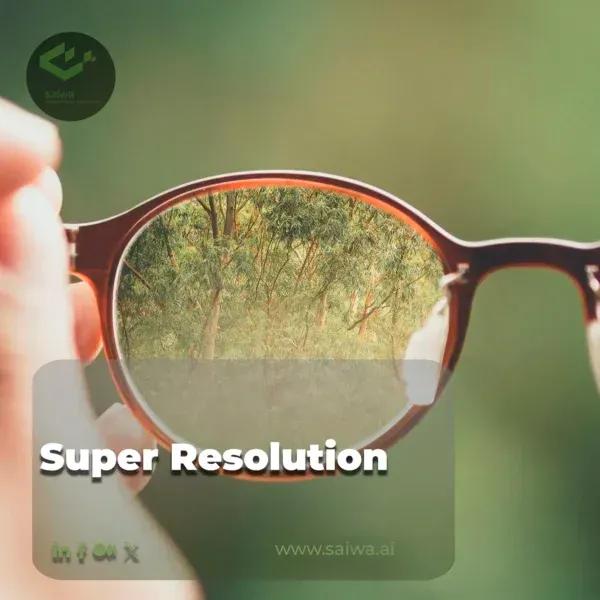
A Quick Overview of image super resolution
A group of techniques for enhancing resolution of images or videos is referred to super-. In image processing or video editing, terms like "upscale," "upsize," "up-convert," and "uprez" are also used to indicate an increase in resolution. In this study, we try to tackle the notion of Super Resolution online and investigate its different aspects.
What is Super Resolution?
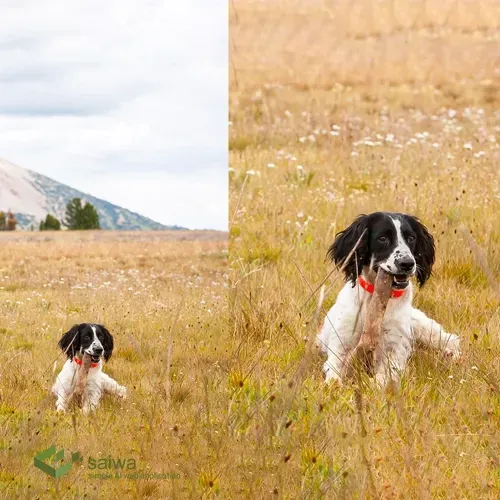
Low-resolution (LR) images contain a low number of pixels representing an object of interest, making it hard to make out the details. This can be either because the image itself is small or because an object is far away from the camera, consequently causing it to occupy a small area within the image.
Super Resolution (SR) is a branch of Artificial Intelligence as a service (AIaaS) that aims to tackle this problem, whereby a given LR image can be upscaled to retrieve an image with higher resolution and thus more discernible details that can then be used in downstream tasks such as object classification, face recognition, and so on.
Sources of LR images include cameras that may output low-quality images, such as mobile phones and surveillance cameras. In other words, Super Resolution is an image modification technology that increases image quality and retrieves high-resolution images from low-resolution ones. It helps us to erase the compression artifacts and turn the blurred images into sharper ones by altering the pixels.
How does Super Resolution work?
When many low-resolution images of the same object have slightly varied perspectives, super-resolution (SR) can be used to a good advantage. Therefore, more information about the item is available overall than in just one frame.
The ideal scenario occurs when an object moves in the video. Then, to improve upscaling, motion detection and tracking are utilized. No further data may be gathered if an item remains in all frames and doesn't move. Also, if an item transforms or travels too quickly, it will appear quite different in each structure, making it difficult to reconstruct the other frames using data from the first.
What are some types of super Resolution?
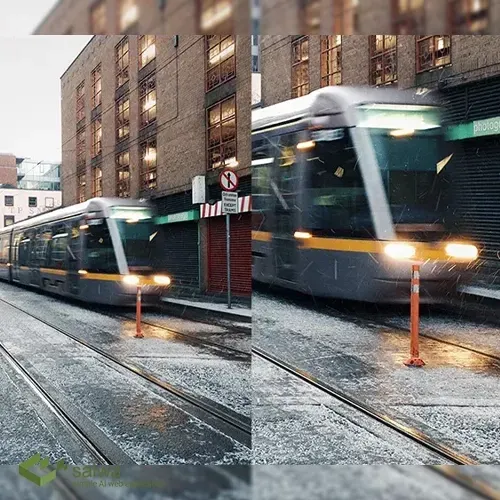
There are several varieties of Super Resolution, including the following:
Image Super Resolution
Depending on the quantity of input data available, there are two different types of Image SR approaches. In single-image SR, there is just one low-resolution picture accessible, and it must be mapped to its high-resolution equivalent. In multi-image SR, several LR photos of the same scene or object are accessible, and all of these images are utilized to map to a single HR image.
Video Super Resolution
Upscaling a video from a low resolution to a high resolution is known as video super-resolution.
What are the applications of super resolution?
