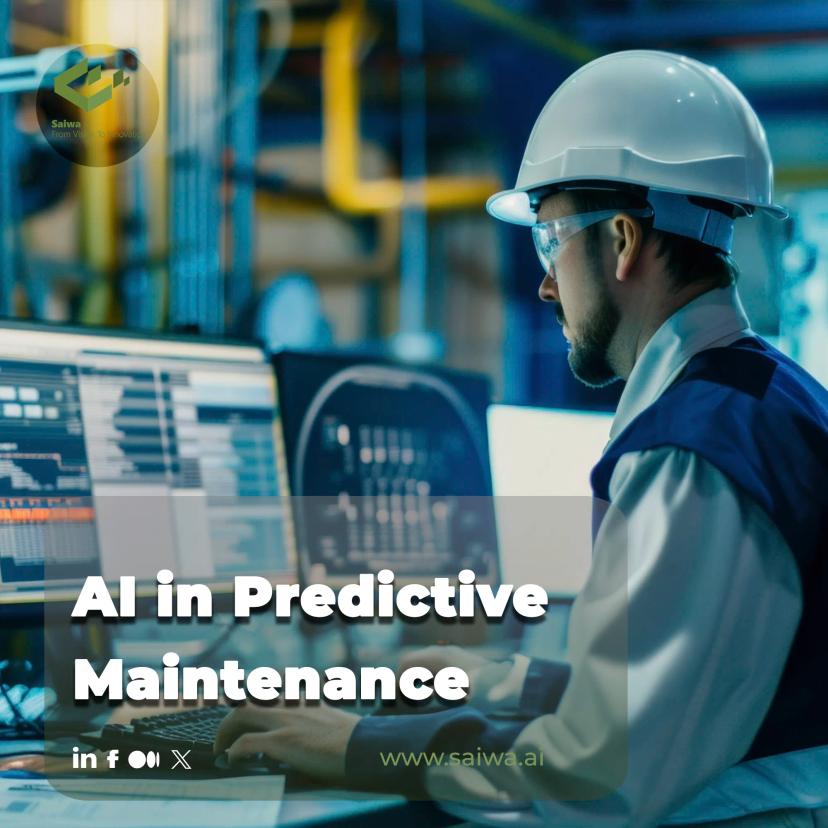
AI in Predictive Maintenance
In today's interconnected and technologically advanced world, maximizing operational efficiency and minimizing downtime are of paramount importance to companies in a wide range of industries. Traditional maintenance approaches, often reactive or based on fixed schedules, are proving inadequate in dealing with the complexity of today's equipment and processes.
A paradigm shift is underway, driven by the convergence of artificial intelligence (AI) and the Industrial Internet of Things (IIoT), resulting in a transformative approach known as predictive maintenance. This innovative strategy harnesses the power of AI to analyze the vast amounts of data generated by connected equipment, enabling accurate prediction of potential failures and optimization of maintenance schedules.
Saiwa is an artificial intelligence (AI) company that provides AI as a Service (AIaaS) and machine learning solutions through its service-oriented platform. Leveraging its expertise in AI and machine learning, Saiwa has the potential to transform industries by optimizing operations, minimizing downtime, and enhancing productivity. As industries increasingly adopt AI technologies, Saiwa’s solutions can play a pivotal role in advancing the capabilities of predictive maintenance systems, ultimately contributing to greater operational efficiency across sectors.
This article examines the intricacies of AI-based predictive maintenance, exploring its underlying principles, benefits, applications, and the promising future it holds for improving industrial operations. We will look at how AI can predict equipment failures, optimize maintenance schedules, and improve overall business continuity, demonstrating its potential to drive greater efficiency, cost savings, and safety improvements.
What is Predictive Maintenance?
Predictive maintenance represents a proactive maintenance strategy that employs data analysis to anticipate the likelihood of equipment failure. This enables maintenance to be conducted solely when required, thereby reducing both downtime and maintenance costs.
