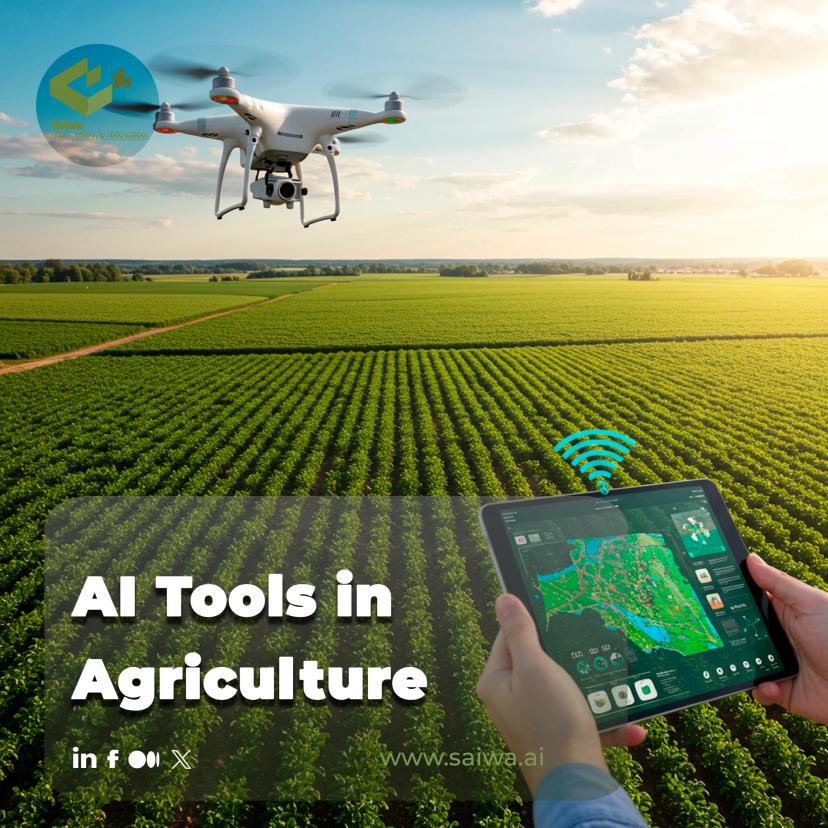
AI Tools in Agriculture | Detect, Predict, and Protect Your Crops
Farming has always been about working with nature, but today it's changing in ways that were once unimaginable. As the world faces greater challenges, from unpredictable weather to the need to grow more food with fewer resources, farmers are looking for smarter solutions. That's where artificial intelligence (AI) comes in. AI is changing the game by providing tools that not only help farmers grow more food, but do so in a way that's better for the environment. By making farming more efficient, reducing waste, and improving decision-making, AI is helping to shape a more sustainable future for agriculture.
Sairone, developed by Saiwa, is a powerful AI and machine learning platform designed to improve farming operations. Integrated with drones, Sairone provides key capabilities such as detecting invasive species, estimating nitrogen levels, and monitoring crop health, helping farmers make data-driven decisions that increase productivity and sustainability.
This article explores how AI is becoming a key part of agriculture and highlights the many AI tools that are changing farming practices. These tools are making farming more efficient and sustainable.
The Growing Role of AI in Solving Agricultural Challenges
AI is no longer a future concept in agriculture-it's being used today to address some of the biggest challenges facing farmers today. From changing weather patterns to limited resources, AI in agriculture is helping to bring greater accuracy and speed to everyday decisions. It's not just about using technology - it's about using it wisely, where it matters most.
Behind this transformation is a growing set of smart tools that are quietly changing the way farms operate. These technologies are helping farmers see more, react faster, and work more efficiently-all with fewer inputs and greater results. As AI continues to evolve, its impact is becoming more visible in the field. So what do these tools look like in the field? Let's take a closer look at the technologies that are driving this change.