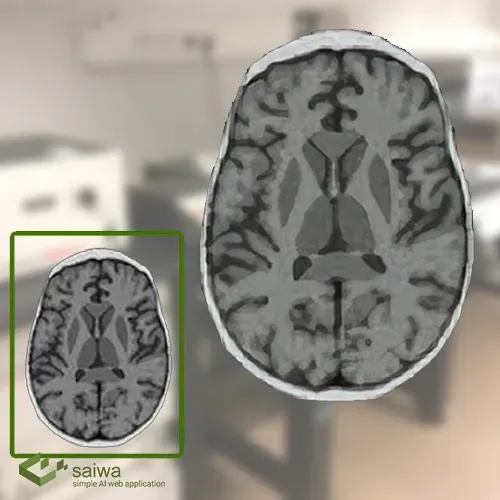
Applications for Super-Resolution include the following:
Surveillance: using low-resolution images from security cameras to spot, identify, and perform facial recognition.
Medical: Due to scan time, spatial coverage, and signal-to-noise ratio (SNR) issues, obtaining high-resolution MRI images can be challenging. By producing high-resolution MRI from otherwise low-quality MRI images, super-resolution aids in solving this problem.
Media: By sending media at a lower resolution and upscaling footage when it's received, super-resolution can help cut server expenses.
Satellite Image Processing: The areas of image rectification, restoration, enhancement, and information extraction are included in the processing of satellite images. These areas frequently require the use of super-resolution technology.
The Techniques of super resolution
Super-Resolution (SR) uses a variety of techniques. Some of those are as follow:
Pre-Upsampling Super Resolution
These techniques use traditional methods like bicubic interpolation and deep learning as a service to improve an upsampled image. SRCNN, the most widely used method, was the first to employ deep learning and has produced exceptional results.
Post-Upsampling Super Resolution
Since the feature extraction process in pre-upsampling SR occurs in the high-resolution space, the computational power required is also on the higher end. Post-upsampling SR tries to solve this by doing feature extraction in the lower resolution space, then doing upsampling only at the end, therefore significantly reducing computation.
Also, instead of using simple bicubic interpolation for upsampling, a learned upsampling in the form of deconvolution/sub-pixel convolution is used, thus making the network trainable end-to-end.
Multi-Stage Residual Networks
Many architectures are taking into consideration a multi-stage design to deal with the task of feature extraction independently in the low-resolution space and the high-resolution space to increase their performance. While the next step enhances it, the initial stage predicts the coarse traits.
Recursive Networks
Convolutional layers in recursive networks use shared network parameters to reduce memory footprint.
Multi-Branch Networks
The difficulty of training deeper networks results from the information flow issue. Shortcut connections are used in residual networks to some extent to address this. By having multiple branches through which information can pass, multi-branch networks improve information flow by combining data from various receptive fields, which leads to better training.
Attention-Based Networks
All spatial locations and channels are given equal importance in the networks that have been explored so far. In general, selectively focusing on specific areas of an image can produce far superior outcomes.
Generative Models
Generative models, often known as GANs, aim to enhance perceptual quality to create pleasing-to-the-eye images.
The frameworks of super resolution
Super-Resolution (SR) techniques typically rely on two key algorithms: motion compensation for locating matching regions in neighboring frames and high-quality spatial (in-frame) upscaling. Numerous real-world applications of super-resolution software double the resolution of the original content. SR is often used twice when we need to upsize anything four times (this can be done internally in implementation).
Super Resolution based on Deep Learning
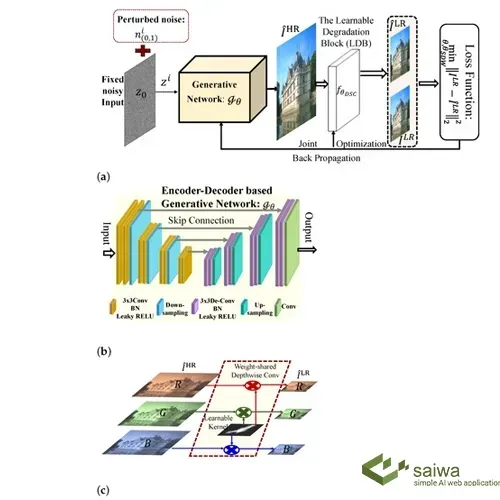
Several Deep Learning-based models, some of which were ground-breaking at the time and served as stepping stones for further research in SR technology, have been brought out over the years to solve the SR challenge. Some of those are as follow:
SRCNN
SRCNN is a fully convolutional network, and the model's main goals were to have a straightforward design and rapid processing times.
SRGAN
As it was the first technique to super-resolve photo-realistic natural images at 4x upsampling, the SRGAN model was a breakthrough in the literature on SR.
ESPCN
ESPCN is a technique of SR, where the final layer of the network performs upscaling, and super-resolution of the LR input to the HR image is accomplished via LR feature maps.
SwinIR
SwinIR is a recently developed image reconstruction technique that uses the well-known Swin Transformer network, which combines the benefits of both Transformers and convolutional neural networks (CNNs).
RRDN
Residual in Residual Dense Networks (RRDN) effectively utilizes residual dense blocks for Super resolution. Residual Dense Network RRDN employs a residual-in-residual structure, in which residual learning is applied at multiple levels and, similarly to RDN, in the primary network path, where network capacity increases due to dense connections.