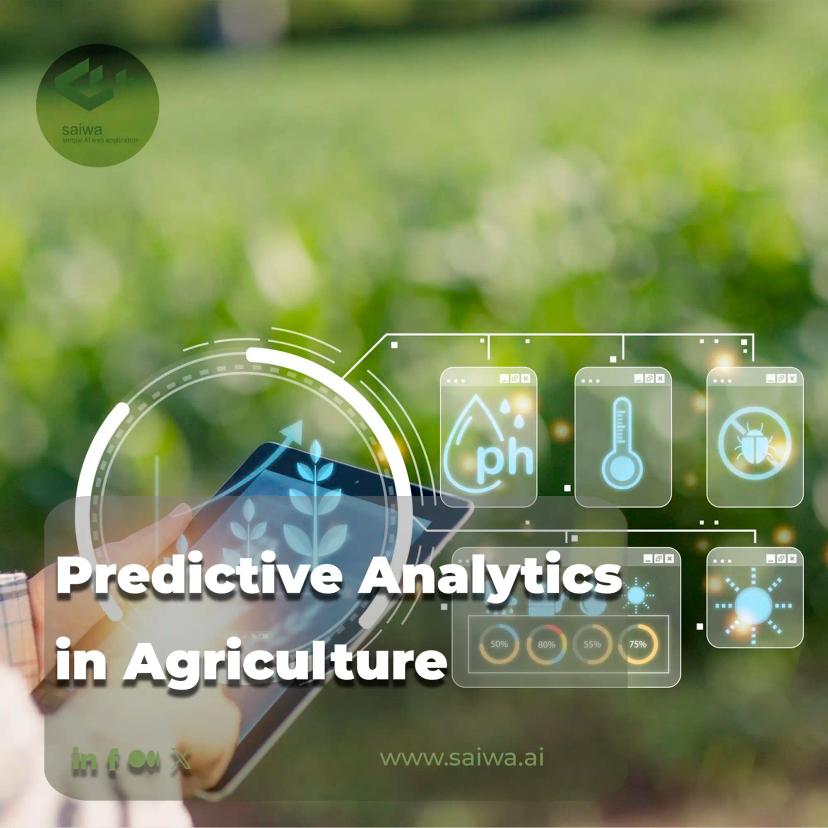
Predictive Analytics in Agriculture | Fields of Insight
Predictive analytics refers to statistical and machine learning techniques that analyze current and historical data to make predictions about future events and conditions. Predictive analytics in agriculture holds immense potential for informing smarter decisions and driving efficiency, productivity, and sustainability improvements. With the increasing availability of agricultural data from sources like sensors, imagery, and equipment, paired with more advanced algorithms, predictive analytics is poised to transform farming. However, thoughtfully implementing predictive tools within complex growing environments and established practices remains an ongoing challenge requiring both technical and social innovation.
Read Also: The impact of machine learning in agriculture
The Growing Role of Agricultural Data
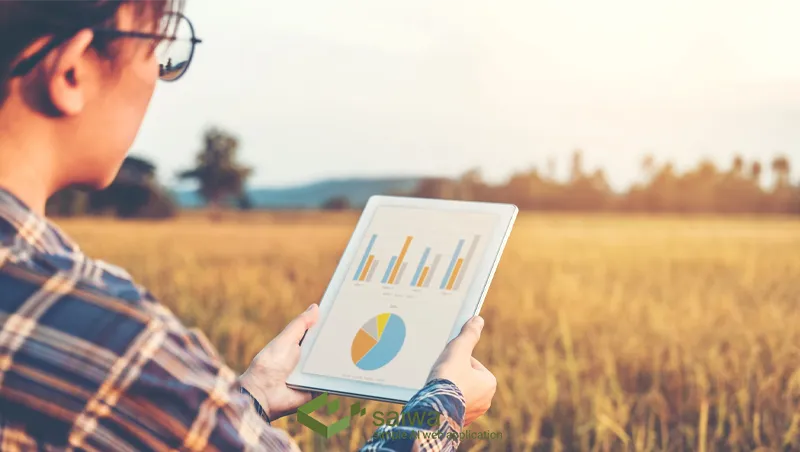
Advanced predictive analytics in agriculture rely on large, high-quality data sets. In agriculture, relevant data is increasingly abundant, including data from planting/harvesting equipment, soil sensor measurements, weather and climate records, satellite imagery, and aerial imagery captured by AI drones. However, effectively managing and preparing multimodal data for modeling requires significant effort. Real-world data require cleaning and preprocessing to handle missing values, redundancies, and inconsistencies before analysis. Domain knowledge also informs useful derived variables and data representations to best capture key agricultural factors.