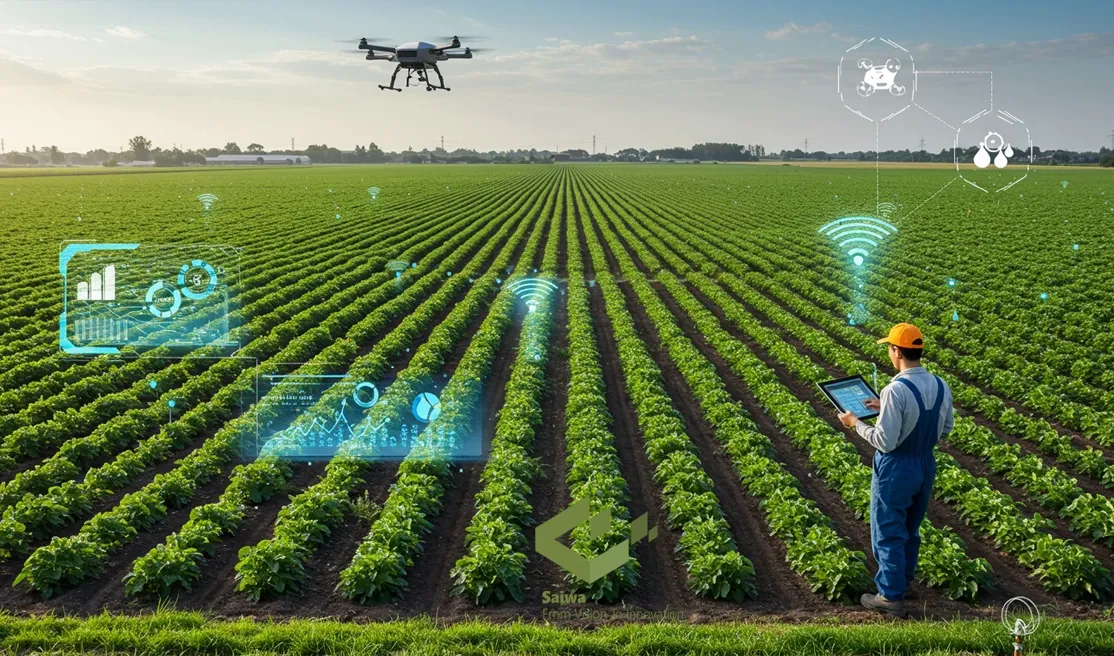
Exploring the Range of AI Tools in Agriculture
The applications of AI in agriculture are diverse and rapidly expanding, encompassing a wide range of tools and technologies designed to address specific challenges and optimize various aspects of farming operations.
These AI-powered tools leverage advancements in machine learning, computer vision, and data analytics to provide farmers with valuable insights and automation capabilities, ultimately leading to increased efficiency, improved yields, and enhanced sustainability. The integration of these tools into existing farming practices is revolutionizing the agricultural landscape and paving the way for a more data-driven and technologically advanced future.
Weed Detection
AI-powered weed detection systems utilize computer vision and machine learning algorithms to identify and differentiate weeds from crops with remarkable precision. This technology enables targeted herbicide application, reducing chemical usage, minimizing environmental impact, and optimizing weed control efforts. By precisely targeting weeds, AI-powered systems minimize herbicide resistance development, protect beneficial plants and insects, and promote sustainable farming practices. This targeted approach also reduces costs associated with herbicide purchase and application, contributing to increased farm profitability.
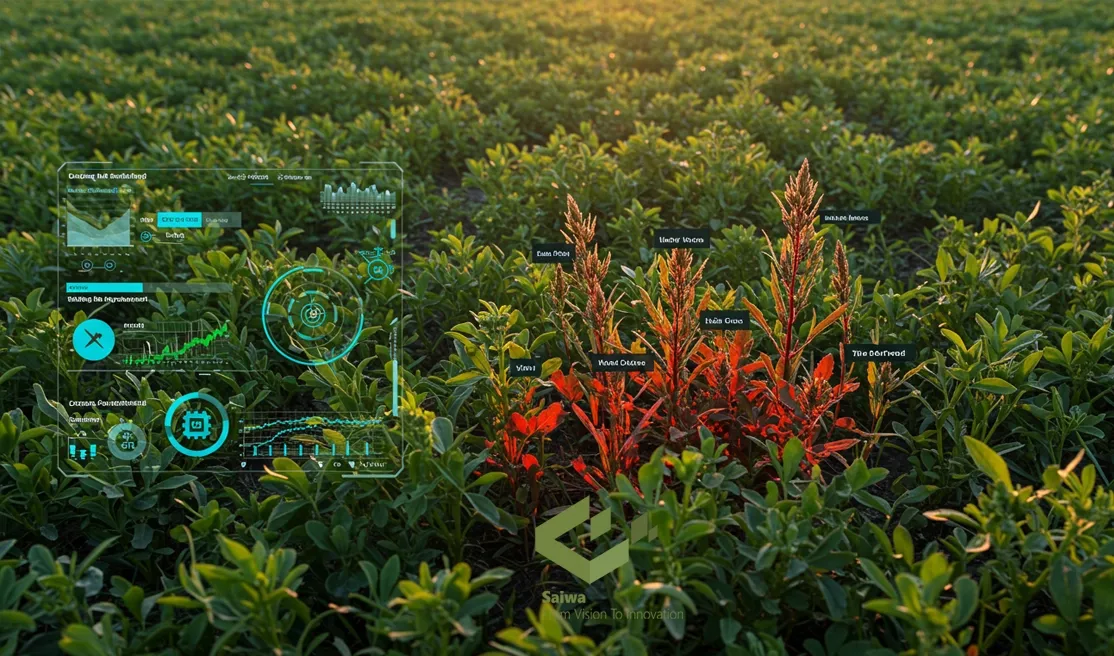
Crop Counting
Accurate crop counting is crucial for yield prediction, resource allocation, and informed farm management decisions. AI-powered systems automate plant counting with remarkable accuracy, eliminating the need for time-consuming and error-prone manual counting methods.
