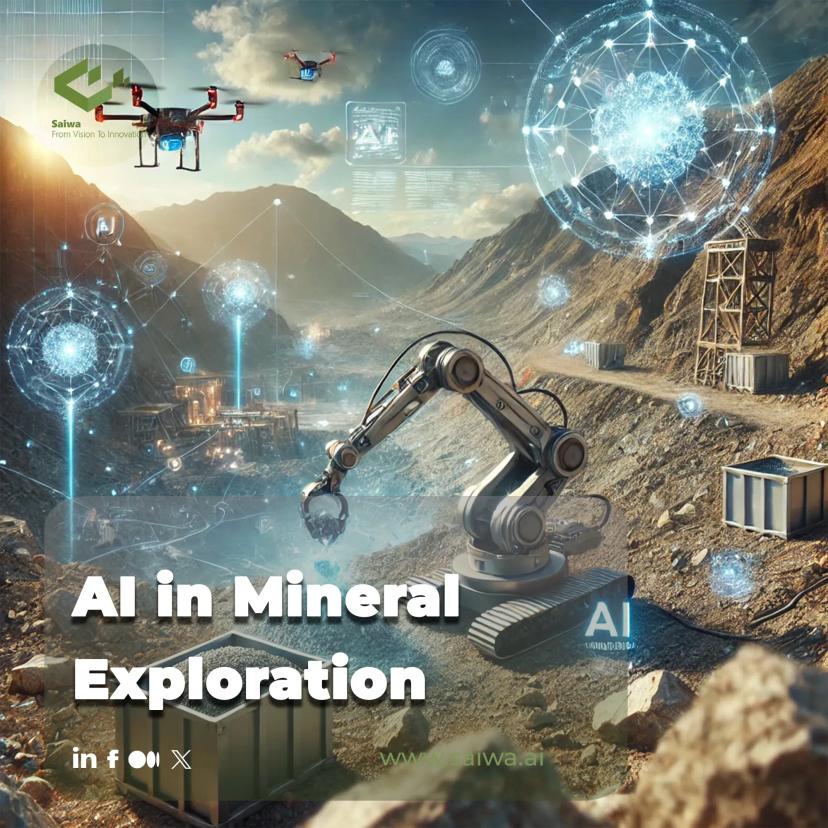
AI in Mineral Exploration | Transforming the Future of Resource Discovery
The global demand for mineral resources is experiencing an unprecedented surge, driven by population growth, urbanization, and the transition to renewable energy technologies. This escalating demand necessitates innovative approaches to mineral exploration, a field traditionally characterized by high costs, long lead times, and inherent geological uncertainties. Artificial Intelligence (AI), with its ability to analyze vast datasets, identify complex patterns, and make data-driven predictions, is emerging as a transformative force in modern mineral exploration.
Saiwa is an artificial intelligence (AI) company that provides AI and machine learning services through a service-oriented platform. In mineral exploration, Saiwa offers services such as anomaly and defect detection. These services utilize AI to analyze geological data, thereby identifying patterns and anomalies that might otherwise be missed through traditional methods. By incorporating these AI-driven techniques, Saiwa supports more accurate and efficient mineral exploration, aiding in the discovery and assessment of valuable resources.
This article provides a comprehensive overview of the applications, benefits, challenges, and future directions of AI in revolutionizing the way we discover and assess mineral resources.
Basics of AI and Machine Learning
Artificial Intelligence encompasses a broad spectrum of computational techniques that empower computers to emulate human cognitive abilities, including learning, reasoning, problem-solving, and decision-making. Machine Learning (ML), a subfield of AI, focuses on developing algorithms that enable computers to learn from data without explicit programming.
In the context of mineral exploration, ML algorithms are trained on extensive datasets comprising geological, geophysical, geochemical, and remote sensing information to identify patterns indicative of mineral deposits, predict their occurrence, and guide exploration efforts toward high-potential targets.
