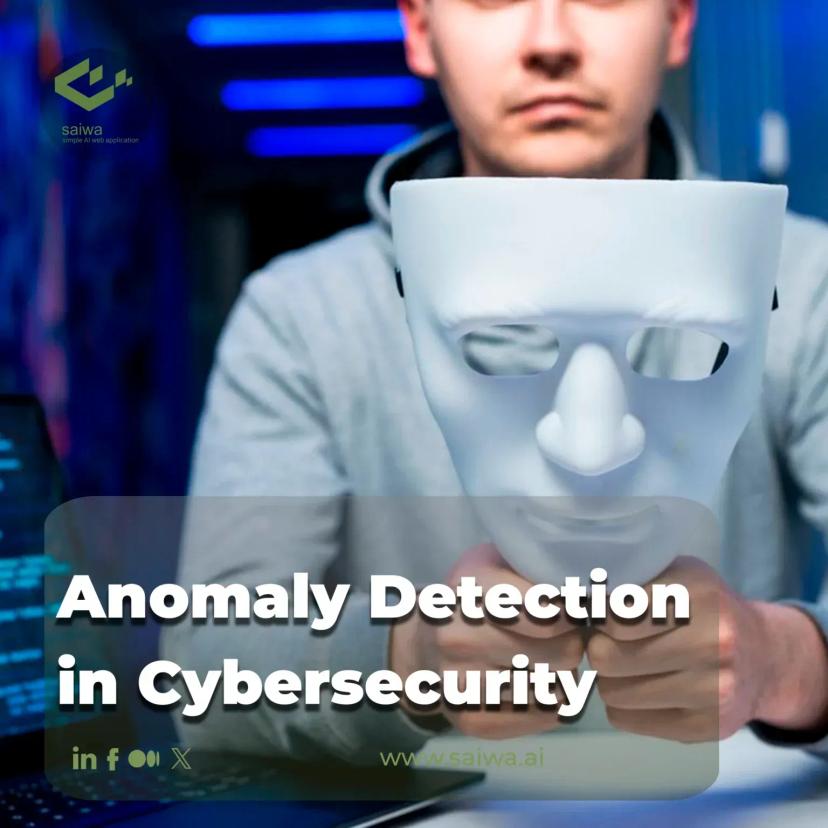
Everything You Need to Know About Anomaly Detection in Cybersecurity
Anomaly detection involves identifying uncommon events, observations, or incidents that drastically deviate from the expected behavior displayed in intricate systems such as computer networks. Analyzing large amounts of operational data using algorithms fine-tuned to differentiate between background noise and critical outliers, cybersecurity teams acquire essential threat detection capabilities. This allows them to detect previously unseen intrusions, solely based on abnormal deviations relative to learned visibility. As a result, teams no longer have to rely on static signature rulebooks containing only known attacks.
Dynamic behavioral profiles that describe standard access patterns, data flows, and privileged operations establish essential baselines to detect anomalies when adversaries breach the perimeter through social engineering, malware, or compromised insider credentials. These incidents often trigger cascading effects internally. The most significant risks tend to be hidden in plain sight, masquerading as routine activities rather than the dramatized versions often seen in Hollywood. Anomaly detection in cybersecurity solutions combats this issue by providing data-driven visibility into detailed system interactions and user digital footprints. This creates comprehensive, differentiable snapshots of the system that are precisely fine-tuned to identify true positive threats.
Enhancing rule-based security systems with regularly updated violation flags, powered by unsupervised machine learning and ensemble models, offers flexible protection even against zero-day threats that lack prior documented examples. By consolidating industry expertise with modern AI that continuously parses detailed system telemetry, robust anomaly detection provides essential threat signals. This achieves what manual reviews cannot achieve, given the inability to realistically scale across exponentially vast networks.
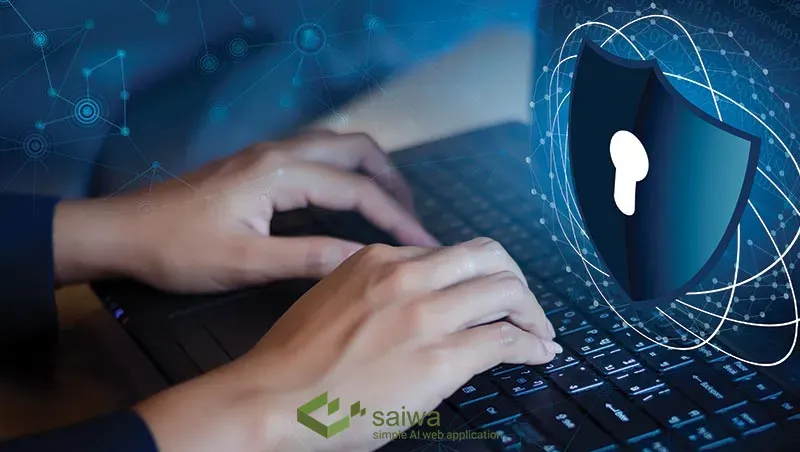
Network Activity Profiling
Effective behavioral analysis fundamentals first require clarity on what normal baseline activity looks substantiates across constantly evolving enterprise systems.
