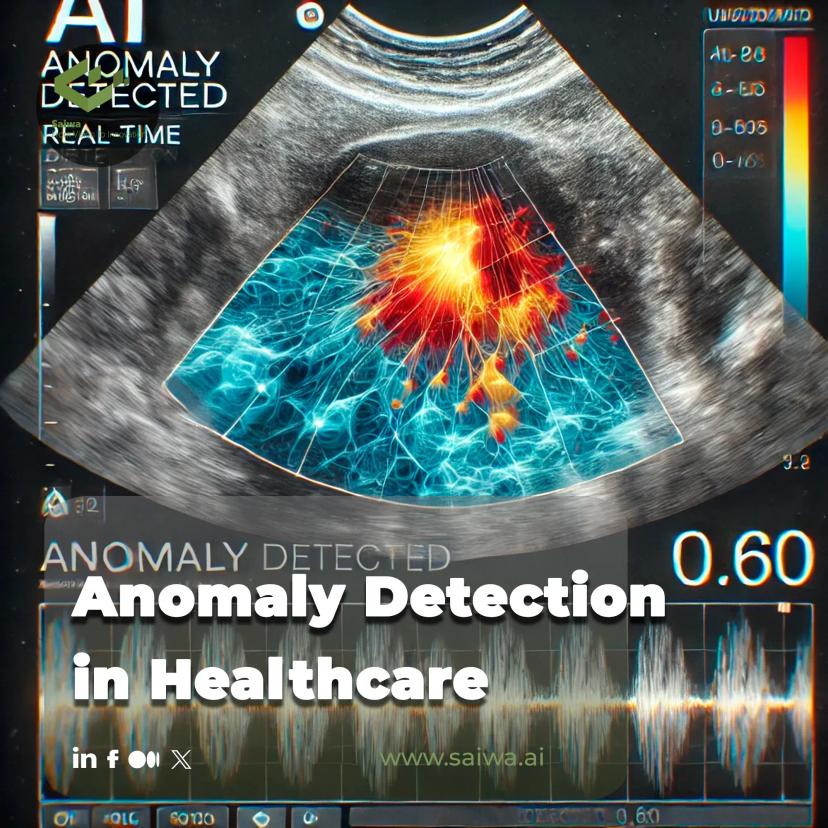
Anomaly Detection in Healthcare | Enhancing Patient Care, Safety and Efficiency
Anomaly detection, a critical component of data analytics, plays a vital role in several industries, including healthcare. It involves identifying unusual patterns, or outliers, in data sets that deviate significantly from established norms or expected behavior. In the context of healthcare, these anomalies can represent a wide range of critical events, from the early onset of disease to fraudulent activity and operational inefficiencies. The ability to detect these anomalies quickly and accurately can have a significant impact on patient care, improve operational efficiency, and reduce healthcare costs.
Saiwa, an AI-driven company, provides innovative solutions through its Fraime platform, which uses advanced machine learning algorithms to detect anomalies in various industries. Designed to handle complex data sets, Fraime provides services such as deep learning, image processing, annotation, and detection. With its robust capabilities, the Fraime Anomaly Detection Service is transforming industries such as healthcare, where it helps detect disease early, prevent fraud, and improve operational processes by identifying outliers that may go unnoticed by traditional methods.
This article explores the importance, applications, techniques, challenges, and future directions of anomaly detection in healthcare.
Importance of Anomaly Detection in Healthcare
The healthcare industry generates massive amounts of data, encompassing patient records, medical images, clinical trials data, and operational logs. Within this data lie hidden insights that can be instrumental in improving healthcare delivery and patient outcomes. Anomaly detection provides a powerful tool for uncovering these insights by identifying patterns that deviate from the norm. This capability is crucial for several reasons:
Proactive Intervention
Early detection of anomalies can enable proactive interventions, particularly in disease diagnosis and treatment. Identifying subtle deviations from normal physiological parameters can indicate the early stages of a disease, allowing for timely treatment and potentially preventing disease progression.
Enhanced Decision-Making
Anomaly detection empowers healthcare professionals with data-driven insights to make more informed decisions. By identifying unusual patterns in patient data, clinicians can gain a deeper understanding of individual patient conditions and tailor treatment plans accordingly.
Improved Resource Allocation
Identifying anomalies in operational data can help optimize resource allocation. Detecting unusual patterns in hospital admissions, emergency room visits, or medication usage can assist in predicting demand and allocating resources effectively.
