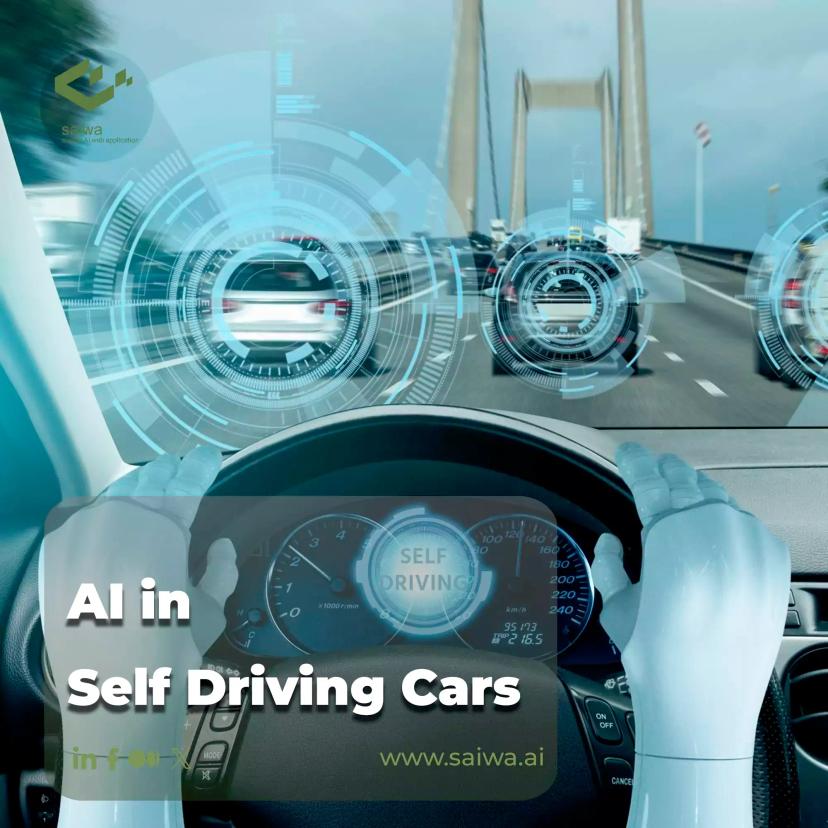
Find the Impact of AI in Self Driving Cars with Our Guide
Self-driving cars, driven by the integration of AI in self driving cars, are poised to revolutionize transportation and mobility. No longer science fiction, autonomous vehicles are becoming a technical reality as advanced AI algorithms in self-driving cars enable vehicles to navigate, operate, and make intelligent decisions without human supervision. This blog post provides an in-depth look at how artificial intelligence is enabling vehicles to drive themselves.
What is a Self Driving Car?
Self-driving, driverless, or autonomous cars are capable of performing all major driving functions, including steering, acceleration, braking, route planning, and more, without active physical control or monitoring by a human driver. They rely on a suite of hardware sensors combined with AI software to understand the environment and perform the subtasks of driving on their own in real-time.
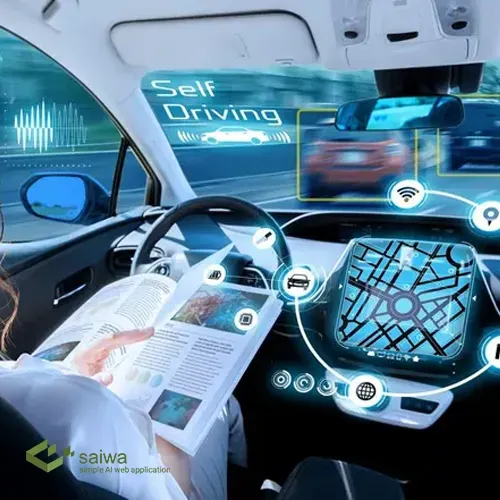
How Self Driving Car Work
Self-driving cars integrate a wide array of sensors, computing modules, connectivity equipment and AI software to enable autonomous operation:
Cameras: High-resolution cameras capture images and video to provide visual data. Some systems use up to 10 cameras for 360-degree coverage.
