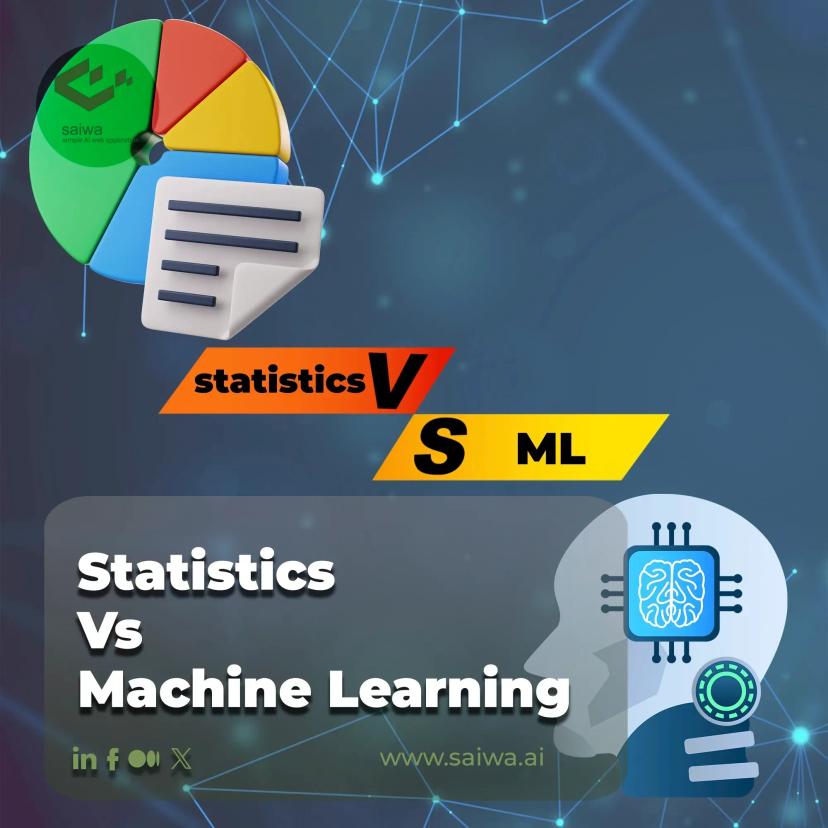
Statistics Vs Machine Learning | Key Differences
Machine learning has rapidly risen to prominence in many industries in recent years due to its unprecedented ability to find patterns and make predictions from complex data. However, machine learning did not emerge in isolation. It relies heavily on concepts and methods originally developed in the more established field of statistics. This begs the question, how exactly do these two quantitative sciences differ, and in what ways are they similar or complementary?
In this blog post, we explore the key features that distinguish statistics vs machine learning, as well as their common strengths. We discuss the appropriate context for each approach and the possibilities for hybrid methods. Statistics vs machine learning offer overlapping but distinct toolsets for extracting insights from data. Understanding their interrelationships is key to determining the most appropriate techniques for a given analytical task.
What are Statistics?
Statistics is the branch of science that focuses on the collection, analysis, interpretation, and presentation of quantitative data. It provides a mathematical framework for making inferences about populations based on sample data. Statistics uses techniques such as descriptive statistics, statistical modeling, regression analysis, hypothesis testing, and experimental design to derive knowledge from empirical observations.
Key aspects of statistics include quantifying variability in data, characterizing uncertainty, and identifying significant relationships among variables. It enables the extraction of signals from noise and the making of data-driven decisions under uncertain conditions. Statistics plays a vital role in many scientific fields, including medicine, social sciences, biology, and physics, as well as public policy, sports analysis, finance, and more.
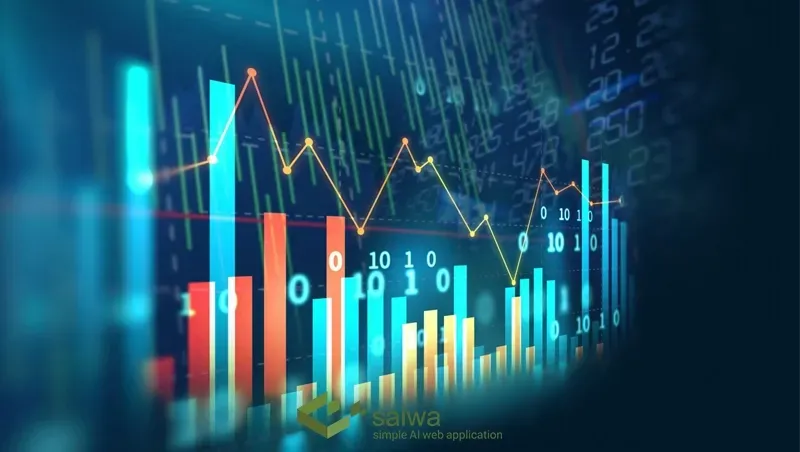
What is Machine Learning?
Machine learning is a subfield of artificial intelligence focused on developing algorithms that can learn and improve tasks through exposure to data without explicit programming. It employs sophisticated statistical modeling and optimization techniques to "train" systems to recognize complex patterns and make predictions by generalizing from examples.
Machine learning powers modern applications from image and speech recognition to natural language processing, robotics, and self-driving cars. Popular techniques include deep neural networks, decision trees, support vector machines, k-nearest neighbors, and ensemble methods. Key differentiators of machine learning include its emphasis on predictive accuracy over model interpretability and its ability to handle multivariate, non-linear, and high-dimensional data relationships.
Read Also: What Is Machine Learning as a Service?
What is the Relationship Between Statistics vs Machine Learning?
