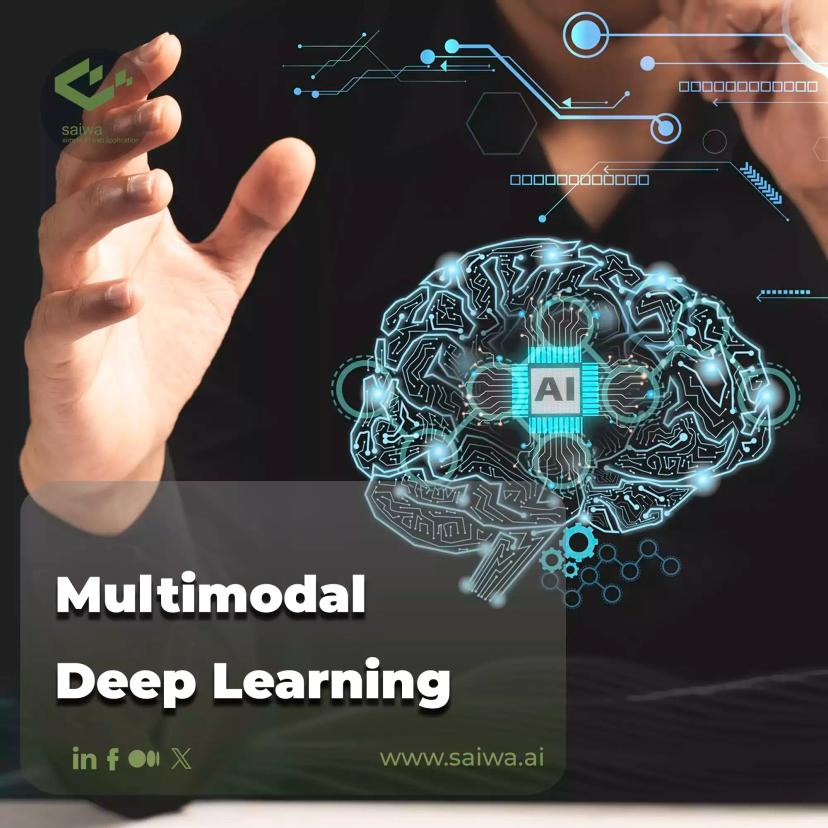
Revolutionizing AI: The Multimodal Deep Learning Paradigm
In recent years, the field of machine learning has made significant strides in various challenges, including image recognition, natural language processing, and others. Nonetheless, several models work on single-modal data, such as images, text, or speech. Conversely, real-world data is typically obtained from multiple sources like images and text, video and audio, or sensor data. However, real-world experiences involve consistent simultaneous exposure across multiple sensory modalities decoding seamless informational inputs and advancing contextual awareness dynamically. Multimodal deep learning has been developed by scientists to overcome challenges and create innovative opportunities in intelligent systems.
This article aims to explore the current fusion techniques used and examine the possible challenges and prospects of Multimodal deep learning.
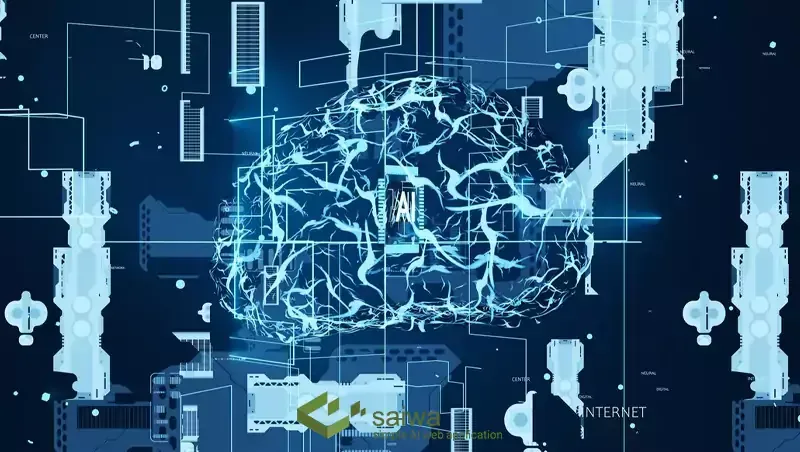
What is Multimodal deep learning?