Key Machine Learning Paradigms for Mineral Exploration
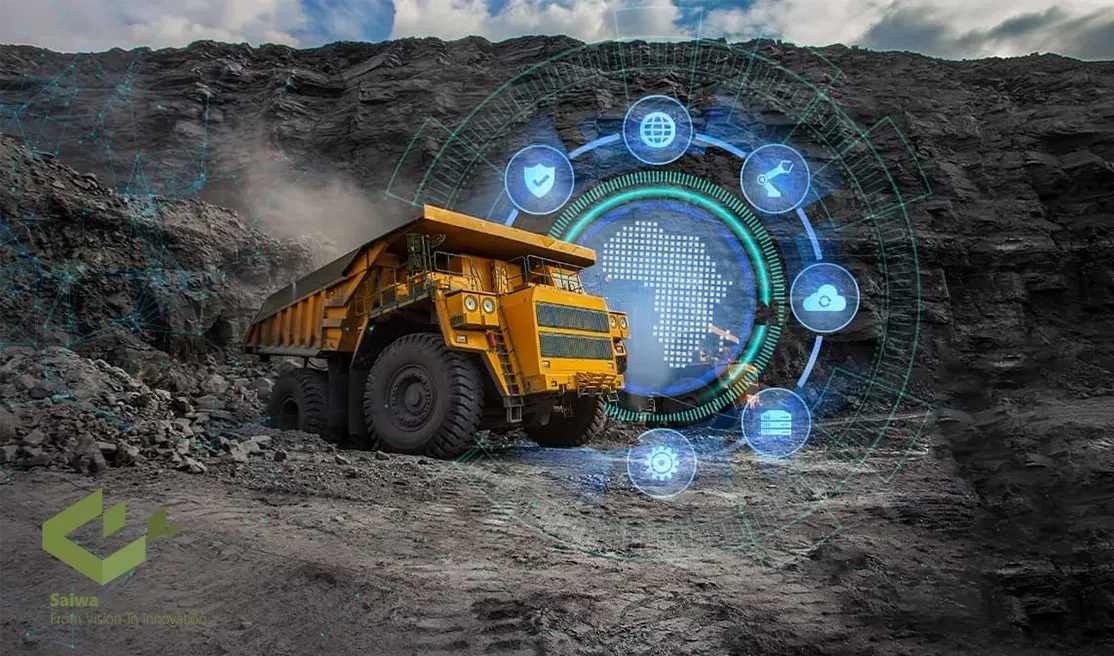
Supervised Learning
In this paradigm, algorithms learn from labeled datasets, where both the input features (e.g., geophysical measurements, geochemical assays) and the desired outputs (e.g., presence or absence of a mineral deposit, or ore grade) are provided. The algorithm learns the relationship between input and output, enabling it to make predictions on new, unseen data.
Unsupervised Learning
In contrast to supervised learning, unsupervised learning algorithms examine unlabeled data to identify intrinsic patterns, structures, and relationships without the a priori knowledge of the desired outputs. This approach is particularly advantageous for exploratory data analysis and the identification of latent patterns in complex datasets.
Deep Learning
A specialized form of ML employing artificial neural networks with multiple layers to extract increasingly complex and abstract features from data. Deep learning models have achieved remarkable success in various domains, including image recognition, natural language processing, and, increasingly, mineral exploration.
Data Sources in Mineral Exploration
The effectiveness of AI in mineral exploration is contingent upon the availability of high-quality, diverse, and comprehensive datasets. These datasets provide the basis for training basic machine learning algorithms, validating their performance, and generating accurate predictions. The following sections examine the principal data sources utilized in AI-driven mineral exploration.
Remote Sensing and Satellite Imagery
Remote sensing technologies, which encompass sensors mounted on satellites, aircraft, or drones, provide a synoptic perspective of the Earth's surface, thereby facilitating the capture of valuable information for mineral exploration at various scales.
