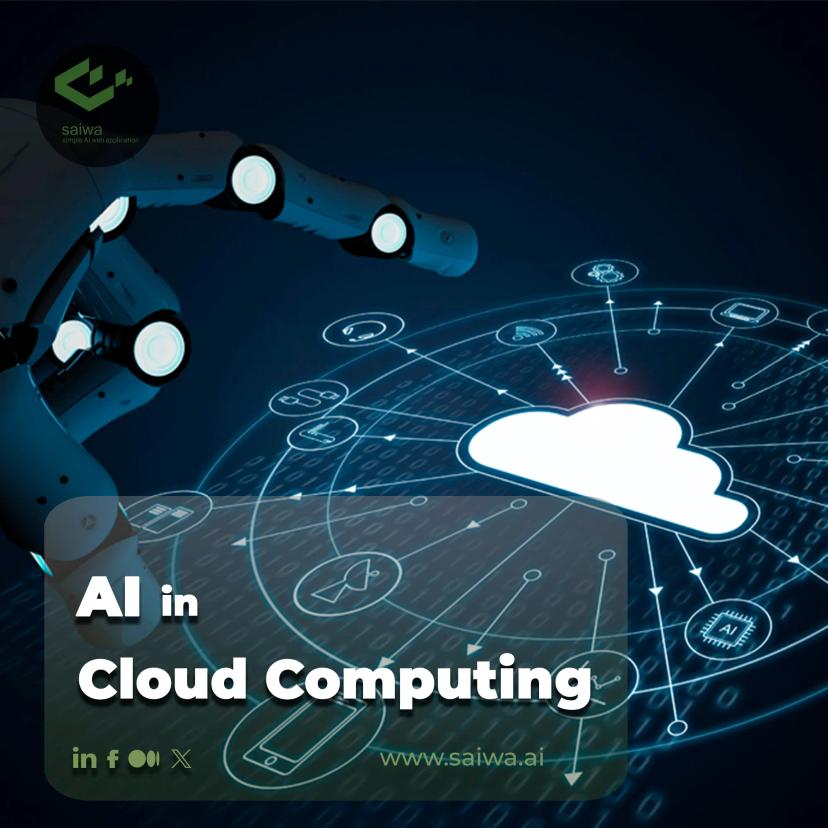
The Future of Artificial Intelligence in Cloud Computing
Artificial intelligence (AI) and cloud computing are two of the most transformative technologies of the 21st century. As AI continues to evolve and become more capable of handling complex tasks, integrating it with cloud computing platforms is enabling innovations and enhanced services. The combination of scalable computing power, vast data storage, and intelligent algorithms is ushering in an era of more efficient, automated, and insightful business processes and data analytics.
Cloud computing provides the infrastructure and services to develop and deploy AI applications at scale. The major cloud platforms offer integrated AI tools and services that simplify building, training, and managing machine learning models. Running artificial intelligence in cloud computing eliminates the high cost of owning and maintaining data centers while leveraging the flexibility to scale computing resources up or down based on real-time requirements. This has accelerated enterprise adoption of AI by making it accessible and cost-effective.
The Basics of Artificial Intelligence in Cloud Computing
Artificial intelligence refers to machines and systems that are capable of performing tasks that typically require human intelligence. This includes learning, reasoning, problem-solving, perception, prediction, and decision-making. Popular AI approaches include machine learning, deep learning, natural language processing, computer vision, and neural networks. Cloud computing provides on-demand access to computing resources, storage, and applications over the Internet on a pay-as-you-go basis. This enables rapid scaling to meet variable demand without investing in local servers or data centers.
There are three main categories of cloud computing services - Infrastructure as a Service (IaaS), Platform as a Service (PaaS), and Software as a Service (SaaS). IaaS offers access to infrastructure resources like virtual machines, storage, and networks. PaaS provides a cloud-based environment for developing, testing, and managing software applications. SaaS allows using web-based applications without needing to install any software locally. Cloud service models offer the flexibility and availability needed to develop, deploy, and operate AI apps efficiently.