In contrast to reactive maintenance, which addresses failures after they occur, or preventive maintenance, which follows a fixed schedule regardless of equipment condition, predictive maintenance optimizes maintenance schedules based on the actual condition of the equipment. This approach minimizes disruptions, extends asset lifespan, and optimizes resource allocation.
Challenges in Traditional Predictive Maintenance Methods
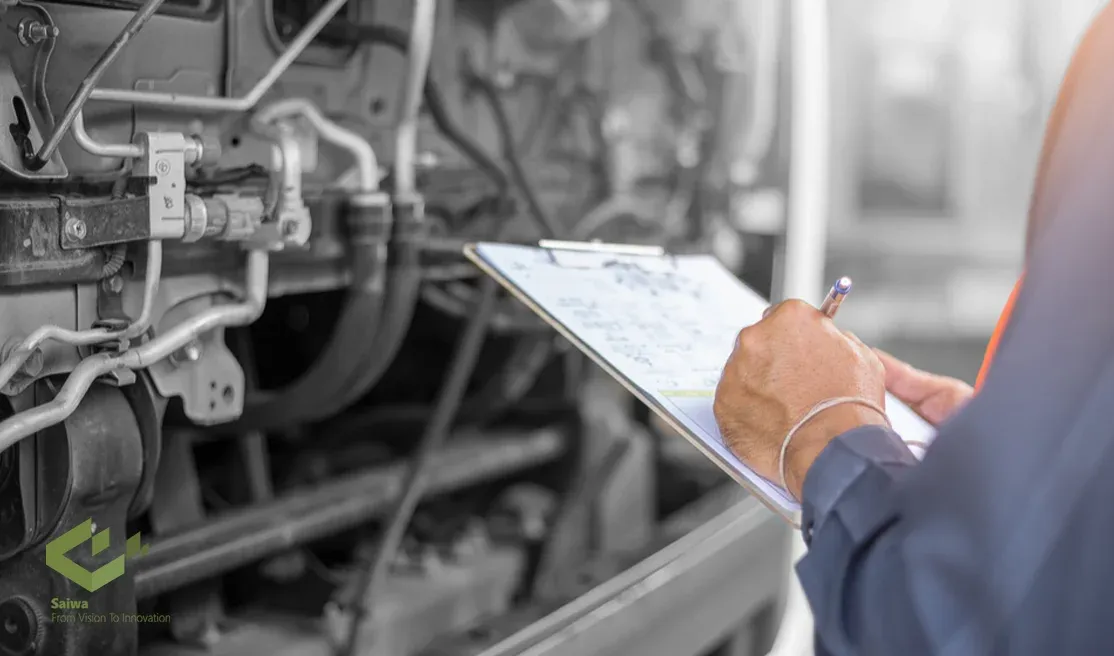
Traditional predictive maintenance methods, often relying on scheduled inspections and statistical models, face several limitations:
Inefficient Data Collection
Manual data collection methods are time-consuming, labor-intensive, and prone to human error. Technicians physically inspect equipment and record measurements, a process that is slow, costly, and prone to inaccuracies due to human fatigue or oversight. In addition, manual data collection often occurs at infrequent intervals, limiting the ability to detect subtle changes in equipment conditions that may precede a failure. This infrequent data collection can lead to delayed detection of potential problems, increasing the risk of unexpected downtime.
Limited Insights
Traditional statistical models may not effectively capture the complex relationships within large data sets, resulting in inaccurate predictions.
These models often rely on linear relationships and simplified assumptions about equipment behavior, which may not accurately reflect the complex interactions and dependencies within modern industrial systems. In addition, traditional statistical methods can struggle to handle the high dimensionality and variability of data generated by complex equipment, limiting their predictive accuracy. The inability to incorporate real-time data further limits the effectiveness of these models.
Reactive Approach
Scheduled maintenance may not align with the actual condition of the equipment, leading to unnecessary maintenance or overlooking potential failures. Preventive maintenance schedules are typically based on average failure rates or manufacturer recommendations, which may not reflect the specific operating conditions or usage patterns of individual pieces of equipment.