While differing philosophically in some regards, statistics vs machine learning share a deep connection. Machine learning has its roots in statistical modeling and leverages many techniques like regression originally developed in statistics. Statistical learning theory provides a framework for understanding machine learning algorithms.
At the same time, machine learning has expanded the boundaries of statistical modeling into areas involving massive datasets, complex feature spaces, and flexible nonlinear models like neural networks. Many consider machine learning a subfield of statistics focused on prediction rather than inference. Others view them as sibling disciplines under a broader data science umbrella.
Regardless of semantics, the two communities share much common ground, and the advances in each field inform the other. Concepts like controlling overfitting and properly validating models are relevant across both disciplines. The interplay between modern machine learning and traditional statistics is a major source of innovation in modern data analysis.
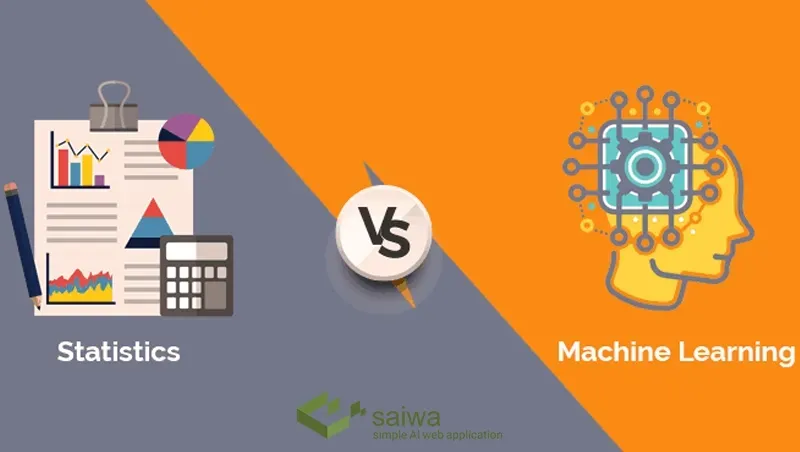
What are the Main Differences Between Statistics and Machine Learning?
There are several key aspects that distinguish statistics vs machine learning:
Goals:
Statistics emphasizes inference, assessing the value and uncertainty of model parameters based on input data. Machine learning focuses more directly on predicting outcomes from new data points.
Interpretability:
Statistical models are designed to be interpretable, with directly understandable parameters. Machine learning models tend to act as black boxes focused solely on predictive accuracy.
Flexibility:
Machine learning models place few restrictions on the mathematical form of relationships uncovered in data. Statistical modeling makes more assumptions about the basic process of generating data.
Overfitting:
Statistics favors parsimony in models, avoiding overfitting to ensure generalizability. Machine learning often opts for higher complexity if it improves fit.
Causality:
Statistical modeling represents causal factors and effects. Machine learning reveals associations but not the direction or mechanism.
In general, statistics takes a more rigid, process-driven modeling approach, while machine learning is more flexible and data-driven.
Statistics vs Machine Learning in the Real World
The complementary strengths of statistics and machine learning align with certain real-world use cases:
Statistics excels in domains like clinical trials or social science experiments where quantifying uncertainty and controlling variables to infer causality is critical. Statistical modeling is essential for confirming drug efficacy or policy impacts.
Machine learning dominates applications like image classification, speech recognition, and language translation. Its flexibility handles the enormous feature spaces and Dataset sizes involved better than traditional statistical approaches.
Problems involving both structured experimental data and multivariate unstructured data benefit from hybrid modeling combining statistical and machine learning techniques. This leverages their respective strengths.
As a rule of thumb, machine learning serves situations focused on optimization and accurate prediction, while statistics aids contexts centered on explanation and causal understanding.
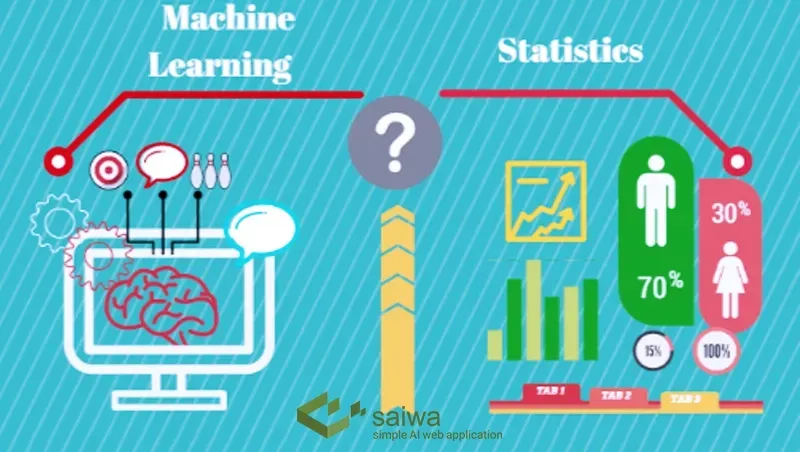
Future Outlook
In this article, we made a comprehensive guide for statistics vs machine learning. As data generation continues accelerating, the power of machine learning will likely grow, driven by computational advances. However, this may require tradeoffs around interpretability as models become more complex. On the other hand, increased model transparency and explainability will remain important priorities for responsible adoption across domains like healthcare, where trust is paramount. Further convergence of statistics and machine learning through cross-disciplinary collaboration appears certain, given their highly complementary nature.
Conclusion
While statistics and machine learning embody different philosophies and assumptions, they have a strong symbiotic relationship. Machine learning adds nonlinear modeling capabilities to the classical statistical toolbox. Statistics provides machine learning with rigor for dealing with uncertainty and overfitting. Integrating their complementary toolsets provides a versatile framework for data analysis that spans inference, prediction, description, and optimization. Cross-disciplinary skills in statistics vs machine learning are increasingly valuable in our data-rich world.