Multispectral Imagery
The sensors record reflected electromagnetic radiation in multiple discrete wavelength bands, thereby enabling the discrimination of different surface materials based on their spectral signatures. The data is instrumental in identifying rock types, alteration minerals associated with ore deposits, and vegetation patterns indicative of underlying geology.
Hyperspectral Imagery
The collection of data in hundreds of narrow, contiguous spectral bands by means of hyperspectral sensors provides detailed spectral fingerprints of surface materials, thus enabling the precise identification and mapping of subtle alteration halos surrounding mineral deposits.
Radar Imagery
The utilization of microwave radiation enables radar systems to penetrate cloud cover and vegetation, thereby facilitating the acquisition of valuable insights into surface roughness, topography, and structural features, even in challenging weather conditions. This capability is particularly advantageous in regions with dense vegetation or high cloud cover.
Other Cases
Beyond remote sensing, a wealth of other data sources contributes significantly to AI-driven mineral exploration:
Geophysical Surveys
Geophysical methods measure variations in the Earth's physical properties to infer subsurface geological structures and rock properties. Common geophysical survey techniques include:
Magnetic Surveys: Measure variations in the Earth's magnetic field caused by differences in the magnetic susceptibility of rocks, useful for mapping igneous intrusions, faults, and iron oxide mineralization.
Electromagnetic (EM) Surveys: Transmit electromagnetic waves into the ground and measure the response, providing information about the electrical conductivity of subsurface materials, valuable for detecting conductive orebodies and mapping groundwater resources.
Gravity Surveys: Measure minute variations in the Earth's gravitational field caused by density contrasts in the subsurface, aiding in identifying dense mineral deposits, salt domes, and sedimentary basin structures.
Geochemical Data
Analysis of soil, rock, stream sediment, and water samples provides insights into the chemical composition of the Earth's surface and subsurface. Geochemical data helps identify geochemical anomalies associated with mineral deposits, delineate geochemical halos, and understand the processes involved in ore formation.
Geological Maps and Reports
Historical exploration data, geological maps, scientific publications, and technical reports provide valuable contextual information on regional geology, known mineral occurrences, structural controls on mineralization, and past exploration efforts.
Drilling Data
Drill core samples and downhole geophysical logs provide direct observations of subsurface geology, mineralization style, ore grade continuity, and structural features. This data is crucial for validating geological models, estimating resource potential, and guiding mine planning.
AI Applications in Geological Mapping
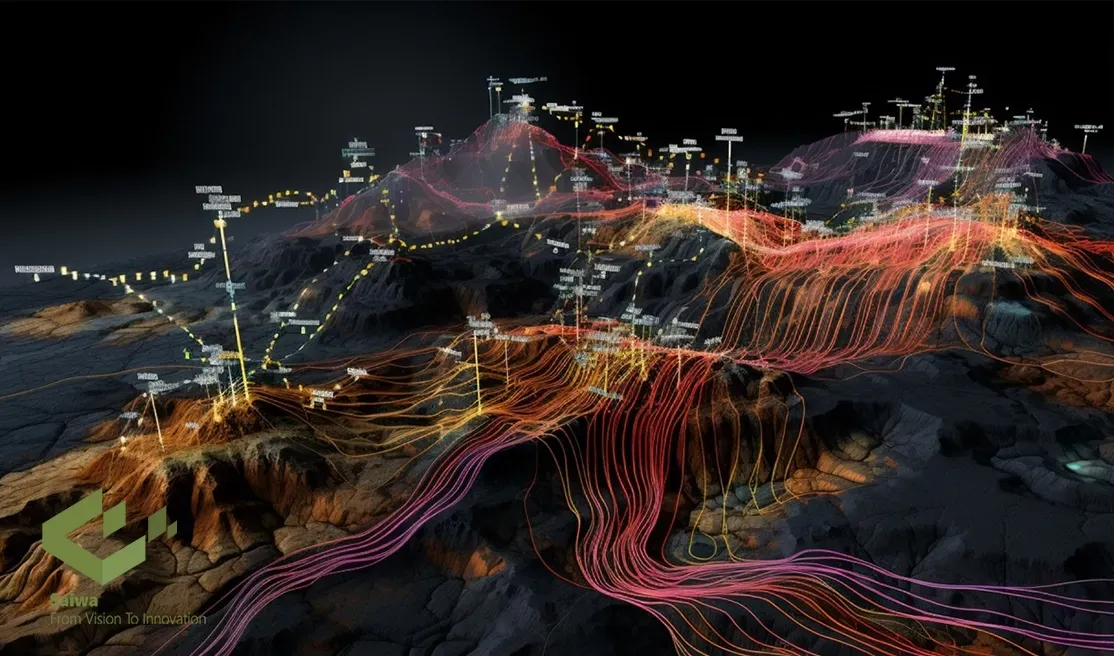
The process of identifying and delineating different rock units and structural features, otherwise known as geological mapping, forms the foundation of mineral exploration. Artificial intelligence is transforming the practice of geological mapping through the automation of tasks, enhanced accuracy, and the extraction of insights from complex datasets.