Data Aggregation and Clustering
This demands intelligently aggregating and clustering massive volumes of identity access logs, network traffic logs, DNS queries, endpoint security events, and vulnerability reports documenting layered interactions between users, devices, applications, and data repositories through time.
Multidimensional profiling
Multidimensional profiling analyzes trends in access locality, including remote authentication from atypical locations, device pairings that are unlikely, irregular user access patterns, and functionally uncharacteristic resource access permissions. Our focus also extends to application traffic metrics, such as unusual outbound data payloads, irregular packet destinations, and privileged process actions that are not strictly necessary for elevated commands.
Ongoing profile optimization adjusts detection rules in response to major enterprise changes, such as mergers, cloud migrations, or new application onboarding. Assumptions built initially on outdated archetypes are recalibrated until stability allows for confidently tighter thresholds. Anomaly detection relies on accurately modeled baselines that reliably flag deviations.
Types of Cyber Anomalies
Although insider threats pose significant risks, especially through compromised credentials or social manipulation, external threats still dominate in terms of frequency. Brute force authentication attacks, trojan malware infections, and supply chain software vulnerabilities that are waiting to be exploited after being discovered remain the most common external threats. Careful anomaly detection in cybersecurity addresses both fronts. It employs supervised rules that characterize suspicious access attempts, unsupervised learning to establish typical traffic patterns, and semi-supervised hybrid approaches that are trainable on known incident data. The system continuously evolves to defend against new attack developments in the wild.
Granular clues such as uncommon outbound traffic payloads, anomalous encryption of endpoint resources, irregular communications with DNS servers, and distorted geo-locations due to GPS all suggest possible compromise events deserving of alerts for further investigation. Ensemble aggregates layering insights to exemplify high-value threat indicators unspottable in isolation, but clear through holistic cross-analysis. These insights include off-hour logins from foreign countries on devices unused by the employee before. The cross-analysis provides multi-variable correlations characteristic of breach behavior, and camouflages. Careful tuning balances security sensitivity with functionality disruptiveness, given some anomalies that are inevitable through normal operations at scale.
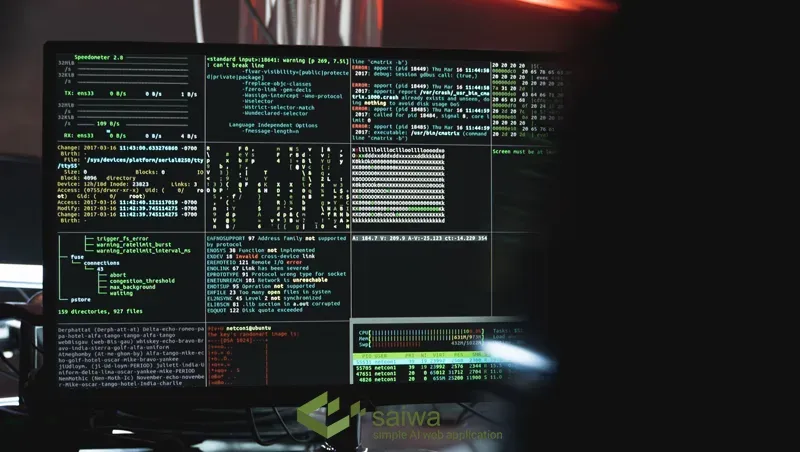
Challenges Detecting Unknown Threats
Adversaries expend significant resources intentionally evading and undermining defensive measures via social engineering maneuvers, easily circumventing traditional protocols. Nation-state groups and cyber criminals with strong motivations study network topologies and operational patterns, tailoring attack vectors that slide past static protections. Additionally, compromised insiders abuse inherent trust and permissions, sidestepping basic access filters.