Multimodal deep learning integrates and analyzes data from different modalities including text, images, video, audio, and sensor data. By combining various methods, it creates a complete representation of the data, leading to improved performance in various machine-learning tasks.
Traditionally, machine learning models were designed to operate on data from a single method, e.g. image classification or speech recognition. However, real-world data is typically derived from multiple sources and methods, rendering analysis more complex and challenging. Multimodal deep learning aims to surmount these issues by incorporating information from different methods to generate models that are more precise and informative.
Read Also: What Is Deep Learning as a Service|Why Does It Matter?
The goal of multifaceted deep learning
Known as multimodal deep learning, architecting model architectures effectively assimilating visual, verbal, and acoustic datasets mutually reinforces robust contextual decision-making nearly matching multifaceted human information processing abilities in applications like generating conversational assistants, self-driving vehicle navigation, or medical diagnostics benefiting doctors. While Multifaceted Deep Learning navigates the intricacies of diverse data facets, Multimodal Deep Learning seamlessly integrates information from multiple sensory modalities.
Read Also: Find the Impact of AI in Self Driving Cars with our guide
Multimodal deep learning aims to develop a joint representation space that can effectively integrate complementary information from various modalities: used image, speech recognition, and natural language processing.
Multifaceted deep learning models usually consist of many neural networks, each of which is an expert in analyzing a specific method. Then the output of these networks is processed using different fusion techniques, such as early fusion, late fusion, or hybrid fusion combined to create a common representation of the data.
Basic fusion involves combining raw data into a single input vector through different methods and feeding it into the network. In contrast, late fusion requires training separate networks for each modality before combining their outputs in the following step. Hybrid fusion combines both early and late fusion elements, resulting in a more flexible and adaptable model.
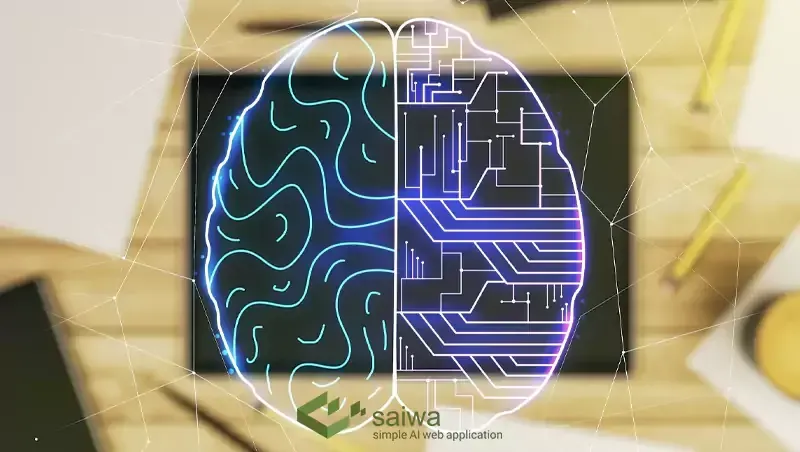
How multimodal learning works
Multimodal deep learning models typically include several unimodal neural networks that separately process each input modality. For instance, an audio-visual model may have two unimodal networks, one for audio and one for visual data. This separate processing is known as encoding.
When unimodal encoding occurs, information taken from each modality should be integrated or combined. Combining multivariate data is crucial to the success of these models. Finally, a decision network accepts the combined encoded information and is trained for the task at hand.
Multimodal architecture generally comprises three components:
Unimodal encoders, which encode each input modality individually, usually with one for each modality.
A fusion network, which combines the features extracted during the encoding phase from each input modality.
A classifier that accepts the fused data and produces predictions.
Encoding Stage
The encoder takes data inputs from each modality and converts them into a common representation that subsequent layers in the model can process. It consists of multiple neural network layers that apply nonlinear transformations to extract abstract features from the input data.
Encoder input may contain data from various modalities such as images, audio, and text, which are typically processed independently. Each modality has its encoder that converts the input data into a set of feature vectors, and subsequently, the output of each encoder is merged into a unified representation that accumulates important information from every modality.