Drones equipped with high-resolution cameras capture aerial images of fields, and AI algorithms analyze these images to identify and count individual plants, providing precise data for informed decision-making regarding planting density, fertilization, and other management practices. This data also facilitates accurate yield predictions and enables more efficient resource allocation.
Invasive Species Detection
Invasive species pose a significant threat to agricultural ecosystems, outcompeting native crops, disrupting biodiversity, and introducing diseases. AI algorithms are trained to recognize and differentiate invasive species from native plants, enabling early detection and rapid response to invasive species threats.
Early intervention prevents their spread, minimizes their impact on agricultural ecosystems and biodiversity, and helps protect native flora and fauna in invasive species monitoring. This proactive approach can significantly reduce the economic and ecological damage caused by invasive species.
Crop Yield Prediction
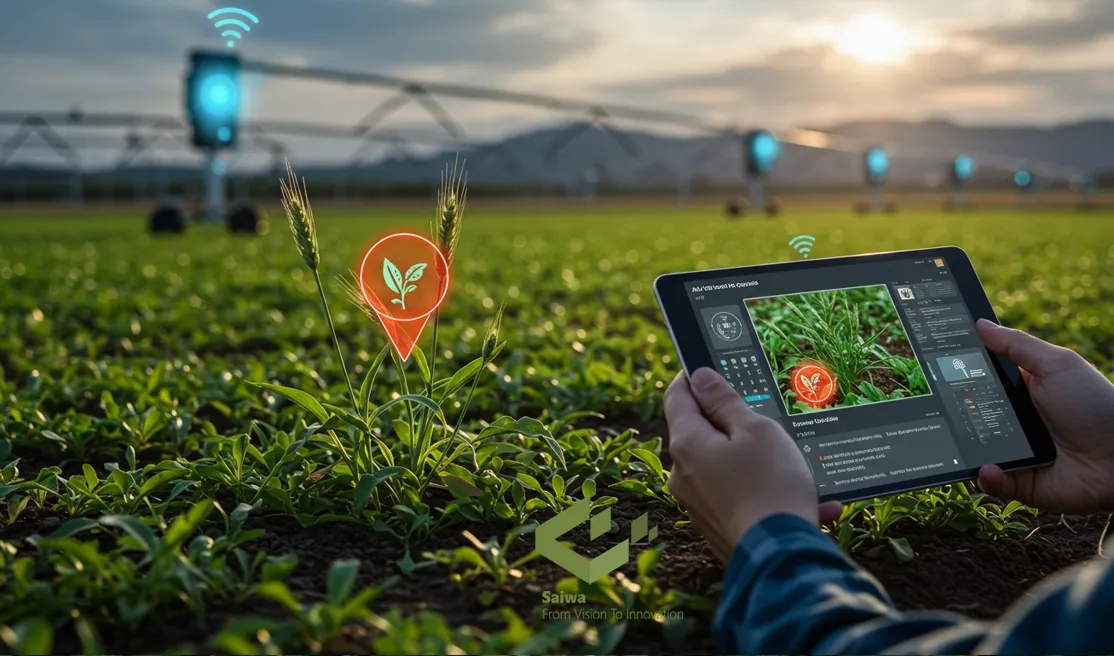
Predicting crop yields accurately is crucial for effective farm management, resource allocation, and market planning. AI-powered systems analyze various data points, including historical yield data, weather patterns, soil conditions, crop health indicators, and real-time sensor data, to provide accurate crop yield prediction using machine learning.
This information enables farmers to make informed decisions regarding planting, harvesting, storage, logistics, and marketing, optimizing their operations and maximizing profitability. Accurate yield predictions also facilitate better planning for labor requirements and market negotiations.
