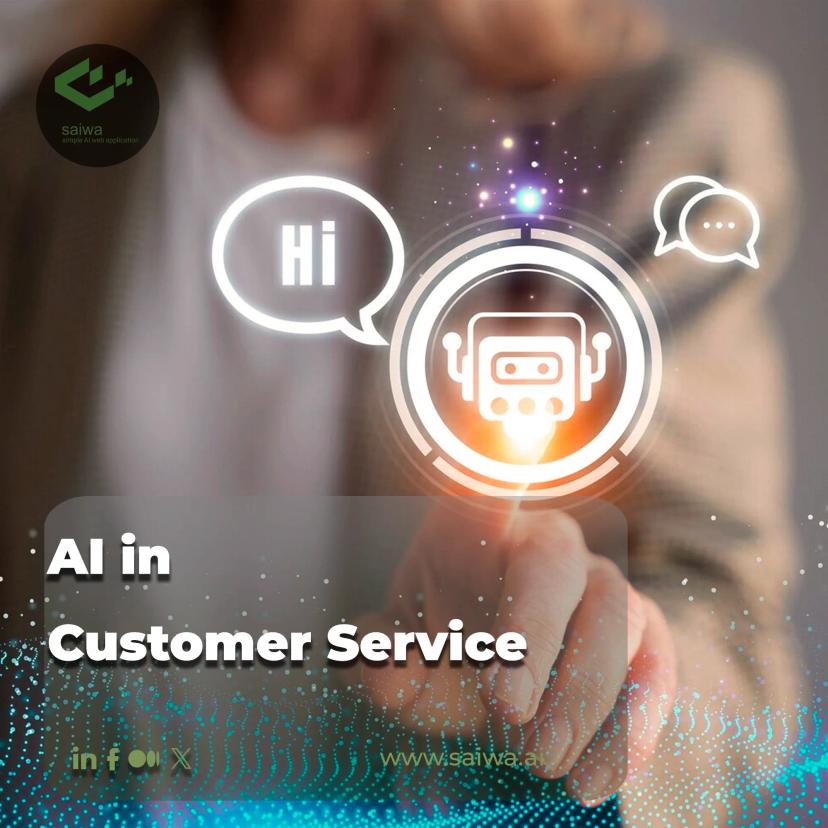
Exploring the Influence of AI in Customer Service
Artificial intelligence (AI) is transforming the customer service industry by offering scalable and personalized support across various communication channels. As customers continue to gravitate towards digital self-service and demand round-the-clock availability, AI solutions, such as chatbots, predictive behavior models, sentiment analysis techniques, and process automation systems, are helping companies manage the surging volumes of customer interactions while simultaneously improving satisfaction.
This blog post highlights key aspects of AI in customer service and how it is being transformed through machine learning and other techniques. The post explores various applications, impacts, latest innovations, implementation challenges, and future outlook of AI integration into service organizations.
Driving Factors for Adopting AI in Customer Service
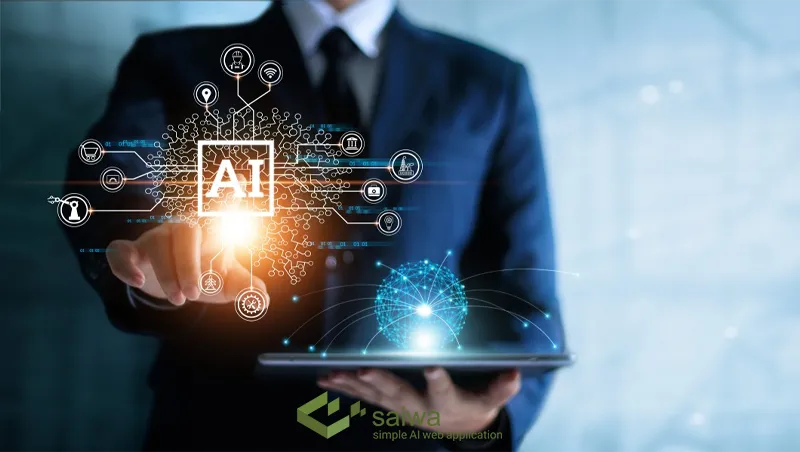
AI plays a crucial role in current customer experience strategies because of various consumer and technology trends. As the number of product types and purchase channels increase, support volumes rise. Mobile apps, social media, and other digital media platforms also generate more contact points. Customers today expect swift and convenient service round the clock across all these channels. Meeting these expectations with human agents alone is a daunting task. Intelligent self-service systems that use machine learning pipelines enable companies to optimize performance while reducing costs.
AI in customer service allows for personalized experiences that would otherwise be unattainable at scale. Personalized interactions that build loyalty are informed by analyzing customer lifetime value, past interactions, and preferences. Emotive human-like exchanges are also made possible by advances in speech processing, dialogue systems, and robotics. Automated backend processes reduce resolution times and increase first-call resolutions through optimized routing and intelligent diagnostics. The AI-based service amplifies human representatives for cost-effective, memorable interactions with customers.