Fraud Prevention and Detection
Anomaly detection plays a crucial role in identifying fraudulent activities within the healthcare system. Detecting unusual billing patterns, prescription drug abuse, or insurance claims fraud can help prevent financial losses and protect patients from harm.
Applications of Anomaly Detection in Healthcare
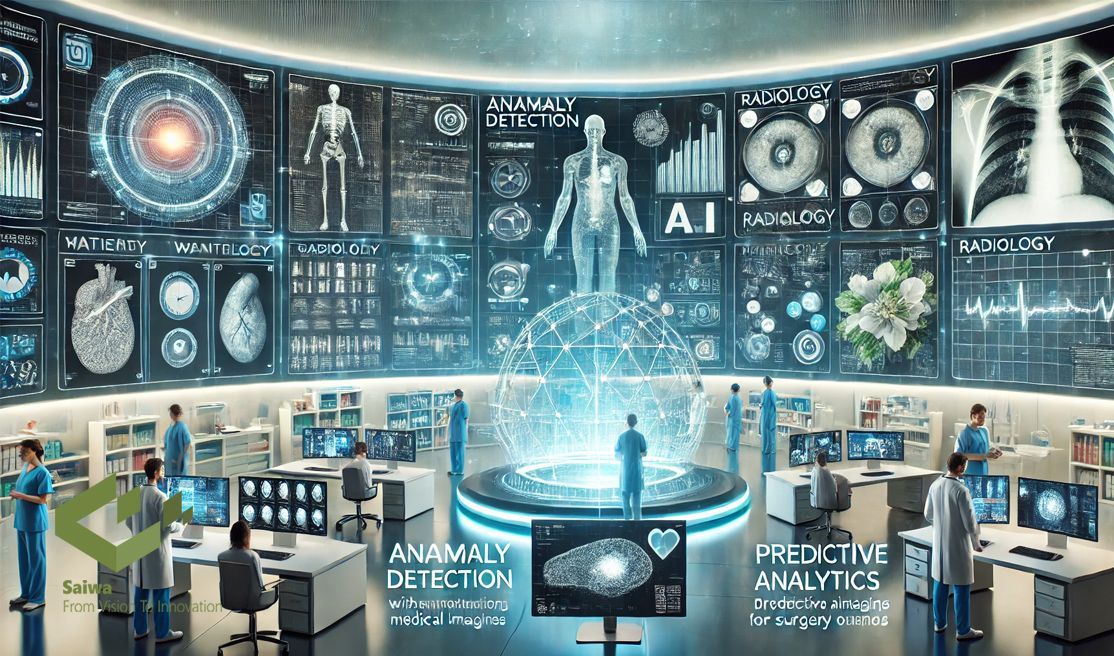
The applications of anomaly detection in healthcare are diverse and constantly expanding. Some key areas where anomaly detection is making a significant impact include:
Early Disease Detection
Anomaly detection offers the potential for early disease detection by identifying subtle changes in patient data that may not be apparent through traditional diagnostic methods. By analyzing physiological data, such as heart rate, blood pressure, and glucose levels, anomaly detection algorithms can identify deviations from normal patterns that may indicate the early onset of diseases like diabetes, heart disease, or cancer.
This early detection can enable timely interventions, improving treatment outcomes and potentially saving lives. Furthermore, anomaly detection can be applied to medical images, such as X-rays, CT scans, and MRI scans, to identify subtle abnormalities that may indicate the presence of tumors or other diseases.
Fraud Detection
Healthcare fraud is a significant problem that costs the industry billions of dollars annually. Anomaly detection techniques can be employed to identify fraudulent activities, such as insurance claims fraud, prescription drug abuse, and medical identity theft.
By analyzing billing patterns, prescription records, and insurance claims data, anomaly detection algorithms can identify suspicious activities that warrant further investigation. This can help prevent financial losses, protect patients from harm, and maintain the integrity of the healthcare system.
Improving Patient Safety
Anomaly detection can contribute to improved patient safety by identifying potential risks and adverse events. By analyzing patient data, such as vital signs, medication dosages, and lab results, anomaly detection algorithms can identify deviations from established safety protocols or expected physiological responses.
This can alert healthcare providers to potential adverse events, such as medication errors, allergic reactions, or infections, allowing for timely interventions and preventing patient harm. Furthermore, anomaly detection can be used to identify anomalies in medical device data, such as heart rate monitors and ventilators, to detect malfunctions or potential safety issues.
