Computer Vision and Its Applications Across Industries
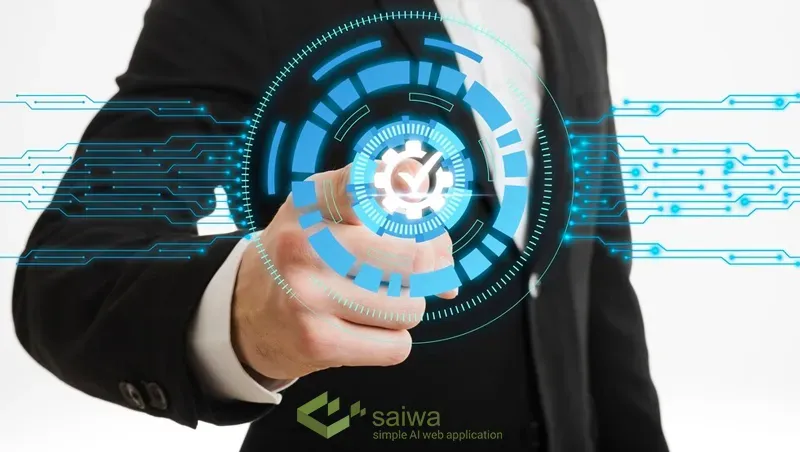
Computer vision, a blend of computer science concepts and AI technology, endows machines with the ability to decipher and act upon visual information. This initiative isn’t just about imitating human sight but also empowering devices to extract meaning from images and videos. By developing algorithms capable of identifying relevant data from visuals, this field broadens its horizons, affecting more than just computer vision farming.
Healthcare
computer vision in agriculture is redefining medical imaging. Its ability to facilitate the early detection of diseases is pivotal, offering healthcare experts a robust tool for interpreting diagnostic scans such as X-rays, MRI, and CT images. The precision of computer vision algorithms enables more accurate diagnoses, reducing the likelihood of human error. Additionally, it enhances surgical assistance through real-time imaging analysis, improving patient outcomes.
Automotive industry
here computer vision serves as the backbone of self-driving technology and driver support systems. It equips vehicles with the perception to navigate surroundings, spot potential hazards, and determine safe outcomes on the road. The integration of advanced vision systems has significantly improved the reliability of autonomous vehicles. Furthermore, it supports driver-assist features such as lane departure warnings and adaptive cruise control, making driving safer for all.
Retail businesses
In computer vision for streamlining inventory control, gaining customer insights, and elevating the shopping journey. Innovations like automated checkouts, shelf surveillance, and facial recognition for tailored consumer experiences are creations of this technology. By analyzing shopper behavior, retailers can offer personalized product recommendations in real time. Additionally, it enables faster restocking through real-time inventory management, enhancing store efficiency.
Manufacturing
In manufacturing, consistent quality and efficiency are achieved through computer vision. It is instrumental in detecting product defects, which contributes to a more uniform production line and automated inspection processes. The ability to monitor production at every stage reduces downtime and increases overall productivity. In addition, computer vision systems can optimize workflows by identifying areas of inefficiency, leading to smarter manufacturing decisions.
Security and Surveillance
The security and surveillance sector benefits immensely from computer vision. It powers technologies such as facial identification, object tracking, and motion analysis, bolstering the efficacy of security systems to act swiftly against threats. These technologies provide real-time monitoring capabilities, enabling rapid response to suspicious activities. Additionally, advanced computer vision systems can predict potential security breaches, increasing the level of preventive action.
Entertainment
The entertainment industry employs computer vision for recognizing gestures, and for enhancing virtual and augmented reality applications, resulting in more engaging gaming and interactive experiences. It brings lifelike animations to the screen through motion capture technology, heightening the realism of visual effects. Computer vision also enables more immersive experiences in AR and VR by precisely tracking user movements and integrating them into virtual environments.
Financial
In the financial world, computer vision strengthens fraud detection and security. Analyzing transactional patterns and pinpointing irregularities are part of its forte, thereby enhancing the safeguarding of financial activities. It can also verify user identities through biometric facial recognition, reducing fraud risks in online banking. In addition, computer vision tools are instrumental in automating document processing, improving efficiency in financial operations.
Education
Educational institutions integrate computer vision for automated grading, attendance monitoring, and developing engaging and interactive learning resources. This fosters the creation of cutting-edge educational tools and customized learning scenarios.
With continual advancements, computer vision’s imprint across industries is only growing besides computer vision farming. It presents creative resolutions to intricate problems and is reshaping our interaction with technology in life’s multiple facets.