Automated Lithological Mapping
Machine learning algorithms, particularly convolutional neural networks trained on labeled geological maps and multispectral or hyperspectral imagery, can automatically classify rock units based on their spectral signatures.
This automation markedly accelerates the mapping process, reduces subjectivity, and permits geologists to concentrate on interpretation and analysis.
Structural Feature Extraction
AI algorithms excel at identifying and delineating geological structures such as faults, folds, lineaments, and shear zones from remote sensing data, digital elevation models (DEMs), and geophysical datasets. These structures often control fluid flow and mineralization, making their accurate mapping crucial for target generation.
3D Geological Modeling
The integration of multiple data sources, including geological maps, geophysical surveys, and drilling data, facilitates the generation of comprehensive three-dimensional geological models. Artificial intelligence algorithms facilitate the interpolation of data between drill holes, the refinement of geological boundaries, and the enhancement of the precision and resolution of subsurface models, thereby providing a more realistic representation of the subsurface geology.
Geophysical Data Interpretation
The interpretation of geophysical data frequently necessitates the handling of extensive and intricate datasets, accompanied by the formulation of subjective interpretations. Artificial intelligence provides sophisticated instruments that facilitate objectivity, efficiency, and accuracy in geophysical data analysis.
Automated Anomaly Detection
ML algorithms can analyze geophysical datasets to identify statistically significant anomalies that deviate from the background noise, highlighting potential areas of interest for further exploration. This automated anomaly detection reduces subjectivity, improves consistency, and allows geophysicists to focus on interpreting the most promising anomalies.
Inversion Modeling
Geophysical inversion involves converting measured geophysical data into models of subsurface physical properties (e.g., density, conductivity, magnetic susceptibility). AI techniques, particularly deep learning, are being explored to improve the accuracy, speed, and robustness of inversion processes, providing more reliable and higher-resolution images of the subsurface.
Integrated Geophysical Interpretation
AI facilitates the integration and joint interpretation of multiple geophysical datasets (e.g., magnetic, gravity, and electromagnetic data) to generate a more comprehensive and holistic understanding of subsurface geology and mineral potential. By combining different geophysical methods, each sensitive to different physical properties, AI can help unravel complex geological relationships and identify subtle anomalies that might be missed by analyzing datasets in isolation.
The potential benefits of AI-driven mineral exploration
The application of artificial intelligence (AI) is revolutionizing the field of mineral exploration, offering a range of significant advantages that enhance the efficiency, accuracy, and sustainability of the process.