Prevalent Categories of AI Applications
Modern AI software can address multiple facets of customer service via diverse techniques:
Chatbots and Virtual Assistants
Using natural language processing, machines can handle common inquiries on websites, apps, and messaging platforms via text or voice. Bots access knowledge bases to resolve repeats. Integration of speech synthesis enables conversational interactions. Read Also: The Evolution of AI Assistant ChatbotPractical Application & Future of AI in Agriculture
Predictive Analytics
By applying time-series forecasting algorithms to customer data, companies predict future support volumes, fraudulent behaviors, churn risk, and purchase needs. This allows preemptive mitigation and personalization.
Sentiment Analysis
Voice calls and open-ended survey responses are algorithmically assessed to determine customer satisfaction, emotional state, and problems. This provides feedback for improvement.
Process Automation
AI in customer service, streamlines support workflows by automatically assigning issues to specialized reps. Machine learning recommender systems also suggest knowledge-based articles for fast resolution.
Adoption Levels and Maturity Curve
Industry surveys indicate that the mainstream adoption of AI in customer service is high, with most companies deploying some applications in both front and back-end operations. Another large portion plans to acquire or develop AI capabilities for their service environments in the coming years. However, the sophistication of AI solutions varies. The most prevalent are conversational chatbots on websites or apps, which handle low-complexity customer queries.
Rapid Growth of Sentiment Analysis Tool
Sentiment analysis tools are rapidly expanding, providing customer feedback based on text and voice data. Advanced diagnostics, predictive systems, and end-to-end process automation are still developing. Adoption rates vary depending on company size, with larger firms building in-house expertise to train enterprise-grade models on internal data at scale, while smaller businesses integrate pre-built AI solutions through vendors.
Progression Along the AI Learning Curve
Many companies are still working their way up the AI learning curve to deploy optimal customer service solutions. Beginning with chatbots that can handle simple conversations, their abilities improve significantly with simulated conversations and feedback loops aligned with machine learning dynamics. Expanding training datasets by applying natural language processing on large volumes of call transcripts, survey responses, and multi-channel conversations also enhances the efficacy of AI over time. Mapping the entire journey, including predicting and alleviating pain points, leads to genuine service transformation.
The overall maturity level is highly correlated with analytics leadership, the executive vision for data-driven decisions, and digital cultures that take risks within companies.
Transforming Service Operations and Metrics
The infusion of AI technologies has demonstrated a strong positive impact across key customer service metrics:
- Significant increase in first contact resolution via fast machine diagnostics. By analyzing the customer issue and historical resolutions, AI in customer service models accurately diagnose problems allowing agents to solve a large proportion of inquiries instantly.
- Large reduction in call volumes as chatbots efficiently handle high volumes of routine inquiries. Automated conversational agents respond to FAQs, account questions, address updates, etc. round the clock thus alleviating human reps.
- Noticeable uptick observed in customer satisfaction driven by 24/7 intelligent self-service systems. The anytime accessibility and fast response times improve perceptions of support quality.
- Major decreases were noted in overall response times via AI-based ticket triaging and routing. Models instantly identify priority issues and allocate them to specialized reps reducing backlogs.
- Substantial decline in customer service operational costs by automating repetitive tasks. Bots reduce human efforts needed for addressing repeats and documenting conversations by smart workflows.
Overall, the AI infusion allows balancing operational efficiencies alongside customer-centricity - once seen as mutually exclusive. Predictive analytics further allows tracing ROI across engagement channels and journey touchpoints to strategically allocate resources.
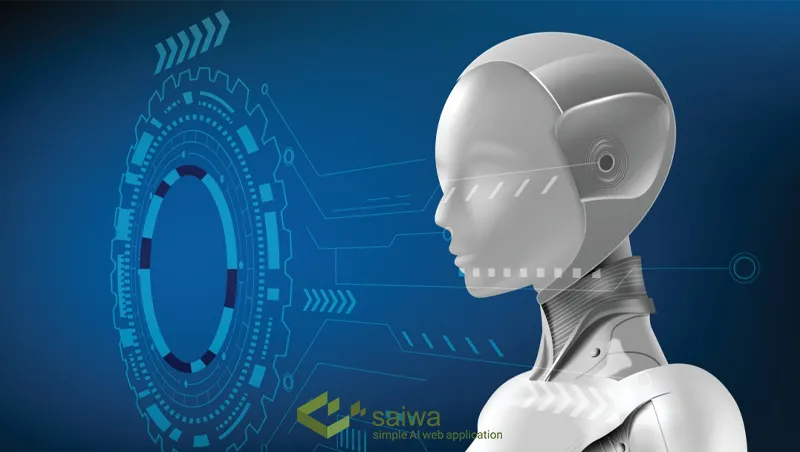
Emerging Innovations and Predictions
Several bleeding-edge innovations will expand the impact of AI in customer service in the coming decade:
Voice biometrics for seamless authentication across channels
Emotion detection via computer vision to gauge reactions
Predictive models leveraging IoT device data signals
Proactive contextual recommendations for issue avoidance
Hyper-personalization via AI avatar assistants
Augmented reality bots on mobile cameras
Autonomous customer support robots with mobility
Industry analysts predict over $1.1 billion in total annual cost savings from AI-enabled customer service by 2025 as machine learning automation replaces human efforts. Gartner expects AI to become the primary customer channel by 2030 handling more than 80% of interactions as capabilities mature. The future of support lies in preemptive, invisible, and fully customized lifecycle service centered around customer needs instead of operational constraints.