Nitrogen Analysis
Nitrogen is a vital nutrient for plant growth, but excessive or insufficient nitrogen levels can negatively impact crop health, yield, and environmental quality. AI-powered tools assess nitrogen levels in crops and soil using spectral analysis and other advanced techniques, providing valuable data for precision nitrogen in plant management. This data enables optimized fertilizer application, minimizing nutrient runoff, reducing environmental pollution, enhancing environmental sustainability, and optimizing fertilizer costs. This precise approach promotes responsible environmental stewardship and contributes to healthier ecosystems.
Farm Data Analytics
AI-powered farm data analytics platforms integrate data from various sources, including sensors, drones, weather stations, farm management software, and historical records, to provide farmers with a comprehensive view of their operations. These platforms utilize machine learning algorithms to identify patterns, trends, and anomalies in the data, providing valuable insights for optimizing resource utilization, improving crop management, enhancing decision-making, and identifying areas for improvement. This data-driven approach empowers farmers to make more informed and strategic decisions.
Crop Monitoring
Real-time crop monitoring is essential for detecting early signs of stress, disease, or nutrient deficiencies. AI-powered systems utilize , sensors, satellite and drone imagery, and other remote sensing technologies to monitor crop health and growth continuously. This data is analyzed by AI algorithms to identify potential problems, enabling timely interventions, preventing widespread crop damage, and optimizing resource application. This proactive approach minimizes yield losses and maximizes resource use efficiency.
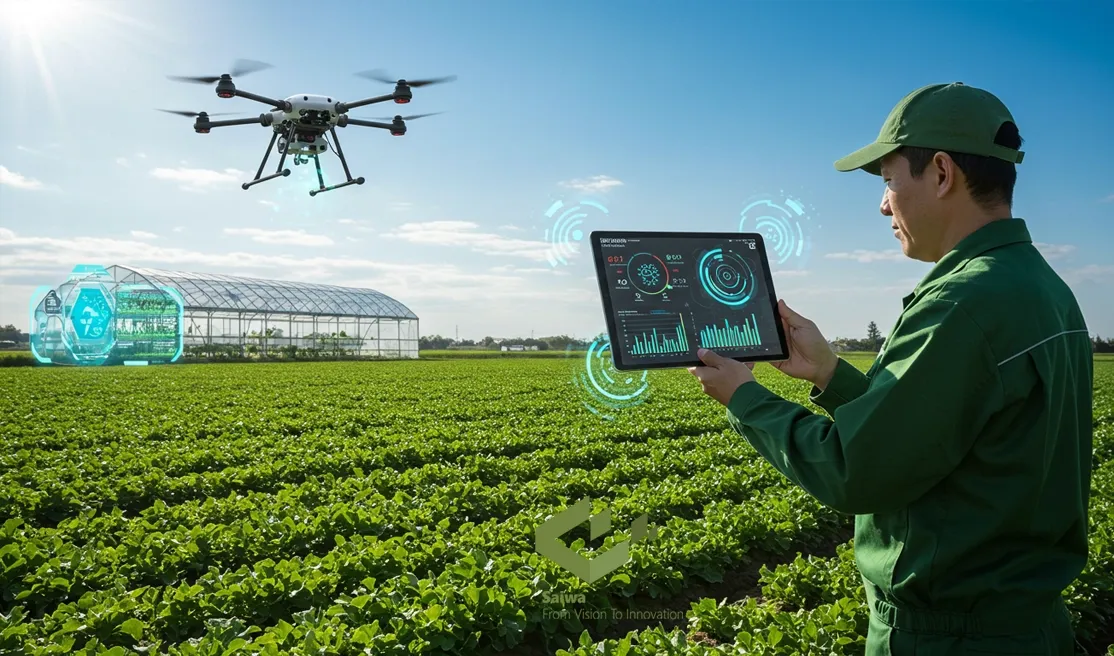
Pest Identification and Detection
Pests can cause significant crop damage, reducing yields and impacting food quality. AI-powered systems detect and identify various pests, including insects, rodents, and birds, using image recognition and machine learning techniques, providing valuable information for targeted AI in pest control measures. This information enables precise application of pesticides, reducing overall pesticide usage, minimizing environmental impact, protecting beneficial insects and pollinators, and promoting a more balanced ecosystem. This targeted approach also reduces costs associated with pesticide purchase and application.