AI Services and Applications in Cloud Computing
Cloud platforms empower various artificial intelligence in cloud computing use cases by providing virtually unlimited data storage and computing power to train machine learning models on huge diverse datasets. They also enable seamlessly serving AI predictions to applications accessed by millions of concurrent users. Major areas where managed cloud services are accelerating AI adoption include:
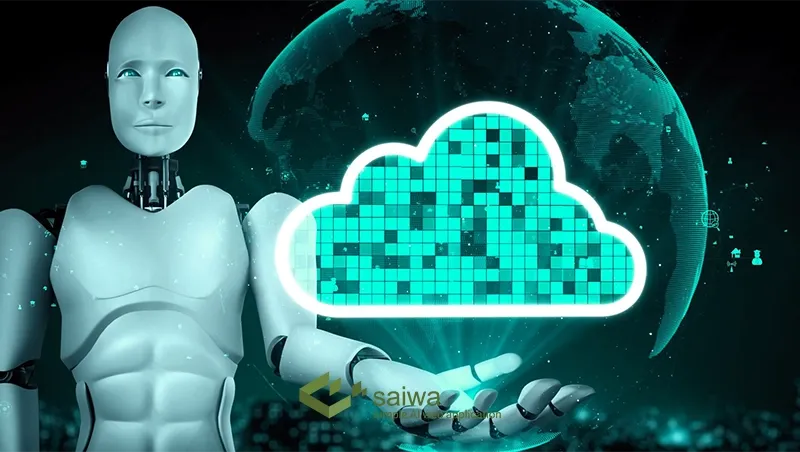
Data Management and Analytics
The cloud offers a centralized location for accumulating large structured and unstructured datasets required for training and continuously improving AI models. Cloud data lakes allow the consolidation of transactional, behavioral, and contextual data from across the organization into a single repository. Serverless data warehouse solutions on the cloud provide cost-effective SQL analytics at scale. Managed analytics services simplify exploring, processing, and visualizing vast data to uncover insights for business intelligence.
Core AI Services
Leading cloud providers offer pre-built AI services including speech recognition, language translation, text analytics, vision APIs, and conversational bots to help developers quickly infuse AI capabilities into applications without needing data science expertise. For example, optical character recognition and natural language processing services can extract meaning from documents and power intelligent workflows. These robust and scalable cloud-based cognitive services reduce the complexities of AI adoption.
AI-powered Cloud Operations
Artificial intelligence in cloud computing is being used to optimize cloud costs and resources. Machine learning analyzes usage patterns to recommend best-fit configurations for compute instances, storage, and databases to minimize infrastructure expenses. AI monitors cloud platform performance and security to enable self-healing capabilities and predictive maintenance. Chatbots handle IT service requests to enhance operational efficiencies. Autonomous cloud data centers use AI planning, scheduling, and automation.
Cloud-based AI for Enterprise
Many enterprise AI applications rely on the cloud to access the required compute intensity for model development and productization. HR analytics solutions apply vision APIs, speech recognition, and semantic analysis to screen resumes and conduct automated interviews. Cloud-hosted AI personalizes customer journeys by offering product recommendations and predicting churn. Smart assistants boost employee productivity by using speech interfaces to retrieve data, schedule meetings, and complete routine tasks. Supply chain optimizations leverage computer vision for tracking inventories and shipments.
Cloud Service Providers and their AI Platforms
The major players providing cloud infrastructure and platforms optimized for AI workloads include Amazon Web Services, Microsoft Azure, Google Cloud Platform, IBM Cloud, and Oracle Cloud.
AWS offers SageMaker for building, training, and deploying machine learning models on its cloud infrastructure. The collaboration with AWS empowers Saiwa to harness the full potential of cloud-based machine learning. This strategic integration with AWS positions Saiwa to meet the unique demands of the agricultural sector, offering innovative solutions that address the challenges faced by farmers.
Azure Machine Learning provides a drag-and-drop interface to prep data, train and manage models, and operationalize them for integration with applications. Google Cloud AI enables the developing of AI and analytics solutions using its advanced pre-trained models, AutoML functionality, and VPC architecture. IBM Cloud Annotations helps label and prepare unstructured data to train AI models leveraging Ground Truth. Oracle Cloud Infrastructure integrates AI services into its second-generation platform architecture to achieve better performance.
The availability of extensively documented SDKs, APIs, and specialized hardware like AI accelerators further simplifies leveraging the AI capabilities offered by cloud platform providers. Companies can choose the most appropriate vendor based on their specific business needs, pricing models, global presence, and existing technology partnerships.