1. Enhanced Data Processing and Predictive Analytics
Artificial intelligence (AI) algorithms are capable of processing vast quantities of data from many sources, including geological surveys, satellite images, and historical mining records, with greater speed and efficacy than traditional methods. Machine learning models, such as neural networks, are capable of detecting patterns and anomalies that may indicate the presence of untapped mineral resources. This enables the identification of opportunities that might otherwise have been overlooked through conventional exploration methods.
2. More Precise Targeted Exploration
The application AI to the analysis of geological and geophysical data enables a more targeted approach to exploration efforts. Artificial intelligence models assist in the identification of regions exhibiting the greatest potential for specific mineral deposits, thereby enabling companies to concentrate their efforts on promising locations. This approach reduces operational costs and minimizes environmental impact by limiting unnecessary exploration in low-potential areas.
3. Increased Efficiency and Cost Savings
The implementation of AI-driven tools has the effect of streamlining the entire exploration workflow. The automation of data analysis facilitates the expeditious identification of viable mining sites, thereby enabling businesses to make informed decisions in a timelier manner. This enhanced efficiency results in a reduction in both the time and costs associated with mineral exploration.
4. Synergistic Collaboration with Human Expertise
Artificial intelligence does not displace human expertise; rather, it serves to augment it. The contributions of geologists and engineers are invaluable in this process, as they provide insights that enhance the precision of AI-generated findings by offering a domain-specific interpretation of the data. The synergy between AI and human expertise elevates the overall quality of exploration, facilitating more precise identification of prospective deposits.
Geochemical Data Analysis
The analysis of geochemical data is of paramount importance in the field of mineral exploration. It enables the identification of geochemical anomalies associated with mineralization, the comprehension of geochemical dispersion patterns, and the navigation towards potential ore bodies. The application of AI techniques serves to enhance the processes of anomaly detection, interpretation, and predictive modeling.
Anomaly Detection in Soil and Rock Samples
Multivariate Statistical Analysis
Techniques such as Principal Component Analysis (PCA) and Factor Analysis, frequently employed in conjunction with ML algorithms, facilitate the identification of multi-element geochemical signatures associated with mineralization, even in intricate geological settings where mineralization is nuanced or obscured by other geochemical processes.
Spatial Anomaly Detection
The integration of geostatistical methods with AI enables the comprehensive consideration of both the magnitude and spatial distribution of geochemical values, thereby facilitating more effective delineation of anomalous zones. These methods account for spatial autocorrelation, which is the tendency for nearby samples to be more similar than those farther apart, and provide a statistically robust approach to anomaly delineation.
Other Cases
Predictive Geochemical Mapping
ML algorithms, trained on existing geochemical data and other relevant parameters (e.g., geology, structure, geophysics), can predict the spatial distribution of geochemical elements, guiding infill sampling programs, refining target boundaries, and optimizing exploration efforts.
Mineral System Modeling
The integration of AI into mineral system models, which simulate the geological processes involved in ore formation, has the potential to enhance predictions of mineral occurrences based on geochemical and geological parameters.
The incorporation of AI into these models enables researchers to gain a deeper comprehension of the intricate interplay of factors that regulate mineralization, thereby enhancing the precision of mineral potential assessments.
Remote Sensing and Satellite Imagery Analysis
The vast quantity and intricate nature of data produced by contemporary remote sensing technologies demands the development of efficient and automated analytical techniques. Artificial intelligence is transforming the analysis of remote sensing data in mineral exploration, facilitating the extraction of valuable insights from extensive datasets.
Automated Mineral Identification
The combination of hyperspectral data analysis with ML algorithms such as Support Vector Machines (SVMs) or Random Forests allows for the identification and mapping of specific alteration minerals associated with ore deposits, even in scenarios where the alteration is subtle or mixed. This capability markedly enhances the capacity to detect and map alteration halos encircling mineral deposits, thereby furnishing invaluable targeting data.