Radars: Radar sensors bounce radio waves to detect objects at long ranges and measure distances, similar to how autonomous cruise control works.
LiDARS: LiDAR (Light Detection and Ranging) systems shoot millions of laser beams per second to generate precise 3D mapping of the car's surroundings.
Ultrasonics: Short-range ultrasonic sensors measure proximity to obstacles during parking and low-speed maneuvers.
GPS: Global positioning system modules provide localization by continuously tracking the vehicle's geographic coordinates.
Maps: Pre-generated high-definition maps support navigation and real-time location data.
Control Units: Actuators control acceleration, braking, and steering through drive-by-wire.
Machine Learning Compute: Powerful GPUs and AI chips provide computing power to run advanced neural networks.
All this hardware generates massive data that feeds into the autonomous driving software stack. The AI-powered perception, prediction, planning, and control modules process sensor data to understand the environment and operate the vehicle.
Read More: PEAS in AI | The Core AI Features
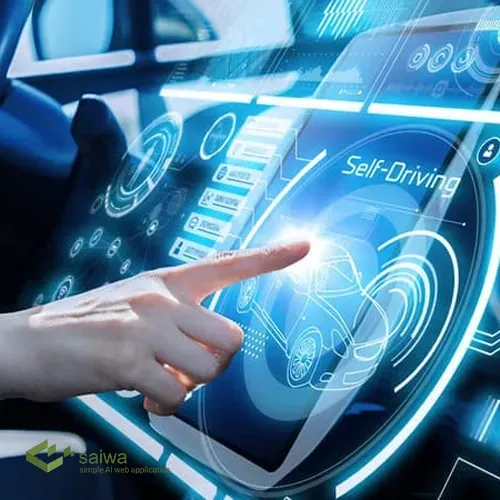
Levels of Autonomy
There are 5 levels of autonomous driving capabilities:
Level 1
Driver assistance features like automated emergency braking, adaptive cruise control or lane keeping. The driver must constantly monitor and be ready to take control.
Level 2
Steering or acceleration/deceleration assistance. The driver must still continuously pay attention to the road and surroundings.
Level 3
The vehicle can independently monitor its surroundings and make some driving decisions, but human override is still required.
Level 4
Fully self-driving within certain conditions like designated areas or good weather. The human takeover may eventually be needed.
Level 5
The highest level of autonomy with robust self-driving abilities in all conditions. No human intervention is ever required. This is the end goal of R&D, but it does not yet exist.
AI Perception Systems
The fundamental capability underpinning autonomous mobility relies on accurately perceiving contextual elements around the vehicle for intelligent adaptations. Multiple cameras, lidar sensors and radars gather continuous streams of visual data arrays tracking static, dynamic and fleeting aspects like road curvatures, traffic signaling systems, junctions, surrounding vehicles, pedestrians, stray animals and obstruction objects along the intended route.
High resolution camera feeds blended with low light infrared or depth detecting lidars offer rich scene understanding for what lies ahead to around 300 meters relative to simple cameras limited to 150 meters. Radar scans penetrate fog, rain and snow conditions where optical systems falter. Sensor fusion algorithms then stitch composite operational views labeling aspects before streaming into path planning modules. Here AI neural networks running object classification and prediction codes categorize trees, pets, potholes and more amidst endless permutations with remarkable accuracy determining influence zones for navigational decisions afterwards.
AI and Self Driving Cars
Artificial intelligence, especially machine learning and deep learning techniques are foundational to enabling cars to drive themselves. AI allows self-driving vehicles to interpret complex environments, make real-time decisions, and respond to unpredictable scenarios safely and accurately without driver intervention.
How Artificial Intelligence Algorithms Are Used in Self Driving Cars?
Some key applications of AI in self driving cars vehicles include:
Computer vision techniques like convolutional neural networks analyze visual data from cameras and video to detect vehicles, pedestrians, lane markings, signs, and other objects.
Object classification and motion prediction algorithms determine the type of objects detected, predict their likely trajectories, and assess collision risks.
Path planning AI plots the safest, most efficient driving routes to navigate from point A to B, considering traffic rules and conditions.
Reinforcement learning optimizes decision-making by modeling the vehicle's interactions with the dynamic environment around it.