Computer Vision in Sustainable Agriculture: Optimizing Resource Use for a Thriving Future
While computer vision offers a multitude of benefits to agriculture, its role in promoting sustainable practices is particularly noteworthy. Let's dig deeper into how computer vision in sustainable agriculture is revolutionizing resource management and paving the way for a greener future.
Precision Irrigation with Eagle Eyes
Traditional irrigation methods often lead to water waste, impacting soil quality and overall sustainability. Computer vision in sustainable agriculture equipped drones and sensors can meticulously monitor crop health and moisture levels. This data empowers farmers to target irrigation precisely, delivering water only where and when it's needed. This not only conserves this precious resource but also minimizes water runoff and associated environmental concerns.
Promoting Biodiversity
Computer Vision in Sustainable Agriculture can help to make a healthy ecosystem. To exemplify, computer vision can be a valuable tool for monitoring biodiversity. Cameras and sensors can track pollinators and other beneficial insects, providing insights into the overall health of the agricultural environment. This data helps farmers to get closer to promote biodiversity, such as planting pollinator-friendly flowers or creating habitats for beneficial insects.
Optimizing Nutrient Delivery
Analyzing soil composition and nutrient levels is another important application of computer vision in sustainable agriculture. By having this knowledge, farmers can create customized fertilization plans, ensuring crops receive the precise nutrients they need for optimal growth. This removes excess fertilizer application, which can leach into waterways and disrupt ecosystems.
Computer Vision for Livestock Management
Computer vision farming is revolutionizing the way we manage livestock. By employing advanced image and video analysis techniques, farmers can enhance animal welfare, improve productivity, and optimize the use of resources. Here are some key applications of computer vision in livestock management:
Animal Health Monitoring: Computer vision algorithms have the capacity to analyze images and videos of livestock to detect early signs of disease or injury. By identifying abnormalities in behavior, posture, or physical appearance, farmers are able to intervene promptly to prevent outbreaks and reduce mortality rates.
Behavior Analysis: Computer vision systems are capable of tracking animal behavior patterns, including feeding, drinking, and resting habits. This information can be utilized to identify potential stress factors, optimize feeding schedules, and enhance overall animal welfare.
Automated Feeding and Watering Systems: Computer vision allows for the monitoring of feed and water levels in troughs and automatically adjust them as required. This helps to ensure that animals have consistent access to food and water, promoting optimal growth and health.
Precision Livestock Farming: The analysis of data collected through computer vision enables farmers to make more informed decisions about breeding, feeding, and housing. This precision approach can lead to increased productivity and reduced environmental impact.
Smart Barns and Automated Milking: The integration of computer vision into smart barn systems enables the monitoring of temperature, humidity, and air quality. Furthermore, it allows for the automation of milking processes, thereby enhancing efficiency and reducing labor costs.
Ethical Considerations and Regulatory Frameworks for Computer Vision in Farming
As the adoption of computer vision in farming continues to grow, it is essential to address the ethical implications and regulatory considerations surrounding these technologies. Some key areas of focus for computer vision in farming include:
Data Privacy and Security
Agricultural data, including images, video, and sensor data, often contains sensitive information about farm operations, crop yields, and individual farmers.
Ensuring the proper collection, storage, and use of this data, while respecting the privacy and data rights of farmers and agricultural workers, is a critical ethical concern.
Developing robust data governance frameworks and security measures to protect against data breaches and unauthorized access is essential.
Algorithmic Bias and Fairness
Computer vision in farming systems, like other AI-powered technologies, can perpetuate or amplify biases present in the data used to train them. Careful consideration must be given to the potential for these biases to lead to unfair or discriminatory outcomes, particularly in areas such as resource allocation, access to technology, and decision-making.
Implementing strategies to identify and mitigate algorithmic bias, and ensuring fairness and inclusivity in the development and deployment of computer vision in agriculture, is crucial.
Transparency and Explainability in Computer Vision in Farming
The "black box" nature of some computer vision in farming algorithms can make it challenging to understand the reasoning behind their decisions and outputs.
Promoting transparency and explainability in the development and deployment of computer vision in farming systems is essential to build trust, enable accountability, and ensure informed decision-making by farmers and agricultural stakeholders.
Farmer Education and Training for Computer Vision in Agriculture
The agricultural industry is undergoing a transformative shift, with computer vision emerging as a cornerstone technology. From revolutionizing disease detection to optimizing irrigation practices, computer vision in agriculture offers a plethora of benefits. However, maximizing its potential requires a strategic focus on farmer education and training specifically in computer vision applications within the agricultural sector.
