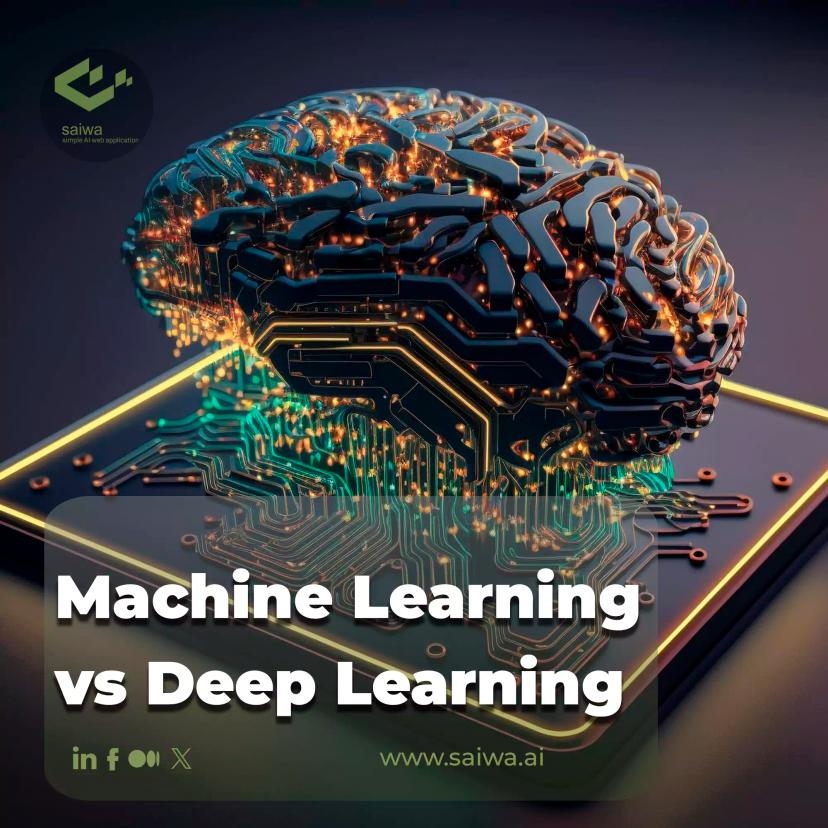
Machine Learning vs Deep Learning | What's the Difference?
This article discusses the idea of two well-liked sub-categories of Artificial Intelligence, i.e., Machine Learning vs Deep Learning. Additionally, to compare ML and DL, we provide background information about each one before attempting to contrast the specific characteristics of both. We also try to envision AI's future.
What is Machine Learning?
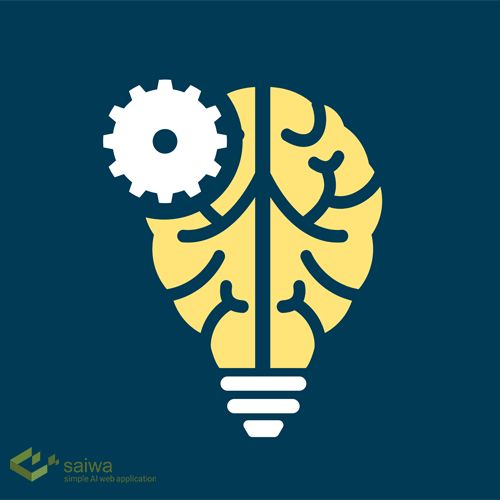
Machine learning is an Artificial intelligence (AI) technique that teaches computers to learn from experience. Machine learning algorithms employ computational techniques to "learn" information directly from data without using a pre-existing equation as a model. The algorithms adjust to their performance as more examples are available for learning. Machine learning aims as a service to create computer systems that can access and utilize data to acquire knowledge on their own. Machine learning is a field that studies the research and creation of algorithms that can learn from and predict data. Because it can solve issues at a pace and scale that cannot be matched by the human intellect alone, ML is advantageous. Machines may be trained to recognize patterns and relationships between incoming data by putting large amounts of processing power behind single or several focused activities. This allows devices to automate repetitive operations.
What is Deep Learning?
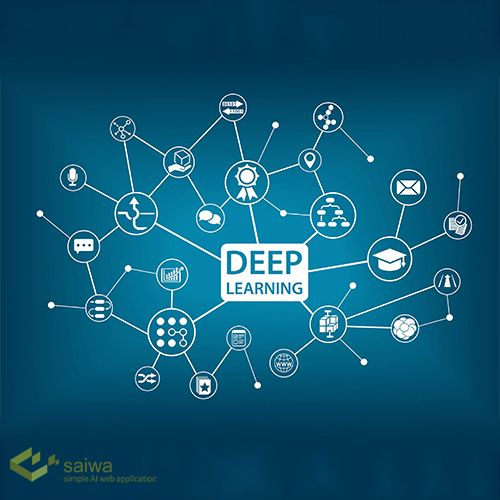
Deep learning is a particular type of machine learning that trains algorithms to perform tasks people accomplish without thinking about them. A computer model learns to carry out categorization tasks directly from pictures, text, or voice using deep learning. High precision can be attained by deep learning models that sometimes even outperform human ability. A sizable collection of labeled data and multi-layered neural network architectures are used to train models. Recently, deep learning has attracted a lot of interest, and for a good reason. It is producing outcomes that were formerly unattainable.