Natural language processing enables voice-based interfaces for the car to understand verbal passenger commands and questions.
Prediction and Motion Planning in AI for Autonomous Vehicles
Central driving policy algorithms plan forward movement strategies factoring identified elements around the vehicle extrapolating positions few seconds ahead based on perceived trajectories. If people or cars likely cross planned route, velocities reduce for collision avoidance.
Lane changing models for AI for autonomous vehicles estimate feasibility and implications from acting on detected tailgaters. Unprotected turns analyze oncoming traffic flows postponing risky attempts. Light stage prediction prepares acceleration profiles minimizing halts. Reinforcement learning allows evaluating outcomes of past decisions for better future judgement. Here deep neural networks adeptly model human-like reasoning for anticipating movements of people and vehicles in vicinity. With sufficient modeled observation data assimilated, the AI for autonomous vehicle can soon emulate pragmatic human driving strategies adapted to diverse contexts.
Machine Learning Algorithms Used in Self Driving Cars
There are different algorithms that can use for AI in self driving cars filed by machine learning. Specific machine learning approaches crucial to self-driving cars include:
Deep convolutional neural networks for processing pixel data from visual sensors
Recurrent neural networks to model temporal contexts like trajectories
Classic computer vision techniques for tasks like edge detection
Clustering algorithms like k-means that can detect outliers
Dimensionality reduction methods like principal component analysis
Regression models for predicting vehicle paths and behaviors
Random forest models for real-time decision trees assessing conditions
Safety and Regulations for AI and Self Driving Cars
Safety is the foremost concern for autonomous vehicle deployment. Self-driving systems must handle emergency maneuvers, navigate lawfully, and avoid crashes diligently. Companies conduct rigorous real-world testing focused on corner case scenarios. Automakers collaborate with regulators to develop safety principles, certification standards, and oversight frameworks balancing innovation and responsibility. Key regulations cover vehicle design, system redundancies, driver engagement monitoring, remote human operator capabilities, and reporting of disengagements. Additional legal considerations around liability in crashes involving autonomous mode remain complex areas still evolving. Thoughtful governance balancing safety and progress continues maturing.
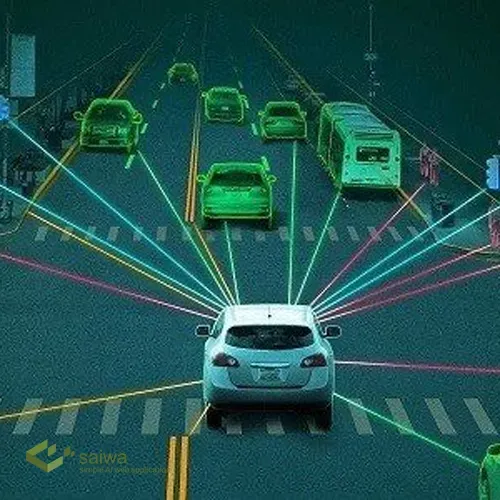
Examples of How does AI in Self Driving Cars Can Be Used
Here are some examples of how AI in self-driving cars empowers key self-driving capabilities:
Detecting traffic lights and signage using computer vision powered by deep learning
Knowing when to merge into traffic based on identifying gaps using reinforcement learning
Assessing driving conditions like slippery roads using algorithms analyzing sensor data patterns
Plotting an alternate route in heavy rain relying on natural language interaction
Avoiding collisions by anticipating pedestrian movements with neural networks
AI for Real-Time Decision Making and Planning
Additional AI techniques allow self-driving cars to make sub-second decisions and plan maneuvers:
Predictive modeling anticipates movements of pedestrians, cyclists, and other cars, assessing collision risks.
Path planning algorithms use maps and environmental data to plot optimal local driving paths in real-time.
Reinforcement learning and neural networks model scenarios to optimize driving strategies and decision making.
Motion planning coordinates acceleration, braking and steering actions.
Natural language processing enables voice-based human-car interaction.