Imagine a scenario where a farmer, upon encountering a struggling crop, utilizes a smartphone application empowered by computer vision. By simply capturing an image, the app leverages computer vision in agriculture analysis to diagnose the issue and recommend targeted solutions. This empowers farmers to make data-driven decisions, transitioning away from traditional methods and intuition. However, to unlock such possibilities, effective farmer training in computer vision in agriculture applications is paramount.
Tailored training programs are crucial to cater to diverse experience levels. For beginners, introductory workshops can demystify core computer vision in agriculture concepts and showcase practical applications in agriculture. For seasoned farmers, the focus can shift towards advanced topics such as data interpretation and seamless integration of computer vision with existing farm management practices.
The onus doesn't solely reside with farmers. Technology developers must prioritize user-friendliness. Envision computer vision applications equipped with interactive tutorials that guide farmers through the process and highlight relevant case studies. This approach not only reduces the barrier to entry but also fosters confidence in utilizing these innovative tools.
Ultimately, successful farmer education and training in computer vision pave the way for a future characterized by informed decision-making, enhanced efficiency, and sustainable practices. By equipping farmers with the necessary knowledge and skills to navigate this technological revolution, we can unlock the true potential of computer vision in agriculture and usher in a new era of agricultural prosperity.
Computer Vision for Post-Harvest Applications
The application of computer vision in agriculture is transforming post-harvest processes, enhancing efficiency, and ensuring product quality.
Shelf Life Prediction
The application of computer vision in agriculture can contribute to extending product shelf life. By analyzing images of agricultural products over time, computer vision systems can identify early signs of spoilage, such as mold growth or color changes. This information can be used to optimize storage conditions and predict product expiration dates, reducing food waste and ensuring consumer safety.
Packaging Optimization
The application of computer vision in agriculture plays a crucial role in optimizing packaging processes. By analyzing product dimensions and shapes, computer vision systems can determine the most efficient packaging configurations, reducing material waste and shipping costs. Moreover, computer vision can verify package integrity and detect defects, ensuring that products reach consumers in optimal condition.
Labor Reduction and Efficiency
The application of computer vision in agriculture can significantly reduce labor requirements and improve efficiency in post-harvest operations. By automating tasks such as sorting, grading, and packing, computer vision systems can free up human workers for more complex and strategic roles. Additionally, computer vision can monitor equipment performance and identify maintenance needs, reducing downtime and optimizing resource utilization.
Optimizing the Supply Chain and Logistics with Agriculture and Food Computer Vision
With the growing complexity of global food distribution networks, agriculture and food computer vision technologies are emerging as key enablers of supply chain and logistics optimization. These advanced systems leverage visual data to streamline processes, enhance food safety, and improve traceability. By integrating computer vision into various stages of the supply chain, from production to delivery, stakeholders can achieve greater transparency, reduce waste, and ensure that high-quality products reach consumers more efficiently.
Enhancing Efficiency with Computer Vision
Agriculture and food computer vision play an important role in the Supply chain and logistics optimization by automating various tasks and providing real-time insights. By using advanced image recognition and basic machine learning algorithms, computer vision systems can monitor the movement of goods through the supply chain, identifying bottlenecks and optimizing routes for delivery. This ensures that fresh produce maintains its quality and reaches its destination promptly.
Ensuring Food Quality and Safety
Food safety is a significant concern in the supply chain, and agriculture and food computer vision technologies provide robust solutions. By inspecting food products for contaminants and verifying packaging integrity, computer vision systems enhance the overall quality control process. These systems can detect even minor defects that human inspectors might miss, ensuring that only the highest quality products reach the market. Furthermore, by continuously monitoring conditions during transport, such as temperature and humidity.
Improving Traceability and Transparency
Consumers are increasingly interested in the origin and journey of their food. Agriculture and food computer vision facilitate enhanced traceability by capturing detailed data at each stage of the supply chain. This information can be used to track products from farm to table, providing transparency and building consumer trust. By integrating computer vision with blockchain technology, stakeholders can create an immutable record of each product's journey, ensuring accountability and facilitating recall processes if necessary.
Read Also: A Deep Dive Into AI in Agricultural Supply Chain
Automated Weed Identification and Management
Automated weed identification is transforming the agricultural sector, primarily through Computer Vision in Agriculture. This technology utilizes advanced imaging systems and AI algorithms to classify and weed detection in real time, leading to improved crop health and reduced chemical use. Key advantages include:
Precision Targeting: With Computer Vision in Agriculture, farmers can precisely locate weed-infested areas, allowing targeted herbicide application rather than blanket spraying. This not only saves costs but also reduces environmental impact by minimizing herbicide runoff, making farms more eco-friendly and compliant with sustainable practices.