The constantly evolving attack sophistication necessitates intelligent adaptive techniques to keep up. Nevertheless, the variety of contemporary digital ecosystems, including cloud microservices, legacy mainframes, and connected IoT devices, significantly complicates behavioral modeling. This poses a challenge in tracking cross-dependencies and privilege escalation paths across complex systems. Carefully defining the scope of monitoring and implementing gradual baselining can facilitate the management of the initial complexity encountered during enterprise anomaly detection rollouts. By prioritizing identity management and monitoring of email security, monitoring foundations can be laid that offer broad initial coverage.
Anomaly Detection in Cybersecurity Techniques
There are various outlier detection algorithms with distinct advantages, through multidimensional data representations, weighted scoring combiners, and tiered escalation workflows. Businesses can optimize the discovery of threat indicators while minimizing false positives. Incidents that exhibit multiple characteristic signals of misuse and malice are the only ones that escalate.
Traditional Vs. Advanced Methods
Traditional methods, such as principal component analysis, isolation forests, and Kalman filters, provide easily understandable threat indicators that technicians of all skill levels can access. In contrast, clustering algorithms and neural networks achieve greater detection accuracy for ever-evolving telemetry patterns and newly labeled incidents of shifting attack developments. Balancing these approaches ensures simultaneous monitoring of known and unknown threats.
Optimizing Anomaly Detection in Cybersecurity
No one solution can maximize all statistical efficacy metrics. However, given the inherent technique tradeoffs, combining supervised and unsupervised methods and making analyst-informed configuration adjustments can overcome the limitations of isolated methods. Leveraging ensemble strengths while smoothing individual accuracy shortcomings through heuristics counterbalances said limitations. Effective anomaly detection in cybersecurity requires a balanced approach, blending the strengths of machine learning with human expertise in responding to incidents and identifying blind spots.
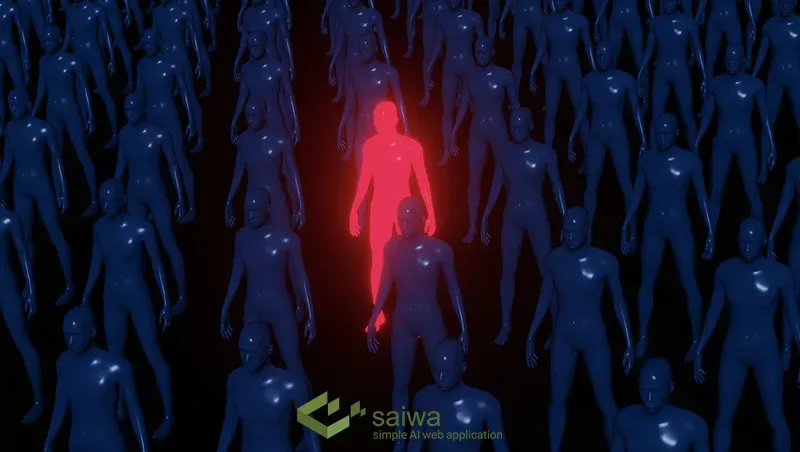
Tools and Mitigation Capabilities
Converting anomaly detection into prevention of damages, rather than solely relying on reactive notifications, is heavily dependent on response workflow tools that automate actions in response to incidents; for example, disabling user accounts temporarily, enforcing password changes, isolating VLAN network segments, and suspending irregular outbound data payloads that show command and control traffic signatures. Integrated SOAR playbooks incorporate escalating containment procedures, enabling human SOC teams to selectively intervene at critical junctures like high-privileged user alerts, before systems unilaterally issue disruptive suspension commands.
Streamlining diagnostic procedures through consolidated dashboards that trace anomalies back to patient zero devices, abnormal authentication nodes, and malware entry points provides efficient starting points for assessing the severity of compromises, identifying eradication needs, and establishing recovery steps to confidently restore typical business operations after threats have been neutralized and mitigated through decisive response protocols. Anomaly detection in cybersecurity, supported by strategic orchestration, reduces attacker footholds by translating discoveries into secure enterprises using practical toolsets.
Conclusion
Anomaly detection in cyber security is crucial in defending against the ever-changing nature of cyber threats. By conducting thorough profiling of network activity, organizations can effectively gather and analyze large datasets, resulting in a detailed comprehension of normal baseline activity. This knowledge enables identifying cyber anomalies, such as unusual remote authentication locations and irregular packet destinations. This approach offers a comprehensive defense against potential threats by exploring different types of anomalies that highlight the diverse nature of potential threats, ranging from irregular outbound data payloads to privileged process actions.