Predictive Modeling Techniques for Agriculture
Time series models such as ARIMA and Prophet capture seasonal patterns and meteorological dependencies in crop yield data. Regression and random forest models incorporate factors such as soil properties, fertilizer inputs, and irrigation to estimate yield outcomes. Recurrent neural networks learn complex temporal dependencies from multidimensional time series data. Physics-based neural networks encode domain knowledge into model architectures and loss functions for improved generalization. Ensemble approaches aggregate disparate models to reduce bias and variance.
However, challenges remain in representing complex agricultural phenomena such as weather, biology, and equipment reliability in tractable modeling paradigms. Complex deep-learning models also face transparency and debugging issues. Hybrid techniques that combine mechanistic and data-driven components are often the most effective, balancing tractability with flexibility. Overall, multivariate modeling, uncertainty quantification, and model ensembling help overcome the inherent variability of agriculture.
Key Application Areas
Various applications of predictive analytics in agriculture can provide many benefits in the agriculture industry for a more optimized and productive food chain.
Crop Yield Predictions
Crop yield predictions guide inventory planning, logistics, and export volumes. Within-season forecasts at higher granularity inform supplemental irrigation, fertilizer, and pesticides to optimize outcomes. Modeling by crop variety and region enables insights at appropriate scales.
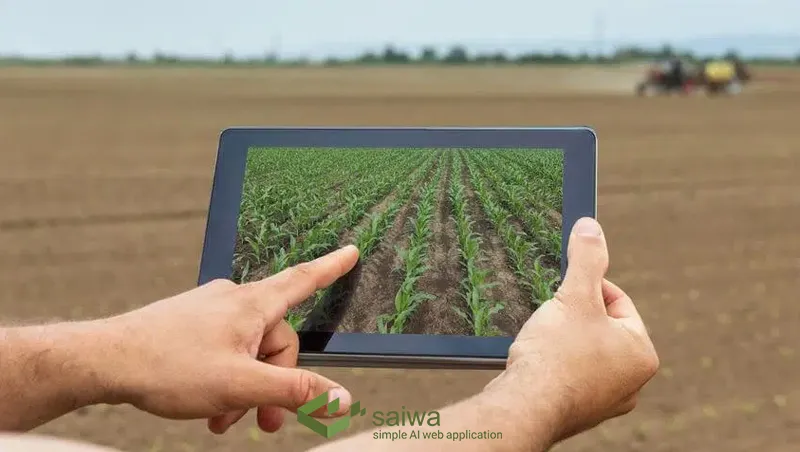
Crop Health Insights
Crop health insights from aerial imagery fuel early disease and infestation detection allowing rapid, targeted response before widespread impact. Computer vision techniques can classify crop stress and nominate affected areas for ground inspection. This shifts disease management from reaction to proactive prevention.
Predictive Maintenance Analytics
Predictive maintenance analytics on telemetry from pumps, vehicles, and other farm equipment reduces downtime through prompt repairs before total failure. Optimization algorithms schedule maintenance to minimize disruptions during critical seasons. This improves equipment reliability and availability.
Optimized Field Treatment
Optimized field treatment prescription maps for fertilizers, pesticides, and irrigation help reduce resource usage and environmental impact while maintaining yields. Prescriptions based on predictive crop needs analysis enable precise efficient application.
Read Also: Practical Application & Future of AI in Agriculture
Generating Actionable Insights
Predictions should be packaged with operational recommendations to enable informed actions. For example, yield forecast reports could include specific suggestions on procuring transportation, storage, and marketing based on the quantified predictions. Crop disease alerts can prescribe appropriate fungicides or insecticides along with application maps. This decision support bridges the gap between raw predictions and field actions.
User experience design is crucial for adoption. Farmer-centric visualizations, notifications, and intuitive interfaces facilitate seamless workflow integration and build trust in model outputs. Interactive tools also support collaborative analysis, customization, and sharing of insights within farmer communities. Respecting end-user contexts maximizes the likelihood of impact.
The Multifaceted Benefits of Predictive Analytics in Agriculture
The rise of data-driven predictive analytics presents agriculture with immense opportunities to enhance productivity, efficiency, sustainability, and resilience against increasing climate volatility. Predictive insights enable proactive agile management as opposed to reactive approaches.