Real-Time Detection: Unlike traditional methods, which can be labor-intensive, automated systems offer rapid, real-time detection. Computer Vision in Agriculture scans fields with drones or ground robots, instantly identifying weeds based on unique shapes, colors, and textures, this enables farmers to address weed growth before it impacts crop yield.
Data-Driven Insights: Besides detection, Computer Vision in Agriculture provides valuable data on weed distribution and density. This information can be integrated into a farm’s management system, allowing for more informed decisions that enhance weed control efficiency and predict future infestations.
The integration of Computer Vision in Agriculture for weed management conserves resources and aligns with sustainable farming practices. By leveraging these innovations, farmers can protect their crops more efficiently, reduce chemical dependency, and ultimately support healthier ecosystems while maintaining high agricultural productivity.
Computer Vision and Controlled Environment Agriculture (CEA)
Controlled Environment Agriculture (CEA) is revolutionizing food production by enabling precise control over growing conditions, independent of external weather. This approach, encompassing greenhouses, vertical farms, and indoor facilities, promises higher yields, reduced resource use, and year-round production. However, realizing the full potential of CEA requires constant, detailed monitoring and automation, and this is where computer vision plays a crucial role. The integration of computer vision in agriculture is transforming CEA from a promising concept into a highly efficient and sustainable reality.
Enhancing Plant Health Monitoring
Instead of relying solely on manual inspections, which are time consuming and prone to human error, cameras and sensors become the eyes of the operation. Systems using computer vision in agriculture analyze plant health, detecting subtle changes in leaf color that indicate nutrient deficiencies or early disease signs long before they are visible to the human eye. This allows growers to take immediate corrective action, preventing widespread problems and maximizing yields.
Automating Key Tasks
Computer vision in agriculture goes beyond monitoring; it drives automation within CEA facilities. Robots, guided by vision systems, can perform tasks like precisely planting seeds, transplanting seedlings, and harvesting crops. This automation reduces labor costs and improves the overall efficiency of operations. The precise control offered by CEA is further enhanced by computer vision's ability to deliver water, nutrients, and energy with pinpoint accuracy, minimizing waste.
Ensuring Quality and Consistency
Quality control systems employing computer vision in agriculture can grade and sort produce based on size, shape, and color. This ensures consistent quality in the harvested crops and minimizes waste, contributing to a more sustainable food supply chain. The synergy between CEA and computer vision is not just about increasing yields; it's about creating a data-driven, highly efficient, and ultimately more resilient approach to food production.
Challenges in Implementing Computer Vision in Agriculture
Despite the significant impact of computer vision in agriculture, it is very important to acknowledge the challenges come with it.
Data Requirements
Computer vision in agriculture systems thrive on data, specifically large amounts of high-quality, labeled images. To train these systems effectively, they need to "see" a wide variety of scenarios. Acquiring these comprehensive datasets across the full spectrum of real-world farming conditions can be a significant undertaking, demanding both time and resources.
Environmental Variability
Variations in lighting, unpredictable weather patterns, and the general condition of the field itself can all negatively impact the accuracy of computer vision models. This necessitates the development of adaptive solutions, and it also requires ongoing fine-tuning of the models to ensure they perform reliably despite these ever-changing conditions.
Initial Investment
The financial commitment required to implement computer vision can be a substantial barrier, particularly for smaller farms. High-resolution cameras, drones, and the computational infrastructure needed to process visual data represent a considerable upfront cost. For some farms, especially those in rural areas, the need for improved internet connectivity and a reliable power supply can further increase this initial investment.
Technical Training
Implementing computer vision in agriculture is not simply a matter of purchasing equipment. Installing and operating these systems effectively requires specialized training. Farmers and their workforce need to learn how to utilize both the hardware and the software components. The vast amounts of data generated also require expertise in data storage, management, and visualization to derive actionable insights. This often requires upgrading existing staff skills or hiring external data experts, increasing operational costs.
A Fusion of Computer Vision Indoor Farming Data and Social Media Analysis
Imagine a future where indoor farms not only optimize growth but also predict consumer desires. This innovative approach is emerging through the combination of computer vision indoor farming data and social media analysis. By analyzing both sets of data, farmers can gain valuable insights into consumer preferences, allowing them to tailor their crops for maximum marketability.