Enhancing Operational Efficiency
Anomaly detection can be applied to operational data to identify inefficiencies and optimize resource allocation. By analyzing data related to hospital admissions, emergency room visits, staffing levels, and bed utilization, anomaly detection algorithms can identify unusual patterns that may indicate bottlenecks, delays, or overutilization of resources.
This information can be used to improve workflow processes, optimize staffing schedules, and allocate resources more effectively, leading to increased operational efficiency and reduced healthcare costs.
Anomaly Detection Techniques in Healthcare
A variety of techniques are employed for anomaly detection in healthcare, each with its strengths and limitations. These techniques can be broadly categorized into:
Statistical Methods
Statistical methods rely on statistical principles to identify data points that deviate significantly from the expected distribution. Common statistical methods used in anomaly detection include:
Control Charts: Control charts are used to monitor processes over time and identify data points that fall outside of pre-defined control limits.
Hypothesis Testing: Hypothesis testing is used to determine whether a observed difference between two groups is statistically significant.
Time Series Analysis: Time series analysis is used to analyze data collected over time and identify trends, seasonality, and anomalies.
Machine Learning Algorithms
Machine learning algorithms are increasingly being used for anomaly detection in healthcare due to their ability to learn complex patterns from data. Common machine learning algorithms used for anomaly detection include:
Clustering: Clustering algorithms group similar data points together and identify outliers that do not belong to any cluster.
Classification: Classification algorithms are trained to classify data points into different categories, such as normal and anomalous.
Regression: Regression algorithms are used to predict a continuous variable based on other variables and identify data points that deviate significantly from the predicted value.
One-Class SVM: One-Class Support Vector Machines are particularly useful for anomaly detection as they learn a boundary around the normal data and identify data points that fall outside of this boundary.
Rule-Based Systems
Rule-based systems rely on pre-defined rules to identify anomalies. These rules are typically based on expert knowledge or established guidelines. While rule-based systems can be effective for detecting known anomalies, they may not be able to detect novel or unexpected anomalies.
Real-Life Examples of Detecting Healthcare Anomalies
Several real-world examples demonstrate the successful application of anomaly detection in healthcare:
Detecting sepsis early using vital signs and lab results
By continuously monitoring vital signs like temperature, heart rate, respiratory rate, and blood pressure, coupled with lab results such as white blood cell counts and lactate levels, AI algorithms can detect subtle deviations from normal patterns that may indicate the onset of sepsis much earlier than traditional methods.
This early detection allows for prompt intervention with antibiotics and other supportive care, significantly improving patient outcomes and reducing mortality rates. The algorithms can be trained on large datasets of patient data to identify complex patterns indicative of sepsis, even in cases where individual vital signs or lab results may not be alarming on their own.
Identifying fraudulent insurance claims based on billing patterns
Anomaly detection algorithms can analyze vast amounts of insurance claims data to identify suspicious billing patterns, such as unusually high charges for specific procedures, discrepancies between diagnoses and treatments, or claims submitted by providers with a history of fraudulent activity.
These algorithms can flag potentially fraudulent claims for further investigation by human reviewers, helping to prevent financial losses due to fraud and ensuring that resources are allocated appropriately. Machine learning models can be trained to recognize complex patterns of fraud that may be difficult for humans to detect.
Predicting hospital readmissions based on patient characteristics and discharge summaries:
By analyzing patient data such as age, medical history, diagnoses, medications, and information from discharge summaries, including post-discharge care instructions and follow-up appointments, AI models can predict the likelihood of a patient being readmitted to the hospital within a certain timeframe.
This allows healthcare providers to implement targeted interventions, such as providing additional post-discharge support, coordinating care with primary care physicians, and ensuring medication adherence, to reduce the risk of readmission and improve patient outcomes.
Detecting adverse drug reactions based on patient medication history and physiological data:
Anomaly detection algorithms can analyze patient medication records, along with physiological data such as heart rate, blood pressure, and lab results, to identify potential adverse drug reactions (ADRs).
These algorithms can detect subtle changes in physiological parameters that may indicate an ADR, even if the changes are not immediately apparent to healthcare providers. Early detection of ADRs can allow for prompt intervention, such as discontinuing the medication or providing supportive care, minimizing the severity of the reaction and improving patient safety.
Optimizing hospital bed utilization based on admission patterns and patient flow:
By analyzing historical data on hospital admissions, discharges, patient demographics, and seasonal trends, AI can predict future bed demand and identify potential bottlenecks in patient flow. This information can be used to optimize bed allocation, staffing levels, and discharge planning, ensuring that beds are available for patients who need them and minimizing delays in patient care.