Crop Detection
AI-powered crop detection systems utilize computer vision and machine learning to identify and classify different crop types within a field, even differentiating between varieties of the same crop. Crop identification is particularly useful for large-scale farming operations and precision agriculture, enabling targeted management practices based on specific crop needs, optimizing resource allocation, and improving overall farm efficiency. This precise approach allows farmers to tailor their management strategies to the specific requirements of each crop type.
Invasive Plant Control
AI-powered tools assist in invasive plant control by identifying and mapping infestations using aerial imagery and machine learning algorithms, enabling targeted interventions such as herbicide application or mechanical removal. This technology helps prevent the spread of invasive plants, protecting native ecosystems and agricultural lands, preserving biodiversity, and promoting sustainable land management practices. Early detection and targeted interventions minimize the long-term impact of invasive species.
Soil Texture and Moisture Modeling
Understanding soil properties is crucial for optimizing irrigation and nutrient management. AI algorithms analyze soil data from various sources, including sensors, soil samples, and historical records, to create detailed models of soil texture and moisture content, providing valuable insights for precision irrigation, targeted fertilizer application, and optimized resource utilization. These models help farmers tailor their irrigation and fertilization strategies to the specific needs of different areas within their fields.
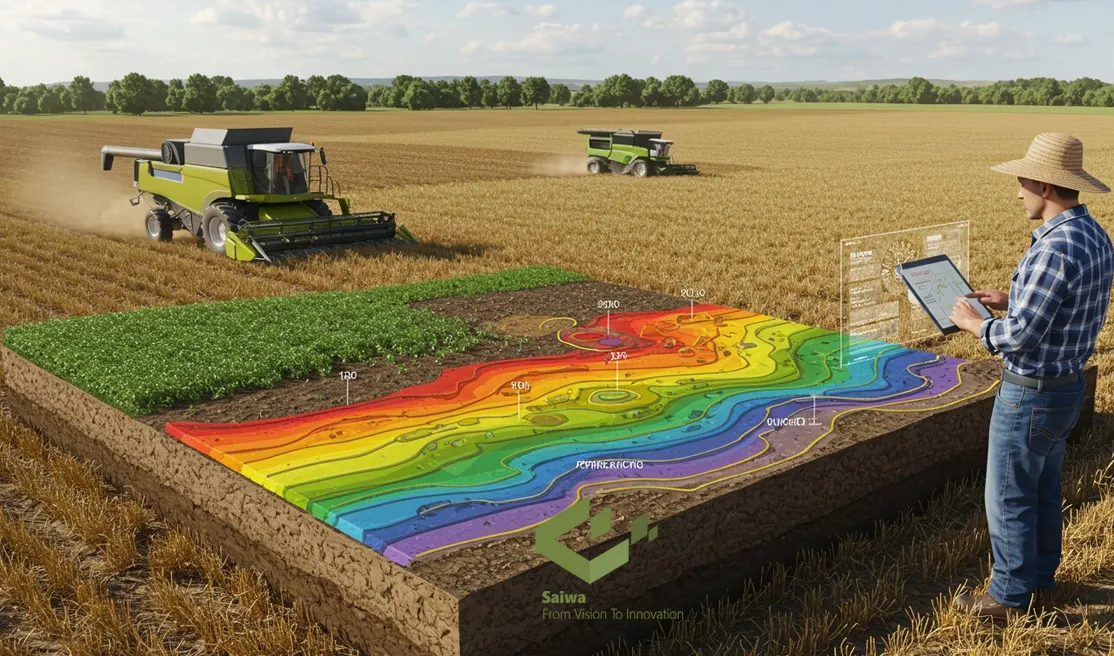
Weather and Soil Analysis
Weather patterns and soil conditions play a significant role in crop growth and yield. AI-powered systems integrate weather data from weather stations and forecasts with soil information from sensors and soil maps to provide farmers with insights into optimal planting times, irrigation scheduling, and fertilizer application, maximizing crop productivity, minimizing environmental impact, and improving resource use efficiency. This integrated approach allows farmers to adapt their management practices to changing environmental conditions.
Final Thoughts
AI is reshaping the way we approach agriculture, giving farmers the tools to solve today's toughest challenges. It's making it possible to use resources more efficiently, increase crop yields, and reduce environmental impact while empowering farmers to make smarter decisions.
As the technology evolves, AI's role in agriculture will only grow, opening up new opportunities for more sustainable and productive farming practices. This marks a shift toward a new era of agriculture—one that is more connected, data-driven, and resilient in the face of global change. With AI, farmers have the power to overcome these challenges and secure a future where food production can keep pace with demand, all while protecting the planet.