This can result in performing maintenance too frequently, wasting resources, and potentially introducing new problems, or too infrequently, increasing the risk of unexpected failures. The lack of real-time condition monitoring further exacerbates this issue.
Difficulty in Handling Complex Systems
Traditional methods struggle to analyze data from complex systems with numerous interconnected components. Modern industrial systems often involve intricate networks of interconnected equipment, making it challenging to isolate the root cause of problems or predict the cascading effects of failures.
Traditional methods often lack the ability to model these complex interdependencies and predict the behavior of the system as a whole. This limitation can lead to inaccurate predictions and ineffective maintenance strategies, particularly in complex manufacturing environments or critical infrastructure systems.
Use Cases of AI in Predictive Maintenance
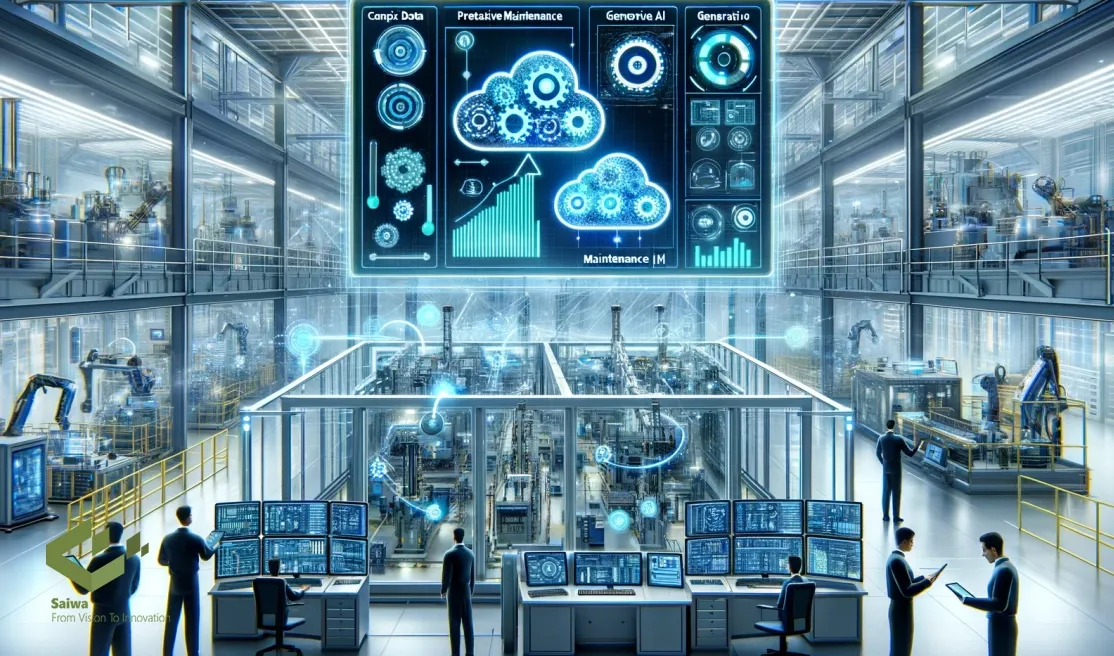
AI enhances predictive maintenance by analyzing complex data from various sources, identifying patterns indicative of impending failures, and providing actionable insights. Some key use cases include:
Predicting Equipment Failure
AI algorithms assess sensor data, previous maintenance logs, and operational parameters to predict the likelihood and timing of equipment failures. By considering a wide range of data sources, including vibration patterns, temperature fluctuations, and operating hours, AI models can identify subtle indicators of impending failures that may not be apparent through traditional methods.
These predictions enable proactive maintenance interventions, preventing catastrophic failures and minimizing downtime. Furthermore, AI can assess the confidence level of its predictions, allowing maintenance teams to prioritize interventions based on the risk level.