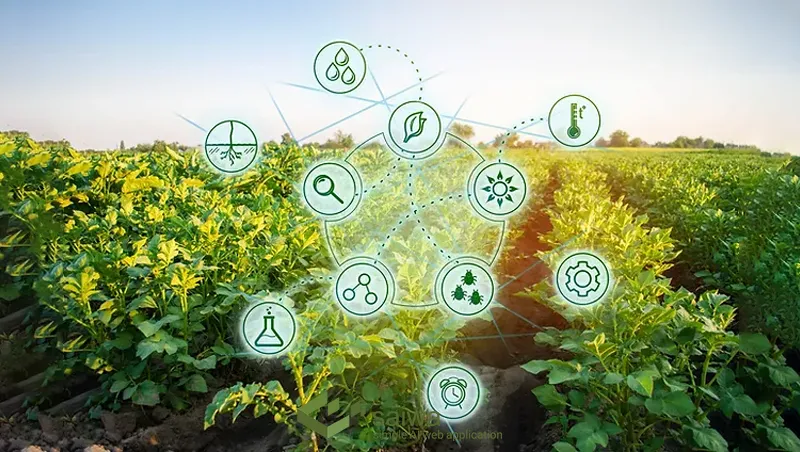
forecasting crop yields
One major benefit is forecasting crop yields months or weeks ahead of harvest with increasing granularity. This allows for optimizing plans around storage, transportation, marketing, and export volumes. During the growing season, yield forecast updates at finer farm-level resolution help guide supplemental irrigation, fertilizers, and pesticides to correct any shortfalls predicted. The precision-optimized application reduces unnecessary wastage and environmental impacts.
Automated predictive crop health
Automated predictive crop health analytics powered by aerial imagery enable early detection of disease outbreaks and infestations before they escalate into devastating losses. This shifts disease management from reaction to prevention. Prompt identification allows the deployment of targeted suppression measures while infections remain localized. Forewarned of potential issues, farms are also better positioned to secure crop insurance and financing contingencies if required.
Predictive maintenance analytics
Predictive maintenance analytics prevent operational disruptions by flagging impending equipment failures based on telemetry monitoring and scheduling preventive repairs. Optimized maintenance routines minimize disruption during critical harvest windows. Improved uptime through predictive maintenance increases farm productivity and profitability.
Intelligent allocation of resources such as irrigation water, fertilizer, pesticides, and labor optimizes usage and reduces waste based on predictive crop demand forecasts. Prescriptive application maps guide variable rate equipment. The overall optimized use of resources increases yields while reducing costs and environmental impact.
In summary, predictive analytics can help transform agriculture into a more efficient, productive, and sustainable data-driven managed ecosystem. Timely, actionable insights improve decision-making in the face of increasing variability and demands. However, careful, ongoing integration of these tools into existing practices and workflows remains critical to realizing the broad potential benefits.
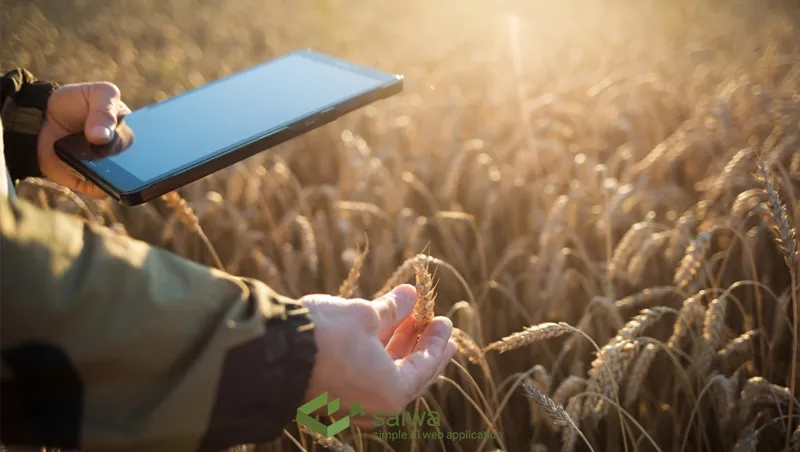
Adoption Challenges
Significant upfront costs for sensors, connectivity, and analytics inhibit adoption by small farms, although cooperatives can pool resources. At the enterprise scale, change management is key as predictive approaches disrupt traditional practices and roles. Without participatory development, tools may fail due to a lack of relevance or mistrust.
On the technical side, data sets spanning different geographies, crops, climates, and equipment are needed to train robust models. High-quality labeled data for model monitoring remains scarce. Agricultural expertise is as important as statistical knowledge to properly structure problems. Democratizing these skills for users without a data science background remains a challenge.
The Future of Predictive Analytics in Agriculture
As research continues to advance techniques such as computer vision, IoT integration, and hybrid AI, predictive insights will become increasingly accurate and pervasive. Cloud platforms are also democratizing access to advanced analytics. However, thoughtful contextualization of these tools through participatory development and inclusive data practices will remain essential to translating predictions into positive impact. Overall, when used responsibly, data-driven intelligence can help address growing agricultural challenges.
Conclusion
The integration of predictive analytics in agriculture has tremendous potential to increase production efficiency, sustainability, and resilience in the face of escalating food demand and climate volatility. However, technological innovation alone is not enough. Collaborative development frameworks that center on the needs and perspectives of farmers are essential to smoothly integrate predictive capabilities into existing practices and workflows. When pursued holistically, data-driven smart agriculture can help feed communities into the future.