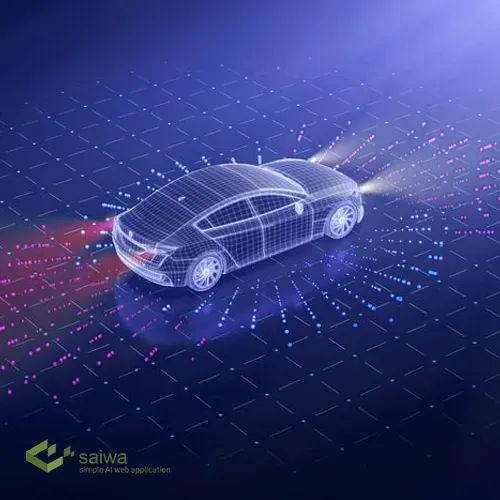
Challenges of Using AI in Self Driving Cars
While indispensable, applying AI in self driving cars to autonomous vehicles presents challenges, including:
Ensuring prediction reliability in novel driving scenarios, the AI has not encountered
Determining liability if an accident occurs due to potential AI system failures
Training models require huge labeled datasets from road testing that is costly to obtain
Identifying and correcting algorithmic biases that could lead to unsafe driving behaviors
Streaming and processing data efficiently from multiple sensors in real-time
Making deep learning models more interpretable for debugging and compliance
Ethical Considerations in AI Decision-Making
The deployment of AI and self driving cars in decision-making processes on the road raises profound ethical considerations that demand comprehensive exploration and careful navigation.
Examining Ethical Dilemmas in Critical Situations
The integration of AI in autonomous vehicles necessitates a meticulous examination of ethical quandaries arising in critical situations. When faced with scenarios that demand split-second decisions, the moral framework guiding these AI algorithms becomes paramount. Questions surrounding the prioritization of various outcomes, from potential harm to pedestrians or passengers to property damage, unveil the intricacies of ethical decision-making in the autonomous world.
Balancing Safety Imperatives with Ethical Considerations
Striking a delicate balance between safety imperatives and ethical considerations becomes a central challenge. While the primary objective is to enhance road safety, decisions made by AI algorithms can have ethical implications. Deciphering how these algorithms prioritize safety without compromising ethical principles is a complex task that requires a nuanced understanding of the societal, moral, and legal dimensions involved.
The Role of Transparency in Ethical AI
Transparency emerges as a cornerstone in the ethical considerations surrounding AI decision-making. Users, regulators, and society at large need a clear understanding of how AI algorithms operate and make decisions. Striving for transparency fosters trust and empowers stakeholders to hold AI systems accountable. Ethical AI requires a commitment to openness, allowing for scrutiny and ensuring that the decision-making process is understandable and justifiable.
Human Oversight and Accountability on AI and Self Driving Cars
Ethical AI decision-making necessitates robust mechanisms for human oversight and accountability. While AI and self driving cars systems are designed to operate autonomously, having a human-in-the-loop ensures a layer of ethical supervision. Establishing accountability frameworks, where developers and organizations are responsible for the ethical implications of their AI systems, becomes crucial in maintaining the ethical integrity of autonomous vehicles.
Industry Players and Innovations
Major automakers like GM, Ford, and Toyota have active autonomous vehicle programs and partnerships augmenting their traditional expertise with startup acquisitions and AI talent recruitment. Ride-hailing giants Uber and Lyft aim to eventually deploy autonomous robotaxi fleets. Tech companies including Waymo, Apple, and Baidu also see transportation as a promising AI application. Smaller startups focus on critical subproblems like simulation platforms, 3D semantic mapping, motion planning algorithms, and sensor hardware. Key innovations include virtual testing, redundant sensor fusion, high-precision localization, interaction protocols, and simulated operators. Billions invested annually reflects the massive opportunity ahead to transform mobility through AI if technical challenges are overcome responsibly.
Conclusion
The meteoric progress in autonomous vehicle technology would not be possible without artificial intelligence. Deep learning and machine learning models empower ai in self driving cars to perceive, understand, plan, and act in complex real-world conditions. But further breakthroughs in AI will be needed to handle corner cases, ensure safety to the highest standards, and enable widespread adoption. The automotive and tech industries still face challenges integrating AI into full Level 5 autonomy in all conditions. But the promise of AI-driven autonomous mobility is closer than ever to being realized.