Predictive models can also be used to anticipate surges in demand due to events such as flu outbreaks or natural disasters, allowing hospitals to proactively allocate resources and prepare for increased patient volume.
Challenges of Anomaly Detection in Healthcare
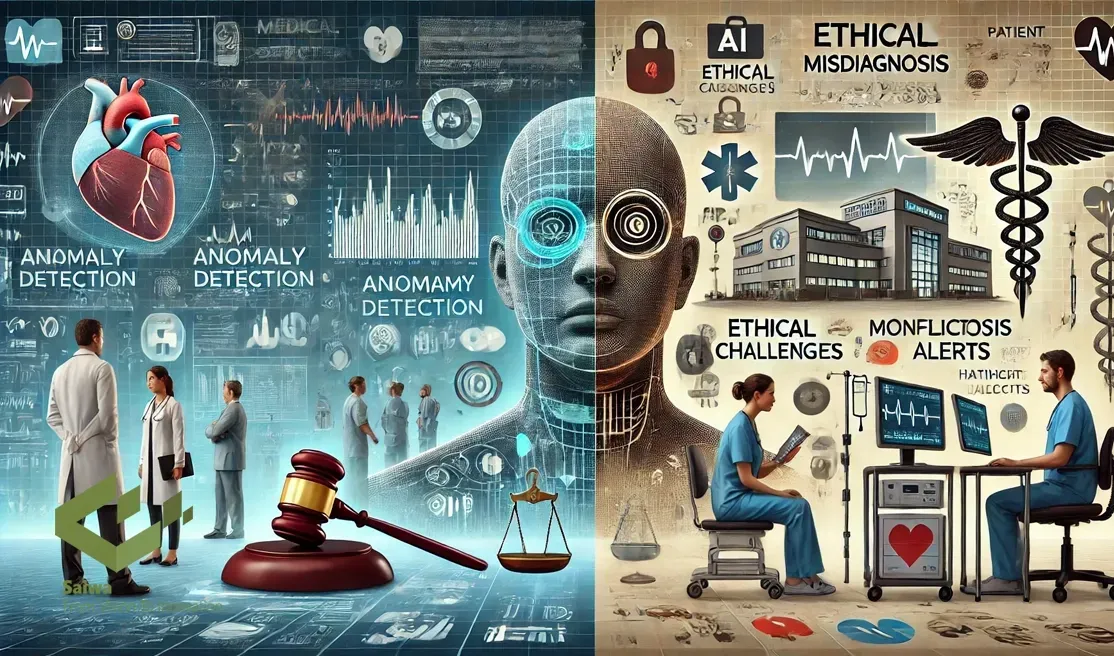
Despite the significant potential of anomaly detection in healthcare, several challenges need to be addressed:
Data Quality and Availability
Healthcare data is often incomplete (missing values), inconsistent (different formats and coding systems), and noisy (errors and inaccuracies). This poor data quality can significantly hinder the performance of anomaly detection algorithms, leading to false positives or false negatives.
Furthermore, accessing and integrating data from disparate sources like EHRs, labs, and imaging systems is challenging due to interoperability issues and strict data privacy regulations like HIPAA. Data preprocessing and cleaning are crucial but complex tasks.
Interpretability
Many powerful machine learning algorithms, especially deep learning models, are complex and opaque, making it difficult to understand why a specific data point is flagged as anomalous. This lack of transparency can erode trust among healthcare professionals, hindering the adoption of these potentially life-saving techniques in clinical practice. Explainable AI (XAI) methods are being developed to address this issue.
Real-Time Detection
Many critical healthcare applications, such as ICU monitoring and sepsis detection, demand real-time anomaly detection. However, processing and analyzing the vast volumes of streaming data generated in these settings requires significant computational resources and specialized infrastructure. Latency in anomaly detection can have serious consequences. Edge computing and optimized algorithms are needed for real-time performance.
Conclusion
Anomaly detection holds immense potential for transforming healthcare by improving disease detection, enhancing patient safety, optimizing operational efficiency, and preventing fraud. While challenges remain, ongoing advancements in data analytics and machine learning are paving the way for more sophisticated and effective anomaly detection techniques. As the volume and complexity of healthcare data continue to grow, anomaly detection will play an increasingly critical role in unlocking valuable insights and improving healthcare outcomes.