Optimizing Maintenance Schedules
AI-driven insights enable the creation of dynamic maintenance schedules that align with the actual condition of the equipment, minimizing downtime and optimizing resource allocation. Instead of relying on fixed maintenance intervals, AI algorithms can determine the optimal time to perform maintenance based on the predicted remaining useful life of equipment and the operational context.
This dynamic approach reduces unnecessary maintenance, saves costs, and extends the lifespan of equipment. Furthermore, AI can optimize maintenance schedules across entire fleets of equipment, considering resource availability and operational priorities.
Detecting Anomalies
AI algorithms can detect subtle anomalies in sensor data that may indicate early signs of equipment degradation, enabling proactive interventions. By continuously monitoring sensor data and comparing it to historical baselines and expected behavior, AI can detect deviations that may signal developing problems.
These early warnings allow maintenance teams to address issues before they escalate into major failures, minimizing downtime and reducing repair costs. Furthermore, AI can identify the root cause of anomalies, enabling more targeted and effective maintenance interventions.
Estimating Remaining Useful Life (RUL)
AI models can predict the remaining useful life of equipment, allowing for informed decisions about replacement or refurbishment. By analyzing historical data, operational parameters, and degradation patterns, AI algorithms can estimate how much longer a piece of equipment is likely to function effectively.
This information enables proactive planning for replacements or refurbishments, optimizing capital expenditures, and minimizing disruptions to operations. Furthermore, RUL predictions can be used to optimize maintenance strategies, ensuring that resources are focused on extending the lifespan of critical assets.
What are the Benefits of Using AI in Predictive Maintenance?
Integrating AI into predictive maintenance strategies yields numerous advantages:
Eliminate Production Losses
Unplanned downtime can significantly impact production, leading to lost revenue, missed deadlines, and customer dissatisfaction. Production losses can stem from various factors, including equipment failures, material shortages, and logistical bottlenecks. AI-powered predictive maintenance minimizes downtime by anticipating failures and enabling proactive interventions.
By analyzing real-time data from sensors and other sources, AI algorithms can identify patterns that indicate impending failures, allowing maintenance teams to address issues before they disrupt operations.
This proactive approach ensures smooth operations, maximizes production output, and improves overall business continuity, protecting revenue streams and maintaining customer satisfaction. In addition, by reducing the frequency and duration of unplanned downtime, AI-based predictive maintenance can improve overall equipment effectiveness (OEE) and contribute to a more efficient and profitable operation.
Increase Worker Productivity
AI automates data analysis and decision-making processes, freeing up maintenance personnel to focus on higher-value tasks. Traditional maintenance practices often involve manual data collection, analysis, and decision-making, which can be time-consuming and inefficient.
AI algorithms can automate these processes, analyze vast amounts of data, and provide actionable insights to maintenance teams. By streamlining maintenance workflows and providing readily available information, AI empowers maintenance teams to work more efficiently and effectively.
This increased productivity translates into cost savings by reducing labor hours spent on routine tasks, and improved operational efficiency by enabling faster response times to maintenance needs. Maintenance personnel can then apply their expertise to more complex tasks such as troubleshooting, root cause analysis and continuous improvement initiatives.
Improve Worker Safety
Predictive maintenance powered by AI can identify potential safety hazards before they escalate into accidents. Equipment failures can create hazardous conditions for workers, such as exposure to dangerous chemicals, electrical hazards, or mechanical malfunctions.
By predicting equipment failures and enabling proactive maintenance, AI helps create a safer work environment for maintenance personnel and other employees. AI algorithms can identify patterns in data that indicate potential safety risks, enabling timely intervention to mitigate those risks.
This proactive approach to safety reduces the risk of accidents and injuries, protecting workers and minimizing operational disruptions. In addition, by reducing the need for emergency repairs, AI-enabled predictive maintenance minimizes the exposure of maintenance personnel to potentially dangerous situations.