Lineament Mapping and Analysis
AI algorithms can automatically extract lineaments (linear features) from remote sensing data, which often represent faults or fractures that can act as conduits for mineralizing fluids. Analyzing the density, orientation, and intersection patterns of lineaments can provide insights into structural controls on mineralization and aid in target generation.
Change Detection Analysis
By comparing multi-temporal remote sensing data acquired at different times, AI algorithms can detect changes in land cover, vegetation health, or spectral signatures over time, potentially indicating mining activities, environmental impacts, or new geological exposures. This information is valuable for monitoring exploration activities, assessing environmental compliance, and identifying new areas of interest.
Drill Core Analysis and Logging
The process of drill core logging, which entails the systematic description and analysis of drill core samples, represents a fundamental aspect of mineral exploration. However, this process can be time-consuming, subjective, and susceptible to human error. The application of AI to drill core analysis offers the potential for automation, objectivity, and enhanced accuracy.
Automated Lithological Logging
ML algorithms, trained on labeled core imagery, hyperspectral data, and/or geochemical assays, can automatically identify and classify different rock types, speeding up the logging process, reducing subjectivity, and improving consistency. This automation frees up geologists to focus on more complex interpretations and analysis.
Mineral Identification and Quantification
AI-powered image analysis techniques can identify and quantify different minerals within drill core samples, providing valuable information on ore mineralogy, mineral associations, and grade distribution. This information is crucial for understanding the nature of mineralization, estimating resource potential, and guiding metallurgical testing.
Structural Feature Detection
AI algorithms can detect structural features like fractures, veins, foliations, and shear zones within core imagery, providing insights into the structural controls on mineralization, fluid flow pathways, and potential ore shoot orientations.
Target Generation and Prioritization
One of the most impactful applications of AI in mineral exploration lies in its ability to integrate multiple datasets to identify, delineate, and prioritize exploration targets:
Prospectivity Mapping
ML algorithms, trained on a combination of geological, geophysical, geochemical, and remote sensing data, can generate prospectivity maps that highlight areas with high potential for hosting specific types of mineral deposits. These maps serve as valuable decision-support tools for focusing exploration efforts on the most promising areas.
Target Ranking and Prioritization
AI can rank and prioritize exploration targets based on their predicted mineral potential, considering factors such as geological favorability, geophysical and geochemical anomalies, and proximity to known mineral occurrences. This ranking allows exploration companies to allocate resources strategically and focus on the targets with the highest likelihood of success.
3D Target Modeling
Integration of multiple datasets in 3D allows for the creation of detailed models of potential mineral deposits, incorporating geological interpretations, geophysical inversions, geochemical distributions, and structural information. These 3D models improve the accuracy of resource estimations, optimize drill hole placement, and provide a more comprehensive understanding of the target geometry and controls on mineralization.
Integration of Multiple Data Sources
The true power of AI in mineral exploration is unleashed when multiple data sources are integrated and analyzed simultaneously. This data fusion approach provides a more holistic understanding of the geological context, reduces uncertainties, and improves the accuracy of predictions:
Data Fusion Techniques
AI facilitates the fusion of data from disparate sources, such as remote sensing, geophysics, geochemistry, drilling data, and geological maps, to create a unified and comprehensive dataset for analysis. Data fusion techniques ensure that data from different sources are properly aligned, scaled, and weighted, maximizing the information extracted from the combined dataset.
Read Also: Remote Sensing in Agriculture| Unleashing the Potential
Knowledge Discovery
By uncovering hidden relationships, patterns, and correlations within complex, multi-dimensional datasets, AI can reveal previously unknown geological insights, identify subtle anomalies, and guide exploration efforts towards new discoveries. AI's ability to handle vast datasets and explore complex relationships allows it to identify patterns that might be missed by traditional data analysis methods.
Decision Support Systems
AI-powered decision support systems can assist exploration geologists in interpreting complex data, evaluating exploration targets, assessing risks, and making informed decisions throughout the exploration lifecycle. These systems provide a platform for integrating data, visualizing results, testing different scenarios, and optimizing exploration strategies.