A popular method of merging individual encoder outputs is to concatenate them into a vector. Alternatively, attention mechanisms can be utilized to assess each method's contribution based on its relevance to the correct task.
The encoder aims to capture the underlying structure and relationships between the input data in numerous ways, allowing the model to produce more precise predictions or create new outputs based on this multimodal input.
Fusion Module
The Fusion Module integrates data from various modes into a solitary representation that can be applied to downstream tasks, such as classification, regression, or generation. Fusion Modules come in diverse forms that rely on the specific architecture and desired assignment.
One common approach is to calculate the weighted sum of method features, with weights learned during training. Another option involves connecting modality features and passing them through a neural network to learn a joint representation.
In some cases, attention mechanisms can be used to learn which modality should be attended to at each time step.
Beyond the specific implementation, the Fusion Module aims to capture complementary information from different methods and create a more robust and informative representation for downstream work. This is especially important in applications such as video analysis where combining visual and audio cues can improve performance.
Classification
The classification module utilizes the combined representation generated by the fusion module to make decisions and predictions. The architecture and approach employed by the classification module depend on the task and data type being processed.
Generally, the classification module involves a neural network, where the combined representation undergoes fully connected layer(s) before final prediction. Nonlinear smoothing functions, dropouts, and other techniques can be included in these layers to prevent overfitting and improve generalization performance.
The output of the classification module depends on the specific task at hand. For example, in the multimodal sentiment analysis process, the output will be a binary decision that indicates whether the text and image input is positive or negative. In a multimodal subtitling task, the output can be a sentence that describes the content of the image.
The classification module is typically trained using supervised learning, where input methods and corresponding labels are used to optimize model parameters. This optimization is commonly achieved through gradient-based methods like random gradient descent or variations.
In multimodal deep learning, the classification module is crucial as it utilizes the joint representation generated by the fusion module to make informed predictions or decisions.
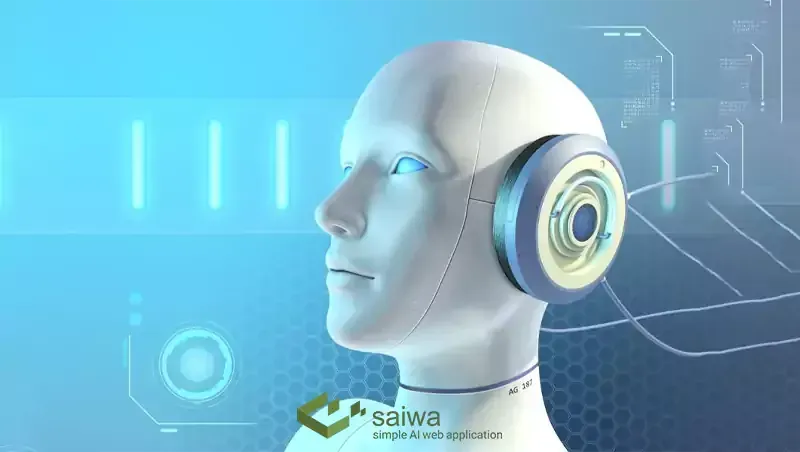
Applications of Multimodal deep learning
In this section, we will discuss some fields in which multimodal learning is used:
Creating descriptive captions for images.
Visual question answering in interactive artificial intelligence systems gives users the ability to ask questions about images and receive relevant answers from the model.
Analyzing medical images in the healthcare industry, enabling accurate diagnosis and treatment planning by combining medical image data such as MRI, CT scan, and X-ray with patient records.
In Various fields, including human-computer interaction, marketing, and mental health, it enables the detection of emotions through the analysis of facial expressions, voice tones, and textual content.
Conclusion
Multimodal Deep Learning shows promise in AI models by utilizing different methods to convey improved information, performance, and application possibilities. It is a thriving area of research in various fields. Exponential progress made in deriving image and language understanding through deep neural networks recently paves the way for pursuing multimodal architecture fusion opportunities unlocking next-generation tools approximating human cognition efficiencies processing views, conversations, and sounds concert emphatically. While the journey travels far scaling mountaintops still, perseverant research community momentum gains daily suggest breaching pinnacles matching multifaceted human intelligence capacities could emerge sooner than previously envisioned.