AI Technologies Used in Predictive Maintenance
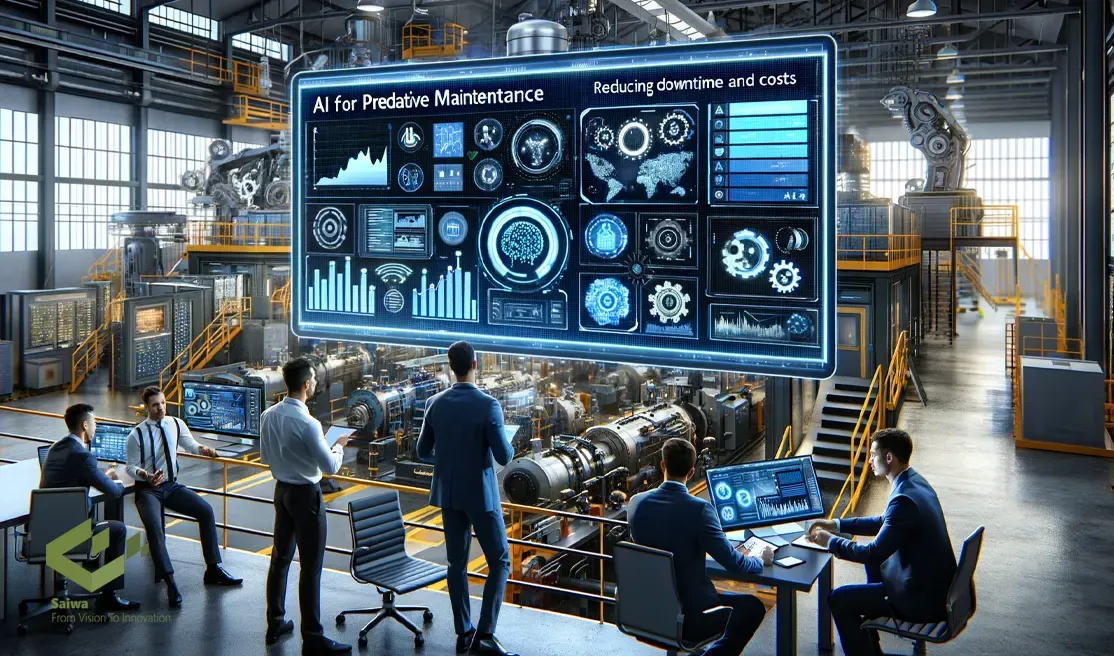
Several AI technologies contribute to the effectiveness of predictive maintenance solutions:
Machine Learning (ML)
ML algorithms analyze historical data to identify patterns and predict future outcomes. In predictive maintenance, ML models can be trained to predict equipment failures based on sensor data, operating parameters, and maintenance records.
These machine learning models learn from past data to identify correlations between various factors and equipment failures, enabling them to predict the likelihood and timing of future failures. Various ML techniques, such as supervised learning (using labeled data), unsupervised learning (identifying patterns in unlabeled data), and reinforcement learning (learning through trial and error) are employed depending on the specific application and data characteristics.
For instance, supervised learning might be used to predict failures based on historical sensor readings, while unsupervised learning could be used to cluster similar equipment based on their operational profiles.
Deep Learning
Deep learning, a subset of ML, utilizes artificial neural networks with multiple layers to analyze complex data and extract intricate patterns. These deep neural networks are inspired by the structure and function of the human brain, allowing them to learn complex hierarchical representations of data.
Deep learning models are particularly effective at handling large data sets and identifying subtle anomalies that may indicate early signs of equipment degradation. They excel at automatically extracting features from raw data, eliminating the need for manual feature engineering.
In predictive maintenance, deep learning can be used to analyze time-series sensor data, images, and other complex data types to identify patterns indicative of impending failures.
Natural Language Processing (NLP)
NLP enables computers to understand and process human language. This involves tasks such as text classification, sentiment analysis, and named entity recognition. In predictive maintenance, NLP can be used to analyze maintenance logs, operator notes, and other textual data to extract valuable insights about equipment performance and potential problems.