AI in Exploration Project Management
Beyond its technical applications in data analysis and target generation, AI is also being leveraged to optimize exploration project management, improve efficiency, and reduce costs:
Resource Allocation
AI algorithms can analyze historical project data, market trends, commodity prices, and exploration costs to optimize resource allocation, ensuring that exploration budgets are used effectively and that resources are directed towards the most promising projects.
Risk Assessment and Mitigation
By analyzing historical exploration data, geological factors, environmental regulations, and other relevant parameters, AI can identify potential risks associated with exploration projects and suggest mitigation strategies. This proactive risk assessment can help companies avoid costly delays, minimize environmental impacts, and improve the overall success rate of exploration projects.
Automated Reporting and Documentation
AI can automate the generation of exploration reports, technical memos, and other documentation, reducing the administrative burden on geologists, improving consistency, and ensuring that reports are generated promptly and efficiently.
Machine Learning Models in Mineral Exploration
A diverse array of machine learning models is employed in mineral exploration, each with its strengths, limitations, and suitability for different types of data and tasks:
Support Vector Machines (SVMs)
SVMs are powerful algorithms for both classification and regression tasks. They are particularly well-suited for handling high-dimensional datasets, making them suitable for mineral prospectivity mapping, geochemical anomaly detection, and lithological classification from hyperspectral data.
Random Forests
Random Forests are ensemble learning methods that combine multiple decision trees to make predictions. They are known for their robustness to noise, outliers, and missing data, making them suitable for handling complex geological datasets with inherent uncertainties. Random Forests are used for tasks such as mineral prospectivity mapping, target ranking, and geochemical anomaly detection.
Artificial Neural Networks (ANNs)
ANNs are inspired by the structure and function of the human brain, consisting of interconnected nodes (neurons) organized in layers. Deep learning models, with their multiple hidden layers, are particularly adept at handling large, complex datasets and extracting high-level features. ANNs are increasingly used for image analysis, geophysical data interpretation, predictive modeling, and other tasks in mineral exploration.
Deep Learning Applications
Deep learning, with its ability to learn complex patterns and representations from vast datasets, is rapidly gaining traction in mineral exploration, offering solutions to previously intractable challenges:
Convolutional Neural Networks (CNNs)
CNNs are specifically designed for analyzing image data, making them ideal for tasks such as automated lithological mapping from remote sensing imagery, mineral identification in drill core photos, and structural feature extraction from geophysical datasets. CNNs excel at identifying spatial patterns and features in images, mimicking the human visual system.
Recurrent Neural Networks (RNNs)
RNNs are designed to handle sequential data, making them suitable for time-series analysis of geophysical data, geochemical data collected along drill holes, or other data with a temporal component. RNNs can capture temporal dependencies and trends, enabling the identification of patterns that might be missed by traditional time-series analysis methods.
Generative Adversarial Networks (GANs)
GANs consist of two neural networks—a generator and a discriminator—trained in an adversarial manner. The generator learns to create synthetic data that mimics the real data, while the discriminator learns to distinguish between real and synthetic data. GANs can be used to generate synthetic geological data, which can be used to augment training datasets, test the performance of exploration models, or explore geological scenarios.
AI-driven Exploration Case Studies
Numerous successful case studies highlight the real-world impact and transformative potential of AI in mineral exploration:
Gold Exploration in Western Australia
Predictive Discovery, a mineral exploration company, utilized a combination of machine learning and geological expertise to identify a new gold deposit at their Bankan Project in Western Australia. By analyzing a range of datasets, including geochemistry, geophysics, and drilling data, the AI algorithms identified previously unrecognized patterns and highlighted a target that led to a significant gold discovery.
