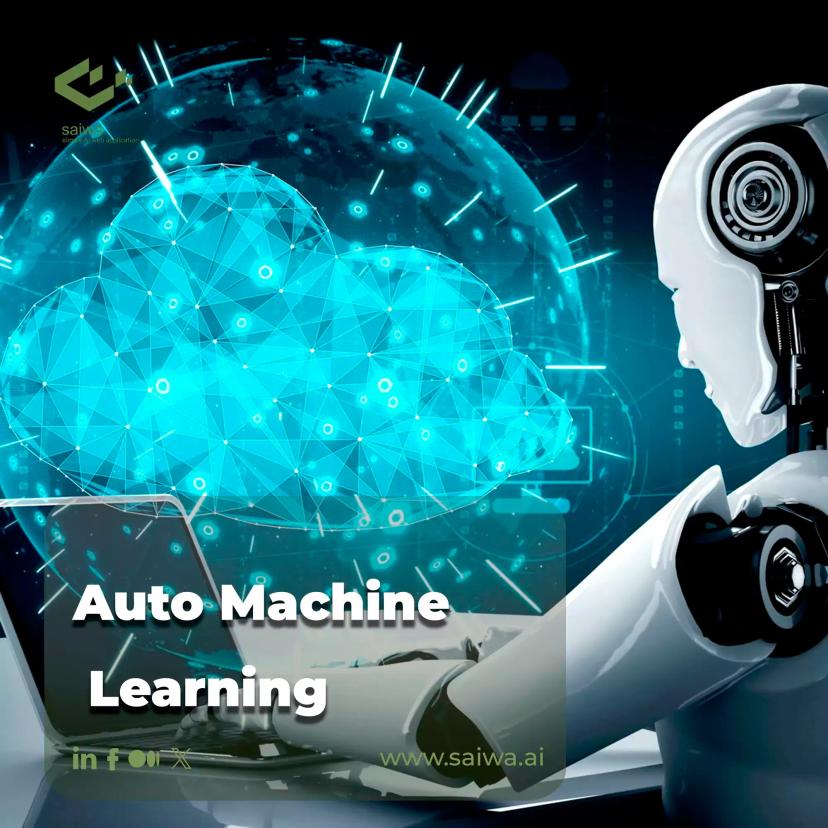
Your Complete Guide To Auto Machine Learning
Automation is a word that is often used when talking about artificial intelligence. This is because the software has the potential to take over the work of accountants, factory workers, writers, therapists, and other jobs. Currently, artificial intelligence has begun to automate itself in a process known as auto-machine learning. Auto machine learning has algorithms that take over the process of building a machine learning model. In fact, with this capability, more repetitive tasks are performed, and the process of artificial intelligence development and access to technology becomes faster.
What is auto machine learning?
Automatic machine learning is a process that uses machine learning models to solve real-world problems through automation. This technology automatically selects, combines, and parameterizes MLaaS models. Automating the machine learning process is much easier to use and typically provides faster and more accurate results than hand-coded algorithms.
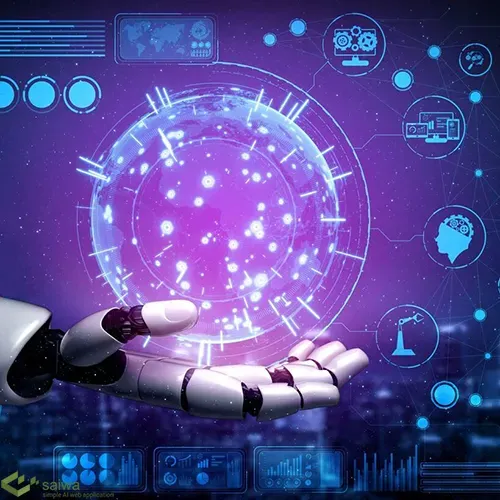
Types of auto machine learning
There are many steps in the data science pipeline that a data team must go through to build a predictive model. Experienced teams of data scientists and ML engineers can benefit greatly from the speed and transparency of Auto machine learning.
Data scientists start with a hypothesis, collect the right data set, try some data visualizations, and then develop additional features to capture all the available signals. Next, data scientists must train a model with hyperparameters and design an optimal architecture for the deep neural network. Automatic machine learning attempts to automate each step in the pipeline, but the most important and time-consuming steps to automate are as follows:
Automated feature engineering
The features used by the model have a direct impact on the performance of ML algorithms. Feature engineering requires a large investment of time and human resources, including data scientists, and involves a lot of trial and error that requires deep knowledge. Automated feature engineering is based on continuously generating new sets of features until the ML model achieves a good prediction.
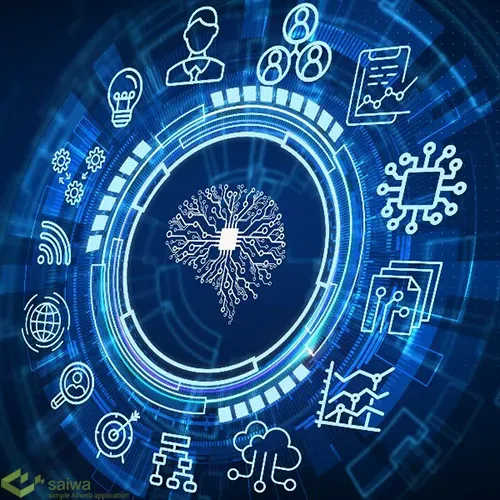
Automatic model selection and hyperparameter optimization
After preprocessing the data, an ML algorithm should be found to train with these features to make predictions with the new observations. In contrast to the previous step, where we said that there are many choices when selecting models. Classification and regression models, neural network-based models, clustering models, and others are among these options.