Adoption Challenges for AI in Customer Service
Realizing the full potential of AI in customer service entails overcoming adoption hurdles across technology, user readiness, and responsible design dimensions. Many companies still struggle with collecting sufficient high-quality customer interaction data across diverse channels like voice calls, chat conversations, emails, and social media posts. This hinders training robust machine learning models that can handle multifaceted inquiries, conversations, and languages. There is also the risk of algorithmic biases creeping in which leads to the exclusion of already underrepresented demographic groups from quality service opportunities.
Issues with Over-Automation
Over-automation can also negatively impact customer experience when the AI fails at context handling or complex multi-step process management.
Evolution of Human Roles in Customer Service
As AI handles more routine tasks, the roles of human customer service agents will evolve into more judgment-intensive work. This requires extensive reskilling and change management programs within organizations to enable smooth transitions.
Transparency and Privacy Concerns
There are concerns about the lack of transparency regarding AI systems' collection and analysis of personal data, and their impact on user experiences. Companies' expansion of their AI stack also leads to new cybersecurity vulnerabilities that malicious hackers can exploit to access customer databases.
To promote ethical and responsible adoption of AI, numerous customer service organizations implement governance frameworks that require technology accountability across various dimensions, such as interpretability, systematic testing procedures, reduction of unfair bias, and the establishment of inclusive oversight groups to monitor developments.
Enhancing User Experience through Collaboration
Improving user experience through human-AI collaboration was a key focus of these initiatives. It is essential to balance customer service levels with AI enablement while ensuring trust and confidence in technological practices for long-term success. Companies invest heavily in transparent AI for customer service communication and cybersecurity to achieve this equilibrium.
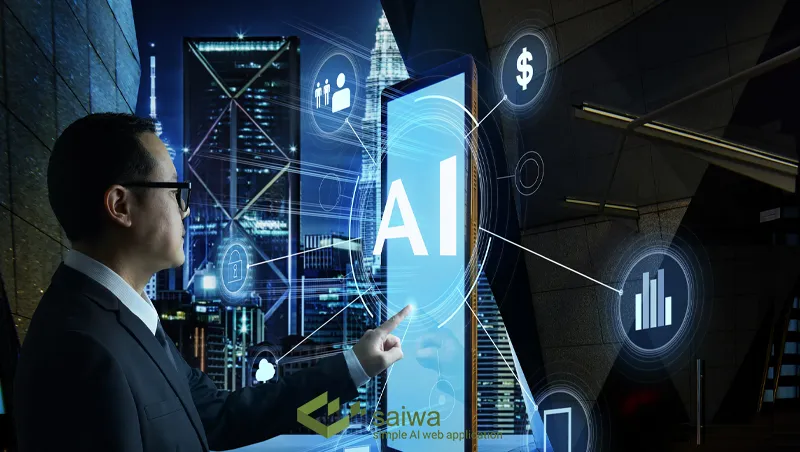
The Road Ahead
AI integration in customer service is an unstoppable trend in light of evolving technologies and market conditions that are redefining customer relationships across industries. Achieving AI mastery leads to a competitive edge. Companies must adopt data-driven decision-making, digitally savvy cultures, and innovative capabilities to meet the needs of ever more sophisticated users. Collaborating with machine learning platform vendors enables the launching of AI programs without major initial investment. Ultimately, preparing for an AI-first customer experience environment is crucial as predictive and autonomous service models will define the future landscape.
Conclusion
From chatbots to predictive analytics using machine learning, AI in customer service represents a significant transformation. It provides personalized, immediate, and predictive assistance, which is essential to retention alongside human representatives. Additionally, AI unlocks self-service models that optimize cost structures within complex customer journeys. The framework of speech analysis, process enhancement, and long-term personalization tools can manage exponential data growth and serve as a versatile system.
AI adoption is gaining momentum as initial implementations demonstrate significant operational and experiential benefits. It is essential to prioritize responsible AI development and user trust during this transformation. AI-powered customer service marks a new era of intelligent customer lifetime value management enabled by predictive insights.