Computer vision indoor farming data plays a crucial role in this process. Cameras within the farm capture detailed images of harvested crops, providing information on size, shape, and color variations. This data is then analyzed to identify trends and characteristics associated with high-demand crops.
Social media analysis acts as another data stream. By analyzing trending hashtags, images, and discussions about fruits and vegetables, farmers can understand the aesthetic preferences of consumers. This social media data can reveal a sudden surge in interest for a particular color of tomato or a unique shape of leafy greens.
By combining computer vision indoor farming data with social media analysis, farmers can predict which crop features are most likely to resonate with consumers. This helps them to make informed decisions about planting varieties, potentially adjusting growing conditions like light exposure to influence the final appearance of the crops.
This data fusion approach not only optimizes yield and resource utilization within the farm but also ensures the final product aligns with consumer preferences. Ultimately, this innovative use of computer vision indoor farming data can create a win-win situation for both farmers and consumers.
Collaboration of IoT and Computer Vision in Agriculture
The collaboration of IoT and computer vision in agriculture has revolutionized the way farmers monitor and manage their crops, enabling more efficient and precise farming practices.
Real-Time Monitoring: IoT sensors deployed in agricultural fields collect real-time data on environmental conditions, such as temperature, humidity, and soil moisture. When integrated with computer vision in agriculture, these sensors can provide visual insights into crop health, allowing farmers to detect issues early. This combined data helps in creating a comprehensive overview of field conditions, aiding in timely decision-making.
Enhanced Crop Management: By using computer vision in agriculture, farmers can monitor the growth of crops through cameras and drones. The data from IoT devices combined with images captured by computer vision systems allow for accurate assessments of crop conditions, helping farmers make informed decisions about irrigation, fertilization, and pest control. This approach ensures that farmers can target specific issues with precision, reducing the need for broad interventions.
Precision Agriculture: The collaboration of IoT and computer vision helps farmers practice precision agriculture, where inputs like water, fertilizers, and pesticides are applied only when and where they are needed. This minimizes waste and maximizes yield while reducing environmental impact. By fine-tuning resource distribution, farmers can optimize operational costs and achieve higher profits.
Automated Processes: IoT-enabled devices can automate tasks like irrigation, and when paired with computer vision in agriculture, they can ensure that only the affected areas are watered or treated, improving efficiency and saving resources. This automation helps reduce human labor while increasing the overall productivity of farming operations.
Read More: IOT and Agriculture
Emerging Trends and Future Directions of Computer Vision in Agriculture
Computer vision in agriculture is constantly growing, driven by advancements in AI and other emerging technologies. Here are some key trends shaping the future:
Predictive Analytics
By using computer vision in agriculture systems, farmers can analyze vast datasets. This gives them valuable insight into crop health, growth patterns, and potential yield. Predictive analytics can forecast potential risks, optimize resource allocation, and improve decision-making for increased productivity and profitability.
Blockchain
Blockchain technology can enhance transparency and traceability, which creates a revolution in the agricultural supply chain. Computer vision in agriculture data integrated with blockchain can track the origin of produce, ensure food safety, and combat fraud, building trust between farmers, consumers, and retailers.
Swarm Intelligence and Collective Robotics
Swarm intelligence leverages the collective behavior of multiple robots or drones to perform complex tasks like pollination, pest control, and crop monitoring more efficiently and effectively than individual machines. This approach offers a promising solution for labor-intensive agricultural practices.
Satellite and Hyperspectral Imaging
Advanced remote sensing technologies such as satellite and hyperspectral imaging provide high-resolution data on crop health, soil conditions, and environmental factors. This data, combined with computer vision analysis, enables large-scale monitoring and precision agriculture at an unprecedented level.
Human-Computer Collaboration
The success of computer vision in agriculture depends on effective human-computer interaction. User-friendly interfaces and tools that empower farmers to understand and utilize computer vision insights are crucial for widespread adoption and successful implementation.
Conclusion
In general, the impact of computer vision systems and deep learning methods in the agricultural sector is very high because they create many improvements. Along with the expansion of agricultural machine learning data, agricultural robotics, and automation, this industry can eliminate many of its problems such as sustainability, climate, and labor shortage. With technological advancements in agriculture, there is no longer a need to spend long hours grading and scanning fruits and vegetables by hand or harming physical health. Considering that technology and agriculture are now together, farmers, botanists, and many workers in the agricultural sector can devote their time to other processes in their field of work.
Saiwa helps companies annotate, tag, and use higher-quality data. If you are looking for a reliable tool to speed up your field, this is the right place.