Each of these algorithms is suitable for specific problems. With automatic model selection, we can find the optimal model by running all the models suitable for a particular task and selecting the most accurate model. No single ML algorithm works well with all data sets. Some algorithms require more hyperparameter tuning than others. During model selection, we try to test different meta parameters.
Selection of automatic neural network architecture
Neural network architecture design is one of the most difficult and complex parts of ML. In traditional ML, data scientists typically spend a lot of time iterating through different neural network architectures with different meta parameters to optimize a model's objective function. This is a time-consuming process that requires deep knowledge and, in some cases, is prone to error.
Why is auto machine learning Important?
Currently, the demand for specialized knowledge in machine learning has increased significantly. The goal of Auto Machine Learning is to bridge the gap between supply and demand by automating processes that are complex for non-experts. This automation has resulted in easy-to-use machine learning software with simple interfaces. Anyone with basic technical knowledge and time to learn the toolkit can use it.
This technology enables non-data science analysts, marketers, and IT staff to incorporate machine learning into their workflow. By scaling machine learning across industries, all organizations can benefit from increased efficiency and effectiveness in the areas they need.
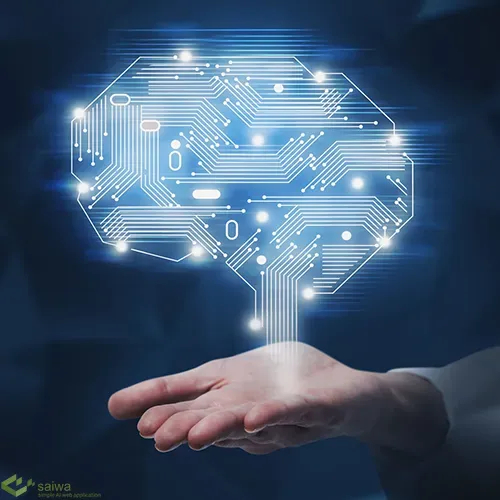
How does the auto machine learning process work?
The automatic machine learning process involves the following steps:
The user provides data that will be used to train the model, and this data is the input to the auto machine learning system. This data is typically a large data set that has been pre-processed and cleaned and is ready to be used in training a machine learning model.
The automatic machine learning system preprocesses the data and typically includes tasks such as feature engineering and normalization. This process helps make the data more suitable for training machine learning models and can improve the accuracy of the final model.
The automatic machine learning system trains multiple machine learning models on the preprocessed data using different algorithms and meta-parameters. This process allows the system to find the best model on the data.
The auto machine learning system evaluates the performance of the trained models and selects the best model with the best performance; then, this model is used as the output of the auto machine learning system.
Next, the user can use the trained model to predict or perform actions based on new data. This is typically done by exposing the model as a web service that other programs or users can access.
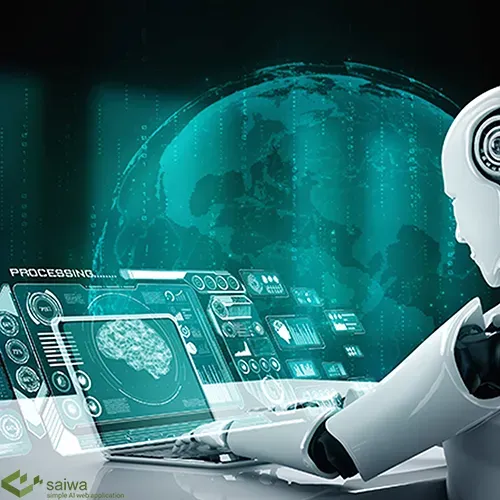
Pros and cons of auto machine learning
Now it's time to examine the pros and cons of this technology.
Advantages of automatic machine learning
You get results faster. With the help of automatic machine learning, you can take many machine learning and data science tasks off your shoulders and save time. You can use artificial intelligence when you want to find a prototype for testing or when you want to get early experience with user response.
The likelihood of the model becoming obsolete is reduced. One of the most common problems in the artificial intelligence development process is the obsolescence of the models built. Artificial intelligence technology moves fast. Auto machine learning solves this problem.
With a solution powered by auto machine learning, you can save money when considering whether or not infrastructure is needed. Most of the code in machine learning solutions is the glue code that creates the infrastructure around the model.
With this technology, you need fewer specialists. Another common problem with artificial intelligence as a service is that it requires a lot of expertise. This is expensive because experts are hard to find. With artificial intelligence, you can skip a lot of that.
Disadvantages of automatic machine learning
This technology gives you less insight into your data. One of the advantages of manual machine learning is that you can investigate why your models do not work. This gives you a good idea of the data needed to achieve the results.
Automatic machine learning is not flexible. You get results quickly, but you need to understand that over time requirements change, and new requirements may be outside the scope of an Auto machine learning solution.
If you want to scale, you may incur high implementation costs. A payment model is great for small to medium solutions, but if you want to use it for high volume, you will spend a lot of money.
Most likely, the final quality will not be of the highest level. Suppose the most important feature of your solution is the highest quality compared to your competitors. In that case, you cannot use this technology because the models resulting from this technology cannot compete with real specialized models in most cases.
Empowering Innovation:
Unleash the Potential of Deep Learning and Machine Learning at Saiwa
At Saiwa, we are proud to present "Deep Learning service", an exceptional service that is at the forefront of revolutionizing the field of deep learning and machine learning. With our cutting-edge platform, we offer comprehensive solutions tailored to your needs, enabling the training of neural networks using personalized datasets. Our service guarantees optimal performance and unmatched accuracy, empowering individuals and organizations alike to unlock valuable insights and drive innovation. With Saiwa's expertise in deep learning and machine learning, you can trust us to elevate your projects to new heights of success.
Experience trying Deep Learning at Saiwa for personalized neural network training. Unleash innovation with Saiwa's expertise in deep learning service.