Copper Exploration in Chile
Goldspot Discoveries employed AI-powered analysis of hyperspectral data to identify a new copper porphyry target at the El Salto property in Chile. The AI algorithms were able to identify subtle alteration mineral assemblages associated with copper mineralization, even in areas with limited outcrop exposure. This discovery highlighted the potential of AI to unlock mineral potential in challenging exploration environments.
Australia and Chile are just two examples of countries leveraging AI in mineral exploration, but the use of AI extends far beyond these nations. Many other countries are adopting AI technologies to enhance their mineral engineering efforts, revolutionizing the discovery and assessment of mineral resources globally.
Challenges and Limitations of AI in Mineral Exploration
Despite its transformative potential, the application of AI in mineral exploration faces several challenges and limitations:
Data Quality and Availability
The success of AI algorithms hinges on the availability of high-quality, comprehensive, and well-labeled datasets for training and validation. In many cases, exploration datasets are incomplete, noisy, or inconsistently collected, posing challenges for AI model development and deployment.
Algorithmic Bias and Interpretability
AI algorithms can inherit biases from the data they are trained on, potentially leading to inaccurate or misleading predictions. Additionally, the "black box" nature of some deep learning models makes it difficult to understand the reasoning behind their predictions, hindering trust and acceptance among geologists.
Integration with Existing Workflows
Integrating AI into existing exploration workflows requires changes in organizational culture, skillsets, and data management practices. Companies need to invest in training, software, and infrastructure to support the adoption and effective utilization of AI technologies.
Responsible AI: Ethical and Environmental Considerations in Mineral Exploration
The rapid adoption of artificial intelligence in mineral exploration presents incredible opportunities, but also necessitates a strong focus on responsible practices. Ethical and environmental considerations are paramount to ensuring that this powerful technology benefits both the industry and the planet.
Minimizing Environmental Impact: Traditional exploration can be disruptive, involving extensive drilling and land disturbance. Artificial intelligence in mineral exploration offers a path to significantly reduce this footprint. By enabling more precise targeting, AI can minimize unnecessary drilling, reducing habitat disruption, water usage, and carbon emissions. Predictive models can also optimize land use planning, identifying areas with the highest potential while avoiding sensitive ecosystems.
Social License to Operate: Building trust with local communities is crucial. AI can assist in monitoring environmental impacts (e.g., water quality, air quality) and providing transparent data to stakeholders. This transparency fosters a stronger social license to operate, promoting collaborative relationships and mitigating potential conflicts. Furthermore, artificial intelligence in mineral exploration projects must respect indigenous rights and cultural heritage.
Data Privacy and Security: Exploration datasets often contain sensitive information about land ownership, resource potential, and environmental conditions. Robust data governance frameworks are essential to protect this data from unauthorized access and misuse. Implementing strong cybersecurity measures and adhering to data privacy regulations are crucial components of responsible AI.
Bias Mitigation: Artificial intelligence in mineral exploration algorithms are trained on data, and if that data reflects existing biases (e.g., historical focus on certain regions or deposit types), the AI may perpetuate those biases. Active efforts are needed to identify and mitigate biases in training data and algorithms to ensure equitable outcomes.
Transparency and Explainability: The 'black box' nature of the AI model can be solved. Explainable AI (XAI) methods are being developed to provide insight and understanding of the AI model outputs.
Conclusion
Artificial intelligence is revolutionizing the field of mineral exploration, offering unprecedented capabilities for analyzing vast datasets, identifying complex patterns, and making data-driven predictions. From automating geological mapping to optimizing target generation and enhancing resource estimation, AI is transforming the way we discover and assess mineral resources. While challenges remain in terms of data quality, algorithmic bias, and integration with existing workflows, the benefits of AI in terms of efficiency, accuracy, cost reduction, and environmental sustainability are undeniable.
As AI technologies continue to advance and the mining industry embraces digital transformation, we can expect even more innovative applications and groundbreaking discoveries in the years to come. The future of mineral exploration is undoubtedly intertwined with the power of artificial intelligence.