How does Machine Learning work?
Machine learning relies on input, such as training data or knowledge graphs, to comprehend things, domains, and their relationships, similar to how the human brain acquires information and comprehends. Entities must be established before machine learning can start. The first step in machine learning is observing data, such as examples, first-hand knowledge, or instructions. It searches for patterns in the data to subsequently conclude the given instances. The main goal of ML is to make it possible for computers to learn independently, without human aid, and to adapt their behavior accordingly.
How does Deep Learning Work?
Deep learning techniques are sometimes called "deep neural networks" since they frequently use neural network structures. The number of a neural network's hidden layers is typically indicated by the word "deep". There may be tens or even hundreds of hidden layers in a network. Large datasets of labeled data and neural network layouts that automatically extract features while learning directly from the data are used to train deep learning models. Convolutional neural networks (CNN or ConvNet) are among the most commonly used varieties of deep neural networks. A CNN employs 2D convolutional layers and combines learned features with input data, making it an excellent architecture for processing 2D data, including images. You do not need to know the components used to categorize pictures because CNNs perform manual feature extraction. Direct feature extraction from images is how CNN operates. The relevant characteristics are not pre-trained; they are discovered when the network is trained on a set of images. Due to this automatic feature extraction, deep learning as a service model are highly accurate for computer vision applications like object classification.
Differences Between Machine Learning vs Deep Learning
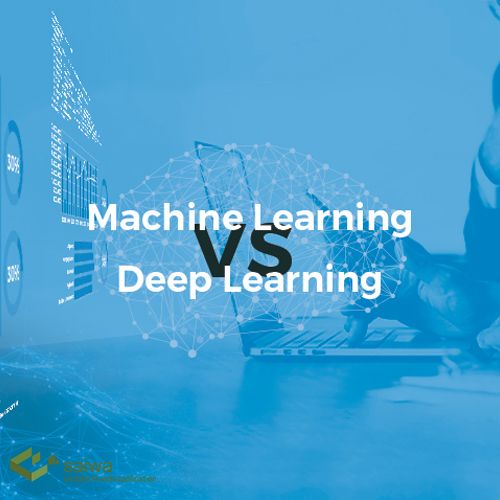
Machine learning vs deep learning are both subsets of artificial intelligence (AI) that involve training algorithms to learn from data. However, there are several key differences between the two:
Human Intervention
While Deep learning learns independently from its surroundings and prior mistakes, Machine Learning requires more human intervention to correct and learn.
Hardware
The CPU (Central Processing Unit) can be used for training in Machine learning models. While Deep learning algorithms mostly require GPUs (Graphics Processing Unit) for training.
Time
The efficiency of Machine learning systems may be limited despite their ease of setup and operation. Deep learning algorithms can deliver results immediately, although they take more training time (The quality will likely improve over time as more data becomes available). Due to the small size, the Machine learning model requires less training time. Conversely, deep learning models take a long time since the amount of data is enormous.
Approach
Deep learning uses multi-layered, complex neural networks to analyze more intricate patterns and relationships. It creates non-linear, complex correlations. Machine learning, on the other hand, involves training algorithms to recognize patterns and relationships in data and also makes simple, linear correlations.
Applications
Machine learning apps are easier to use than deep learning and can run on regular PCs. Deep learning systems have access to far more advanced hardware and resources. Regression analysis, classification, and clustering are just a few of the many uses for machine learning. On the other hand, deep understanding is typically employed for challenging tasks, including speech and image recognition, natural language processing, and autonomous systems.
The Future of Machine Learning vs Deep Learning
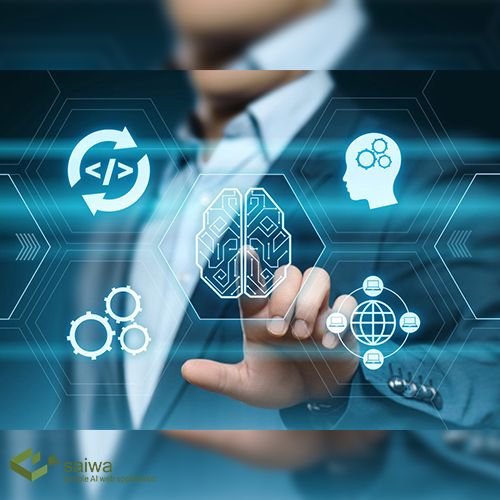
The future of Machine learning vs Deep Learning is quite intriguing. Their applications currently power practically all common domains. Healthcare, search engines, digital marketing, and education are some areas that benefit the most. The value of ML and DL to a business or organization may not be debatable since, in the future, jobs that are currently carried out manually will be entirely completed by computers. As a result of Machine Learning vs Deep Learning, the human species benefits from AI to the fullest extent possible. New computer vision and natural language processing (NLP) developments are occurring that no one could have foreseen. We encounter both daily—face recognition on our smartphones, software that translates languages, self-driving automobiles, etc. Science fiction-like concepts are becoming a reality. It isn't easy to envision a day without Machine Learning vs Deep Learning regarding how pervasive technology is in our lives.