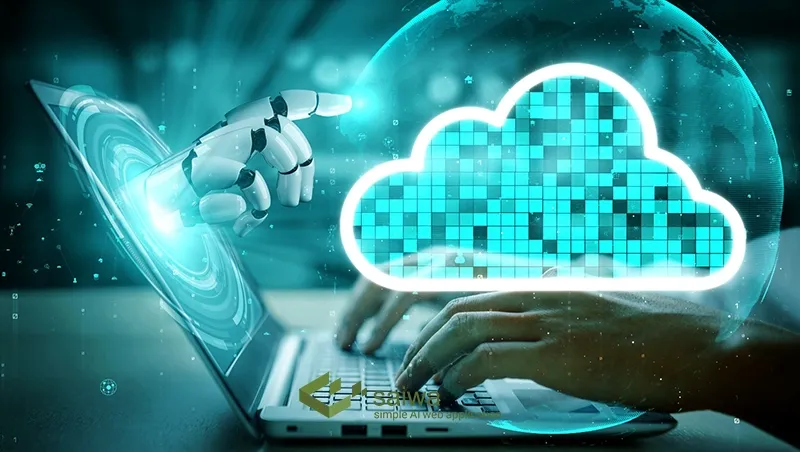
The Benefits of Using AI in Cloud Computing
The synergies between AI and cloud computing offer multiple advantages that are accelerating AI adoption across industries:
Cost Savings
Cloud infrastructure allows leveraging AI capabilities on demand instead of investing in on-premise high-performance computing resources which have significant capital and operational expenses. The pay-per-use pricing lowers the total cost of ownership.
Scalability
Serverless artificial intelligence in cloud computing platforms provides virtually unlimited scale to handle enterprise data volumes required for model development and production deployments capable of serving millions. This simplifies innovations.
Speed and Agility
Pre-built AI building blocks and automated machine learning solutions help prototype and experiment quickly. Faster time to market gives a competitive advantage.
Security
Cloud service providers implement robust physical and application-level security, timely software updates, and advanced cyber threat detection leveraging AI which exceeds enterprise capabilities.
Reliability
Multiple availability zones, failover mechanisms, and redundancy offered by cloud data centers ensure resilience for mission-critical AI apps.
Collaboration
Cloud-based development environments connect distributed teams to collectively work on AI projects and integrate with DevOps pipelines for continuous delivery.
Ethical Considerations in the Cloud Computing AI Era
One of the most important concerns is bias in AI algorithms. Training data stored and processed in the cloud can unknowingly perpetuate historical biases, leading to discriminatory outcomes. Cloud computing AI platforms need robust mechanisms for auditing data sets and mitigating bias throughout the development process.
Security is another paramount concern. Cloud computing AI systems store and analyze vast amounts of sensitive data. Stringent security protocols within cloud platforms are essential to prevent unauthorized access and data breaches. Additionally, robust encryption practices for data at rest and in transit are crucial.
Accountability is a complex issue in cloud computing AI. When AI models make decisions that have negative consequences, who is responsible? Cloud providers, application developers, or the end-users? Clear lines of accountability need to be established to ensure responsible development and deployment of cloud computing AI.
To navigate these ethical considerations, collaboration is key. Cloud computing AI providers, developers, and users all have a role to play. Transparency in development processes, clear communication about limitations, and ongoing monitoring are essential.
By proactively addressing ethical concerns, we can ensure that cloud computing AI is a force for good, fostering innovation and progress while upholding ethical principles.
Conclusion
The integration of artificial intelligence in cloud computing platforms is unlocking unprecedented capabilities through a synergistic combination of cutting-edge technologies. AI adds an intelligent layer of automated decision-making, insights discovery, and predictive recommendations to scale cloud infrastructure and services. The union of abundant computing capacity, storage for massively diverse data, and algorithms capable of learning from such varied information is driving unparalleled innovation.
As AI cloud adoption gains momentum across industries, companies need to formulate deliberate strategies for harnessing these capabilities based on their specific business context instead of ad hoc implementations. A systematic approach includes assessing use cases, auditing datasets, consolidating data pipelines, determining required skills, evaluating cloud vendors, accounting for governance needs, and mapping realistic milestones. Partnerships with specialized AI cloud platform providers can effectively navigate enterprises through the end-to-end AI cloud journey to transform processes and evolve offerings leveraging actionable intelligence