By automatically processing unstructured text data, NLP can uncover hidden patterns and correlations that might be missed by manual analysis. For example, NLP can identify recurring issues reported by operators or extract keywords related to specific equipment problems. This information can be integrated with other data sources to improve the accuracy of predictive maintenance models.
Computer Vision
Computer vision enables computers to "see" and interpret images. It includes tasks such as object detection, image classification, and image segmentation. In predictive maintenance, computer vision can be used to analyze images and video of equipment to detect defects, assess wear, and identify potential safety hazards.
By automating visual inspections, computer vision can reduce the need for manual inspections, saving time and improving efficiency. For example, computer vision models can be used to detect cracks in pipelines, corrosion on equipment surfaces, or misalignments in machinery. This information can be used to trigger proactive maintenance interventions and prevent costly failures.
Key Components of AI-Based Predictive Maintenance
A successful AI-based predictive maintenance system comprises several key components:
Sensors
Sensors collect data about the condition and performance of equipment, providing the raw input for AI algorithms. These sensors act as the eyes and ears of the system, continuously monitoring various parameters such as vibration, temperature, pressure, current, and acoustic emissions.
The choice of sensors depends on the specific equipment being monitored and the types of failures that need to be predicted. For example, vibration sensors are commonly used to detect imbalances in rotating machinery, while temperature sensors can identify overheating components. The data collected by these sensors is then transmitted to a central system for processing and analysis.
Data Preprocessing
Data preprocessing involves cleaning, transforming, and preparing the sensor data for analysis by AI algorithms. Raw sensor data is often noisy, incomplete, and inconsistent, requiring careful preprocessing to ensure the accuracy and reliability of predictive models.
This may include handling missing data (e.g., through imputation or interpolation), removing noise (e.g., using filtering techniques), and converting data into a suitable format for the chosen AI model (e.g., normalization or standardization). Data preprocessing is crucial for ensuring the quality and consistency of the data used to train and evaluate AI algorithms.
AI Algorithms
AI algorithms analyze the preprocessed data to identify patterns, predict failures, and provide actionable insights. The choice of AI algorithm depends on the specific application and the characteristics of the data.
Commonly used algorithms include regression models (for predicting continuous values like remaining useful life), classification models (for predicting discrete events like failure or no failure), and clustering algorithms (for grouping similar equipment or identifying anomalies). The performance of these algorithms is evaluated using appropriate metrics, such as accuracy, precision, and recall.
Decision-Making Modules
Decision-making modules translate the insights generated by AI algorithms into actionable recommendations for maintenance personnel. These modules bridge the gap between raw data analysis and practical maintenance actions.
They may incorporate rules-based logic (e.g., if the predicted probability of failure exceeds a certain threshold, trigger a maintenance alert), optimization algorithms (e.g., to determine the optimal maintenance schedule based on resource availability and risk levels), and human expertise (e.g., to validate recommendations and incorporate domain knowledge).
The output of these modules is clear and concise guidance for maintenance teams.
Conclusion
AI-powered predictive maintenance is reshaping industries by offering a transformative approach to managing equipment reliability, optimizing operations, and driving cost savings. By harnessing the power of AI, organizations can move from reactive and scheduled maintenance to proactive strategies that predict failures before they occur, resulting in fewer disruptions and increased productivity. By integrating AI with the Industrial Internet of Things (IIoT), real-time sensor data, and advanced analytics, organizations can make more informed decisions, maximize asset life, and significantly improve resource allocation.
As AI technologies continue to evolve, predictive maintenance will only become more sophisticated, providing real-time, data-driven insights that improve safety and minimize operational risk. From manufacturing plants to energy grids to healthcare facilities, the potential for AI to optimize maintenance practices is vast, ensuring that industries can remain agile, efficient, and competitive in an increasingly digital world. The future of AI-powered predictive maintenance isn't just about improving operations - it's about reshaping how organizations approach sustainability, reliability, and long-term success.